使用mnist数据集训练GAN(附pytorch代码)
准备数据
加载数据集 MNIST
from torchvision import datasets, transforms
transform = transforms.Compose([
transforms.ToTensor(), # value of pixel: [0, 255] -> [0, 1]
transforms.Normalize(mean = (0.5,), std = (0.5,)) # value of tensor: [0, 1] -> [-1, 1]
])
mnist = datasets.MNIST(root='data', train=True, download=True, transform=transform)
transforms.Normalize()
用于将图像进行标准化:\(\rm{\frac{(x - mean)}{std}}\),使得处理的数据呈正态分布。
由于 MNIST 数据集图像为灰度图只有一个通道,因此只需要设置单个通道的 mean 与 std 即可。
这里的取值,可以是将图像像素值 [0,255] 缩放至 [0, 1]后求得均值和方差,也可以是根据经验设置,即 mean=0.5, std=0.5。
查看数据
img, label = mnist[len(mnist)-500]
print(f"Label: {label}")
print(f"Some pixel values: {img[0, 10:15, 10:15]}")
print(f"Min value: {img.min()}, Max value: {img.max()}")
# Label: 3
# Some pixel values: tensor([[-0.9451, -0.6392, -0.9843, -1.0000, -1.0000],
# [-1.0000, -1.0000, -1.0000, -0.9529, -0.7725],
# [-1.0000, -0.8745, -0.0196, 0.5765, 0.7725],
# [-1.0000, 0.0902, 0.9922, 0.9922, 0.9922],
# [-1.0000, -0.3569, 0.1216, 0.1216, -0.5686]])
# Min value: -1.0, Max value: 1.0
import matplotlib.pyplot as plt
import torch
def dnorm(x:torch.Tensor):
min_value = -1
max_value = 1
out = (x - min_value) / (max_value - min_value)
return out.clamp(0,1) # plt expects values in [0,1]
img_norm = dnorm(img) # shape: (1, 28, 28)
plt.imshow(img_norm.squeeze(0), cmap='gray')
# <matplotlib.image.AxesImage at 0x187d76c7990>
制作Dataloader
from torch.utils.data import DataLoader
batch_size = 100
data_loader = DataLoader(mnist, batch_size, shuffle=True)
训练前准备
生成器/判别器定义
# model.py
import torch.nn as nn
import torch.nn.functional as F
# 判别器网络
class Discriminator(nn.Module):
def __init__(self, image_size: int, hidden_size: int):
super(Discriminator, self).__init__()
self.linear1 = nn.Linear(image_size, hidden_size)
self.linear2 = nn.Linear(hidden_size, hidden_size)
self.linear3 = nn.Linear(hidden_size, 1)
def forward(self, x):
out = self.linear1(x)
out = F.leaky_relu(out, negative_slope=0.2, inplace=True)
out = self.linear2(out)
out = F.leaky_relu(out, negative_slope=0.2, inplace=True)
out = self.linear3(out)
return F.sigmoid(out)
# 生成器网络
class Generator(nn.Module):
def __init__(self, image_size: int, latent_size: int, hidden_size: int):
super(Generator, self).__init__()
self.linear1 = nn.Linear(latent_size, hidden_size)
self.linear2 = nn.Linear(hidden_size, hidden_size)
self.linear3 = nn.Linear(hidden_size, image_size)
def forward(self, x):
out = self.linear1(x)
out = F.relu(out)
out = self.linear2(out)
out = F.relu(out)
out = self.linear3(out)
return F.tanh(out)
实例化模型并测试
from model import Generator, Discriminator
image_size = 28 * 28
hidden_size = 256
latent_size = 64
G = Generator(image_size=image_size, hidden_size=hidden_size, latent_size=latent_size)
D = Discriminator(image_size=image_size, hidden_size=hidden_size)
# 简单测试下模型
untrained_G_out = G(torch.randn(latent_size)) # Shape: [latent_size]
untrained_D_out = D(untrained_G_out.view(1, -1))
print(f"Result from Discriminator: {untrained_D_out.item():.4f}")
plt.imshow(untrained_G_out.view(28, 28).detach(), cmap='gray')
# Result from Discriminator: 0.5166
准备其他组件(优化器、损失函数等)
from torch import optim
from torch import nn
num_epochs = 300
device = "cuda:0" if torch.cuda.is_available() else "cpu"
D.to(device=device)
G.to(device=device)
d_optim = optim.Adam(D.parameters(), lr=0.002)
g_optim = optim.Adam(G.parameters(), lr=0.002)
criterion = nn.BCELoss()
d_loss_list, g_loss_list, real_score_list, fake_score_list = ([] for _ in range(4))
模型训练
定义判别器/生成器训练
分别训练判别器、生成器,但是训练其中一个时,都用到了另一个。两次训练均使用BCE loss。
- 在判别器训练中,梯度更新只更新判别器,真实数据标签为1,生成的数据标签为0,判别器输出样本得到预测结果,使用BCE计算预测与标签损失。
- 在生成器训练中,梯度更新只更新生成器,输入判别器的样本只有生成器的生成结果,标签为1(鼓励生成器生成更真实的数据)。同样使用BCE计算预测与标签损失。
# training.py
import torch
import torch.nn as nn
from torch import optim
from torch.utils.data import DataLoader
from torchvision.utils import save_image
import os
