转自:https://blog.csdn.net/lujiandong1/article/details/53376802
Tensorflow数据读取有三种方式:
- Preloaded data: 预加载数据
- Feeding: Python产生数据,再把数据喂给后端。
- Reading from file: 从文件中直接读取
这三种有读取方式有什么区别呢? 我们首先要知道TensorFlow(TF)是怎么样工作的。
TF的核心是用C++写的,这样的好处是运行快,缺点是调用不灵活。而Python恰好相反,所以结合两种语言的优势。涉及计算的核心算子和运行框架是用C++写的,并提供API给Python。Python调用这些API,设计训练模型(Graph),再将设计好的Graph给后端去执行。简而言之,Python的角色是Design,C++是Run。
一、预加载数据:
- import tensorflow as tf
-
- x1 = tf.constant([2, 3, 4])
- x2 = tf.constant([4, 0, 1])
- y = tf.add(x1, x2)
-
- with tf.Session() as sess:
- print sess.run(y)
二、python产生数据,再将数据喂给后端
- import tensorflow as tf
-
- x1 = tf.placeholder(tf.int16)
- x2 = tf.placeholder(tf.int16)
- y = tf.add(x1, x2)
-
- li1 = [2, 3, 4]
- li2 = [4, 0, 1]
-
- with tf.Session() as sess:
- print sess.run(y, feed_dict={x1: li1, x2: li2})
说明:在这里x1, x2只是占位符,没有具体的值,那么运行的时候去哪取值呢?这时候就要用到sess.run()
中的feed_dict
参数,将Python产生的数据喂给后端,并计算y。
这两种方案的缺点:
1、预加载:将数据直接内嵌到Graph中,再把Graph传入Session中运行。当数据量比较大时,Graph的传输会遇到效率问题。
2、用占位符替代数据,待运行的时候填充数据。
前两种方法很方便,但是遇到大型数据的时候就会很吃力,即使是Feeding,中间环节的增加也是不小的开销,比如数据类型转换等等。最优的方案就是在Graph定义好文件读取的方法,让TF自己去从文件中读取数据,并解码成可使用的样本集。
三、从文件中读取,简单来说就是将数据读取模块的图搭好
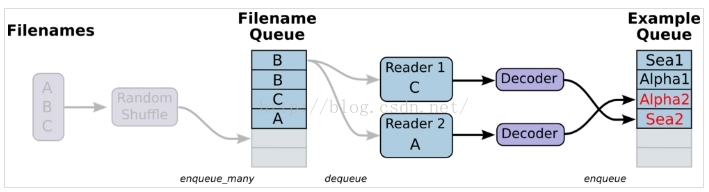
1、准备数据,构造三个文件,A.csv,B.csv,C.csv
- $ echo -e "Alpha1,A1\nAlpha2,A2\nAlpha3,A3" > A.csv
- $ echo -e "Bee1,B1\nBee2,B2\nBee3,B3" > B.csv
- $ echo -e "Sea1,C1\nSea2,C2\nSea3,C3" > C.csv
2、单个Reader,单个样本
-
- import tensorflow as tf
-
- filenames = ['A.csv', 'B.csv', 'C.csv']
- filename_queue = tf.train.string_input_producer(filenames, shuffle=False)
-
- reader = tf.TextLineReader()
- key, value = reader.read(filename_queue)
-
- example, label = tf.decode_csv(value, record_defaults=[['null'], ['null']])
-
-
- with tf.Session() as sess:
- coord = tf.train.Coordinator()
- threads = tf.train.start_queue_runners(coord=coord)
- for i in range(10):
- print example.eval(),label.eval()
- coord.request_stop()
- coord.join(threads)
说明:这里没有使用tf.train.shuffle_batch,会导致生成的样本和label之间对应不上,乱序了。生成结果如下:
Alpha1 A2
Alpha3 B1
Bee2 B3
Sea1 C2
Sea3 A1
Alpha2 A3
Bee1 B2
Bee3 C1
Sea2 C3
Alpha1 A2
解决方案:用tf.train.shuffle_batch,那么生成的结果就能够对应上。
-
- import tensorflow as tf
-
- filenames = ['A.csv', 'B.csv', 'C.csv']
- filename_queue = tf.train.string_input_producer(filenames, shuffle=False)
-
- reader = tf.TextLineReader()
- key, value = reader.read(filename_queue)
-
- example, label = tf.decode_csv(value, record_defaults=[['null'], ['null']])
- example_batch, label_batch = tf.train.shuffle_batch([example,label], batch_size=1, capacity=200, min_after_dequeue=100, num_threads=2)
-
- with tf.Session() as sess:
- coord = tf.train.Coordinator()
- threads = tf.train.start_queue_runners(coord=coord)
