CG
链接:https://www.zhihu.com/question/299068775/answer/525874350
来源:知乎
著作权归作者所有。商业转载请联系作者获得授权,非商业转载请注明出处。
我感觉CV还好,毕竟是大趋势,要是研究CG试试,简直是坑得吐血,本人研究方向运动捕捉,使用机器学习重构人体姿态,注意不是依据图像信息重构人体姿态,而是利用动捕数据来生成满足特定任务的行为,比如达到某个目标点,处于某种特定姿态,等等。这个我个人感觉只有游戏或者动画能用,但是这些领域一般都是专业人士捕捉好的,鬼才用机器学习生成的效果还不好的那些动画。
像openai这样做的虽然很厉害,但是无法真正投入工程使用,这生成的人体姿态简直无法吐槽:
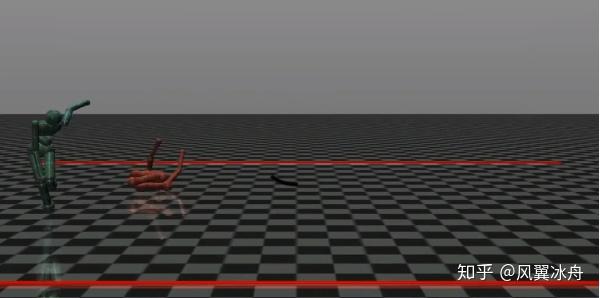
相比之下,近年来siggraph上面部分文章做的还好,比如这篇论文:
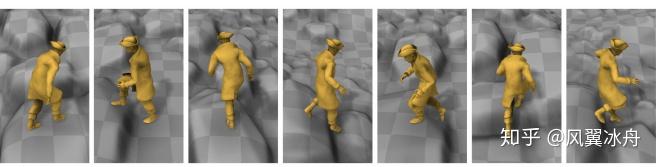
但是细节部分,比如脚的位置,有时候也不是特别好,会嵌入地面内部,实际使用还要经过大量运动学优化,然鹅用运动学以后,矫正太狠就无法保证自然性。
