数据挖掘基础
目录
- 二.Numpy
- 三、Pandas高级处理 1
一 、Matplotlib
Matplotlib 三层结构
-
1.容器层 : 画板层 画布层plt.figure()
绘图区/坐标系axes: plt.subplots() x、y轴张成的区域
- 2.辅助显示层 横纵坐标,网格等 辅助显示
- 3.图像层 指的是不同的同,如折线图,柱状图等
注:2 , 3 置于 1 之上
1.常见图表的使用
-
折线图 (plot)
- 适用于连续数据 变量之间随时间变化趋势
-
散点图 (scatter)
- 适用于离散数据 变量之间是否存在数量关联趋势 关系/规律
-
柱状图 (bar)
- 适用于离散数据 统计和比较数据之间的差别 统计/对比
-
直方图 (histogram)
- 适用于连续数据 展示一组或者多组数据的分布 分布状况
-
饼图(pie)
- 适用于分类数据 展示不同分类的占比情况 占比
import matplotlib.pyplot as plt
import random
# # 显示中文绘图
plt.rcParams['font.sans-serif'] = ['SimHei'] # 用来正常显示中文标签
plt.rcParams['axes.unicode_minus'] = False
2.画法一
# 需求:画出城市11点到23点1小时内每分钟的温度变化折线图,温度范围在15度-18度
import matplotlib.pyplot as plt
import random
# 1.准备数据 x y
x = range(60)
y_shanghai = [random.uniform(15,18) for i in x]
y_beijing = [random.uniform(1,3) for i in x]
# 2.创建画布
plt.figure(figsize=(15,5),dpi=80)
# 3.绘制图像
# 颜色字符 r g b w c m y k 风格字符 - -- -. : ''
plt.plot(x,y_shanghai,color="r",linestyle = "--",label="上海")
plt.plot(x,y_beijing,color="b",linestyle="-",label="北京")
#显示图例 ---》label ="上海" ,label = "北京"
#位置 0 1 2 3 4 5 6 7 8 9
#位置 upper low center + left right / best
# plt.legend(loc = 0)
plt.legend(loc="upper right")
#修改x,y刻度值
#准备x的刻度说明
x_lable = ["11点{}分".format(i) for i in x]
plt.xticks(x[::5],x_lable[::5])
plt.yticks(range(0,41,5))
#添加网格显示
plt.grid(linestyle="--",alpha=0.5)
#添加描述信息
plt.xlabel("时间变化")
plt.ylabel("温度变化")
plt.title("上海、北京11点到12点的温度变化状况")
# 4.显示图像
plt.show()
3.画法二
# 需求:将北京、上海 在同一画布上,显示不同的图
# 1.准备数据 x y
x = range(60)
y_shanghai = [random.uniform(15,18) for i in x ]
y_beijing = [random.uniform(1,3) for i in x ]
# 2.创建画布
plt.figure(figsize=(10,5),dpi=50)
#一行两列
figure , axes = plt.subplots(nrows = 1 ,ncols = 2
,figsize=(10,5),dpi=80)
# 3.绘制图像
axes[0].plot(x,y_shanghai,color = "r",linestyle="--",label="上海")
axes[1].plot(x,y_beijing,color="b",linestyle="-",label = "北京")
#显示图例 ---》label ="上海" ,label = "北京"
#位置 0 1 2 3 4 5 6 7 8 9
#位置 upper low center + left right / best
# plt.legend(loc = 0)
axes[0].legend(loc="upper right")
axes[1].legend(loc=0)
#添加网格显示
axes[0].grid(linestyle="--",alpha=0.3)
axes[1].grid(linestyle="-",alpha = 0.4)
# 添加刻度
x_lable = ["12:{}".format(i) for i in x ]
axes[0].set_xticks(x[::5],x_lable[::5])
axes[0].set_yticks(range(0,41,5))
axes[1].set_xticks(x[::5],x_lable[::5])
axes[1].set_yticks(range(0,41,5))
# 添加描述信息
axes[0].set_xlabel("时间变化")
axes[0].set_ylabel("温度变化")
axes[0].set_title("上海12点到13点的温度变化状况")
axes[1].set_xlabel("时间变化")
axes[1].set_ylabel("温度变化")
axes[1].set_title("北京12点到13点的温度变化状况")
plt.show()
<Figure size 500x250 with 0 Axes>
import numpy as np
4.绘制数学函数图像
# 1.准备 x,y数据
x = np.linspace(-1,1,1000)
y = 2 * x * x
# 2.创建画布
plt.figure(figsize=(20, 8), dpi=80)
# 3、绘制图像
plt.plot(x,y,color = "g",linestyle="-",label="抛物线")
plt.legend(loc=0)
# 添加网格信息
plt.grid(linestyle="--",alpha=0.5)
# 添加刻度
x_label = ["x={}".format(i) for i in x]
plt.xticks(x[::100],x_label[::100])
plt.yticks(y[::100])
# 添加描述信息
plt.xlabel("x自变量")
plt.ylabel("y变量")
plt.title("抛物线变化情况")
# 4显示图像
plt.show()
5.散点图绘制
# 需求:探究房屋面积和房屋价格的关系
# 1、准备数据
x = [225.98, 247.07, 253.14, 457.85, 241.58, 301.01, 20.67, 288.64,
163.56, 120.06, 207.83, 342.75, 147.9 , 53.06, 224.72, 29.51,
21.61, 483.21, 245.25, 399.25, 343.35]
y = [196.63, 203.88, 210.75, 372.74, 202.41, 247.61, 24.9 , 239.34,
140.32, 104.15, 176.84, 288.23, 128.79, 49.64, 191.74, 33.1 ,
30.74, 400.02, 205.35, 330.64, 283.45]
# 2、创建画布
plt.figure(figsize=(10,5),dpi=50)
# 3、绘制图像
plt.scatter(x,y,color="r")
# 4、显示图像
plt.show()
5.综合案例
需求1-对比每部电影的票房收入----单直方图
# 1、准备数据
movie_names = ['雷神3:诸神黄昏','正义联盟','东方快车谋杀案','寻梦环游记','全球风暴', '降魔传','追捕','七十七天','密战','狂兽','其它']
tickets = [73853,57767,22354,15969,14839,8725,8716,8318,7916,6764,52222]
# 2、创建画布
plt.figure(figsize=(20,8),dpi=80)
# 3、绘制柱状图
x_ticks = range(len(movie_names))
plt.bar(x_ticks,tickets,color=['b','r','g','y','c','m','y','k','c','g','b'])
#修改刻度
plt.xticks(x_ticks,movie_names)
# 添加标题
plt.title("电影票房收入对比")
# 添加网格
plt.grid(linestyle="--",alpha=0.8)
# 4、显示图像
plt.show()
需求2-如何对比电影票房收入才更能加有说服力?---双直方图
# 1、准备数据
movie_name = ['雷神3:诸神黄昏','正义联盟','寻梦环游记']
first_day = [10587.6,10062.5,1275.7]
first_weekend=[36224.9,34479.6,11830]
# 2、创建画布
plt.figure(figsize=(10,5),dpi=80)
# 3、绘制柱状图
plt.bar(range(3),first_day,color="r",label="首日票房",width=0.2)
plt.bar([0.2,1.2,2.2],first_weekend,width=0.2,label="首周票房")
# 显示图例
plt.legend()
# 修改刻度
plt.xticks([0.1,1.1,2.1],movie_name)
plt.show()
6.直方图绘制
# 需求:电影时长分布状况
# 1、准备数据
time = [131, 98, 125, 131, 124, 139, 131, 117, 128, 108, 135, 138, 131, 102, 107, 114, 119, 128, 121, 142, 127, 130, 124, 101, 110, 116, 117, 110, 128, 128, 115, 99, 136, 126, 134, 95, 138, 117, 111,78, 132, 124, 113, 150, 110, 117, 86, 95, 144, 105, 126, 130,126, 130, 126, 116, 123, 106, 112, 138, 123, 86, 101, 99, 136,123, 117, 119, 105, 137, 123, 128, 125, 104, 109, 134, 125, 127,105, 120, 107, 129, 116, 108, 132, 103, 136, 118, 102, 120, 114,105, 115, 132, 145, 119, 121, 112, 139, 125, 138, 109, 132, 134,156, 106, 117, 127, 144, 139, 139, 119, 140, 83, 110, 102,123,107, 143, 115, 136, 118, 139, 123, 112, 118, 125, 109, 119, 133,112, 114, 122, 109, 106, 123, 116, 131, 127, 115, 118, 112, 135,115, 146, 137, 116, 103, 144, 83, 123, 111, 110, 111, 100, 154,136, 100, 118, 119, 133, 134, 106, 129, 126, 110, 111, 109, 141,120, 117, 106, 149, 122, 122, 110, 118, 127, 121, 114, 125, 126,114, 140, 103, 130, 141, 117, 106, 114, 121, 114, 133, 137, 92,121, 112, 146, 97, 137, 105, 98, 117, 112, 81, 97, 139, 113,134, 106, 144, 110, 137, 137, 111, 104, 117, 100, 111, 101, 110,105, 129, 137, 112, 120, 113, 133, 112, 83, 94, 146, 133, 101,131, 116, 111, 84, 137, 115, 122, 106, 144, 109, 123, 116, 111,111, 133, 150]
# 2、创建画布
plt.figure(figsize=(20, 8), dpi=80)
# 3、绘制直方图
distance = 2
group_num = int((max(time) - min(time)) / distance)
plt.hist(time, bins=group_num, density=True)
# 修改x轴刻度
plt.xticks(range(min(time), max(time) + 2, distance))
# 添加网格
plt.grid(linestyle="--", alpha=0.5)
# 4、显示图像
plt.show()
# 1、准备数据
movie_name = ['雷神3:诸神黄昏','正义联盟','东方快车谋杀案','寻梦环游记','全球风暴','降魔传','追捕','七十七天','密战','狂兽','其它']
place_count = [60605,54546,45819,28243,13270,9945,7679,6799,6101,4621,20105]
# 2、创建画布
plt.figure(figsize=(20, 8), dpi=80)
# 3、绘制饼图
plt.pie(place_count, labels=movie_name, colors=['b','r','g','y','c','m','y','k','c','g','y'], autopct="%1.3f%%")
# 显示图例
plt.legend()
plt.axis('equal')
# 4、显示图像
plt.show()
二.Numpy
- 科学数据库
- ndarray ---- n维数组
import numpy as np
score = np.array([[80, 89, 86, 67, 79],
[78, 97, 89, 67, 81],
[90, 94, 78, 67, 74],