- for i in range(10):
- e_val,l_val = sess.run([example_batch, label_batch])
- print e_val,l_val
- coord.request_stop()
- coord.join(threads)
3、单个Reader,多个样本,主要也是通过tf.train.shuffle_batch来实现
-
- import tensorflow as tf
- filenames = ['A.csv', 'B.csv', 'C.csv']
- filename_queue = tf.train.string_input_producer(filenames, shuffle=False)
- reader = tf.TextLineReader()
- key, value = reader.read(filename_queue)
- example, label = tf.decode_csv(value, record_defaults=[['null'], ['null']])
-
-
-
- example_batch, label_batch = tf.train.batch(
- [example, label], batch_size=5)
- with tf.Session() as sess:
- coord = tf.train.Coordinator()
- threads = tf.train.start_queue_runners(coord=coord)
- for i in range(10):
- e_val,l_val = sess.run([example_batch,label_batch])
- print e_val,l_val
- coord.request_stop()
- coord.join(threads)
说明:下面这种写法,提取出来的batch_size个样本,特征和label之间也是不同步的
-
- import tensorflow as tf
- filenames = ['A.csv', 'B.csv', 'C.csv']
- filename_queue = tf.train.string_input_producer(filenames, shuffle=False)
- reader = tf.TextLineReader()
- key, value = reader.read(filename_queue)
- example, label = tf.decode_csv(value, record_defaults=[['null'], ['null']])
-
-
-
- example_batch, label_batch = tf.train.batch(
- [example, label], batch_size=5)
- with tf.Session() as sess:
- coord = tf.train.Coordinator()
- threads = tf.train.start_queue_runners(coord=coord)
- for i in range(10):
- print example_batch.eval(), label_batch.eval()
- coord.request_stop()
- coord.join(threads)
说明:输出结果如下:可以看出feature和label之间是不对应的
['Alpha1' 'Alpha2' 'Alpha3' 'Bee1' 'Bee2'] ['B3' 'C1' 'C2' 'C3' 'A1']
['Alpha2' 'Alpha3' 'Bee1' 'Bee2' 'Bee3'] ['C1' 'C2' 'C3' 'A1' 'A2']
['Alpha3' 'Bee1' 'Bee2' 'Bee3' 'Sea1'] ['C2' 'C3' 'A1' 'A2' 'A3']
4、多个reader,多个样本
-
- import tensorflow as tf
- filenames = ['A.csv', 'B.csv', 'C.csv']
- filename_queue = tf.train.string_input_producer(filenames, shuffle=False)
- reader = tf.TextLineReader()
- key, value = reader.read(filename_queue)
- record_defaults = [['null'], ['null']]
-
- example_list = [tf.decode_csv(value, record_defaults=record_defaults)
- for _ in range(2)]
-
- example_batch, label_batch = tf.train.batch_join(
- example_list, batch_size=5)
- with tf.Session() as sess:
- coord = tf.train.Coordinator()
- threads = tf.train.start_queue_runners(coord=coord)
- for i in range(10):
- e_val,l_val = sess.run([example_batch,label_batch])
- print e_val,l_val
- coord.request_stop()
- coord.join(threads)
tf.train.batch
与tf.train.shuffle_batch
函数是单个Reader读取,但是可以多线程。tf.train.batch_join
与tf.train.shuffle_batch_join
可设置多Reader读取,每个Reader使用一个线程。至于两种方法的效率,单Reader时,2个线程就达到了速度的极限。多Reader时,2个Reader就达到了极限。所以并不是线程越多越快,甚至更多的线程反而会使效率下降。