def run_discriminator_one_batch(d_net: nn.Module,
g_net: nn.Module,
batch_size: int,
latent_size: int,
images: torch.Tensor,
criterion: nn.Module,
optimizer: optim.Optimizer,
device: str):
# 定义真实样本与假样本的标签
real_labels = torch.ones(batch_size, 1).to(device)
fake_labels = torch.zeros(batch_size, 1).to(device)
# 使用真实样本训练鉴别器
outputs = d_net(images)
d_loss_real = criterion(outputs, real_labels)
real_score = outputs
# 使用生成样本训练鉴别器
z = torch.randn(batch_size, latent_size).to(device)
fake_images = g_net(z)
outputs = d_net(fake_images.detach())
d_loss_fake = criterion(outputs, fake_labels)
fake_score = outputs
d_loss = d_loss_real + d_loss_fake # 计算总损失
d_loss.backward() # 反向传播
optimizer.step() # 更新参数
optimizer.zero_grad() # 清空梯度
return d_loss, real_score, fake_score
def run_generator_one_batch(d_net: nn.Module,
g_net: nn.Module,
batch_size: int,
latent_size: int,
criterion: nn.Module,
optimizer: optim.Optimizer,
device: str):
# 定义生成样本的标签和噪声
real_labels = torch.ones(batch_size, 1).to(device)
z = torch.randn(batch_size, latent_size).to(device)
# 训练生成器
fake_images = g_net(z)
outputs = d_net(fake_images)
g_loss = criterion(outputs, real_labels) # 计算判别器结果和真实标签的损失
g_loss.backward() # 反向传播
optimizer.step() # 更新参数
optimizer.zero_grad() # 清空梯度
return g_loss, fake_images
def generate_and_save_images(g_net: nn.Module,
batch_size: int,
latent_size: int,
device: str,
image_prefix: str,
index: int) -> bool:
def dnorm(x: torch.Tensor):
min_value = -1
max_value = 1
out = (x - min_value) / (max_value - min_value)
return out.clamp(0, 1) # plt expects values in [0,1]
sample_vectors = torch.randn(batch_size, latent_size).to(device)
fake_images = g_net(sample_vectors)
fake_images = fake_images.view(batch_size, 1, 28, 28)
if os.path.exists(image_prefix) is False:
os.makedirs(image_prefix)
save_image(dnorm(fake_images), os.path.join(image_prefix, f'fake_images-{index:03d}.png'), nrow=10)
return True
def run_epoch(d_net: nn.Module,
g_net: nn.Module,
train_loader: DataLoader,
criterion: nn.Module,
d_optim: optim.Optimizer,
g_optim: optim.Optimizer,
batch_size: int,
latent_size: int,
device: str,
d_loss_list: list,
g_loss_list: list,
real_score_list: list,
fake_score_list: list,
epoch: int, num_epochs: int):
d_net.train()
g_net.train()
for idx, (images, _) in enumerate(train_loader):
images = images.view(batch_size, -1).to(device)
# 训练鉴别器
d_loss, real_score, fake_score = run_discriminator_one_batch(d_net, g_net, batch_size, latent_size, images,
criterion, d_optim, device)
# 训练生成器
g_loss, _ = run_generator_one_batch(d_net, g_net, batch_size, latent_size, criterion, g_optim, device)
if (idx + 1) % 300 == 0:
num = f"Epoch: [{epoch + 1}/{num_epochs}], Batch: [{idx + 1}/{len(train_loader)}]"
loss_info = f"Discriminator Loss: {d_loss.item():.4f}, Generator Loss: {g_loss.item():.4f}"
real_sample_score = f"Real sample score for Discriminator D(x): {real_score.mean().item():.4f}"
fake_sample_score = f"Fake sample score for Discriminator D(G(x)): {fake_score.mean().item():.4f}"
print(num + loss_info)
print(num + real_sample_score)
print(num + fake_sample_score)
d_loss_list.append(d_loss.item())
g_loss_list.append(g_loss.item())
real_score_list.append(real_score.mean().item())
fake_score_list.append(fake_score.mean().item())
训练框架
from training import run_epoch, generate_and_save_images
image_prefix = "./sample"
for epoch in range(num_epochs):
run_epoch(d_net=D, g_net=G,
train_loader=data_loader, criterion=criterion,
d_optim=d_optim, g_optim=g_optim,
batch_size=batch_size, latent_size=latent_size, device=device,
d_loss_list=d_loss_list, g_loss_list=g_loss_list,
real_score_list=real_score_list, fake_score_list=fake_score_list,
epoch=epoch, num_epochs=num_epochs)
if (epoch+1) % 10 == 0:
if generate_and_save_images(g_net=G, batch_size=batch_size,
latent_size=latent_size, device=device,
image_prefix=image_prefix, index=epoch+1):
print(f"Generated images at epoch {epoch+1}")