[91, 91, 90, 67, 69],
[76, 87, 75, 67, 86],
[70, 79, 84, 67, 84],
[94, 92, 93, 67, 64],
[86, 85, 83, 67, 80]])
score
----result----------
array([[80, 89, 86, 67, 79],
[78, 97, 89, 67, 81],
[90, 94, 78, 67, 74],
[91, 91, 90, 67, 69],
[76, 87, 75, 67, 86],
[70, 79, 84, 67, 84],
[94, 92, 93, 67, 64],
[86, 85, 83, 67, 80]])
type(score)
----result----------
numpy.ndarray
1.ndarray与Python原生list运算效率对比
- 具有相同数据类型的多维数组
- 底层是c封装,运行速度快
- 存储是紧凑型,占有空间少
import random
import time
# 生成一个大数组
python_list = []
for i in range(100000000):
python_list.append(random.random())
python_list = np.array(python_list)
python_list
----result----------
array([0.23401045, 0.59441637, 0.58932156, ..., 0.94788828, 0.27280735,
0.44378139])
# 原生Python list 求和
t1 = time.time()
a = sum(python_list)
t2 = time.time()
d1 = t2 -t1
# ndarray 求和
t3 = time.time()
b = np.sum(python_list)
t4 = time.time()
d2 = t4 -t3
d1
----result----------
6.524392366409302
d2
----result----------
0.11269235610961914
2. naarray属性
# shape(数组维度的元组)
# ndim(数组维数)
# size(数组中的元素数量)
# dtype(一个数组元素的长度(字节))
# itemesize(数组元素的类型)
score
----result----------
array([[80, 89, 86, 67, 79],
[78, 97, 89, 67, 81],
[90, 94, 78, 67, 74],
[91, 91, 90, 67, 69],
[76, 87, 75, 67, 86],
[70, 79, 84, 67, 84],
[94, 92, 93, 67, 64],
[86, 85, 83, 67, 80]])
# shape(数组维度的元组)
score.shape # 8行5列
----result----------
(8, 5)
# ndim(数组维数)
score.ndim # 二维数组
----result----------
2
# size(数组中的元素数量)
score.size # 数组中有40个元素
----result----------
40
# dtype(一个数组元素的长度(字节))
score.dtype # 数据类型是 int32
----result----------
dtype('int32')
# itemesize(数组元素的类型)
score.itemsize
----result----------
4
2.1 ndarray的形状
a = np.array([[1,2,3],[4,5,6]])
b = np.array([1,2,3,4])
c = np.array([[[1,2,3],[4,5,6]],[[1,2,3],[4,5,6]]])
# 二维数组
a
----result----------
array([[1, 2, 3],
[4, 5, 6]])
a.shape
----result----------
(2, 3)
# 一维数组
b
----result----------
array([1, 2, 3, 4])
b.shape
----result----------
(4,)
# 三维数组
c
----result----------
array([[[1, 2, 3],
[4, 5, 6]],
[[1, 2, 3],
[4, 5, 6]]])
c.shape
----result----------
(2, 2, 3)
2.2 ndarray的类型
data = np.array([1.1,2.2,3.3])
data.dtype
----result----------
dtype('float64')
# 创建数组的时候指定类型
data1 = np.array([1.1,2.2,3.3],dtype=np.float32)
data1.dtype
----result----------
dtype('float32')
3.ndarray的基本操作
3.1生成0和1的数组
# 生成0和1的数组
np.zeros(shape=(3,4),dtype="float32")
----result----------
array([[0., 0., 0., 0.],
[0., 0., 0., 0.],
[0., 0., 0., 0.]], dtype=float32)
np.ones(shape=(4,3),dtype="int32")
----result----------
array([[1, 1, 1],
[1, 1, 1],
[1, 1, 1],
[1, 1, 1]])
3.2从现有数组中生成
score
----result----------
array([[80, 89, 86, 67, 79],
[78, 97, 89, 67, 81],
[90, 94, 78, 67, 74],
[91, 91, 90, 67, 69],
[76, 87, 75, 67, 86],
[70, 79, 84, 67, 84],
[94, 92, 93, 67, 64],
[86, 85, 83, 67, 80]])
# 深拷贝(常用) 直接开辟一块新空间,元素的数值改变不受影响
data_deepcopy = np.array(score,dtype="float64")
data_deepcopy
----result----------
array([[80., 89., 86., 67., 79.],
[78., 97., 89., 67., 81.],
[90., 94., 78., 67., 74.],
[91., 91., 90., 67., 69.],
[76., 87., 75., 67., 86.],
[70., 79., 84., 67., 84.],
[94., 92., 93., 67., 64.],
[86., 85., 83., 67., 80.]])
# 浅拷贝 只是复制索引,当原数据改变的时候,索引的相应位置也会改变
data_copy = np.asarray(score)
data_copy
----result----------
array([[80, 89, 86, 67, 79],
[78, 97, 89, 67, 81],
[90, 94, 78, 67, 74],
[91, 91, 90, 67, 69],
[76, 87, 75, 67, 86],
[70, 79, 84, 67, 84],
[94, 92, 93, 67, 64],
[86, 85, 83, 67, 80]])
score[3,1] = 10000
score
----result----------
array([[ 80, 89, 86, 67, 79],
[ 78, 97, 89, 67, 81],
[ 90, 94, 78, 67, 74],
[ 91, 10000, 90, 67, 69],
[ 76, 87, 75, 67, 86],
[ 70, 79, 84, 67, 84],
[ 94, 92, 93, 67, 64],
[ 86, 85, 83, 67, 80]])
data_deepcopy
----result----------
array([[80., 89., 86., 67., 79.],
[78., 97., 89., 67., 81.],
[90., 94., 78., 67., 74.],
[91., 91., 90., 67., 69.],
[76., 87., 75., 67., 86.],
[70., 79., 84., 67., 84.],
[94., 92., 93., 67., 64.],
[86., 85., 83., 67., 80.]])
data_copy
----result----------
array([[ 80, 89, 86, 67, 79],
[ 78, 97, 89, 67, 81],
[ 90, 94, 78, 67, 74],
[ 91, 10000, 90, 67, 69],
[ 76, 87, 75, 67, 86],
[ 70, 79, 84, 67, 84],
[ 94, 92, 93, 67, 64],
[ 86, 85, 83, 67, 80]])
3.3生成固定范围的数组
np.linspace(0,10,4) # 范围[0,10] 4个等距离数
----result----------
array([ 0. , 3.33333333, 6.66666667, 10. ])
np.arange(0,10,4) #范围[0,10) 步长为4
----result----------
array([0, 4, 8])
3.4生成随机数组
import matplotlib.pyplot as plt
# 均匀分布 最低为 -1 最高为 1 数量为1000000
data1 = np.random.uniform(-1,1,1000000)
# 1、创建画布
plt.figure(figsize=(20, 8), dpi=80)
# 2、绘制直方图
plt.hist(data1, 1000)
# 3、显示图像
plt.show()
# 正态分布 loc -> 均值 0 scale -> 标准差 1 size 数据个数 10000
data2 = np.random.normal(0,1,1000000)
# 1、创建画布
plt.figure(figsize=(20, 8), dpi=80)
# 2、绘制直方图
plt.hist(data2, 1000)
# 3、显示图像
plt.show()
案例:随机生成8只股票两周的交易日涨幅数据
# 生成数组
#使用正态分布生成数据 按照 8 行 10列
stock_change = np.random.normal( 0,1,(8,10))
stock_chang
----result----------
array([[ 0.78322177, 0.507861 , 1.85003005, 1.19323072, 0.81847297,
-0.63094271, -0.57060276, 0.30855245, 1.94698162, -0.88928573],
[ 0.06893518, -0.40478084, -1.65714139, -0.81447523, 0.64866496,
0.39132671, 0.04355432, 0.0446165 , -0.51806464, 0.43098731],
[ 0.16193423, -0.59461836, 0.09053752, -0.45878007, -0.09133374,
-0.10657109, -0.41976839, -0.76897636, -0.89682933, 0.44727093],
[-0.21787426, -0.70705768, 0.14948141, 1.88738647, -2.41193373,
-0.86842017, 1.51809276, 0.39750412, 1.13165355, -0.18717137],
[-1.9212302 , -0.85526221, -0.68535196, -1.8369455 , 2.30827726,
0.58769356, -1.29491169, 0.70073049, 1.36375486, 0.31760057],
[ 0.88214854, 0.81825296, 1.70235699, -0.85743748, 1.67908554,
0.02161739, 1.49909361, -1.43709353, -0.0857922 , 1.09128374],
[-1.48763567, -0.71125736, 0.20389856, -0.10618373, -0.09510078,
-1.16947122, -1.09477064, 1.45625879, -0.93677276, 1.41664427],
[-1.36546057, 0.27390585, -2.00117963, 0.48013552, -1.37752954,
1.1236394 , -0.82104285, 0.63678403, 0.23191035, 0.44840676]])
4.数组的索引、切片
# 获取第一个股票的前三个交易日的涨跌幅数据
# TODO 对二维数据如何切片? 第一个参数是第几行,第二参数是第几列
# [ : , : ] 0 代表二维数组第一个数组 :3 这个时候是一维数组 中前三个
stock_change [0,:3]
----result----------
array([0.78322177, 0.507861 , 1.85003005])
stock_change [0,:3]
----result----------
array([0.78322177, 0.507861 , 1.85003005])
a1 = np.array([[[1,2,3],[4,5,6]],[[12,3,34],[5,6,7]]])
# 使用索引定位到34
a1[1,0,2]
----result----------
34
# TODO 需求:让刚才的股票行,日期列放过来,变成日期行,股票
5.数据形状的修改
5.1 reshape
# reshape 并没有把行列进行转换,只是将数组的形状进行重新划分 ,
stock_change.shape
----result----------
(8, 10)
stock_change.reshape((10,8)).shape
----result----------
(10, 8)
5.2 resize