5、迭代控制,设置epoch参数,指定我们的样本在训练的时候只能被用多少轮
-
- import tensorflow as tf
- filenames = ['A.csv', 'B.csv', 'C.csv']
-
- filename_queue = tf.train.string_input_producer(filenames, shuffle=False,num_epochs=3)
- reader = tf.TextLineReader()
- key, value = reader.read(filename_queue)
- record_defaults = [['null'], ['null']]
-
- example_list = [tf.decode_csv(value, record_defaults=record_defaults)
- for _ in range(2)]
-
- example_batch, label_batch = tf.train.batch_join(
- example_list, batch_size=1)
-
- init_local_op = tf.initialize_local_variables()
- with tf.Session() as sess:
- sess.run(init_local_op)
- coord = tf.train.Coordinator()
- threads = tf.train.start_queue_runners(coord=coord)
- try:
- while not coord.should_stop():
- e_val,l_val = sess.run([example_batch,label_batch])
- print e_val,l_val
- except tf.errors.OutOfRangeError:
- print('Epochs Complete!')
- finally:
- coord.request_stop()
- coord.join(threads)
- coord.request_stop()
- coord.join(threads)
在迭代控制中,记得添加tf.initialize_local_variables()
,官网教程没有说明,但是如果不初始化,运行就会报错。
=========================================================================================对于传统的机器学习而言,比方说分类问题,[x1 x2 x3]是feature。对于二分类问题,label经过one-hot编码之后就会是[0,1]或者[1,0]。一般情况下,我们会考虑将数据组织在csv文件中,一行代表一个sample。然后使用队列的方式去读取数据
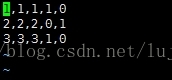
说明:对于该数据,前三列代表的是feature,因为是分类问题,后两列就是经过one-hot编码之后得到的label
使用队列读取该csv文件的代码如下:
-
- import tensorflow as tf
-
- filenames = ['A.csv']
- filename_queue = tf.train.string_input_producer(filenames, shuffle=False)
-
- reader = tf.TextLineReader()
- key, value = reader.read(filename_queue)
-
- record_defaults = [[1], [1], [1], [1], [1]]
- col1, col2, col3, col4, col5 = tf.decode_csv(value,record_defaults=record_defaults)
- features = tf.pack([col1, col2, col3])
- label = tf.pack([col4,col5])
- example_batch, label_batch = tf.train.shuffle_batch([features,label], batch_size=2, capacity=200, min_after_dequeue=100, num_threads=2)
-
- with tf.Session() as sess:
- coord = tf.train.Coordinator()
- threads = tf.train.start_queue_runners(coord=coord)
- for i in range(10):
- e_val,l_val = sess.run([example_batch, label_batch])
- print e_val,l_val
- coord.request_stop()
- coord.join(threads)
输出结果如下:
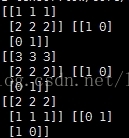
说明:
record_defaults = [[1], [1], [1], [1], [1]]
代表解析的模板,每个样本有5列,在数据中是默认用‘,’隔开的,然后解析的标准是[1],也即每一列的数值都解析为整型。[1.0]就是解析为浮点,['null']解析为string类型
Tensorflow数据读取有三种方式:
- Preloaded data: 预加载数据
- Feeding: Python产生数据,再把数据喂给后端。
- Reading from file: 从文件中直接读取
这三种有读取方式有什么区别呢? 我们首先要知道TensorFlow(TF)是怎么样工作的。
TF的核心是用C++写的,这样的好处是运行快,缺点是调用不灵活。而Python恰好相反,所以结合两种语言的优势。涉及计算的核心算子和运行框架是用C++写的,并提供API给Python。Python调用这些API,设计训练模型(Graph),再将设计好的Graph给后端去执行。简而言之,Python的角色是Design,C++是Run。
