博客地址:https://www.cnblogs.com/zylyehuo/
开发环境
- anaconda
- 集成环境:集成好了数据分析和机器学习中所需要的全部环境
- 安装目录不可以有中文和特殊符号
- jupyter
- anaconda提供的一个基于浏览器的可视化开发工具
丢失数据的类型
两种丢失数据的区别
import pandas as pd
from pandas import DataFrame
import numpy as np
type(None)
NoneType
type(np.nan)
float
为什么在数据分析中需要用到的是浮点类型的空而不是对象类型?
- 数据分析中会常常使用某些形式的运算来处理原始数据,如果原数数据中的空值为NAN的形式,则不会干扰或者中断运算。
- NAN可以参与运算的
- None是不可以参与运算
np.nan + 1
nan
None + 1
---------------------------------------------------------------------------
TypeError Traceback (most recent call last)
<ipython-input-5-3fd8740bf8ab> in <module>
----> 1 None + 1
TypeError: unsupported operand type(s) for +: 'NoneType' and 'int'
在pandas中如果遇到了None形式的空值则pandas会将其强转成NAN的形式。
df = DataFrame(data=np.random.randint(0,100,size=(7,5)))
df.iloc[2,3] = None
df.iloc[4,2] = np.nan
df.iloc[5,4] = None
df
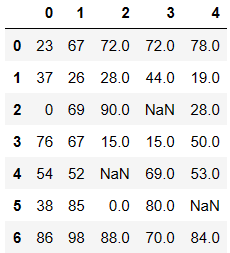
pandas处理空值操作
- isnull
- notnull
- any
- all
- dropna
- fillna
方式1:对空值进行过滤(删除空所在的行数据)
- isnull --> any
- notnull --> all
df.isnull()
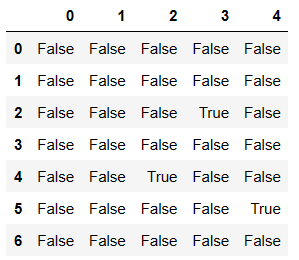
# 哪些行中有空值
# any(axis=1)检测哪些行中存有空值
df.isnull().any(axis=1) # any会作用isnull返回结果的每一行
# true对应的行就是存在缺失数据的行
0 False
1 False
2 True
3 False
4 True
5 True
6 False
dtype: bool
df.notnull()
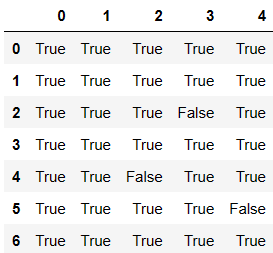
df.notnull().all(axis=1)
0 True
1 True
2 False
3 True
4 False
5 False
6 True
dtype: bool
# 将布尔值作为源数据的行索引
df.loc[df.notnull().all(axis=1)]
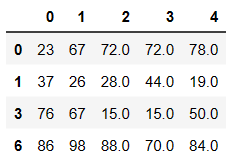
# 获取空对应的行数据
df.loc[df.isnull().any(axis=1)]
# 获取空对应行数据的行索引
indexs = df.loc[df.isnull().any(axis=1)].index
indexs
Int64Index([2, 4, 5], dtype='int64')
df.drop(labels=indexs,axis=0)
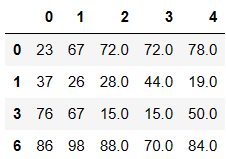
方式2:dropna 直接将缺失的行或者列进行删除
df.dropna(axis=0)
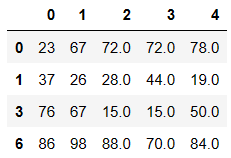
# 对缺失值进行覆盖 fillna
df.fillna(value=999) # 使用指定值将源数据中所有的空值进行填充
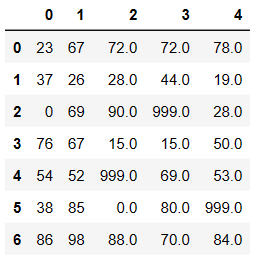
# 使用空的近邻值进行填充
# method=ffill向前填充,bfill向后填充
df.fillna(axis=0,method='bfill')
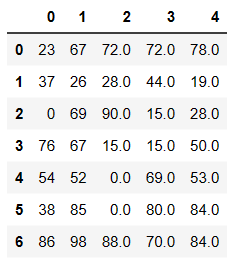
dropna和fillna的选用
- 什么时候用dropna什么时候用fillna?
- 尽量使用dropna
- 如果删除成本比较高,则使用fillna
方式3:使用空值对应列的均值进行空值填充
for col in df.columns:
# 检测哪些列中存有空值
if df[col].isnull().sum() > 0: # 说明df[col]中存有空值
mean_value = df[col].mean()
df[col] = df[col].fillna(value=mean_value)
df
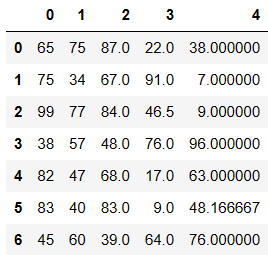
面试题
- 数据说明:
- 数据是1个冷库的温度数据,1-7对应7个温度采集设备,1分钟采集一次。
- 数据处理目标:
- 用1-4对应的4个必须设备,通过建立冷库的温度场关系模型,预估出5-7对应的数据。
- 最后每个冷库中仅需放置4个设备,取代放置7个设备。
- f(1-4) --> y(5-7)
- 数据处理过程:
- 1、原始数据中有丢帧现象,需要做预处理;
- 2、matplotlib 绘图;
- 3、建立逻辑回归模型。
- 无标准答案,按个人理解操作即可。
- 测试数据为testData.xlsx
data = pd.read_excel('./data/testData.xlsx').drop(labels=['none','none1'],axis=1)
data
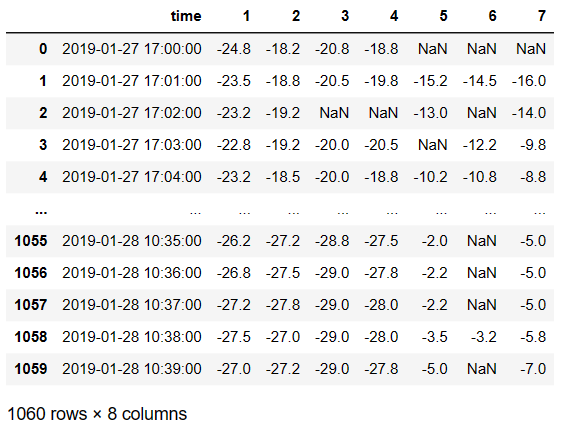
data.shape
(1060, 8)
# 删除空对应的行数据
data.dropna(axis=0).shape
(927, 8)
# 填充
data.fillna(method='ffill',axis=0).fillna(method='bfill',axis=0)
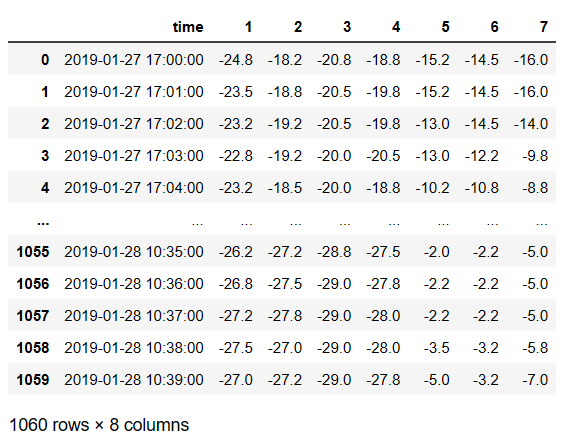