# resize 没有返回值,只是对原始数据进行修改
stock_change.resize((10,8))
stock_change.shape
----result----------
(10, 8)
5.3 T 转置
# 使用转置,将原本数据的行列 进行列行的转换
stock_change.T
----result----------
array([[ 0.78322177, 1.94698162, 0.04355432, -0.09133374, 0.14948141,
-1.9212302 , 1.36375486, 1.49909361, -0.09510078, -2.00117963],
[ 0.507861 , -0.88928573, 0.0446165 , -0.10657109, 1.88738647,
-0.85526221, 0.31760057, -1.43709353, -1.16947122, 0.48013552],
[ 1.85003005, 0.06893518, -0.51806464, -0.41976839, -2.41193373,
-0.68535196, 0.88214854, -0.0857922 , -1.09477064, -1.37752954],
[ 1.19323072, -0.40478084, 0.43098731, -0.76897636, -0.86842017,
-1.8369455 , 0.81825296, 1.09128374, 1.45625879, 1.1236394 ],
[ 0.81847297, -1.65714139, 0.16193423, -0.89682933, 1.51809276,
2.30827726, 1.70235699, -1.48763567, -0.93677276, -0.82104285],
[-0.63094271, -0.81447523, -0.59461836, 0.44727093, 0.39750412,
0.58769356, -0.85743748, -0.71125736, 1.41664427, 0.63678403],
[-0.57060276, 0.64866496, 0.09053752, -0.21787426, 1.13165355,
-1.29491169, 1.67908554, 0.20389856, -1.36546057, 0.23191035],
[ 0.30855245, 0.39132671, -0.45878007, -0.70705768, -0.18717137,
0.70073049, 0.02161739, -0.10618373, 0.27390585, 0.44840676]])
6.类型修改 astype 、tostring
6.1 astype 转换数据类型
stock_change.astype("int32").dtype
----result----------
dtype('int32')
6.2 tostring 序列化到本地
stock_change.tostring()
C:\Users\wyk15\AppData\Local\Temp\ipykernel_9792\2974151914.py:1: DeprecationWarning: tostring() is deprecated. Use tobytes() instead.
stock_change.tostring(
b'\'hJ\x17\'\x10\xe9?\xce9\xde\xb3e@\xe0?!\xf6i\x1b\xb9\x99\xfd?\x97\xa6\xb5\x18y\x17\xf3?\x97b7;\xee0\xea?{5\x9b\xc1\xae0\xe4\xbfqx\xf3\xb9`B\xe2\xbf\xdb\x0f\x11\xc8R\xbf\xd3??\x96\x184\xd6&\xff?\xb5\xdc\x96V\x07u\xec\xbf3\x01X{\xbc\xa5\xb1? ......................
7.数组的去重 set 、 unique
7.1 set
set([1,2,3,4,3,2])
----result----------
{1, 2, 3, 4}
temp = np.array([[1,2,3,4],[3,4,5,6]])
# set(temp)
# unhashable type: 'numpy.ndarray'
set(temp.flatten())
----result----------
{1, 2, 3, 4, 5, 6}
7.2 unique
np.unique(temp)
----result----------
array([1, 2, 3, 4, 5, 6])
8.ndarray运算
8.1 逻辑运算
8.1.1 逻辑判断 ---布尔索引 data[ 判断条件 ]
stock_change = np.random.normal(loc=0,scale=1,size=(8,10))
stock_change
----result----------
array([[-0.0245709 , 0.68795842, -0.95063999, -0.53223192, 1.303397 ,
-0.06299074, -0.95125381, -0.82551705, 0.42587723, -0.11330232],
[-0.23024816, -0.41551718, -0.01701004, 0.25067482, -0.63199918,
0.76156926, -1.41413847, 1.71607016, 1.40861841, 0.56333108],
[-0.33762242, -0.9108106 , -0.57667507, -1.71265711, -0.14329169,
-0.42219945, -1.30530528, -1.66632015, 0.66312763, -0.15927883],
[ 0.95633189, -0.76190147, 3.62513955, 0.79395741, -1.05363437,
-1.19484002, 1.05985014, -0.34990448, 0.5154208 , -0.61577867],
[ 1.62141953, -0.45131205, 1.22414137, -0.12214553, -0.94923934,
0.07767484, 0.30012161, -0.78144416, -0.5917796 , 0.42159082],
[ 0.77102353, -0.66785462, -0.18199085, 0.51546877, 0.89751432,
-1.20205727, -1.22355634, 0.9701325 , 0.66979969, -1.31552754],
[ 1.48046995, 0.76282364, -1.00391509, 2.32600983, 0.37431788,
-0.54506338, 0.44654218, -0.26642661, -1.0385316 , -0.29532037],
[ 0.32563363, 0.73159911, -0.6522114 , 0.0418548 , -0.02176352,
-0.34409397, -0.59366906, 0.25135939, 1.4580353 , 0.29256462]])
# 布尔索引 逻辑判断,如果过涨跌幅大于0.5就标记为True 否则为False
stock_change > 0.5
----result----------
array([[False, True, False, False, True, False, False, False, False,
False],
[False, False, False, False, False, True, False, True, True,
True],
[False, False, False, False, False, False, False, False, True,
False],
[ True, False, True, True, False, False, True, False, True,
False],
[ True, False, True, False, False, False, False, False, False,
False],
[ True, False, False, True, True, False, False, True, True,
False],
[ True, True, False, True, False, False, False, False, False,
False],
[False, True, False, False, False, False, False, False, True,
False]])
# 布尔索引 把逻辑判断节点,放在索引中,可以获取满足条件的数据
stock_change[stock_change>0.5] = 1.1
stock_change[stock_change>0.5]
----result----------
array([1.1, 1.1, 1.1, 1.1, 1.1, 1.1, 1.1, 1.1, 1.1, 1.1, 1.1, 1.1, 1.1,
1.1, 1.1, 1.1, 1.1, 1.1, 1.1, 1.1, 1.1, 1.1, 1.1, 1.1])
8.1.2 通用判断函数 all 、 any
# 通用判断函数 判断stock_change[0:2,0:5] 是否全是上涨的
stock_change[0:2,0:5] > 0
----result----------
array([[False, True, False, False, True],
[False, False, False, True, False]])
# np.all() 判断条件是 是否索引判断中都满足条件 即都返回true
# 若满足则为 true 若不满足则为false
np.all(stock_change[0:2,0:5] > 0)
----result----------
False
# np.any() 判断条件是 索引中是否有满足条件 即都返回true
np.any(stock_change[0:2,0:5] > 0 )
----result----------
True
8.2 三元运算符 where
# 判断前四个股票前四天的涨跌幅 大于的置为1 ,否为为0
temp = stock_change[:4,:4]
temp
----result----------
array([[-0.0245709 , 1.1 , -0.95063999, -0.53223192],
[-0.23024816, -0.41551718, -0.01701004, 0.25067482],
[-0.33762242, -0.9108106 , -0.57667507, -1.71265711],
[ 1.1 , -0.76190147, 1.1 , 1.1 ]])
temp>0
----result----------
array([[False, True, False, False],
[False, False, False, True],
[False, False, False, False],
[ True, False, True, True]])
# np.where 进行三元运算,并见满足条件的值置为 1 不满足条件的置为 0
np.where(temp > 0 , 1 ,0 )
----result----------
array([[0, 1, 0, 0],
[0, 0, 0, 1],
[0, 0, 0, 0],
[1, 0, 1, 1]])
8.3 符合逻辑需要结合np.logical_and 和 np.logical_or 使用
# 判断 前四个股票前四天的涨跌幅 大于0.5并且小于1的,换为1,否则为0
# 判断 前四个股票前四天的涨跌幅 大于0.5或者小于-0.5 , 换位1 ,否则为0
# temp > 0.5 and temp < 1
# ---------------------------------------------------------------------------
# ValueError Traceback (most recent call last)
# Input In [134], in <cell line: 1>()
# ----> 1 temp > 0.5 and temp < 1
# ValueError: The truth value of an array with more
# than one element is ambiguous. Use a.any() or a.all()
# 判断 前四个股票前四天的涨跌幅 大于0.5并且小于1的,换为1,否则为0
log= np.logical_and(temp > 0.5 ,temp < 1)
log
----result----------
array([[False, False, False, False],
[False, False, False, False],
[False, False, False, False],
[False, False, False, False]])
np.where(log,1,0)
----result----------
array([[0, 0, 0, 0],
[0, 0, 0, 0],
[0, 0, 0, 0],
[0, 0, 0, 0]])
log2= np.logical_or(temp > 0.5 ,temp < -0.5)
log2
----result----------
array([[False, True, True, True],
[False, False, False, False],
[False, True, True, True],
[ True, True, True, True]])
np.where(log2,1,0)
----result----------
array([[0, 1, 1, 1],
[0, 0, 0, 0],
[0, 1, 1, 1],
[1, 1, 1, 1]])
9.统计计算
9.1.统计指标
# 统计指标
# 统计指标函数 min \ max \ mean \ median \ var \ std
# np.函数名 或者 np.方法名
temp
----result----------
array([[-0.0245709 , 1.1 , -0.95063999, -0.53223192],