一、预加载数据:
1
2
3
4
5
6
7
8
|
import tensorflow as tf
x1 = tf.constant([ 2 , 3 , 4 ])
x2 = tf.constant([ 4 , 0 , 1 ])
y = tf.add(x1, x2)
with tf.Session() as sess:
print sess.run(y)
|
二、python产生数据,再将数据喂给后端
1
2
3
4
5
6
7
8
9
10
11
|
import tensorflow as tf
x1 = tf.placeholder(tf.int16)
x2 = tf.placeholder(tf.int16)
y = tf.add(x1, x2)
li1 = [ 2 , 3 , 4 ]
li2 = [ 4 , 0 , 1 ]
with tf.Session() as sess:
print sess.run(y, feed_dict = {x1: li1, x2: li2})
|
说明:在这里x1, x2只是占位符,没有具体的值,那么运行的时候去哪取值呢?这时候就要用到sess.run()中的feed_dict参数,将Python产生的数据喂给后端,并计算y。
这两种方案的缺点:
1、预加载:将数据直接内嵌到Graph中,再把Graph传入Session中运行。当数据量比较大时,Graph的传输会遇到效率问题。
2、用占位符替代数据,待运行的时候填充数据。
前两种方法很方便,但是遇到大型数据的时候就会很吃力,即使是Feeding,中间环节的增加也是不小的开销,比如数据类型转换等等。最优的方案就是在Graph定义好文件读取的方法,让TF自己去从文件中读取数据,并解码成可使用的样本集。
三、从文件中读取,简单来说就是将数据读取模块的图搭好
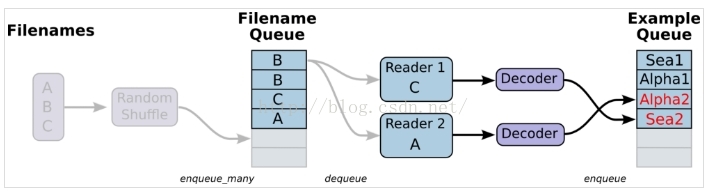
1、准备数据,构造三个文件,A.csv,B.csv,C.csv
1
2
3
|
$ echo - e "Alpha1,A1\nAlpha2,A2\nAlpha3,A3" > A.csv
$ echo - e "Bee1,B1\nBee2,B2\nBee3,B3" > B.csv
$ echo - e "Sea1,C1\nSea2,C2\nSea3,C3" > C.csv
|
2、单个Reader,单个样本
1
2
3
4
5
6
7
8
9
10
11
12
13
14
15
16
17
18
19
|
import tensorflow as tf
filenames = [ 'A.csv' , 'B.csv' , 'C.csv' ]
filename_queue = tf.train.string_input_producer(filenames, shuffle = False )
reader = tf.TextLineReader()
key, value = reader.read(filename_queue)
example, label = tf.decode_csv(value, record_defaults = [[ 'null' ], [ 'null' ]])
with tf.Session() as sess:
coord = tf.train.Coordinator()
threads = tf.train.start_queue_runners(coord = coord)
for i in range ( 10 ):
print example. eval (),label. eval ()
coord.request_stop()
coord.join(threads)
|
说明:这里没有使用tf.train.shuffle_batch,会导致生成的样本和label之间对应不上,乱序了。生成结果如下:
Alpha1 A2
Alpha3 B1
Bee2 B3
Sea1 C2
Sea3 A1
Alpha2 A3
Bee1 B2
Bee3 C1
Sea2 C3
Alpha1 A2
解决方案:用tf.train.shuffle_batch,那么生成的结果就能够对应上。
1
2
3
4
5
6
7
8
9
10
11
12
13
14
15
16
17
18
19
20
|
import tensorflow as tf
filenames = [ 'A.csv' , 'B.csv' , 'C.csv' ]
filename_queue = tf.train.string_input_producer(filenames, shuffle = False )
reader = tf.TextLineReader()
key, value = reader.read(filename_queue)
example, label = tf.decode_csv(value, record_defaults = [[ 'null' ], [ 'null' ]])
example_batch, label_batch = tf.train.shuffle_batch([example,label], batch_size = 1 , capacity = 200 , min_after_dequeue = 100 , num_threads = 2 )
with tf.Session() as sess:
coord = tf.train.Coordinator()
threads = tf.train.start_queue_runners(coord = coord)
for i in range ( 10 ):
e_val,l_val = sess.run([example_batch, label_batch])
print e_val,l_val
coord.request_stop()
coord.join(threads)
|
3、单个Reader,多个样本,主要也是通过tf.train.shuffle_batch来实现
1
2
3
4
5
6
7
8
9
10
11
12
13
14
15
16
17
