Autonomous multi-floor indoor navigation with a computationally constrained MAV
Abstract:
In this paper, we consider the problem of autonomous navigation with a micro aerial vehicle (MAV) in indoor environments. In particular, we are interested in autonomous navigation in buildings with multiple floors. To ensure that the robot is fully autonomous, we require all computation to occur on the robot without need for external infrastructure, communication, or human interaction beyond high-level commands. Therefore, we pursue a system design and methodology that enables autonomous navigation with real time performance on a mobile processor using only onboard sensors. Specifically, we address multi-floor mapping with loop closure, localization, planning, and autonomous control, including adaptation to aerodynamic effects during traversal through spaces with low vertical clearance or strong external disturbances. We present experimental results with ground truth comparisons and performance analysis.
室内场景的无人机自主导航,多层建筑物中的自主导航,完全板载cpu计算,无需任何外部设施,通信,人为干预。闭环(loop closure),空气动力学aerodynamic
Gesture-based piloting of an aerial robot using monocular vision
Abstract:
Aerial robots are becoming popular among general public, and with the development of artificial intelligence (AI), there is a trend to equip aerial robots with a natural user interface (NUI). Hand/arm gestures are an intuitive way to communicate for humans, and various research works have focused on controlling an aerial robot with natural gestures. However, the techniques in this area are still far from mature. Many issues in this area have been poorly addressed, such as the principles of choosing gestures from the design point of view, hardware requirements from an economic point of view, considerations of data availability, and algorithm complexity from a practical perspective. Our work focuses on building an economical monocular system particularly designed for gesture-based piloting of an aerial robot. Natural arm gestures are mapped to rich target directions and convenient fine adjustment is achieved. Practical piloting scenarios, hardware cost and algorithm applicability are jointly considered in our system design. The entire system is successfully implemented in an aerial robot and various properties of the system are tested.
用户交互界面,经济解决方案,无人机系统设计,基于手势的无人机控制
论文提出的系统架构
Real-Time Onboard Mapping and Localization of an Indoor MAV Using Laser Range Finder
Abstract:
In this paper, we focus on the problem of micro aerial vehicle's (MAV's) localization in unknown, GPS-denied indoor condition. For this problem, we present a system to obtain the pose estimation and the occupancy grid map of the environment by using laser range finder. In addition, to improve the accuracy and robustness of tracking algorithm, we design a method by fusing the pose estimation from SLAM with IMU data. Furthermore, because of the length of the corridor may exceed the measurement range of the laser range finder, we specifically put forward an approach by fusing optical flow and IMU to compensate the error for this. Plenty of real flights and static precision experiments have proved the validity and accuracy of the proposed method.
无人机室内重建,无GPS下,激光测距获取姿态,提出一种SLAM姿态评估和IMU数据融合的方法
weedNet: Dense Semantic Weed Classification Using Multispectral Images and MAV for Smart Farming
Abstract:
Selective weed treatment is a critical step in autonomous crop management as related to crop health and yield. However, a key challenge is reliable and accurate weed detection to minimize damage to surrounding plants. In this letter, we present an approach for dense semantic weed classification with multispectral images collected by a micro aerial vehicle (MAV). We use the recently developed encoder–decoder cascaded convolutional neural network, SegNet, that infers dense semantic classes while allowing any number of input image channels and class balancing with our sugar beet and weed datasets. To obtain training datasets, we established an experimental field with varying herbicide levels resulting in field plots containing only either crop or weed, enabling us to use the normalized difference vegetation index as a distinguishable feature for automatic ground truth generation. We train six models with different numbers of input channels and condition (fine tune) it to achieve ∼0.8 F1-score and 0.78 area under the curve classification metrics. For the model deployment, an embedded Graphics Processing Unit (GPU) system (Jetson TX2) is tested for MAV integration. Dataset used in this letter is released to support the community and future work.
提出一种网络用于对田地中作物和杂草进行分类,使用无人机采集的数据作为判断来源

Waypoint navigation of quad-rotor MAV
Abstract:
Quad-rotor Micro Aerial Vehicle (MAV) is a multi-rotor MAV with 4 propellers which propel the MAV up to the air and move around. It has high maneuverability to move around, such as roll, pitch and yaw movements. However, line of sight and radio control effective range are the major limitation for the MAVs which significantly shorten the travel distance. Therefore, we proposed a waypoint navigation quad-rotor MAV based on PID controller in this paper. User can set mission with multiple waypoint and the PID controller to control MAV autonomously moving along the waypoint to the desired position without remotely controlled by radio control and guidance of pilot. The results show PID controller is capable to control MAV to move to the desired position with high accuracy. As the conclusion, the result of real flight experiment shows that the %OS of designed PID controller for x is 13% while y is 11.89% and z is 2.34%. Meanwhile, steady-state error for all axis are 0%. This shows that the performance of PID controller is satisfied. Hence, the quadrotor MAV could move to the desired location via waypoint navigation without guidance of pilot.
基于PID控制器对无人机控制,自主移动到所需位置。主要为自主控制方面的研究
偏无人机控制,控制算法的研究等等
来源:2017 4th International Conference on Information Science and Control Engineering (ICISCE)
老师推荐的文章,相关度很高,重点阅读!!
http://openaccess.thecvf.com/content_ICCV_2017/papers/Roberts_Submodular_Trajectory_Optimization_ICCV_2017_paper.pdf
Submodular Trajectory Optimization for Aerial 3D Scanning
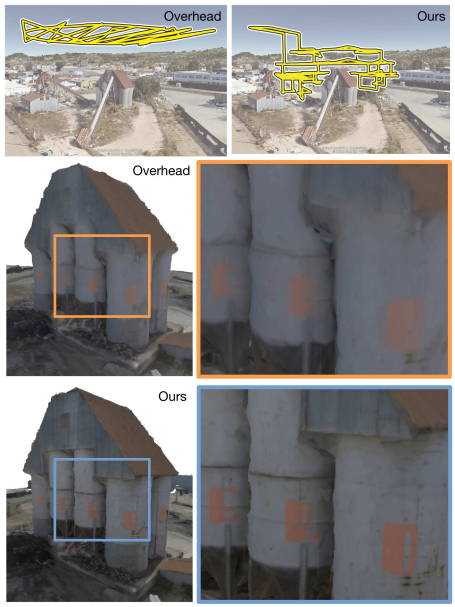
Abstract:
Drones equipped with cameras are emerging as a powerful tool for large-scale aerial 3D scanning, but existing automatic flight planners do not exploit all available information about the scene, and can therefore produce inaccurate and incomplete 3D models. We present an automatic method to generate drone trajectories, such that the imagery acquired during the flight will later produce a high- fidelity 3D model. Our method uses a coarse estimate of the scene geometry to plan camera trajectories that: (1) cover the scene as thoroughly as possible; (2) encourage observations of scene geometry from a diverse set of viewing angles; (3) avoid obstacles; and (4) respect a user-specified flight time budget. Our method relies on a mathematical model of scene coverage that exhibits an intuitive diminishing returns property known as submodularity. We leverage this property extensively to design a trajectory planning algorithm that reasons globally about the non-additive coverage reward obtained across a trajectory, jointly with the cost of traveling between views. We evaluate our method by using it to scan three large outdoor scenes, and we perform a quantitative evaluation using a photorealistic video game simulator.