SQLAlchemy加载数据到数据库
SQLAlchemy加载数据到数据库
最近在研究基于知识图谱的问答系统,想要参考网上分享的关于NLPCC 2016 KBQA任务的经验帖,自己实现一个原型。不少博客都有提到,nlpcc-kbqa训练数据只提供了问题和答案,没有标注三元组,因此需要根据答案(尾实体)从知识图谱中反向查找头实体和关系,进而构建一条训练样例的(头实体,关系,尾实体)三元组标注。由于知识图谱规模比较大,三元组的数量超过了4000万条,直接根据文件进行查询不方便,因此考虑用数据库来管理这些三元组。本文记录了通过SQLAlchemy加载数据到MySQL数据库中的过程,主要内容包括建立数据连接、定义数据库表模式等。
连接数据库
其实加载数据(尤其是表格型数据)到数据库不一定需要自己写代码,一些工具比如SQL Server连接到平面数据源,或者Navicat的导入向导,完全能胜任这样的工作,但是观察到知识图谱存在比较多的噪声数据,想要在导入数据库前做一些预处理,所以才选用Python+SQLAlchemy的方式来实现导入数据到数据库的需求。
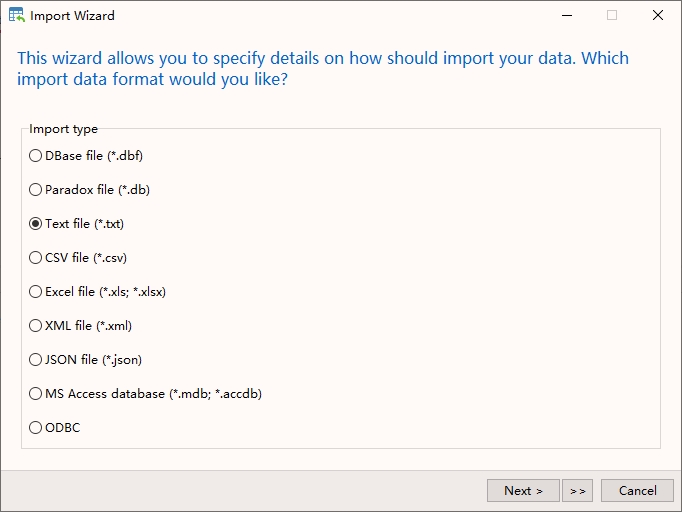
-
准备工作
# 安装SQLAlchemy和MySQL驱动 conda install sqlalchemy conda install mysql-connector
# 导入需要用到的类或函数 from sqlalchemy import create_engine from sqlalchemy.ext.declarative import declarative_base from sqlalchemy import Column, Integer, String from sqlalchemy.orm import sessionmaker
-
建立数据库连接
# 注意指定字符集为`utf8mb4` engine = create_engine('mysql+mysqlconnector://****:******@***.***.***.***:3306/kbqa?charset=utf8mb4', echo=False) Base = declarative_base() Session = sessionmaker(bind=engine) session = Session()
定义数据库表模式
SQLALchemy提供了易用的ORM接口,可以把关系型数据库的表结构映射到对象上,同样我们可以通过定义Python的类来指定数据库的表模式。考虑到后面会有根据头实体、关系名、尾实体查询数据库的需求,为了提升查询效率,这里为这三个字段都设置了索引;类属性__table_args__
可以指定编码、存储引擎等配置项。
-
定义表模式
Base = declarative_base() class KnowledgeTuple(Base): """定义知识库三元组的数据库模式""" __tablename__ = 'knowledge_tuples' __tableargs__ = { 'mysql_charset': 'utf8mb4' } id = Column(Integer, primary_key=True, comment='三元组id') entity = Column(String(250), nullable=False, index=True, comment='头实体') attribute = Column(String(250), nullable=False, index=True, comment='关系') value = Column(String(250), nullable=False, index=True, comment='尾实体')
-
数据库中的表
SHOW CREATE TABLE knowledge_tuples; CREATE TABLE `knowledge_tuples` ( `id` int(11) NOT NULL AUTO_INCREMENT COMMENT '三元组id', `entity` varchar(250) NOT NULL COMMENT '头实体', `attribute` varchar(250) NOT NULL COMMENT '关系', `value` varchar(250) NOT NULL COMMENT '尾实体', PRIMARY KEY (`id`), KEY `ix_knowledge_tuples_entity` (`entity`), KEY `ix_knowledge_tuples_attribute` (`attribute`), KEY `ix_knowledge_tuples_value` (`value`) ) ENGINE=InnoDB DEFAULT CHARSET=utf8mb
数据清洗
通过简单规则的方式统计,发现有超过10万条的三元组的关系名包含空格、特殊字符或引用(中括号夹一个数字),因此考虑在把数据加载到数据库前对数据进行清洗。
import re
BLANK_PATTERN = re.compile(r'\s')
REFERENCE_PATTERN = re.compile(r'\[[0-9]*\]')
def remove_special_characters(field: str) -> str:
"""过滤含特殊字符的前缀、后缀"""
return field.strip(' 。,、·•-:!!#$%&*+,-./:;=?@\\^_`|')
def remove_blank(field: str) -> str:
"""去掉空格"""
return BLANK_PATTERN.sub('', field)
def remove_reference(field: str) -> str:
"""去掉引用(中括号+数字)"""
return REFERENCE_PATTERN.sub('', field)
def is_illegal_relation(tpl: str) -> bool:
"""测试关系名长度是否过长以及关系名和尾实体是否相同"""
sub, relation, obj = tpl.split(' ||| ')
if len(relation) > 20 or (relation == obj):
return True
else: return False
加载数据到数据库
考虑到知识图谱的数据量比较大,只通过单个进程加载数据效率不高,这里populate_database
函数接收一个范围(start
到stop
)作为参数,这样就可以对数据集进行分片,再创建多个进程,不同进程处理数据的不同范围(或区间),多进程并行能够在一定程度上提高任务的效率。
from itertools import islice
from pathlib import Path
from tqdm import tqdm # 用于显示进度条
basedir = Path(r'D:\Datasets\nlpcc2018\nlpcc-kbqa')