18
19
20
|
import tensorflow as tf
filenames = [ 'A.csv' , 'B.csv' , 'C.csv' ]
filename_queue = tf.train.string_input_producer(filenames, shuffle = False )
reader = tf.TextLineReader()
key, value = reader.read(filename_queue)
example, label = tf.decode_csv(value, record_defaults = [[ 'null' ], [ 'null' ]])
example_batch, label_batch = tf.train.batch(
[example, label], batch_size = 5 )
with tf.Session() as sess:
coord = tf.train.Coordinator()
threads = tf.train.start_queue_runners(coord = coord)
for i in range ( 10 ):
e_val,l_val = sess.run([example_batch,label_batch])
print e_val,l_val
coord.request_stop()
coord.join(threads)
|
说明:下面这种写法,提取出来的batch_size个样本,特征和label之间也是不同步的
1
2
3
4
5
6
7
8
9
10
11
12
13
14
15
16
17
18
19
|
import tensorflow as tf
filenames = [ 'A.csv' , 'B.csv' , 'C.csv' ]
filename_queue = tf.train.string_input_producer(filenames, shuffle = False )
reader = tf.TextLineReader()
key, value = reader.read(filename_queue)
example, label = tf.decode_csv(value, record_defaults = [[ 'null' ], [ 'null' ]])
example_batch, label_batch = tf.train.batch(
[example, label], batch_size = 5 )
with tf.Session() as sess:
coord = tf.train.Coordinator()
threads = tf.train.start_queue_runners(coord = coord)
for i in range ( 10 ):
print example_batch. eval (), label_batch. eval ()
coord.request_stop()
coord.join(threads)
|
说明:输出结果如下:可以看出feature和label之间是不对应的
['Alpha1' 'Alpha2' 'Alpha3' 'Bee1' 'Bee2'] ['B3' 'C1' 'C2' 'C3' 'A1']
['Alpha2' 'Alpha3' 'Bee1' 'Bee2' 'Bee3'] ['C1' 'C2' 'C3' 'A1' 'A2']
['Alpha3' 'Bee1' 'Bee2' 'Bee3' 'Sea1'] ['C2' 'C3' 'A1' 'A2' 'A3']
4、多个reader,多个样本
1
2
3
4
5
6
7
8
9
10
11
12
13
14
15
16
17
18
19
20
21
|
import tensorflow as tf
filenames = [ 'A.csv' , 'B.csv' , 'C.csv' ]
filename_queue = tf.train.string_input_producer(filenames, shuffle = False )
reader = tf.TextLineReader()
key, value = reader.read(filename_queue)
record_defaults = [[ 'null' ], [ 'null' ]]
example_list = [tf.decode_csv(value, record_defaults = record_defaults)
for _ in range ( 2 )]
example_batch, label_batch = tf.train.batch_join(
example_list, batch_size = 5 )
with tf.Session() as sess:
coord = tf.train.Coordinator()
threads = tf.train.start_queue_runners(coord = coord)
for i in range ( 10 ):
e_val,l_val = sess.run([example_batch,label_batch])
print e_val,l_val
coord.request_stop()
coord.join(threads)
|
tf.train.batch与tf.train.shuffle_batch函数是单个Reader读取,但是可以多线程。tf.train.batch_join与tf.train.shuffle_batch_join可设置多Reader读取,每个Reader使用一个线程。至于两种方法的效率,单Reader时,2个线程就达到了速度的极限。多Reader时,2个Reader就达到了极限。所以并不是线程越多越快,甚至更多的线程反而会使效率下降。
5、迭代控制,设置epoch参数,指定我们的样本在训练的时候只能被用多少轮
1
2
3
4
5
6
7
8
9
10
11
12
13
14
15
16
17
18
19
20
21
22
23
24
25
26
27
28
29