[-0.23024816, -0.41551718, -0.01701004, 0.25067482],
[-0.33762242, -0.9108106 , -0.57667507, -1.71265711],
[ 1.1 , -0.76190147, 1.1 , 1.1 ]])
# 按列求得最大值 ,axis = 0
temp.max(axis = 0)
----result----------
array([1.1, 1.1, 1.1, 1.1])
# 按行求得最小值 axis = -1 或者 1
np.min(temp,axis = 1)
np.min(temp,axis = -1)
----result----------
array([-0.95063999, -0.41551718, -1.71265711, -0.76190147])
# 返回最大值得索引 -- 按行 求最大值
np.argmax(temp,axis= 1)
----result----------
array([1, 3, 0, 0], dtype=int64)
# 返回最小值得索引 -- 按列 求最小值
temp.argmin(axis = 0)
----result----------
array([2, 2, 0, 2], dtype=int64)
10.数组间的运算
10.1 数组与数的运算
# 数组可以与数直接计算
arr = np.array([[1, 2, 3, 2, 1, 4], [5, 6, 1, 2, 3, 1]])
arr = arr / 10
arr
----result----------
array([[0.1, 0.2, 0.3, 0.2, 0.1, 0.4],
[0.5, 0.6, 0.1, 0.2, 0.3, 0.1]])
# 列表不能进行直接计算
a = [1,2,3]
a * 3
----result----------
[1, 2, 3, 1, 2, 3, 1, 2, 3]
10.2 数组与数组的运算
arr1 = np.array([[1, 2, 3, 2, 1, 4], [5, 6, 1, 2, 3, 1]])
arr2 = np.array([[1, 2, 3, 4], [3, 4, 5, 6]])
arr1.shape
----result----------
(2, 6)
arr1.ndim
----result----------
2
arr2.shape
----result----------
(2, 4)
arr2.ndim
----result----------
2
# arr1 + arr2
# ---------------------------------------------------------------------------
# ValueError Traceback (most recent call last)
# Input In [182], in <cell line: 1>()
# ----> 1 arr1 + arr2
# ValueError: operands could not be broadcast together with shapes (2,6) (2,4)
# 广播机制 满足下列条件 可以进行数组间的运算
# 1.维度相等
# 2.shape(其中相对应的一个地方为1)
arr1 = np.array([[1, 2, 3, 2, 1, 4], [5, 6, 1, 2, 3, 1]])
arr2 = np.array([[1], [3]])
arr1.shape
----result----------
(2, 6)
arr2.shape
----result----------
(2, 1)
arr3 = arr1 + arr2
arr3.shape
----result----------
(2, 6)
10.3 矩阵的运算
# 矩阵matrix ---- 二维数组
# 矩阵 与 二维数组区别
# 矩阵可以直接进行运算符运算 , array 的二维数组需要调用方法
# ndarray存储矩阵
data = np.array([[80, 86],
[82, 80],
[85, 78],
[90, 90],
[86, 82],
[82, 90],
[78, 80],
[92, 94]])
data
----result----------
array([[80, 86],
[82, 80],
[85, 78],
[90, 90],
[86, 82],
[82, 90],
[78, 80],
[92, 94]])
# matrix存储矩阵
data_mat = np.mat([[80, 86],
[82, 80],
[85, 78],
[90, 90],
[86, 82],
[82, 90],
[78, 80],
[92, 94]])
data_mat
----result----------
matrix([[80, 86],
[82, 80],
[85, 78],
[90, 90],
[86, 82],
[82, 90],
[78, 80],
[92, 94]])
10.4 矩阵乘法运算
# 矩阵乘法的两个关键
# 1.形状改变
# 2.运算规则
# (M行,N列) * (N行 ,L列 ) 注:N列与N行 要对应,否则不能进行矩阵运算
weights = np.array([[0.3],[0.7]])
weights
----result----------
array([[0.3],
[0.7]])
weights_mat = np.mat([[0.3],[0.7]])
# 两种方法
# 1. np.matmul 矩阵相乘
# 2. np.dot 点乘
# 3. 拓展(了解)
np.matmul(data,weights)
----result----------
array([[84.2],
[80.6],
[80.1],
[90. ],
[83.2],
[87.6],
[79.4],
[93.4]])
np.dot(data,weights)
----result----------
array([[84.2],
[80.6],
[80.1],
[90. ],
[83.2],
[87.6],
[79.4],
[93.4]])
data_mat * weights_mat
----result----------
matrix([[84.2],
[80.6],
[80.1],
[90. ],
[83.2],
[87.6],
[79.4],
[93.4]])
11. 合并与分割
11.1 合并
11.1.1 水平拼接 hstack
a = np.array((1,2,3))
b = np.array((2,3,4))
np.hstack((a,b))
----result----------
array([1, 2, 3, 2, 3, 4])
a = np.array([[1],[2],[3]])
b = np.array([[2],[3],[4]])
np.hstack((a,b))
----result----------
array([[1, 2],
[2, 3],
[3, 4]])
11.1.2 竖直拼接 hstack
a = np.array((1,2,3))
b = np.array((2,3,4))
np.vstack((a,b))
----result----------
array([[1, 2, 3],
[2, 3, 4]])
a = np.array([[1],[2],[3]])
b = np.array([[2],[3],[4]])
np.vstack((a,b))
----result----------
array([[1],
[2],
[3],
[2],
[3],
[4]])
11.1.3 合并拼接 concatenate axis = 0 水平 1或-1 竖直
a = np.array([[1,2],[3,4]])
b = np.array([[5,6]])
np.concatenate((a,b),axis = 0)
----result----------
array([[1, 2],
[3, 4],
[5, 6]])
np.concatenate((a,b.T),axis = 1)
----result----------
array([[1, 2, 5],
[3, 4, 6]])
11.2 分割
x = np.arange(9.0)
x
----result----------
array([0., 1., 2., 3., 4., 5., 6., 7., 8.])
# 按照步长进行分割
np.split(x,3)
----result----------
[array([0., 1., 2.]), array([3., 4., 5.]), array([6., 7., 8.])]
# 按照索引进行分割
np.split(x,[3,5,6,10])
----result----------
[array([0., 1., 2.]),
array([3., 4.]),
array([5.]),
array([6., 7., 8.]),
array([], dtype=float64)]
12. IO操作与数据处理
12.1 Numpy读取
data = np.genfromtxt("./day3/day3资料/02-代码/test.csv",delimiter=",")
12.2 如何处理缺失值
# 两种处理缺失值数据方法
# 1.直接删除缺失值数据
# 2.将缺失值替换补全数据
# nan NAN 类型为 float64
# t = data[2,2]
# type(t)
def fill_nan_by_column_mean(t):
for i in range(t.shape[1]):
# 计算nan的个数
nan_num = np.count_nonzero(t[:, i][t[:, i] != t[:, i]])
if nan_num > 0:
now_col = t[:, i]
# 求和
now_col_not_nan = now_col[np.isnan(now_col) == False].sum()
# 和/个数
now_col_mean = now_col_not_nan / (t.shape[0] - nan_num)
# 赋值给now_col
now_col[np.isnan(now_col)] = now_col_mean
# 赋值给t,即更新t的当前列
t[:, i] = now_col
return t
# fill_nan_by_column_mean(data)
三、Pandas高级处理 1
-
数据处理工具
- panel + data + analysis
- panel面板数据 - 计量经济学 三维数据
-
为什么使用pandas
- 便捷的数据处理能力
- 读取文件方便
- 封装了Matplotlib、Numpy的画图和计算
-
DataFrame
- 既有行索引又有列索引
- 二维数组(固定不变的)
- 属性:
- shape
- index
- columns
- values
- T
- 方法:
- head()
- tail()
- DataFrame索引设置
- 修改行列索引值
- 重设索引
- 设置新索引
-
Panel
- DataFrame的容器
-
Series
- 带索引的一维数组
- 属性
- index
- values
-
总结
- DataFrame是Series的容器
- Panel 是DataFrame的容器
import numpy as np
# 创建一个符合正态分布的10个股票5天的涨跌幅数据
# 均值为 0 标准差为 1 生成的数据为 10行5列
stock_change = np.random.normal(0,1,(10,5))
stock_change
----result----------
array([[ 0.62392828, 1.49780091, -2.24893185, 1.42890353, 1.0371102 ],
[ 1.26229577, -0.01889369, -1.03854223, -0.84674139, -0.58421933],
[ 0.16216979, -0.99435009, 0.95895474, -0.98746556, -0.98492345],
[ 0.16194925, 0.2109128 , -0.45130401, 1.32244156, 1.0676456 ],
[ 0.26151688, 0.25919852, -0.6945317 , 1.35207356, -0.03483866],
[-0.03017989, -0.35822256, -0.83436719, 0.55108206, 0.10087387],
[-1.29781219, 0.93603652, -0.54495118, -0.60376686, -2.24740919],
[-1.05103675, -0.15612166, -0.3558128 , -0.30679821, -1.38390409],
[ 0.26297504, -0.57099557, 0.40567368, -0.87836647, 0.4373559 ],
[ 1.95955588, 1.36344196, -1.0547673 , 0.08483486, 0.72349534]])
1.DataFrame
import pandas as pd
pd.DataFrame(stock_change) # 将其转换为二维数组表形式
1.1 添加行 、列索引
# 构造一个行索引列表
stock_name = [ "股票{}".format(i) for i in range(10)]
# 构造一个列索时间列表 --- 使用pd 时间工具
date = pd.date_range(start ="20180101", periods=5,freq="B")
# index 为行索引 columns 为列索引
data = pd.DataFrame(stock_change,
index= stock_name,
columns = date)
1.2 数据属性之行索引
data.index
--------result-------------
Index(['股票0', '股票1', '股票2', '股票3', '股票4', '股票5', '股票6', '股票7', '股票8', '股票9'], dtype='object')
1.3 数据属性之列索引
data.columns
--------result-------------
DatetimeIndex(['2018-01-01', '2018-01-02', '2018-01-03', '2018-01-04',
'2018-01-05'],
dtype='datetime64[ns]', freq='B')
