凸集 Convex Sets
仿射集 Affine Sets
线和线段
线 line
线段 line segment
上述条件当带有约束条件\(0 \le \theta \le 1\)时,\(y\)是线段
仿射集affine set
也就是说,仿射集中任意两点的线性组合仍然属于仿射集,注意必须满足约束条件coefficients sum to one
仿射集一定是凸集
仿射组合affine combination
An affine set contains every affine combination of its points
仿射包 affine hull
\({\rm aff} \ C\)是一个仿射包,通俗理解,仿射包就是包含集合\(C\)的最小的仿射集合
对任意集合A,增加最少的元素使A变为仿射集B,则仿射集A是B的仿射包,即A是包含B的最小仿射集。
线段的仿射包是包含这条线段的直线,平面多边形(三角形,正方形等)的仿射包是包含此多边形的整个平面,仿射集的仿射包是它自身
仿射集的子空间
上述仿射集成立的条件必须有\(\sum_{i=1}^k \theta_i=1\)的约束
那么什么情况下可以去掉该约束呢
从下图可以看出
- 不过原点的直线(仿射集C)上任取两点,向量\(x_1,x_2\)的和\(x_1+x_2\)(其实是线性组合\(\alpha \cdot x_1+\beta \cdot x_2,\ \alpha=\beta=1\),此时不满足\(\sum_{i=1}^k \theta_i=1\)的约束)并不属于仿射集C
- 但过原点的直线(另一个仿射集)上向量的任意线性组合依然在该仿射集上(因为\(x_1,x_2\)线性相关)
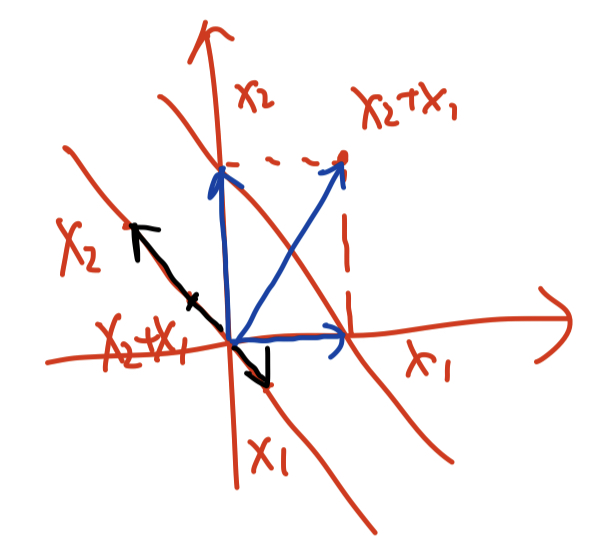