kb_file = basedir / 'knowledge' / 'nlpcc-iccpol-2016.kbqa.kb'
def populate_database(start: int, stop: int, buffer_size: int):
if stop < start:
raise ValueError(f'Invalid arguments: (start={start}, stop={stop})')
num_knowledge_tpls = stop - start
session = Session()
knowlege_buffer = []
old_count, new_count = 0, 0
with tqdm(total=num_knowledge_tpls) as pbar, \
kb_file.open(encoding='utf8') as fp:
for line in islice(fp, start, stop):
entity, attribute, value = line.strip().split(' ||| ')
# 清洗数据
new_attribute = remove_special_characters(attribute)
new_attribute = remove_blank(new_attribute)
new_attribute = remove_reference(new_attribute)
new_entity = remove_reference(entity.strip())
new_value = remove_reference(value.strip())
if is_illegal_relation(line) or len(new_entity) > 250 or len(new_value) > 250:
pbar.update(1)
continue
knowledge_tpl = KnowledgeTuple(entity=new_entity, attribute=new_attribute, value=new_value)
knowlege_buffer.append(knowledge_tpl)
if len(knowlege_buffer) > buffer_size:
# 达到缓冲区容量的上限后,通过一次事务提交所有的三元组
session.add_all(knowlege_buffer)
session.commit()
knowlege_buffer = []
new_count += buffer_size
pbar.update(new_count - old_count)
old_count = new_count
session.add_all(knowlege_buffer)
session.commit()
new_count += len(knowlege_buffer)
session.close()
engine.dispose() # !important
print(f'数据库`{KnowledgeTuple.__tablename__}`: 新增{new_count}条记录')
# 提供命令行接口
import argparse
if __name__ == '__main__':
parser = argparse.ArgumentParser()
parser.add_argument("--start", required=True, type=int, help="The start argument of slice")
parser.add_argument("--stop", required=True, type=int, help="The end argument of slice")
parser.add_argument("--buffer_size", default=20000, type=int, help="Number of records in one transaction")
args = parser.parse_args()
populate_database(args.start, args.stop, args.buffer_size)
遇到的问题
-
Incorrect string value
异常KBQA数据集一方面规模大、信息全,另一方面由于是通过异构数据源(爬虫,人工标注,其他知识库)进行构建的,包含了大量的噪声。在加载数据到数据库的过程中,我遇到了
Incorrect string value: '...' for cloumn...
的异常。通过观察错误信息可以发现,三元组中包含了不常用的字符'𦈏',它的Unicode编码是U+2620F,位于中日韩统一表意文字扩充B区,占4个字节。>>> len('𦈏'.encode()) 4 >>> len('婚'.encode()) 3
UTF-8最大的一个特点,就是它是一种变长的编码方式,可以使用1~4个字节表示一个符号,根据不同的符号而变化字节长度。在MySQL中,字符集指定为UTF-8、字段类型是字符串的字段只能存储最多三个字节的字符,而存不了包含四个字节的字符。 要在MySQL中保存4字节长度的UTF-8字符,需要使用utf8mb4('mb4'指most bytes 4)字符集。
# 1. 创建数据库连接时指定编码方式为'utf8mb4' engine = create_engine('dialect+driver://username:password@host:port/database?charset=utf8mb4') # 或engine = create_engine('dialect+driver://username:password@host:port/database', encoding='utf8mb4') # 2. 定义数据库表模式时,通过类属性`__table_args__`指定编码方式 class KnowledgeTuple(Base): __tableargs__ = { 'mysql_charset': 'utf8mb4' }
-
Text类型的字段不能设置索引
我遇到的第二个问题是不能为Text类型的字段创建索引
-
SQLAlchemy关闭数据库连接
尽管我在使用完数据库连接后,都显式地调用
session.close()
关闭了会话,但是只是这样实际上并没有断开数据库连接,通过执行SHOW STATUS LIKE '%Connection%';
能看到还有大量的数据库连接存在。That is, the Engine is a factory for connections as well as a pool of connections, not the connection itself. When you say conn.close(), the connection is returned to the connection pool within the Engine, not actually closed.
# 通过以下方式断开数据库连接 session.close() engine.dispose() # !important