Transformer
论文链接:Attention is all you need
Intro
之前的RNN和LSTM方法是基于时序的,不能并行化(parallelizable),也就是计算了t-1时刻之后才能计算t时刻,训练效率就比较低。
Self-Attention
An attention mechanism relating different positions of a single sequence in order to compute a representation of the sequence
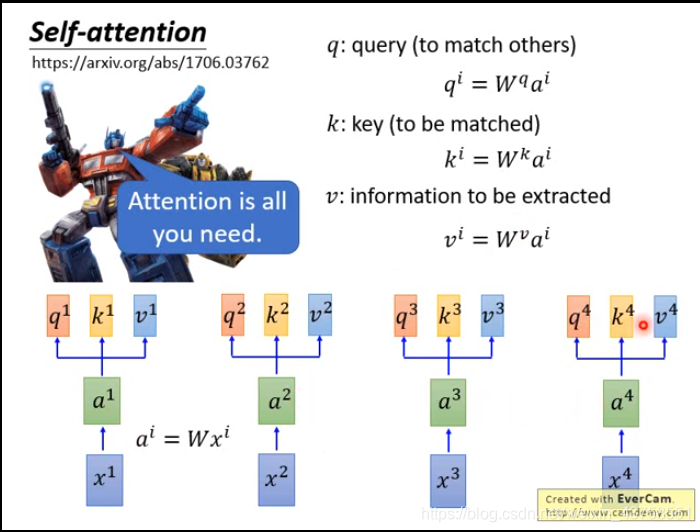
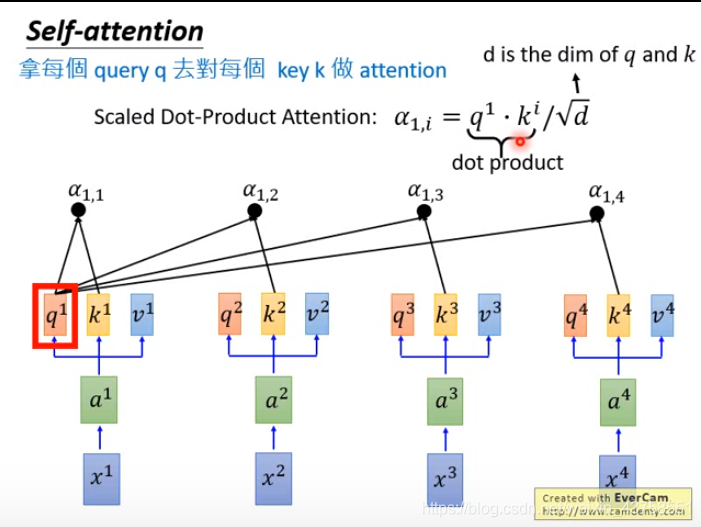
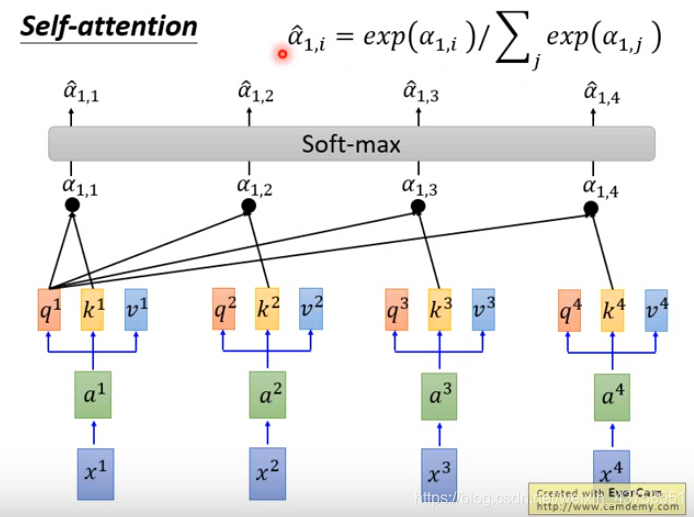
