import scvelo as scv
adata = scv.read("E:/data_exercise/day1_27/scV/data/Pancreas/adata_dynamics.h5ad", cache=True)
adata
AnnData object with n_obs × n_vars = 3696 × 2000
obs: 'clusters_coarse', 'clusters', 'S_score', 'G2M_score', 'initial_size_spliced', 'initial_size_unspliced', 'initial_size', 'n_counts'
var: 'highly_variable_genes', 'gene_count_corr', 'means', 'dispersions', 'dispersions_norm', 'highly_variable', 'fit_r2', 'fit_alpha', 'fit_beta', 'fit_gamma', 'fit_t_', 'fit_scaling', 'fit_std_u', 'fit_std_s', 'fit_likelihood', 'fit_u0', 'fit_s0', 'fit_pval_steady', 'fit_steady_u', 'fit_steady_s', 'fit_variance', 'fit_alignment_scaling'
uns: 'clusters_coarse_colors', 'clusters_colors', 'day_colors', 'neighbors', 'pca', 'recover_dynamics'
obsm: 'X_pca', 'X_umap'
varm: 'loss'
layers: 'Ms', 'Mu', 'fit_t', 'fit_tau', 'fit_tau_', 'spliced', 'unspliced'
obsp: 'connectivities', 'distances'
# 计算速度矢量
scv.tl.velocity(adata, mode='dynamical')
scv.tl.velocity_graph(adata)
computing velocities
finished (0:00:06) --> added
'velocity', velocity vectors for each individual cell (adata.layers)
computing velocity graph
finished (0:00:11) --> added
'velocity_graph', sparse matrix with cosine correlations (adata.uns)
#汇出嵌入模型
scv.pl.velocity_embedding_grid(adata, basis='umap')
computing velocity embedding
finished (0:00:01) --> added
'velocity_umap', embedded velocity vectors (adata.obsm)
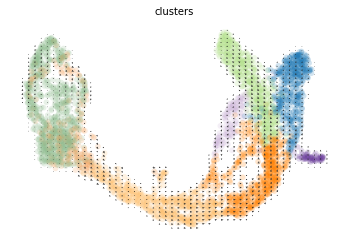
#汇出嵌入模型
scv.pl.velocity_embedding_stream(adata, basis='umap')
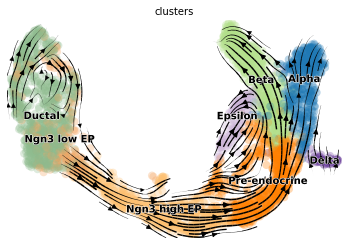
#计算cell cycle score并可视化
scv.tl.score_genes_cell_cycle(adata)
scv.pl.scatter(adata, color_gradients=['S_score', 'G2M_score'], basis="umap", smooth=True, perc=[5, 95])
calculating cell cycle phase
--> 'S_score' and 'G2M_score', scores of cell cycle phases (adata.obs)
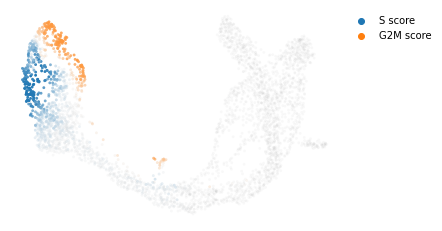
#3.5 计算细胞状态并可视化
scv.tl.terminal_states(adata)
scv.pl.scatter(adata, color=[ 'root_cells', 'end_points'], basis='umap')
#'root_cells', root cells of Markov diffusion process (adata.obs)
#'end_points', end points of Markov diffusion process (adata.obs)
computing terminal states
WARNING: Uncertain or fuzzy root cell identification. Please verify.
identified 2 regions of root cells and 1 region of end points .