1.4 数据属性之数据结构类型
# shape 数据结构类型 10行5列
data.shape
--------result-------------
(10, 5)
1.5 数据属性之数据值
# values 直接获取其中array的值
data.values
--------result-------------
array([[ 0.62392828, 1.49780091, -2.24893185, 1.42890353, 1.0371102 ],
[ 1.26229577, -0.01889369, -1.03854223, -0.84674139, -0.58421933],
[ 0.16216979, -0.99435009, 0.95895474, -0.98746556, -0.98492345],
[ 0.16194925, 0.2109128 , -0.45130401, 1.32244156, 1.0676456 ],
[ 0.26151688, 0.25919852, -0.6945317 , 1.35207356, -0.03483866],
[-0.03017989, -0.35822256, -0.83436719, 0.55108206, 0.10087387],
[-1.29781219, 0.93603652, -0.54495118, -0.60376686, -2.24740919],
[-1.05103675, -0.15612166, -0.3558128 , -0.30679821, -1.38390409],
[ 0.26297504, -0.57099557, 0.40567368, -0.87836647, 0.4373559 ],
[ 1.95955588, 1.36344196, -1.0547673 , 0.08483486, 0.72349534]])
1.6 数据属性之转置
# T 转置
data.T
1.7 属性常用方法
1.7.1 head: 显示前几行
data.head(3)
1.7.2 tail:显示后几行
data.tail(3)
2.DataFrame之索引设置
2.1 修改行、列索引值 (只能整体修改)
# data.index[2] = "股票88" #不能单独修改索引
# TypeError: Index does not support mutable operations
# 修改行、列索引操作
stock_ = ["股票{}".format(i) for i in range(10,20)]
col = pd.date_range(start="20190101",periods=5,freq="B")
data.index = stock_
data.columns = col
stock_
-------------result------------------
['股票10',
'股票11',
'股票12',
'股票13',
'股票14',
'股票15',
'股票16',
'股票17',
'股票18',
'股票19']
data.index
-------------result------------------
Index(['股票10', '股票11', '股票12', '股票13', '股票14', '股票15', '股票16', '股票17', '股票18',
'股票19'],
dtype='object')
data.columns
-------------result------------------
DatetimeIndex(['2019-01-01', '2019-01-02', '2019-01-03', '2019-01-04',
'2019-01-07'],
dtype='datetime64[ns]', freq='B')
2.2 重设行索引
data.reset_index().shape
-------------result------------------
(10, 6)
data.reset_index(drop=True).shape
-------------result------------------
(10, 5)
2.3 设置新索引
df = pd.DataFrame({
'month': [1,4,7,10],
'year' : [2012,2014,2013,2014],
'sale' : [55,40,84,31]
})
df
# 以月份设置新的列索引
df.set_index("month",drop=False)
# 设置多个索引,以年和月份
new_df = df.set_index(['year','month'],drop=False)
new_df.index
-------------result------------------
MultiIndex([(2012, 1),
(2014, 4),
(2013, 7),
(2014, 10)],
names=['year', 'month'])
2.4 MultiIndex
- 多级或分成索引对象
- index属性
- names: levels的名称
- levels:每个level的元组值
new_df.index.names
-------------result------------------
FrozenList(['year', 'month'])
new_df.index.levels
-------------result------------------
FrozenList([[2012, 2013, 2014], [1, 4, 7, 10]])
3.Panel
- 存储3维数组的Panel结构
# #最新版本已经移除了panel
# p = pd.Panel(np.arange(24).reshape(4,3,2),
# items=list('ABCD'),
# major_axis=pd.date_range('20130101', periods=3),
# minor_axis=['first', 'second'])
# module 'pandas' has no attribute 'Panel'
4.Series
4.1 series属性
# series结构只有行索引
sr = data.iloc[1,:]
sr
-------------result------------------
2019-01-01 1.262296
2019-01-02 -0.018894
2019-01-03 -1.038542
2019-01-04 -0.846741
2019-01-07 -0.584219
Freq: B, Name: 股票11, dtype: float64
sr.index
-------------result------------------
DatetimeIndex(['2019-01-01', '2019-01-02', '2019-01-03', '2019-01-04',
'2019-01-07'],
dtype='datetime64[ns]', freq='B')
sr.values
-------------result------------------
array([ 1.26229577, -0.01889369, -1.03854223, -0.84674139, -0.58421933])
type(sr.values)
-------------result------------------
numpy.ndarray
4.2 指定索引
# 指定内容,默认索引
pd.Series(np.arange(3,9,2))
-------------result------------------
0 3
1 5
2 7
dtype: int32
# 指定索引
pd.Series(np.arange(3,9,2),index=["a","b","c"])
-------------result------------------
a 3
b 5
c 7
dtype: int32
4.3 通过字段数据创建
# 通过字段数据创建
pd.Series({'red':100, 'blue':200, 'green': 500, 'yellow':1000})
-------------result------------------
red 100
blue 200
green 500
yellow 1000
dtype: int64
5.基本数据操作
5.1 数据获取
data = pd.read_csv("./day3/day3资料/02-代码/stock_day/stock_day.csv")
data
5.2 删除字段
# 可以将不想看到的字段进行删除 按照列进行删除
data = data.drop(["ma5","ma10","ma20","v_ma5","v_ma10","v_ma20"], axis=1)
data
6.索引操作
6.1 直接索引
# numpy下索引
stock_change[1,1]
# pandas中DataFrame不能够直接索引
# data[1,2]
-------------result-----------
-0.01889369395385527
# 直接定位------必须先列后行
data["open"]["2018-02-26"]
-------------result-----------
22.8
6.2 名字索引(常用)
# 通过列表的形式 可以先行后列索引
data.loc["2018-02-26","open"]
-------------result-----------
22.8
6.3 数字索引(常用)
data.iloc[1,0]
-------------result-----------
22.8
6.4 组合索引
# 现在这个方法不能使用了
# data.ix[:4, ['open', 'close', 'high', 'low']]
# 'DataFrame' object has no attribute 'ix'
# 推荐使用 ------ loc + index 方式
data.loc[data.index[0:4],['open','close','high','low'] ]
# 推荐使用 ----- iloc + columns
col = data.columns.get_indexer(['open','close','high','low'])
col
-------------result-----------
array([0, 2, 1, 3], dtype=int64)
data.iloc[0:4,col]
7.赋值操作
7.1 对整列数据进行赋值
# 对整列数据进行赋值
data.open = 100
data
7.2 对某一个值进行赋值
# 对某一个值进行赋值
data.iloc[1,0] = 222
data
8.排序
8.1 内容排序
# 对单键或多健进行排序,ascending = True : 升序 False :降序
data.sort_values(by=["high"],ascending=True)
data.sort_values(by=["high","low"],ascending=False)
8.2 索引排序
data.sort_index().head()
9.算术运算
9.1 作用于一列
# 直接使用算术加法
data["open"].head()
--------result------------------
2018-02-27 100
2018-02-26 222
2018-02-23 100
2018-02-22 100
2018-02-14 100
Name: open, dtype: int64
data["open"] + 3
--------result------------------
2018-02-27 103
2018-02-26 225
2018-02-23 103
2018-02-22 103
2018-02-14 103
...
2015-03-06 103
2015-03-05 103
2015-03-04 103
2015-03-03 103
2015-03-02 103
Name: open, Length: 643, dtype: int64
# 使用函数进行加法运算
data["open"].add(3).head()
--------result------------------
2018-02-27 103
2018-02-26 225
2018-02-23 103
2018-02-22 103
2018-02-14 103
Name: open, dtype: int64
# 减法运算
data.sub(100).head()
# 查看股票的涨跌情况
data["close"].sub(data["open"]).head()
--------result------------------
2018-02-27 -75.84
2018-02-26 -198.47
2018-02-23 -77.18
2018-02-22 -77.72
2018-02-14 -78.08
dtype: float64
9.2 直接作用于整个数据集合
data / 10
10.逻辑运算 < 、> 、| 、 &
10.1 布尔索引
# 例如筛选p_change > 2 的日期数据
data[data["p_change"] > 2 ].head()
# 完成一个多个逻辑判断,筛选p_change > 2 并且 low > 15
data[(data["p_change"] > 2 ) & (data["low"] > 15)]
data[(data["p_change"] > 2 ) | (data["low"] < 15)]
11. 统计计算
11.1 describe()
# 数据描述
data.describe()
# count 数量 mean 平均值 std 标准差
# min 最小值 max 最大值
#最大值
data.max(axis=0)
--------result------------------
open 222.00
high 36.35
close 35.21
low 34.01
volume 501915.41
price_change 3.03
p_change 10.03
turnover 12.56
dtype: float64
# 最小值
data.min(axis=1)
--------result------------------
2018-02-27 0.63
2018-02-26 0.69
2018-02-23 0.54
2018-02-22 0.36
2018-02-14 0.44
...