30
31
|
import tensorflow as tf
filenames = [ 'A.csv' , 'B.csv' , 'C.csv' ]
filename_queue = tf.train.string_input_producer(filenames, shuffle = False ,num_epochs = 3 )
reader = tf.TextLineReader()
key, value = reader.read(filename_queue)
record_defaults = [[ 'null' ], [ 'null' ]]
example_list = [tf.decode_csv(value, record_defaults = record_defaults)
for _ in range ( 2 )]
example_batch, label_batch = tf.train.batch_join(
example_list, batch_size = 1 )
init_local_op = tf.initialize_local_variables()
with tf.Session() as sess:
sess.run(init_local_op)
coord = tf.train.Coordinator()
threads = tf.train.start_queue_runners(coord = coord)
try :
while not coord.should_stop():
e_val,l_val = sess.run([example_batch,label_batch])
print e_val,l_val
except tf.errors.OutOfRangeError:
print ( 'Epochs Complete!' )
finally :
coord.request_stop()
coord.join(threads)
coord.request_stop()
coord.join(threads)
|
在迭代控制中,记得添加tf.initialize_local_variables(),官网教程没有说明,但是如果不初始化,运行就会报错。
对于传统的机器学习而言,比方说分类问题,[x1 x2 x3]是feature。对于二分类问题,label经过one-hot编码之后就会是[0,1]或者[1,0]。一般情况下,我们会考虑将数据组织在csv文件中,一行代表一个sample。然后使用队列的方式去读取数据
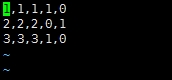
说明:对于该数据,前三列代表的是feature,因为是分类问题,后两列就是经过one-hot编码之后得到的label
使用队列读取该csv文件的代码如下:
1
2
3
4
5
6
7
8
9
10
11
12
13
14
15
16
17
18
19
20
21
22
23
|
import tensorflow as tf
filenames = [ 'A.csv' ]
filename_queue = tf.train.string_input_producer(filenames, shuffle = False )
reader = tf.TextLineReader()
key, value = reader.read(filename_queue)
record_defaults = [[ 1 ], [ 1 ], [ 1 ], [ 1 ], [ 1 ]]
col1, col2, col3, col4, col5 = tf.decode_csv(value,record_defaults = record_defaults)
features = tf.pack([col1, col2, col3])
label = tf.pack([col4,col5])
example_batch, label_batch = tf.train.shuffle_batch([features,label], batch_size = 2 , capacity = 200 , min_after_dequeue = 100 , num_threads = 2 )
with tf.Session() as sess:
coord = tf.train.Coordinator()
threads = tf.train.start_queue_runners(coord = coord)
for i in range ( 10 ):
e_val,l_val = sess.run([example_batch, label_batch])
print e_val,l_val
coord.request_stop()
coord.join(threads)
|
输出结果如下:
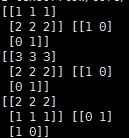
说明:
1
|
record_defaults = [[ 1 ], [ 1 ], [ 1 ], [ 1 ], [ 1 ]]
|
代表解析的模板,每个样本有5列,在数据中是默认用‘,'隔开的,然后解析的标准是[1],也即每一列的数值都解析为整型。[1.0]就是解析为浮点,['null']解析为string类型
二、此处给出了几种不同的next_batch方法,该文章只是做出代码片段的解释,以备以后查看:
1
2
3
4
5
6
7
8
9
10
11
12
13
14
15
16
17
18
19
20
21
22
23
24
25
26
27
|
def next_batch( self , batch_size, fake_data = False ):
if fake_data:
fake_image = [ 1 ] * 784
if self .one_hot:
fake_label = [ 1 ] + [ 0 ] * 9
else :
fake_label = 0
return [fake_image for _ in xrange (batch_size)], [
fake_label for _ in xrange (batch_size)