finished (0:00:00) --> added
'root_cells', root cells of Markov diffusion process (adata.obs)
'end_points', end points of Markov diffusion process (adata.obs)
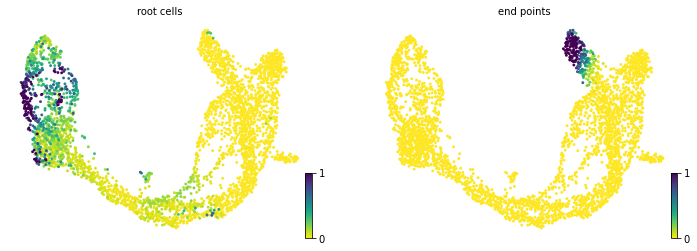
adata
AnnData object with n_obs × n_vars = 3696 × 2000
obs: 'clusters_coarse', 'clusters', 'S_score', 'G2M_score', 'initial_size_spliced', 'initial_size_unspliced', 'initial_size', 'n_counts', 'velocity_self_transition', 'phase', 'clusters_gradients', 'root_cells', 'end_points'
var: 'highly_variable_genes', 'gene_count_corr', 'means', 'dispersions', 'dispersions_norm', 'highly_variable', 'fit_r2', 'fit_alpha', 'fit_beta', 'fit_gamma', 'fit_t_', 'fit_scaling', 'fit_std_u', 'fit_std_s', 'fit_likelihood', 'fit_u0', 'fit_s0', 'fit_pval_steady', 'fit_steady_u', 'fit_steady_s', 'fit_variance', 'fit_alignment_scaling', 'velocity_genes'
uns: 'clusters_coarse_colors', 'clusters_colors', 'day_colors', 'neighbors', 'pca', 'recover_dynamics', 'velocity_params', 'velocity_graph', 'velocity_graph_neg', 'clusters_gradients_colors'
obsm: 'X_pca', 'X_umap', 'velocity_umap'
varm: 'loss'
layers: 'Ms', 'Mu', 'fit_t', 'fit_tau', 'fit_tau_', 'spliced', 'unspliced', 'velocity', 'velocity_u'
obsp: 'connectivities', 'distances'
#计算pseudotime,可视化,与导出
scv.tl.velocity_pseudotime(adata)
scv.pl.scatter(adata=adata, color='velocity_pseudotime', cmap='gnuplot', basis="umap")
adata.obs["velocity_pseudotime"].to_csv('velocyto_pseudotime.csv')
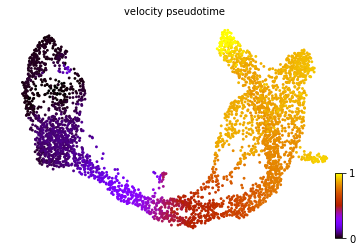
#查看特定基因的velocity
var_names = ['Snhg6', 'Sbspon', 'Eif2s3y', 'Sntg1'] #adata.var 下的index就是基因名
scv.pl.velocity(adata, var_names=var_names, colorbar=True, ncols=2)
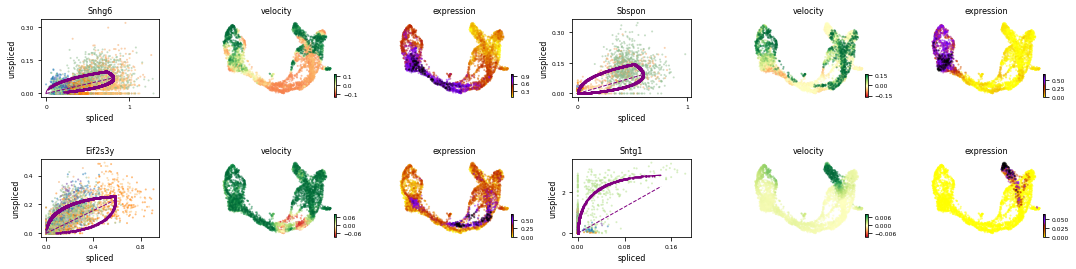
adata
AnnData object with n_obs × n_vars = 3696 × 2000
obs: 'clusters_coarse', 'clusters', 'S_score', 'G2M_score', 'initial_size_spliced', 'initial_size_unspliced', 'initial_size', 'n_counts', 'velocity_self_transition', 'phase', 'clusters_gradients', 'root_cells', 'end_points', 'velocity_pseudotime'
var: 'highly_variable_genes', 'gene_count_corr', 'means', 'dispersions', 'dispersions_norm', 'highly_variable', 'fit_r2', 'fit_alpha', 'fit_beta', 'fit_gamma', 'fit_t_', 'fit_scaling', 'fit_std_u', 'fit_std_s', 'fit_likelihood', 'fit_u0', 'fit_s0', 'fit_pval_steady', 'fit_steady_u', 'fit_steady_s', 'fit_variance', 'fit_alignment_scaling', 'velocity_genes'