计算出的每一个\(\hat a\)是相对于\(v\)的权重(是一个数值而不是向量),权重越大表示越关注这个\(v\),权重就是query和key的相似度
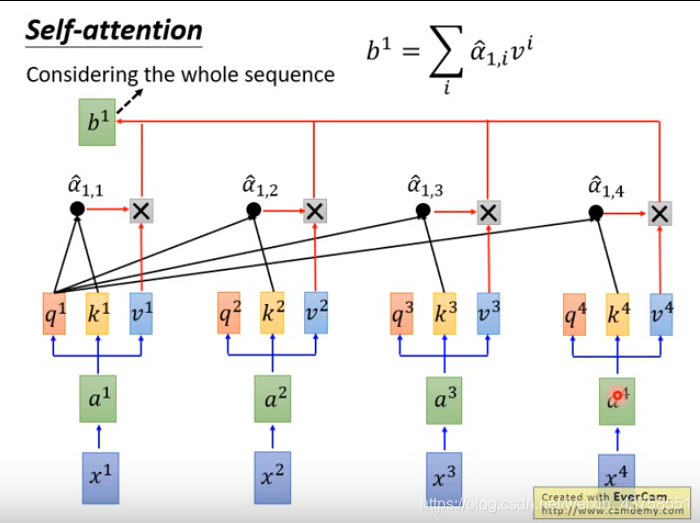
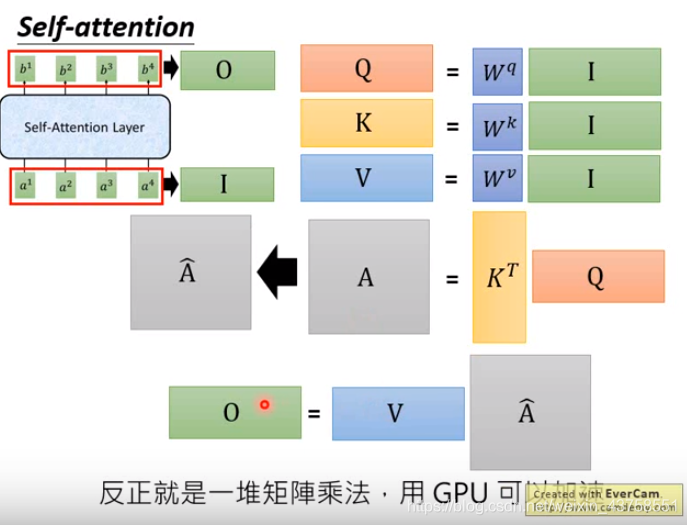
矩阵表示
上述操作都是矩阵乘法,可以并行化处理
Multi-head Self-Attention
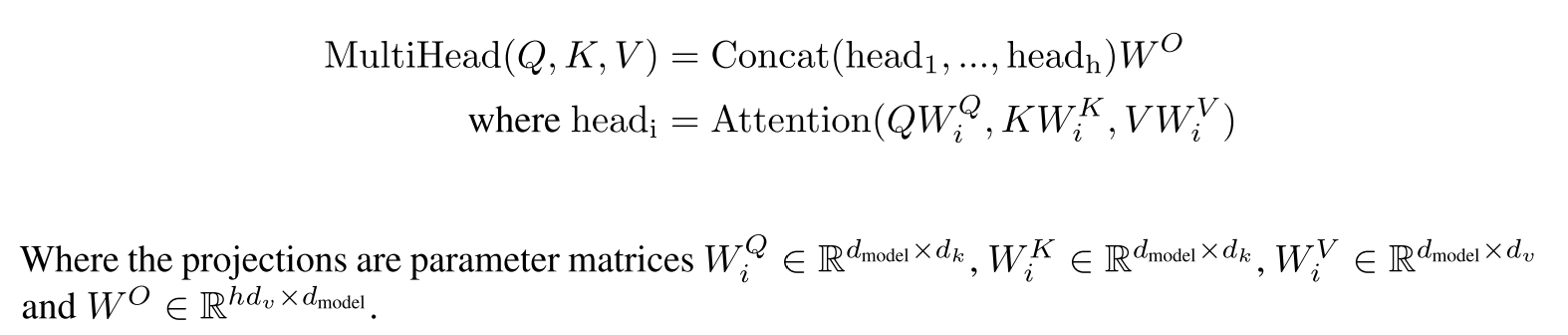
将\(q^1\)拆分成\(q^{1,1}\)和\(q^{1,2}\),原论文中是均分,比如把\((1,1,0,1)\)分成\((1,1)\)和\((0,1)\)
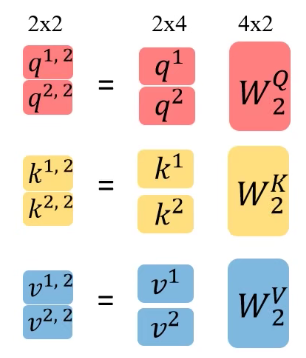
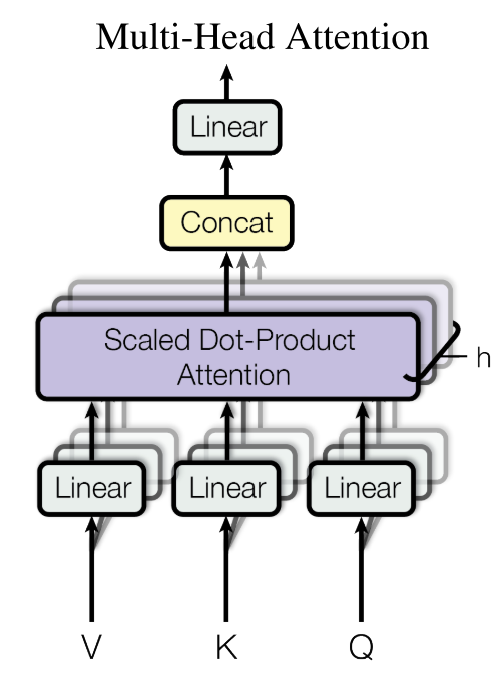
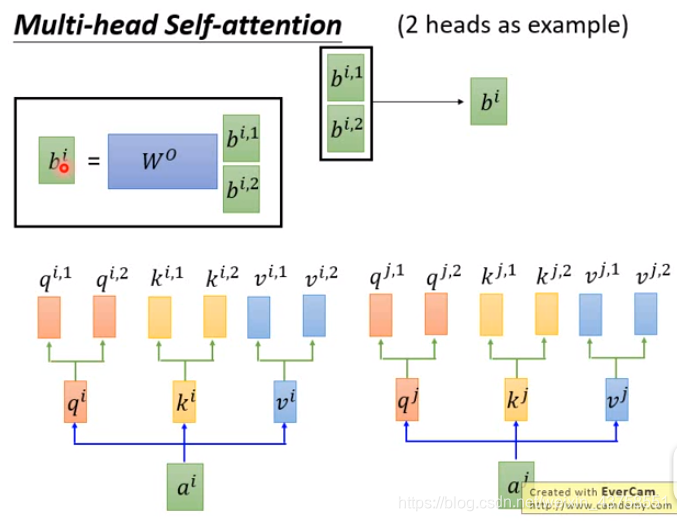