]
start = self ._index_in_epoch
self ._index_in_epoch + = batch_size
if self ._index_in_epoch > self ._num_examples:
self ._epochs_completed + = 1
perm = numpy.arange( self ._num_examples)
numpy.random.shuffle(perm)
self ._images = self ._images[perm]
self ._labels = self ._labels[perm]
start = 0
self ._index_in_epoch = batch_size
assert batch_size < = self ._num_examples
end = self ._index_in_epoch
return self ._images[start:end], self ._labels[start:end]
|
该段代码摘自mnist.py文件,从代码第12行start = self._index_in_epoch开始解释,_index_in_epoch-1是上一次batch个图片中最后一张图片的下边,这次epoch第一张图片的下标是从 _index_in_epoch开始,最后一张图片的下标是_index_in_epoch+batch, 如果 _index_in_epoch 大于语料中图片的个数,表示这个epoch是不合适的,就算是完成了语料的一遍的遍历,所以应该对图片洗牌然后开始新一轮的语料组成batch开始
1
2
3
4
5
6
7
8
9
10
11
12
13
14
15
16
17
18
19
20
21
22
23
24
25
26
27
28
29
30
31
32
33
34
35
36
|
def ptb_iterator(raw_data, batch_size, num_steps):
raw_data = np.array(raw_data, dtype = np.int32)
data_len = len (raw_data)
batch_len = data_len / / batch_size
data = np.zeros([batch_size, batch_len], dtype = np.int32)
for i in range (batch_size):
data[i] = raw_data[batch_len * i:batch_len * (i + 1 )]
epoch_size = (batch_len - 1 ) / / num_steps
if epoch_size = = 0 :
raise ValueError( "epoch_size == 0, decrease batch_size or num_steps" )
for i in range (epoch_size):
x = data[:, i * num_steps:(i + 1 ) * num_steps]
y = data[:, i * num_steps + 1 :(i + 1 ) * num_steps + 1 ]
yield (x, y)
|
第三种方式:
1
2
3
4
5
6
7
8
9
10
11
12
13
|
def next ( self , batch_size):
if self .batch_id = = len ( self .data):
self .batch_id = 0
batch_data = ( self .data[ self .batch_id: min ( self .batch_id +
batch_size, len ( self .data))])
batch_labels = ( self .labels[ self .batch_id: min ( self .batch_id +
batch_size, len ( self .data))])
batch_seqlen = ( self .seqlen[ self .batch_id: min ( self .batch_id +
batch_size, len ( self .data))])
self .batch_id = min ( self .batch_id + batch_size, len ( self .data))
return batch_data, batch_labels, batch_seqlen
|
第四种方式:
1
2
3
4
5
6
7
8
9
10
11
12
13
14
15
16
17
|
def batch_iter(sourceData, batch_size, num_epochs, shuffle = True ):
data = np.array(sourceData)
data_size = len (sourceData)
num_batches_per_epoch = int ( len (sourceData) / batch_size) + 1
for epoch in range (num_epochs):
if shuffle:
shuffle_indices = np.random.permutation(np.arange(data_size))
shuffled_data = sourceData[shuffle_indices]
else :
shuffled_data = sourceData
for batch_num in range (num_batches_per_epoch):
start_index = batch_num * batch_size
end_index = min ((batch_num + 1 ) * batch_size, data_size)
yield shuffled_data[start_index:end_index]
|
迭代器的用法,具体学习Python迭代器的用法
另外需要注意的是,前三种方式只是所有语料遍历一次,而最后一种方法是,所有语料遍历了num_epochs次