2015-03-06 1.12
2015-03-05 0.26
2015-03-04 0.20
2015-03-03 0.18
2015-03-02 0.32
Length: 643, dtype: float64
# 标准差
data.std()
--------result------------------
open 4.811210
high 4.077578
close 3.942806
low 3.791968
volume 73879.119354
price_change 0.898476
p_change 4.079698
turnover 2.079375
dtype: float64
# 最大值所在索引
data.idxmax()
--------result------------------
open 2018-02-26
high 2015-06-10
close 2015-06-12
low 2015-06-12
volume 2017-10-26
price_change 2015-06-09
p_change 2015-08-28
turnover 2017-10-26
dtype: object
12.累计统计函数
# 函数 作用
# cumsum 1 + 2 + 3 + 4 + ... =
# cumprod 1 * 2 * 3 * 4 * ... =
# cummax 计算1/2/3/.../n 个数的最大值
# cummin 计算1/2/3/.../n 个数的最小值
data["p_change"]
--------result------------------
2018-02-27 2.68
2018-02-26 3.02
2018-02-23 2.42
2018-02-22 1.64
2018-02-14 2.05
...
2015-03-06 8.51
2015-03-05 2.02
2015-03-04 1.57
2015-03-03 1.44
2015-03-02 2.62
Name: p_change, Length: 643, dtype: float64
data["p_change"].cumsum()
--------result------------------
2018-02-27 2.68
2018-02-26 5.70
2018-02-23 8.12
2018-02-22 9.76
2018-02-14 11.81
...
2015-03-06 114.70
2015-03-05 116.72
2015-03-04 118.29
2015-03-03 119.73
2015-03-02 122.35
Name: p_change, Length: 643, dtype: float64
data["p_change"].cumprod()
--------result------------------
2018-02-27 2.680000
2018-02-26 8.093600
2018-02-23 19.586512
2018-02-22 32.121880
2018-02-14 65.849853
...
2015-03-06 -0.000000
2015-03-05 -0.000000
2015-03-04 -0.000000
2015-03-03 -0.000000
2015-03-02 -0.000000
Name: p_change, Length: 643, dtype: float64
data["p_change"].cummax()
--------result------------------
2018-02-27 2.68
2018-02-26 3.02
2018-02-23 3.02
2018-02-22 3.02
2018-02-14 3.02
...
2015-03-06 10.03
2015-03-05 10.03
2015-03-04 10.03
2015-03-03 10.03
2015-03-02 10.03
Name: p_change, Length: 643, dtype: float64
data["p_change"].cummin()
--------result------------------
2018-02-27 2.68
2018-02-26 2.68
2018-02-23 2.42
2018-02-22 1.64
2018-02-14 1.64
...
2015-03-06 -10.03
2015-03-05 -10.03
2015-03-04 -10.03
2015-03-03 -10.03
2015-03-02 -10.03
Name: p_change, Length: 643, dtype: float64
data["p_change"].sort_index().cumsum().plot()
--------result------------------[AxesSubplot:](AxesSubplot:)
13.自定义运算
# apply(func,axis=0)
# func:自定义函数
# axis = 0 默认按列运算 axis = 1 按行进行运算
# 定义一个对列 进行最大值,最小值的函数
data.apply(lambda x : x.max() - x.min() )
--------result------------------
open 122.00
high 23.68
close 22.85
low 21.81
volume 500757.29
price_change 6.55
p_change 20.06
turnover 12.52
dtype: float64
14.pandas画图
# data.plot( x = , y = , kind = )
#kind = line (默认) ,bar barth hist ,scatter ,pie
data.plot(x="p_change",y="turnover",kind="scatter")
--------result------------------
<AxesSubplot:xlabel='p_change', ylabel='turnover'>
data.plot(x="volume",y="turnover",kind="scatter")
<AxesSubplot:xlabel='volume', ylabel='turnover'>
data.plot(x="high",y="low",kind="scatter")
--------result------------------
<AxesSubplot:xlabel='high', ylabel='low'>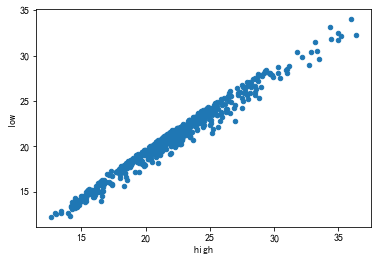
15.文件的读取与存储(重点)
15.1 CSV文件的读取和存储
# pandas.read_csv(filepath_or_buffer, sep=',', delimiter=None, header='infer',names=None, index_col=None, usecols=None)
# usecols 使用哪些字段
# names 为各个字段取名
# index_col 将某一字段设为索引
# sep 用sep参数设置分隔符
# nrows 需要获取的行数
pd.read_csv("./day3/day3资料/02-代码/stock_day/stock_day.csv",usecols = ["high","low","open","close"])
# CSV文件的读取
data = pd.read_csv("./day3/day3资料/02-代码/stock_day2.csv", names=["open", "high", "close", "low", "volume", "price_change", "p_change", "ma5", "ma10", "ma20", "v_ma5", "v_ma10", "v_ma20", "turnover"])
data
# CSV文件的存储
data[:10].to_csv("./test.csv",
columns=["open","close"],
index=False,
mode = "a",
header =False)
pd.read_csv("test.csv")
15.2 SQL的读取与存储
# import pymysql
# import pandas as pd
# from sklearn.preprocessing import LabelEncoder
# # 1.获取数据
# conn = pymysql.connect(host='localhost',
# user='root',
# password='123456',
# database='pms')
# data = pd.read_sql("select * from test_lcc limit 100 ;",con=conn)
# df = pd.DataFrame(data)
15.3 JSON文件的读取与存储
# JSON文件读取
sa = pd.read_json("./day3/day3资料/02-代码/Sarcasm_Headlines_Dataset.json",orient="records",lines = True)
sa
sa.to_json("test.json",orient = "records",lines = True)
pd.read_json("test.json",orient = "records",lines = True)
15.4 HDF5文件的读取与存储
day_close = pd.read_hdf("./day3/day3资料/02-代码/stock_data/day/day_close.h5")
day_close
day_close.to_hdf("test.h5",key="close")
pd.read_hdf("test.h5")
Pandas 高级处理2
1.如何进行缺失值处理
两种思路
- 删除含有缺失值的样本
- 替换/插补
import pandas as pd
import numpy as np
movie = pd.read_csv("./day4/day4资料/02-代码/IMDB/IMDB-Movie-Data.csv")
movie.head()
1.1 查看是否有缺失值
1.1.1 方法一 isnull + any
# any 有一个True 就返回true 所以当movie中有空值则返回 true
np.any(pd.isnull(movie)) # 返回true,说明数据中存在缺失值
--------result------------------
True
# 统计各个字段是否存在缺失值
# Revenue (Millions) True
# Metascore True
pd.isnull(movie).any() # 返回true 有空值,返回false 无空值
--------result------------------
Rank False
Title False
Genre False
Description False
Director False
Actors False
Year False
Runtime (Minutes) False
Rating False
Votes False
Revenue (Millions) True
Metascore True
dtype: bool
1.1.2 方法一 notnull + all
# all 全部是True 就返回true 否则返回false 所以当movie中有 空值则返回true
np.all(pd.notnull(movie)) # 返回false,说明数据汇总存在缺失值
--------result------------------
False
pd.notnull(movie).all() # 返回false 有空值 , 返回true 无空值
--------result------------------
Rank True
Title True
Genre True
Description True
Director True
Actors True
Year True
Runtime (Minutes) True
Rating True
Votes True
Revenue (Millions) False
Metascore False
dtype: bool
1.1.3 方法三 isnull + sum
# 统计各个字段的缺失值个数
pd.isnull(movie).sum()
--------result------------------
Rank 0
Title 0
Genre 0
Description 0
Director 0
Actors 0
Year 0
Runtime (Minutes) 0
Rating 0
Votes 0
Revenue (Millions) 128
Metascore 64
dtype: int64
movie.isnull().sum()
--------result------------------
Rank 0
Title 0
Genre 0
Description 0
Director 0
Actors 0
Year 0
Runtime (Minutes) 0
Rating 0
Votes 0
Revenue (Millions) 128
Metascore 64
dtype: int64
2.缺失值处理
2.1 方法一 : 删除含有缺失值的样本
# 方法一 : 删除含有缺失值的样本
# dropna(axis=0, how="any", thresh=None, subset=None, inplace=False)
data1 = movie.dropna()
data1.head()
# 处理过的数据不存在缺失值
data1.isnull().any()
--------result------------------
Rank False
Title False
Genre False
Description False
Director False
Actors False
Year False
Runtime (Minutes) False
Rating False
Votes False
Revenue (Millions) False
Metascore False
dtype: bool
data1.isnull().sum()
--------result------------------
Rank 0
Title 0
Genre 0
Description 0
Director 0
Actors 0
Year 0
Runtime (Minutes) 0
Rating 0
Votes 0
Revenue (Millions) 0
Metascore 0
dtype: int64
2.2 方法二 : 替换
# 方法二 替换
# fillna(value=None, method=None, axis=None, inplace=False, limit=None)
# 1.使用平均值替换 inplace = true 对原有数据集进行修改
# 使用 method{‘backfill’, ‘bfill’, ‘pad’, ‘ffill’, None}, default None
# 使用上一个有效值填充
# df.fillna(method='backfill')
# # 同 backfill
# df.fillna(method='bfill')
# # 把当前值广播到后边的缺失值
# df.fillna(method='pad')
# # 同 pad
# df.fillna(method='ffill')
movie["Revenue (Millions)"].fillna(movie["Revenue (Millions)"].mean(),
