【机器学习算法-python实现】扫黄神器-朴素贝叶斯分类器的实现
(转载请注明出处:http://blog.csdn.net/buptgshengod)
1.背景
嗯,如今最终用到了。朴素贝叶斯分类器据说是好多扫黄软件使用的算法。贝叶斯公式也比較简单,大学做概率题常常会用到。核心思想就是找出特征值对结果影响概率最大的项。
公式例如以下:
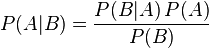
2.数据集
[['my','dog','has','flea','problems','help','please'], 0
['maybe','not','take','him','to','dog','park','stupid'], 1
['my','dalmation','is','so','cute','I','love','him'], 0
['stop','posting','stupid','worthless','garbage'], 1
['mr','licks','ate','my','steak','how','to','stop','him'], 0
['quit','buying','worthless','dog','food','stupid']] 1
我们通过分析每一个句子中的每一个词,在粗口句或是正常句出现的概率,能够找出那些词是粗口。
3.代码
#以矩阵形式创建数据集
def loadDataSet():
postingList=[['my', 'dog', 'has', 'flea', 'problems', 'help', 'please'],
['maybe', 'not', 'take', 'him', 'to', 'dog', 'park', 'stupid'],
['my', 'dalmation', 'is', 'so', 'cute', 'I', 'love', 'him'],
['stop', 'posting', 'stupid', 'worthless', 'garbage'],
['mr', 'licks', 'ate', 'my', 'steak', 'how', 'to', 'stop', 'him'],
['quit', 'buying', 'worthless', 'dog', 'food', 'stupid']]
classVec = [0,1,0,1,0,1] #1 is abusive, 0 not
return postingList,classVec
#将矩阵内容加入到列表,set获取list中不反复的元素
def createVocabList(dataSet):
vocabSet = set([]) #create empty set
for document in dataSet:
vocabSet = vocabSet | set(document) #union of the two sets
return list(vocabSet)
#推断list中每一个词在总共词语list中的位置
def setOfWords2Vec(vocabList, inputSet):
returnVec = [0]*len(vocabList)
for word in inputSet:
if word in vocabList:
returnVec[vocabList.index(word)] = 1
else: print "the word: %s is not in my Vocabulary!" % word
return returnVec
def trainNB0(trainMatrix,trainCategory):
numTrainDocs = len(trainMatrix)
numWords = len(trainMatrix[0])
pAbusive = sum(trainCategory)/float(numTrainDocs)#脏句的比例
p0Num = zeros(numWords); p1Num = zeros(numWords) #zero是numpy带的函数。zeros(i)长度为i的list
p0Denom = 0.0; p1Denom = 0.0
for i in range(numTrainDocs):
if trainCategory[i] == 1:#假设是粗口句,每一个词在p1num加一
p1Num += trainMatrix[i]
p1Denom += sum(trainMatrix[i])
else:
p0Num += trainMatrix[i]
p0Denom += sum(trainMatrix[i])
p1Vect = p1Num/p1Denom #粗口字概率
p0Vect = p0Num/p0Denom
return p0Vect,p1Vect,pAbusive
实现效果:
[ 0. 0. 0. 0.05263158 0.05263158 0. 0.
0. 0.05263158 0.05263158 0. 0. 0.
0.05263158 0.05263158 0.05263158 0.05263158 0.05263158 0.
0.10526316 0. 0.05263158 0.05263158 0. 0.10526316
0. 0.15789474 0. 0.05263158 0. 0. 0. ]
出现概率最大项:
0.157894736842
相应的词是:stupid
['cute', 'love', 'help', 'garbage', 'quit', 'I', 'problems', 'is', 'park', 'stop', 'flea', 'dalmation', 'licks', 'food', 'not', 'him', 'buying', 'posting', 'has', 'worthless', 'ate', 'to', 'maybe', 'please', 'dog', 'how', 'stupid', 'so', 'take', 'mr', 'steak', 'my']
4.下载
posted on 2019-05-04 19:04 xfgnongmin 阅读(255) 评论(0) 编辑 收藏 举报