uns: 'clusters_coarse_colors', 'clusters_colors', 'day_colors', 'neighbors', 'pca', 'recover_dynamics', 'velocity_params', 'velocity_graph', 'velocity_graph_neg', 'clusters_gradients_colors'
obsm: 'X_pca', 'X_umap', 'velocity_umap'
varm: 'loss'
layers: 'Ms', 'Mu', 'fit_t', 'fit_tau', 'fit_tau_', 'spliced', 'unspliced', 'velocity', 'velocity_u'
obsp: 'connectivities', 'distances'
df = adata.var
df
|
highly_variable_genes |
gene_count_corr |
means |
dispersions |
dispersions_norm |
highly_variable |
fit_r2 |
fit_alpha |
fit_beta |
fit_gamma |
... |
fit_std_s |
fit_likelihood |
fit_u0 |
fit_s0 |
fit_pval_steady |
fit_steady_u |
fit_steady_s |
fit_variance |
fit_alignment_scaling |
velocity_genes |
index |
|
|
|
|
|
|
|
|
|
|
|
|
|
|
|
|
|
|
|
|
|
Sntg1 |
True |
NaN |
0.005065 |
0.131135 |
0.214469 |
True |
0.401981 |
0.015726 |
0.005592 |
0.088792 |
... |
0.030838 |
0.406523 |
0.0 |
0.0 |
0.159472 |
2.470675 |
0.094304 |
0.149138 |
5.355590 |
False |
Snhg6 |
False |
NaN |
0.375835 |
0.158585 |
0.040765 |
True |
0.125072 |
0.389126 |
2.981982 |
0.260322 |
... |
0.245248 |
0.243441 |
0.0 |
0.0 |
0.403409 |
0.106128 |
0.596630 |
0.762252 |
2.037296 |
True |
Ncoa2 |
False |
NaN |
0.106945 |
0.145879 |
0.298358 |
True |
-2.313923 |
NaN |
NaN |
NaN |
... |
NaN |
NaN |
NaN |
NaN |
NaN |
NaN |
NaN |
NaN |
NaN |
False |
Sbspon |
True |
NaN |
0.143032 |
0.277016 |
1.044508 |
True |
0.624803 |
0.464865 |
2.437113 |
0.379645 |
... |
0.178859 |
0.252135 |
0.0 |
0.0 |
0.182088 |
0.164805 |
0.430623 |
0.674312 |
1.193015 |
True |
Ube2w |
False |
NaN |
0.018522 |
0.109459 |
0.091136 |
True |
-5.324582 |
NaN |
NaN |
NaN |
... |
NaN |
NaN |
NaN |
NaN |
NaN |
NaN |
NaN |
NaN |
NaN |
False |
... |
... |
... |
... |
... |
... |
... |
... |
... |
... |
... |
... |
... |
... |
... |
... |
... |
... |
... |
... |
... |
... |
Tmem27 |
True |
NaN |
1.297619 |
2.475960 |
3.254982 |
True |
0.527855 |
3.954723 |
21.314334 |
0.253763 |
... |
5.014219 |
0.292956 |
0.0 |
0.0 |
0.496411 |
0.168456 |
12.425889 |
0.389388 |
3.163113 |
False |
Uty |
False |
NaN |
0.018673 |
0.182256 |
0.505338 |
True |
-0.555835 |
NaN |
NaN |
NaN |
... |
NaN |