作者之前的论文也做了一个简单的工作,文章戳这里:
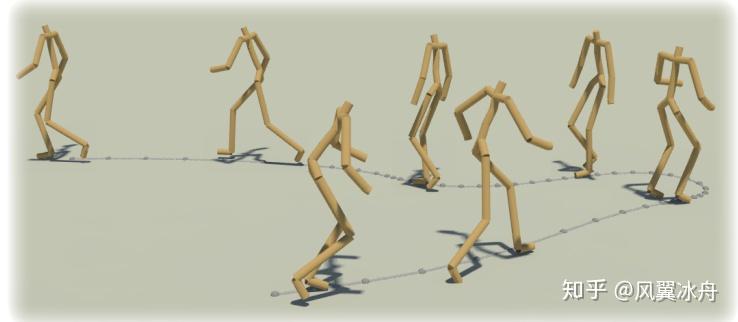
————————更新2019-2-24————————
没想到还有这么多人点赞 T_T ,那我再贴点图:
还能用来控制动物走跑跳站立,用深度学习,文章戳这里:
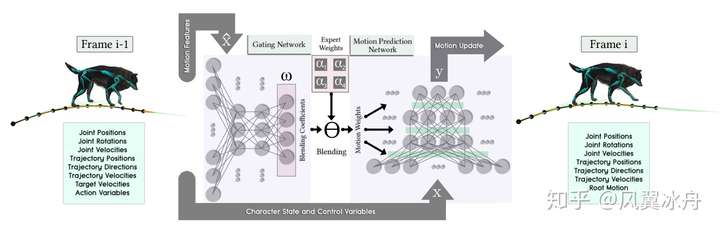
还有飞天龙,用深度强化学习,文章戳这里:
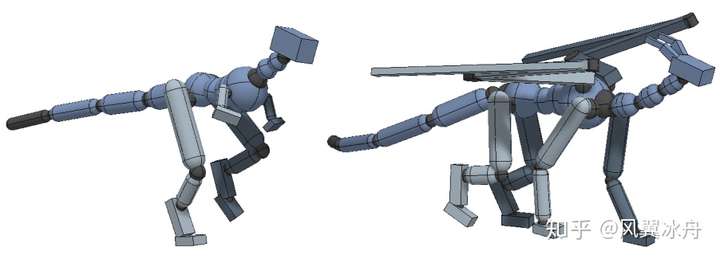
走钢丝,爬楼梯,深度强化学习,文章同上
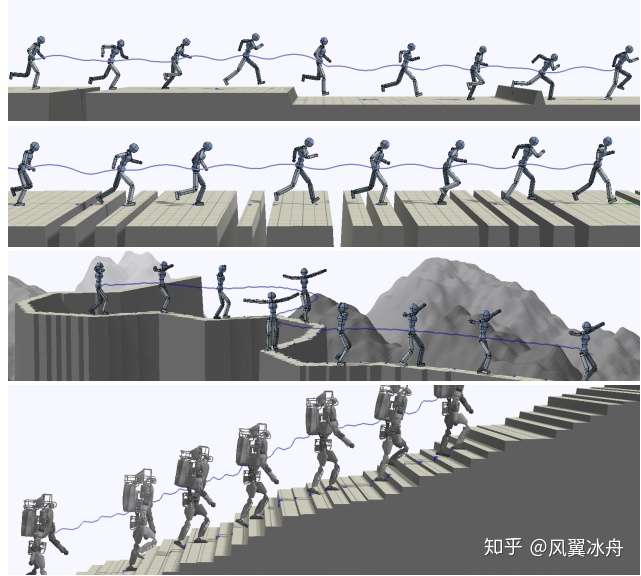
拳击手,高斯过程属于机器学习,不过是12年的文章了,所以还莫得深度强化学习加进来,文章戳这里
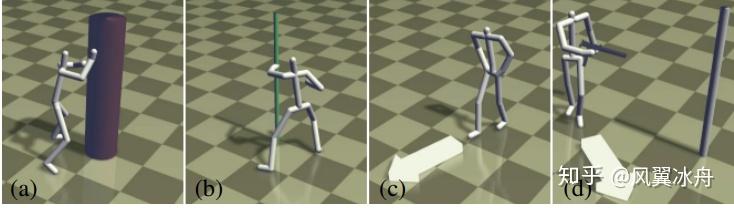
鬼畜,高斯过程,文章同上:
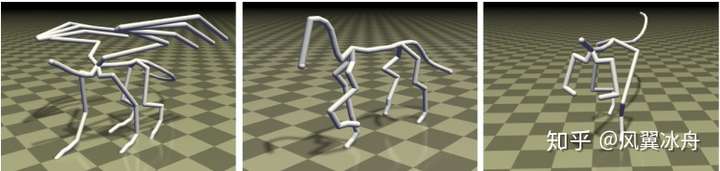
但是自己的研究方向,哭着也要做下去,嗯!!