那么貌似有些头绪了,下面定义
V是C的子空间,这个子空间中向量的任意线性组合依然属于子空间
因此仿射集\(C\)可以表示为其子空间\(V\)加上一个偏置
即对仿射集C, 首先选出C中任意一个元素,再用C中所有元素都同时减去这个元素,此时得到一个新的集合,这个集合必有一个0元素,这样得到的新集合就是一个与C相关的子空间。
子空间相当于是基于\(x_0\)做了一个平移,使之必过原点,子空间是仿射集。
相对内部 relative interier
相对内部
相当于一个不包含边缘的开集
凸集 Convex Sets
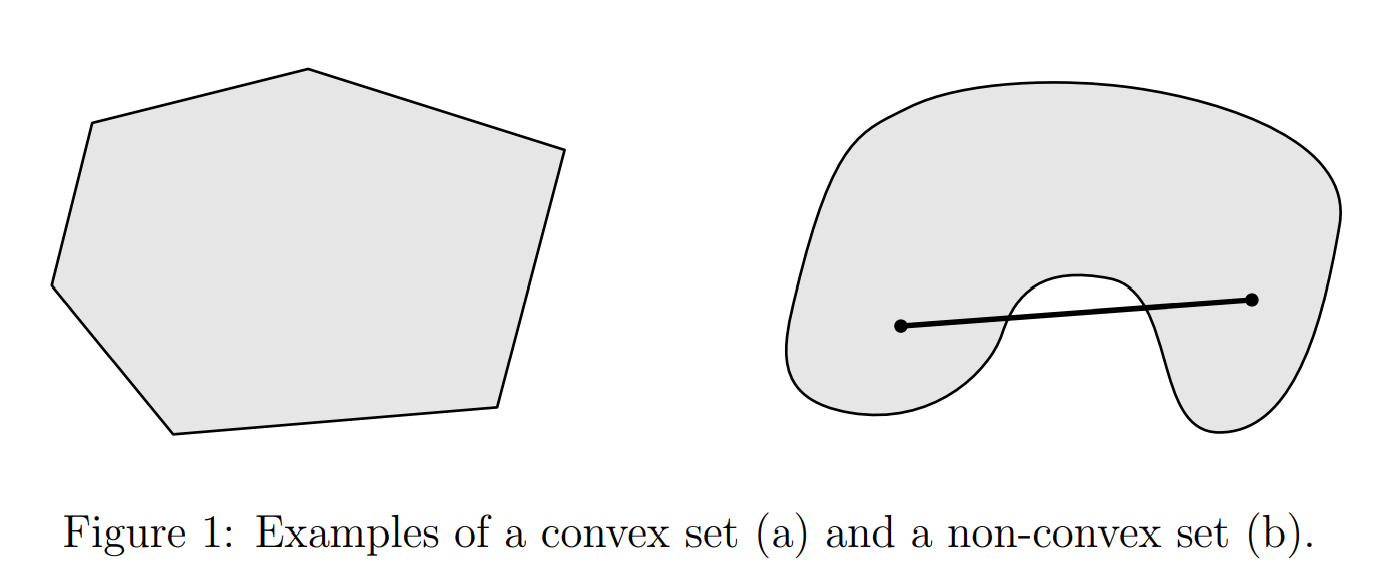
凸集的定义
$$ \forall x_1,x_2 \in C, 0\le\theta\le1\\ \theta\cdot x_1+(1-\theta)\cdot x_2 \in C $$ 也就是说集合中的两点连成的线段也要在集合中,凸集比仿射集多的限制就是$\theta \in [0,1]$凸组合 convex combination
凸包 convex hull
凸包是包含\(C\)(任意集合,不一定是凸集)的最小凸集
所以凸包就是将非凸集合通过凸组合的定义形式形成一个凸集
凸包的例子如下图所示:
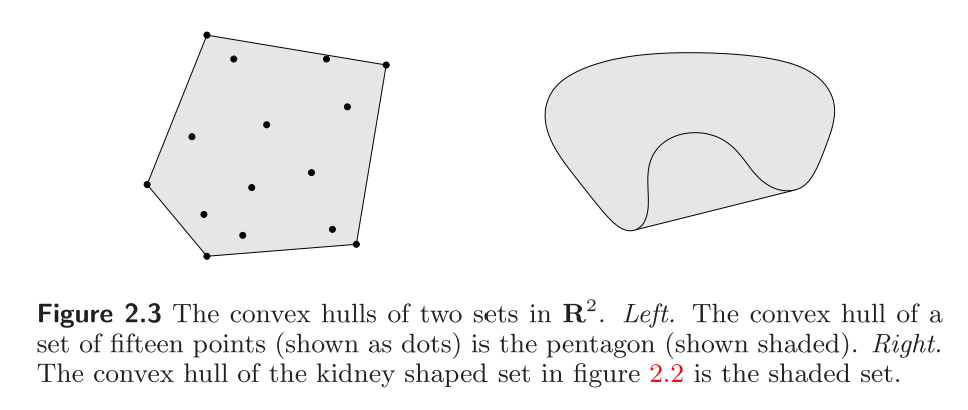
锥 Cones
锥的定义
Cone 又叫 nonnegative homogeneous
锥必过原点,如在二维平面中一条以原点作为端点的射线是锥,由多条这样的射线构成的集合也是锥。
比如:齐次方程组的解是锥
凸锥 convex cone
A set C is a convex cone if it is convex and a cone.