inplace=True)
movie["Metascore"].fillna(movie["Metascore"].mean()
, inplace=True)
# 查看是否有缺失值 返回true 则不存在缺失值
movie.notnull().all()
--------result------------------
Rank True
Title True
Genre True
Description True
Director True
Actors True
Year True
Runtime (Minutes) True
Rating True
Votes True
Revenue (Millions) True
Metascore True
dtype: bool
2.3 不是缺失值NaN,有默认标记的
# 读取数据
path = "https://archive.ics.uci.edu/ml/machine-learning-databases/breast-cancer-wisconsin/breast-cancer-wisconsin.data"
name = ["Sample code number", "Clump Thickness", "Uniformity of Cell Size", "Uniformity of Cell Shape", "Marginal Adhesion", "Single Epithelial Cell Size", "Bare Nuclei", "Bland Chromatin", "Normal Nucleoli", "Mitoses", "Class"]
data = pd.read_csv(path, names=name)
data
# 1.替换
# 将 ? 替换成 nan
data_new = data.replace(to_replace= "?",value= np.nan)
# 2.查看是否存在缺失值
# Bare Nuclei True
data_new.isnull().any()
--------result------------------
Sample code number False
Clump Thickness False
Uniformity of Cell Size False
Uniformity of Cell Shape False
Marginal Adhesion False
Single Epithelial Cell Size False
Bare Nuclei True
Bland Chromatin False
Normal Nucleoli False
Mitoses False
Class False
dtype: bool
# 3.删除缺失值
data_new.dropna(inplace=True)
# 4.查看是否存在缺失值
data_new.isnull().any()
--------result------------------
Sample code number False
Clump Thickness False
Uniformity of Cell Size False
Uniformity of Cell Shape False
Marginal Adhesion False
Single Epithelial Cell Size False
Bare Nuclei False
Bland Chromatin False
Normal Nucleoli False
Mitoses False
Class False
dtype: bool
# 5.补充:
# 5.1 查看数据集合中字段的数据类型
data_new.dtypes
--------result------------------
Sample code number int64
Clump Thickness int64
Uniformity of Cell Size int64
Uniformity of Cell Shape int64
Marginal Adhesion int64
Single Epithelial Cell Size int64
Bare Nuclei object
Bland Chromatin int64
Normal Nucleoli int64
Mitoses int64
Class int64
dtype: object
# 5.2 观察数据
data_new.info()
--------result------------------
<class 'pandas.core.frame.DataFrame'>
Int64Index: 683 entries, 0 to 698
Data columns (total 11 columns):
# Column Non-Null Count Dtype
--- ------ -------------- -----
0 Sample code number 683 non-null int64
1 Clump Thickness 683 non-null int64
2 Uniformity of Cell Size 683 non-null int64
3 Uniformity of Cell Shape 683 non-null int64
4 Marginal Adhesion 683 non-null int64
5 Single Epithelial Cell Size 683 non-null int64
6 Bare Nuclei 683 non-null object
7 Bland Chromatin 683 non-null int64
8 Normal Nucleoli 683 non-null int64
9 Mitoses 683 non-null int64
10 Class 683 non-null int64
dtypes: int64(10), object(1)
memory usage: 64.0+ KB
# 5.3 统计每个字段缺失值个数
data_new.isnull().sum()
--------result------------------
Sample code number 0
Clump Thickness 0
Uniformity of Cell Size 0
Uniformity of Cell Shape 0
Marginal Adhesion 0
Single Epithelial Cell Size 0
Bare Nuclei 0
Bland Chromatin 0
Normal Nucleoli 0
Mitoses 0
Class 0
dtype: int64
data_new["Bare Nuclei"] = data_new["Bare Nuclei"].astype("int64")
data_new.info()
--------result------------------
<class 'pandas.core.frame.DataFrame'>
Int64Index: 683 entries, 0 to 698
Data columns (total 11 columns):
# Column Non-Null Count Dtype
--- ------ -------------- -----
0 Sample code number 683 non-null int64
1 Clump Thickness 683 non-null int64
2 Uniformity of Cell Size 683 non-null int64
3 Uniformity of Cell Shape 683 non-null int64
4 Marginal Adhesion 683 non-null int64
5 Single Epithelial Cell Size 683 non-null int64
6 Bare Nuclei 683 non-null int64
7 Bland Chromatin 683 non-null int64
8 Normal Nucleoli 683 non-null int64
9 Mitoses 683 non-null int64
10 Class 683 non-null int64
dtypes: int64(11)
memory usage: 64.0 KB
# 5.4 pandas内置的处理函数 to_numeric to_datetime
# df['Jan Units'] = pd.to_numeric(df['Jan Units'], errors='coerce').fillna(0)
# errors参数有: raise, errors默认为raise ignore 忽略错误 coerce 将错误数据标注为NaN
#df['Start_Date'] = pd.to_datetime(df[['Month', 'Day', 'Year']])
3.数据离散化
# 为什么要离散化 --------简化数据结构
# one_hot编码 哑变量
# 如何实现数据的离散化
# 1) 分组
# 自动分组 pd.qcut( data , bins)
# 自定义分组 pd.cut( data ,[] )
# 2) 将分组好的结果转换成one_hot编码
# str 是Series 类型
# pd.get_dummies(str,prefix=)
# 1)准备数据
data = pd.Series([165,174,160,180,159,163,192,184],
index=['No1:165', 'No2:174','No3:160', 'No4:180', 'No5:159', 'No6:163', 'No7:192', 'No8:184'])
data
--------result------------------
No1:165 165
No2:174 174
No3:160 160
No4:180 180
No5:159 159
No6:163 163
No7:192 192
No8:184 184
dtype: int64
3.1 自动分组 + one-hot编码
# 2)分组
# 自动分组 --- 将数据 分为 3 组
sr = pd.qcut(data, 3)
sr
--------result------------------
No1:165 (163.667, 178.0]
No2:174 (163.667, 178.0]
No3:160 (158.999, 163.667]
No4:180 (178.0, 192.0]
No5:159 (158.999, 163.667]
No6:163 (158.999, 163.667]
No7:192 (178.0, 192.0]
No8:184 (178.0, 192.0]
dtype: category
Categories (3, interval[float64, right]): [(158.999, 163.667] < (163.667, 178.0] < (178.0, 192.0]]
#### one-hot编码
pd.get_dummies(sr,prefix="height")
3.2 自定义分组 + one-hot编码
# 自定义分组
bins = [150,165,180,195]
sr = pd.cut(data,bins)
sr
--------result------------------
No1:165 (150, 165]
No2:174 (165, 180]
No3:160 (150, 165]
No4:180 (165, 180]
No5:159 (150, 165]
No6:163 (150, 165]
No7:192 (180, 195]
No8:184 (180, 195]
dtype: category
Categories (3, interval[int64, right]): [(150, 165] < (165, 180] < (180, 195]]
# 查看分组各个区间的个数
sr.value_counts()
--------result------------------
(150, 165] 4
(165, 180] 2
(180, 195] 2
dtype: int64
# 转换成one-hot编码
pd.get_dummies(sr,prefix="身高")
4.案例:股票的涨跌幅离散化
# 我们对p_change 进行离散化
# 1.读取数据
stock = pd.read_csv("./day4/day4资料/02-代码/stock_day/stock_day.csv")
p_change = stock["p_change"]
p_change.head()
--------result------------------
2018-02-27 2.68
2018-02-26 3.02
2018-02-23 2.42
2018-02-22 1.64
2018-02-14 2.05
Name: p_change, dtype: float64
# 2.观察数据信息
p_change.info()
--------result------------------
<class 'pandas.core.series.Series'>
Index: 643 entries, 2018-02-27 to 2015-03-02
Series name: p_change
Non-Null Count Dtype
-------------- -----
643 non-null float64
dtypes: float64(1)
memory usage: 10.0+ KB
# 3.观察是否有缺失值
p_change.isnull().sum()
p_change.isnull().any()
p_change.notnull().all()
--------result------------------
True
# 4.处理缺失值 ----- 这里没有空值,所以没有处理
# ① pd.dropna() 删除缺失值
# ② pd.fillna() 填充缺失值
# 5.数据离散化
# ① 分组
sr = pd.qcut(p_change,10)
# ② 查看分组
sr.value_counts()
--------result------------------
(-10.030999999999999, -4.836] 65
(-0.462, 0.26] 65
(0.26, 0.94] 65
(5.27, 10.03] 65
(-4.836, -2.444] 64
(-2.444, -1.352] 64
(-1.352, -0.462] 64
(1.738, 2.938] 64
(2.938, 5.27] 64
(0.94, 1.738] 63
Name: p_change, dtype: int64
# ③ 离散化
pd.get_dummies(sr,prefix="涨跌幅")
# ④自定义分组
bins = [-100,-7,-5,-3,0,3,5,7,100]
sr = pd.cut(p_change,bins)
sr
--------result------------------
2018-02-27 (0, 3]
2018-02-26 (3, 5]
2018-02-23 (0, 3]
2018-02-22 (0, 3]
2018-02-14 (0, 3]
...