# Epoch: [1/300], Batch: [300/600]Discriminator Loss: 1.1440, Generator Loss: 0.5215
# Epoch: [1/300], Batch: [300/600]Real sample score for Discriminator D(x): 0.8644
# Epoch: [1/300], Batch: [300/600]Fake sample score for Discriminator D(G(x)): 0.6283
# Epoch: [1/300], Batch: [600/600]Discriminator Loss: 1.3556, Generator Loss: 0.8904
# Epoch: [1/300], Batch: [600/600]Real sample score for Discriminator D(x): 0.9466
# Epoch: [1/300], Batch: [600/600]Fake sample score for Discriminator D(G(x)): 0.6932
# ...
# Epoch: [300/300], Batch: [600/600]Discriminator Loss: 1.1809, Generator Loss: 0.5166
# Epoch: [300/300], Batch: [600/600]Real sample score for Discriminator D(x): 0.8612
# Epoch: [300/300], Batch: [600/600]Fake sample score for Discriminator D(G(x)): 0.6094
# Generated images at epoch 300
结果处理
保存checkpoint
import os
checkpoint_path = "./checkpoints"
if not os.path.exists(checkpoint_path):
os.makedirs(checkpoint_path)
torch.save(G.state_dict(), os.path.join(checkpoint_path, "G.pt"))
torch.save(D.state_dict(), os.path.join(checkpoint_path, "D.pt"))
损失变化与判别器评判损失/分数
plt.plot(d_loss_list[::200], label="Discriminator Loss")
plt.plot(g_loss_list[::200], label="Generator Loss")
plt.xlabel("Step")
plt.ylabel("Loss")
plt.legend(loc='upper right', bbox_to_anchor=(1, 1))
plt.show()
plt.plot(real_score_list[::200], label="Real Score")
plt.plot(fake_score_list[::200], label="Fake Score")
plt.xlabel("Step")
plt.ylabel("Score")
plt.legend(loc='upper right', bbox_to_anchor=(1, 1))
plt.show()
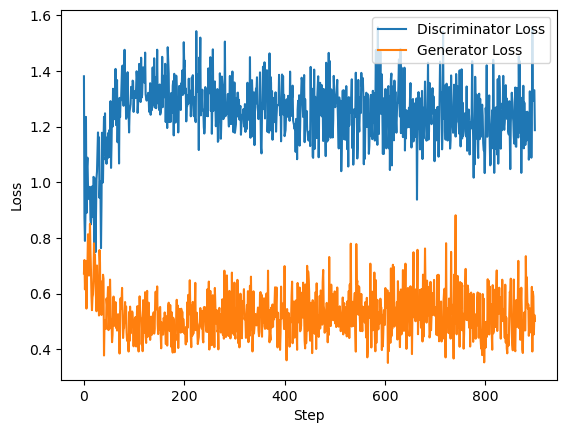
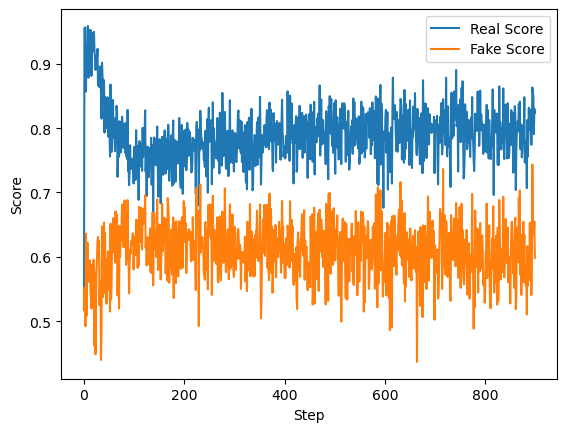
生成的图像
from IPython.display import Image
Image(os.path.join(image_prefix, "fake_images-010.png"))
Image(os.path.join(image_prefix, "fake_images-300.png"))
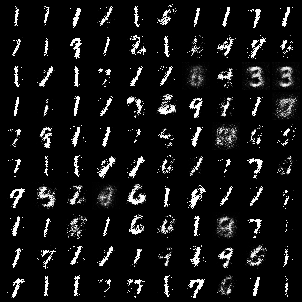
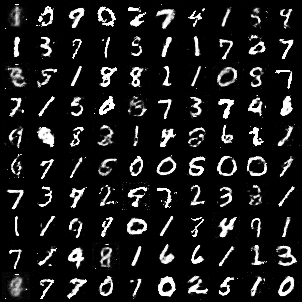
运行环境
torch==2.1.1
torchvision==0.16.1