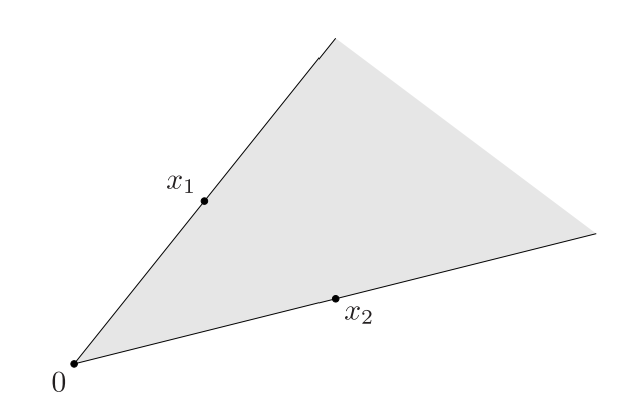
锥组合 conic combination
锥包 conic hull
与前面仿射包和凸包的定义类似,锥包就是所有锥组合的集合
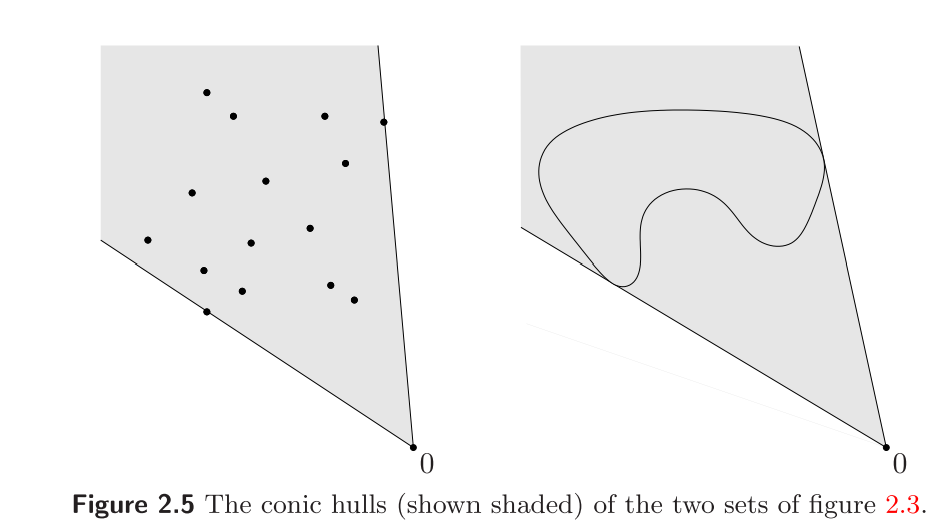
仿射集、凸集、凸锥
仿射集:\(\sum \theta_i=1\)
凸集:\(\sum \theta_i=1,\theta_i \ge 0\)
凸锥:\(\theta_i \ge 0\)
常见的凸集
The empty set; single point; the whole space \(R^n\)
Any line is affine. If it passes through zero, it is a subspace, hence also a convex cone.
A line segment is convex, but not affine (unless it reduces to a point).
A ray, which has the form \(\{x0 + θv | θ ≥ 0\}\), where \(v \ne 0\), is convex, but not affine. It is a convex cone if its base \(x_0\) is 0.
Any subspace is affine, and a convex cone (hence convex).
\(\forall x_1,x_2 \in C, \theta \in \R \Rightarrow \theta\cdot x_1+(1-\theta)\cdot x_2 \in C\),那么\(C\)是仿射函数,仿射集是凸集,仿射集的交集也为凸集
超平面 Hyperplanes
超平面是仿射集,凸集
半空间 Halfspace
半空间是凸集,但不是仿射集