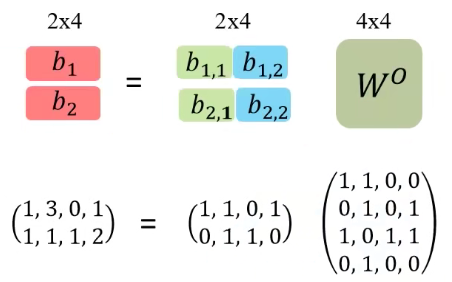
Encoder and Decoder
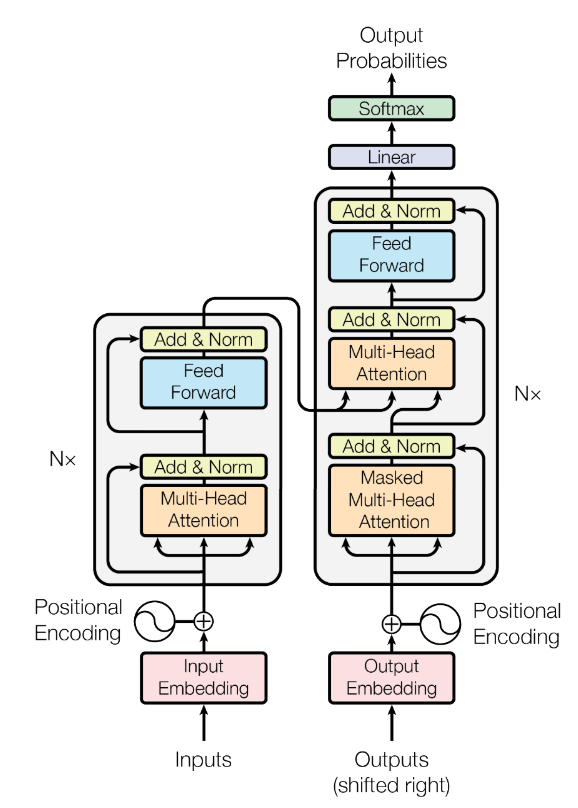
左边为encoder
multi-head self-attention + MLP
有一个residual connection, LayerNorm(x + Sublayer(x))
在变长的应用中使用LayerNorm而不是BatchNorm,对样本进行normalization而不是feature
右边为decoder
当前时刻的输入是上一时刻的输出
decoder在训练时基于t时刻之前的数据,不应该知道t以后的输入,而self-attention机制能看到所有时刻的数据
所以使用Mask,保证t时刻不会看到之后的数据,使训练与预测行为一致
Reference
[1] 这么多年,终于有人讲清楚 Transformer 了!