其实用机器学习比如深度强化学习做MOCAP不仅要让虚拟角色学习如何去完成指定任务,还得保证运动自然性,这才是难点,我jio得目前出现的AI玩游戏中使用的深度强化学习,只关注如何做出正确决策,并未关注角色执行决策时应遵循基本的运动学知识吧。
—————————更新一波:2019-4-2————————
把部分文章代码和网址都丢出来,方便有兴趣的大佬研究研究。
- 《Robust Generation of Dynamical Patterns in Human Motion by a Deep Belief Nets》
[主页](Sainbayar Sukhbaatar),[论文](Robust Generation of Dynamical Patterns in Human Motion by a Deep Belief Nets),[代码](tesatory/TDBN),[视频](https://youtu.be/aGLBFuennXI)
- 《Continuous Character Control with Low-Dimensional Embeddings》
[主页](http://graphics.stanford.edu/projects/ccclde/),[论文](http://graphics.stanford.edu/projects/ccclde/ccclde.pdf),[代码](http://graphics.stanford.edu/projects/ccclde/ccclde_code.zip),[视频](http://graphics.stanford.edu/projects/ccclde/ccclde.mp4)
- 《A Deep Learning Framework For Character Motion Synthesis and Editing》
[主页](A Deep Learning Framework For Character Motion Synthesis and Editing),[论文](http://theorangeduck.com/media/uploads/motionsynthesis.pdf),[代码](http://theorangeduck.com/media/uploads/other_stuff/motionsynth_code.zip),[数据](http://theorangeduck.com/media/uploads/other_stuff/motionsynth_data.zip),[视频](https://www.youtube.com/watch?v=urf-AAIwNYk)
- 《Phase-Functioned Neural Networks for Character Control》
[主页](Phase-Functioned Neural Networks for Character Control),[论文](http://theorangeduck.com/media/uploads/other_stuff/phasefunction.pdf),[代码](http://theorangeduck.com/media/uploads/other_stuff/pfnn.zip),[视频](NVIDIA SIGGRAPH 2017)
- 《DeepLoco: Dynamic Locomotion Skills Using Hierarchical Deep Reinforcement Learning》
[主页](DeepLoco: Dynamic Locomotion Skills Using Hierarchical Deep Reinforcement Learning),[论文](https://xbpeng.github.io/projects/DeepLoco/2017_TOG_DeepLoco.pdf),[代码](xbpeng/DeepLoco),[视频1](https://youtu.be/G4lT9CLyCNw),[视频2](https://youtu.be/hd1yvLWm6oA),[视频3](https://youtu.be/x-HrYko_MRU)
- 《DeepMimic: Example-Guided Deep Reinforcement Learning of Physics-Based Character Skills》
[主页](DeepMimic: Example-Guided Deep Reinforcement Learning of Physics-Based Character Skills),[论文](https://xbpeng.github.io/projects/DeepMimic/2018_TOG_DeepMimic.pdf),[代码](xbpeng/DeepMimic),[视频1](https://youtu.be/vppFvq2quQ0),[视频2](https://youtu.be/8KdDwRLtNHQ)
- 《Terrain-Adaptive Locomotion Skills Using Deep Reinforcement Learning》
[论文](https://xbpeng.github.io/projects/DeepTerrainRL/2016_TOG_DeepTerrainRL.pdf),[代码](xbpeng/DeepTerrainRL),[视频](https://youtu.be/KPfzRSBzNX4)
- 《Mode-Adaptive Neural Networks for Quadruped Motion Control》
[主页](sebastianstarke/AI4Animation),[论文](http://homepages.inf.ed.ac.uk/tkomura/dog.pdf),[代码](sebastianstarke/AI4Animation),[视频](https://www.youtube.com/watch?v=uFJvRYtjQ4c)
- 《PiGraphs: Learning Interaction Snapshots from Observations》
[主页](http://graphics.stanford.edu/projects/pigraphs/),[论文](http://graphics.stanford.edu/projects/pigraphs/pigraphs.pdf),[代码](msavva/pigraphs),[视频](https://youtu.be/Ws9jPxCAFrg)
之前开源过的论文,代码在我百度云,**开山鼻祖**:
- 《Modeling human motion using binary latent variables 》
[论文](http://www2.egr.uh.edu/~zhan2/ECE6111_Fall2015/modeling%20human%20motion%20using%20binary%20latent%20variables.pdf),代码:[链接](https://pan.baidu.com/s/1CykAB4Dis2XcEq_bnYeRww ) 提取码:p353
- 《Factored Conditional Restricted Boltzmann Machines
for Modeling Motion Style》
[论文](http://www.cs.toronto.edu/~fritz/absps/fcrbm_icml.pdf),代码:[链接](https://pan.baidu.com/s/16i4tMu-cw1vLvWULZd-Bww ) 提取码:42i2
- [Dynamical binary latent variable models for 3D human pose tracking]
[论文](https://www.cs.ubc.ca/~lsigal/Publications/cvpr2010gwtaylor.pdf),[代码](gwtaylor/imCRBM)