2015-03-06 (7, 100]
2015-03-05 (0, 3]
2015-03-04 (0, 3]
2015-03-03 (0, 3]
2015-03-02 (0, 3]
Name: p_change, Length: 643, dtype: category
Categories (8, interval[int64, right]): [(-100, -7] < (-7, -5] < (-5, -3] < (-3, 0] < (0, 3] < (3, 5] < (5, 7] < (7, 100]]
# ⑤查看分组
sr.value_counts()
--------result------------------
(0, 3] 215
(-3, 0] 188
(3, 5] 57
(-5, -3] 51
(5, 7] 35
(7, 100] 35
(-100, -7] 34
(-7, -5] 28
Name: p_change, dtype: int64
# ⑥数据离散化
stock_change = pd.get_dummies(sr,"涨跌幅")
5.数据合并
# numpy 数据合并
# 水平拼接 np.hstack()
# 竖直拼接 np.vstack()
# 拼接 np.concatnate((a,b),axis=)
5.1 按方向拼接 pd.concat()
# 处理好的one-hot编码与原数据合并
stock.head()
stock_change.head()
5.1.1 水平拼接
# 水平拼接
pd.concat([stock,stock_change],axis = 1)
5.1.2 竖直拼接
# 竖直拼接
pd.concat([stock,stock_change],axis = 0)
5.2 按索引合并 pd.merge()
# pd.merge(left,right,how="连接方式",on=[索引])
left = pd.DataFrame({'key1': ['K0', 'K0', 'K1', 'K2'],
'key2': ['K0', 'K1', 'K0', 'K1'],
'A': ['A0', 'A1', 'A2', 'A3'],
'B': ['B0', 'B1', 'B2', 'B3']})
right = pd.DataFrame({'key1': ['K0', 'K1', 'K1', 'K2'],
'key2': ['K0', 'K0', 'K0', 'K0'],
'C': ['C0', 'C1', 'C2', 'C3'],
'D': ['D0', 'D1', 'D2', 'D3']})
left
right
# 内连接 -- 左索引 等于 右索引 ,则返回
pd.merge(left,right,how="inner",on=["key1","key2"])
# 左连接 -- 以左边索引为主,符合左边索引的就返回
pd.merge(left,right,how="left",on=["key1","key2"])
# 右连接 -- 以右边索引为主,符合右边索引的就返回
pd.merge(left,right,how="right",on=["key1","key2"])
# 外连接 -- 以两边为主 即 笛卡尔积
pd.merge(left,right,how="outer",on=["key1","key2"])
6. 交叉表与透视表什么作用
6.1 交叉表
# 交叉表 pd.crosstab(): 交叉表用于计算一列数据对于另外一列数的分组个数
# 即:寻找连个列之间的关系
# 星期数据以及涨跌幅好坏的 数据
# pd.crosstab(星期数据列,涨跌幅数据列)
stock.index
--------result------------------
Index(['2018-02-27', '2018-02-26', '2018-02-23', '2018-02-22', '2018-02-14',
'2018-02-13', '2018-02-12', '2018-02-09', '2018-02-08', '2018-02-07',
...
'2015-03-13', '2015-03-12', '2015-03-11', '2015-03-10', '2015-03-09',
'2015-03-06', '2015-03-05', '2015-03-04', '2015-03-03', '2015-03-02'],
dtype='object', length=643)
# pandas 处理日期类型 API
date = pd.to_datetime(stock.index)
date.weekday
--------result------------------
Int64Index([1, 0, 4, 3, 2, 1, 0, 4, 3, 2,
...
4, 3, 2, 1, 0, 4, 3, 2, 1, 0],
dtype='int64', length=643)
# 在原始数据集中添加一列数据
stock["weekday"] = date.weekday
stock["weekday"]
--------result------------------
2018-02-27 1
2018-02-26 0
2018-02-23 4
2018-02-22 3
2018-02-14 2
..
2015-03-06 4
2015-03-05 3
2015-03-04 2
2015-03-03 1
2015-03-02 0
Name: weekday, Length: 643, dtype: int64
# 准备涨跌幅数据列 大于 0 返回 1 否则返回 0
stock["pona"] = np.where(stock["p_change"] > 0 , 1 , 0)
stock["pona"]
--------result------------------
2018-02-27 1
2018-02-26 1
2018-02-23 1
2018-02-22 1
2018-02-14 1
..
2015-03-06 1
2015-03-05 1
2015-03-04 1
2015-03-03 1
2015-03-02 1
Name: pona, Length: 643, dtype: int32
stock.head()
# 交叉表
data = pd.crosstab(stock["weekday"],stock["pona"])
data
# 按行就行求和
data.sum(axis = 1)
--------result------------------
weekday
0 125
1 131
2 132
3 128
4 127
dtype: int64
# 按列进行求和
data.sum(axis = 0)
--------result------------------
pona
0 301
1 342
dtype: int64
# pd 除法方法 第一个参数是 每行的求和 第二个参数是 每行的数据值
plot_data = data.div(data.sum(axis=1),axis=0)
plot_data
# pd.plot()绘图 bar 为柱状图 stacked = True 为图像是否堆叠
plot_data.plot(kind="bar",stacked=True)
--------result------------------[AxesSubplot:xlabel='weekday'](AxesSubplot:xlabel='weekday')
pd.crosstab(stock["weekday"],stock["pona"])
data.div(data.sum(axis=1),axis=0)
6.2 透视表
# 透视表
stock.pivot_table(["pona"],index=["weekday"])
7.分组与聚合
col =pd.DataFrame({'color': ['white','red','green','red','green'],
'object': ['pen','pencil','pencil','ashtray','pen'],
'price1':[5.56,4.20,1.30,0.56,2.75],
'price2':[4.75,4.12,1.60,0.75,3.15]},index=["a","b","c","d","e"])
col
# 进行分组,对颜色进行分组,price进行聚合
col.groupby(by="color")["price1"].max()
--------result------------------
color
green 2.75
red 4.20
white 5.56
Name: price1, dtype: float64
col["price1"].groupby(col["color"]).count()
--------result------------------
color
green 2
red 2
white 1
Name: price1, dtype: int64
8.案例:星巴克零售店铺数据案列
# 1.读取数据
data = pd.read_csv("./day4/day4资料/02-代码/directory.csv")
data.head()
# 2.按照国家进行分组,并求出数量
data.groupby(by="Country").count()
# 按照国家进行分组,取出 Brand字段数据
count_data = data.groupby(by="Country").count()["Brand"]
count_data
--------result------------------
Country
AD 1
AE 144
AR 108
AT 18
AU 22
...
TT 3
TW 394
US 13608
VN 25
ZA 3
Name: Brand, Length: 73, dtype: int64
# 按照国家进行分组,取出 Brand字段数据 并排序,最后绘制出图表
count_data.sort_values(ascending=False)[:10].plot(kind = "bar")
--------result------------------[AxesSubplot:xlabel='Country'](AxesSubplot:xlabel='Country')
9.综合案例
# 1.准备数据
movie = pd.read_csv("./day4/day4资料/02-代码/IMDB/IMDB-Movie-Data.csv")
movie.head()
# 问题1:我们想知道这些电影数据中评分的平均分,
# 导演的人数等信息,我们怎么获取?
movie["Rating"].mean()
--------result------------------
6.723200000000003
np.unique(movie["Director"]).size
--------result------------------
644
# 问题2: 对于这一组电影数据,
# 如果我们想rating,runtime的分布情况,应该如何呈现数据
movie["Rating"].plot(kind="hist",figsize=(20,8))
--------result------------------[AxesSubplot:ylabel='Frequency'](AxesSubplot:ylabel='Frequency')
import matplotlib.pyplot as plt
# 1.创建图像
plt.figure(figsize = (10,5),dpi = 100 )
# 2.绘制直方图
plt.hist(movie["Runtime (Minutes)"],20)
plt.legend()
# 修改刻度
plt.xticks(np.linspace(movie["Runtime (Minutes)"].min(),movie["Runtime (Minutes)"].max(),20))
# 添加网格
plt.grid(linestyle = "--",alpha=0.5)
# 3显示图像
plt.show()
No artists with labels found to put in legend. Note that artists whose label start with an underscore are ignored when legend() is called with no argument.
# 问题3:对于这一组电影数据,如果我们希望统计电影分类(genre)的情况,
# 应该如何处理数据?
# 先统计电影类别都有哪些
movie_genre = [ i.split(",") for i in movie["Genre"]]
movie_genre[:20]
--------result------------------
[['Action', 'Adventure', 'Sci-Fi'],
['Adventure', 'Mystery', 'Sci-Fi'],
['Horror', 'Thriller'],
['Animation', 'Comedy', 'Family'],
['Action', 'Adventure', 'Fantasy'],
['Action', 'Adventure', 'Fantasy'],
['Comedy', 'Drama', 'Music'],
['Comedy'],
['Action', 'Adventure', 'Biography'],
['Adventure', 'Drama', 'Romance'],
['Adventure', 'Family', 'Fantasy'],
['Biography', 'Drama', 'History'],
['Action', 'Adventure', 'Sci-Fi'],
['Animation', 'Adventure', 'Comedy'],
['Action', 'Comedy', 'Drama'],
['Animation', 'Adventure', 'Comedy'],
['Biography', 'Drama', 'History'],
['Action', 'Thriller'],
['Biography', 'Drama'],
['Drama', 'Mystery', 'Sci-Fi']]
# 第一个循环 for i in movie_genre
# 第二个循环 for j in i
# 去重 np.unique
list = [ j for i in movie_genre for j in i ]
movie_class = np.unique(list)
movie_class
--------result------------------
array(['Action', 'Adventure', 'Animation', 'Biography', 'Comedy', 'Crime',
'Drama', 'Family', 'Fantasy', 'History', 'Horror', 'Music',
'Musical', 'Mystery', 'Romance', 'Sci-Fi', 'Sport', 'Thriller',
'War', 'Western'], dtype='<U9')
# 统计每个类别有几个电影
count = pd.DataFrame(np.zeros(shape=(1000,20)),dtype="int32",columns=movie_class)
count
count.head()
# 计数填表
for i in range(1000) :
a = []
for j in movie_genre[i] :
for k in range(20):
if j == movie_class[k] :
a.append(k)
count.iloc[i, a] = 1
count
count.sum(axis=0).sort_values(ascending=False).plot(kind="bar",
figsize=(20, 9),
fontsize=40,
colormap="cool")
--------result------------------[AxesSubplot:](AxesSubplot:)
count1 = count
for i in range(1000) :
count1.loc[count1.index[i], movie_genre[i] ] = 1
count1
count1.sum(axis=0).sort_values(ascending=False).plot(kind="bar",
figsize=(20, 9),
fontsize=40,
color="b")
[AxesSubplot:](AxesSubplot:)