NaN |
NaN |
NaN |
NaN |
NaN |
NaN |
NaN |
NaN |
False |
Ddx3y |
True |
NaN |
0.165256 |
0.350978 |
1.465338 |
True |
-2.538731 |
NaN |
NaN |
NaN |
... |
NaN |
NaN |
NaN |
NaN |
NaN |
NaN |
NaN |
NaN |
NaN |
False |
Eif2s3y |
True |
NaN |
0.182643 |
0.318686 |
1.281603 |
True |
0.352190 |
0.193458 |
0.746410 |
0.295085 |
... |
0.158051 |
0.262140 |
0.0 |
0.0 |
0.390710 |
0.219716 |
0.424033 |
0.748614 |
2.312621 |
True |
Erdr1 |
True |
NaN |
0.344720 |
0.215833 |
0.288351 |
True |
-0.193263 |
NaN |
NaN |
NaN |
... |
NaN |
NaN |
NaN |
NaN |
NaN |
NaN |
NaN |
NaN |
NaN |
False |
2000 rows × 23 columns
df.to_csv("adata.var.csv",index=True, header=True )
# 数据框筛选那些基因
df = df[(df['fit_likelihood'] > .1) & df['velocity_genes'] == True]
kwargs = dict(xscale='log', fontsize=16)
df
|
highly_variable_genes |
gene_count_corr |
means |
dispersions |
dispersions_norm |
highly_variable |
fit_r2 |
fit_alpha |
fit_beta |
fit_gamma |
... |
fit_std_s |
fit_likelihood |
fit_u0 |
fit_s0 |
fit_pval_steady |
fit_steady_u |
fit_steady_s |
fit_variance |
fit_alignment_scaling |
velocity_genes |
index |
|
|
|
|
|
|
|
|
|
|
|
|
|
|
|
|
|
|
|
|
|
Snhg6 |
False |
NaN |
0.375835 |
0.158585 |
0.040765 |
True |
0.125072 |
0.389126 |
2.981982 |
0.260322 |
... |
0.245248 |
0.243441 |
0.0 |
0.0 |
0.403409 |
0.106128 |
0.596630 |
0.762252 |
2.037296 |
True |
Sbspon |
True |
NaN |
0.143032 |
0.277016 |
1.044508 |
True |
0.624803 |
0.464865 |
2.437113 |
0.379645 |
... |
0.178859 |
0.252135 |
0.0 |
0.0 |
0.182088 |
0.164805 |
0.430623 |
0.674312 |
1.193015 |
True |
Fam135a |
True |
NaN |
0.257955 |
0.171546 |
0.096820 |
True |
0.384662 |
0.172335 |
0.118088 |
0.204538 |
... |
0.149868 |
0.283343 |
0.0 |
0.0 |
0.387921 |
1.345830 |
0.393197 |
0.671096 |
3.390194 |
True |
Tbc1d8 |
True |
NaN |
0.070612 |
0.123167 |
0.169130 |
True |
0.613194 |
0.083656 |
0.118139 |
0.227401 |
... |
0.081595 |
0.246594 |
0.0 |
0.0 |
0.392402 |
0.659434 |
0.240100 |
0.829478 |
2.914215 |
True |
Fhl2 |
True |
NaN |
0.232482 |
0.818012 |
2.892669 |
True |
0.325223 |
0.224027 |
0.330934 |
0.121456 |
... |
0.317963 |
0.262849 |
0.0 |
0.0 |
0.476793 |
0.539878 |
1.073687 |
0.695997 |
4.785407 |
True |
... |
... |
... |
... |
... |
... |
... |
... |
... |
... |
