【python实现卷积神经网络】池化层实现
代码来源:https://github.com/eriklindernoren/ML-From-Scratch
卷积神经网络中卷积层Conv2D(带stride、padding)的具体实现:https://www.cnblogs.com/xiximayou/p/12706576.html
激活函数的实现(sigmoid、softmax、tanh、relu、leakyrelu、elu、selu、softplus):https://www.cnblogs.com/xiximayou/p/12713081.html
损失函数定义(均方误差、交叉熵损失):https://www.cnblogs.com/xiximayou/p/12713198.html
优化器的实现(SGD、Nesterov、Adagrad、Adadelta、RMSprop、Adam):https://www.cnblogs.com/xiximayou/p/12713594.html
卷积层反向传播过程:https://www.cnblogs.com/xiximayou/p/12713930.html
全连接层实现:https://www.cnblogs.com/xiximayou/p/12720017.html
批量归一化层实现:https://www.cnblogs.com/xiximayou/p/12720211.html
包括D的平均池化和最大池化:
class PoolingLayer(Layer): """A parent class of MaxPooling2D and AveragePooling2D """ def __init__(self, pool_shape=(2, 2), stride=1, padding=0): self.pool_shape = pool_shape self.stride = stride self.padding = padding self.trainable = True def forward_pass(self, X, training=True): self.layer_input = X batch_size, channels, height, width = X.shape _, out_height, out_width = self.output_shape() X = X.reshape(batch_size*channels, 1, height, width) X_col = image_to_column(X, self.pool_shape, self.stride, self.padding) # MaxPool or AveragePool specific method output = self._pool_forward(X_col) output = output.reshape(out_height, out_width, batch_size, channels) output = output.transpose(2, 3, 0, 1) return output def backward_pass(self, accum_grad): batch_size, _, _, _ = accum_grad.shape channels, height, width = self.input_shape accum_grad = accum_grad.transpose(2, 3, 0, 1).ravel() # MaxPool or AveragePool specific method accum_grad_col = self._pool_backward(accum_grad) accum_grad = column_to_image(accum_grad_col, (batch_size * channels, 1, height, width), self.pool_shape, self.stride, 0) accum_grad = accum_grad.reshape((batch_size,) + self.input_shape) return accum_grad def output_shape(self): channels, height, width = self.input_shape out_height = (height - self.pool_shape[0]) / self.stride + 1 out_width = (width - self.pool_shape[1]) / self.stride + 1 assert out_height % 1 == 0 assert out_width % 1 == 0 return channels, int(out_height), int(out_width) class MaxPooling2D(PoolingLayer): def _pool_forward(self, X_col): arg_max = np.argmax(X_col, axis=0).flatten() output = X_col[arg_max, range(arg_max.size)] self.cache = arg_max return output def _pool_backward(self, accum_grad): accum_grad_col = np.zeros((np.prod(self.pool_shape), accum_grad.size)) arg_max = self.cache accum_grad_col[arg_max, range(accum_grad.size)] = accum_grad return accum_grad_col class AveragePooling2D(PoolingLayer): def _pool_forward(self, X_col): output = np.mean(X_col, axis=0) return output def _pool_backward(self, accum_grad): accum_grad_col = np.zeros((np.prod(self.pool_shape), accum_grad.size)) accum_grad_col[:, range(accum_grad.size)] = 1. / accum_grad_col.shape[0] * accum_grad return accum_grad_col
需要注意的是池化层是没有可学习的参数的(如果不利用带步长的卷积来代替池化的作用),还有就是池化层反向传播的过程,这里参考:https://blog.csdn.net/Jason_yyz/article/details/80003271
为了结合代码看直观些,就将其内容摘了下来:
Pooling池化操作的反向梯度传播
CNN网络中另外一个不可导的环节就是Pooling池化操作,因为Pooling操作使得feature map的尺寸变化,假如做2×2的池化,假设那么第l+1层的feature map有16个梯度,那么第l层就会有64个梯度,这使得梯度无法对位的进行传播下去。其实解决这个问题的思想也很简单,就是把1个像素的梯度传递给4个像素,但是需要保证传递的loss(或者梯度)总和不变。根据这条原则,mean pooling和max pooling的反向传播也是不同的。
1、mean pooling
mean pooling的前向传播就是把一个patch中的值求取平均来做pooling,那么反向传播的过程也就是把某个元素的梯度等分为n份分配给前一层,这样就保证池化前后的梯度(残差)之和保持不变,还是比较理解的,图示如下 :
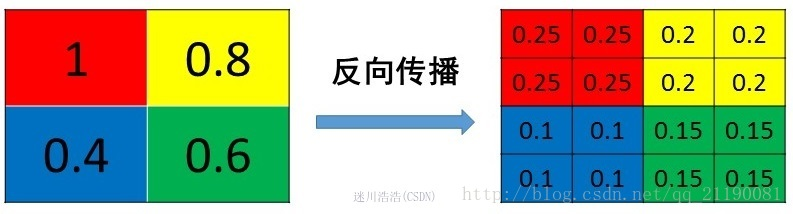
mean pooling比较容易让人理解错的地方就是会简单的认为直接把梯度复制N遍之后直接反向传播回去,但是这样会造成loss之和变为原来的N倍,网络是会产生梯度爆炸的。
2、max pooling
max pooling也要满足梯度之和不变的原则,max pooling的前向传播是把patch中最大的值传递给后一层,而其他像素的值直接被舍弃掉。那么反向传播也就是把梯度直接传给前一层某一个像素,而其他像素不接受梯度,也就是为0。所以max pooling操作和mean pooling操作不同点在于需要记录下池化操作时到底哪个像素的值是最大,也就是max id,这个变量就是记录最大值所在位置的,因为在反向传播中要用到,那么假设前向传播和反向传播的过程就如下图所示 :
【推荐】编程新体验,更懂你的AI,立即体验豆包MarsCode编程助手
【推荐】凌霞软件回馈社区,博客园 & 1Panel & Halo 联合会员上线
【推荐】抖音旗下AI助手豆包,你的智能百科全书,全免费不限次数
【推荐】博客园社区专享云产品让利特惠,阿里云新客6.5折上折
【推荐】轻量又高性能的 SSH 工具 IShell:AI 加持,快人一步