... |
... |
... |
... |
... |
... |
... |
... |
... |
... |
... |
... |
Map3k15 |
True |
NaN |
0.180941 |
0.427601 |
1.901315 |
True |
0.437069 |
0.252467 |
0.348783 |
0.275921 |
... |
0.225956 |
0.289070 |
0.0 |
0.0 |
0.434454 |
0.611380 |
0.560048 |
0.510572 |
2.208756 |
True |
Rai2 |
True |
NaN |
0.231965 |
0.651755 |
2.173640 |
True |
0.769033 |
0.792172 |
2.885604 |
0.647345 |
... |
0.276047 |
0.271263 |
0.0 |
0.0 |
0.311969 |
0.243741 |
0.716996 |
0.707595 |
1.063908 |
True |
Rbbp7 |
False |
NaN |
1.017536 |
0.422314 |
0.153713 |
True |
0.344213 |
1.446806 |
5.332362 |
0.326539 |
... |
0.796754 |
0.281498 |
0.0 |
0.0 |
0.477365 |
0.208398 |
2.985787 |
0.704522 |
2.100311 |
True |
Ap1s2 |
True |
NaN |
0.127856 |
0.319473 |
1.286081 |
True |
0.716659 |
0.202643 |
0.596662 |
0.392769 |
... |
0.140455 |
0.286893 |
0.0 |
0.0 |
0.140955 |
0.315635 |
0.366964 |
0.671211 |
1.775751 |
True |
Eif2s3y |
True |
NaN |
0.182643 |
0.318686 |
1.281603 |
True |
0.352190 |
0.193458 |
0.746410 |
0.295085 |
... |
0.158051 |
0.262140 |
0.0 |
0.0 |
0.390710 |
0.219716 |
0.424033 |
0.748614 |
2.312621 |
True |
1006 rows × 23 columns
#RNA转录,剪接和降解的速率。
with scv.GridSpec(ncols=3) as pl:
pl.hist(df['fit_alpha'], xlabel='transcription rate', **kwargs)
pl.hist(df['fit_beta'] * df['fit_scaling'], xlabel='splicing rate', xticks=[.1, .4, 1], **kwargs)
pl.hist(df['fit_gamma'], xlabel='degradation rate', xticks=[.1, .4, 1], **kwargs)
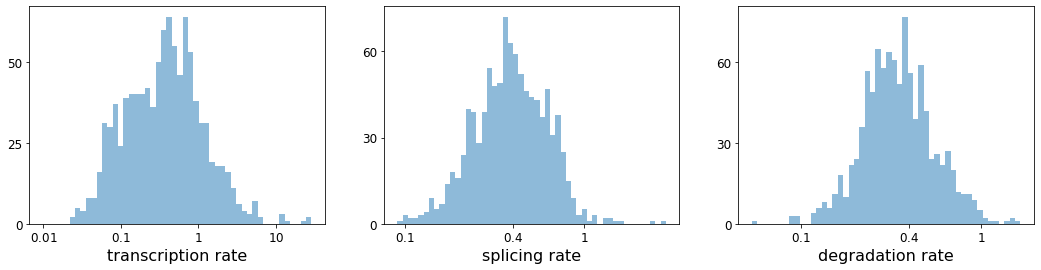
adata
AnnData object with n_obs × n_vars = 3696 × 2000
obs: 'clusters_coarse', 'clusters', 'S_score', 'G2M_score', 'initial_size_spliced', 'initial_size_unspliced', 'initial_size', 'n_counts', 'velocity_self_transition', 'phase', 'clusters_gradients', 'root_cells', 'end_points', 'velocity_pseudotime'
var: 'highly_variable_genes', 'gene_count_corr', 'means', 'dispersions', 'dispersions_norm', 'highly_variable', 'fit_r2', 'fit_alpha', 'fit_beta', 'fit_gamma', 'fit_t_', 'fit_scaling', 'fit_std_u', 'fit_std_s', 'fit_likelihood', 'fit_u0', 'fit_s0', 'fit_pval_steady', 'fit_steady_u', 'fit_steady_s', 'fit_variance', 'fit_alignment_scaling', 'velocity_genes'
uns: 'clusters_coarse_colors', 'clusters_colors', 'day_colors', 'neighbors', 'pca', 'recover_dynamics', 'velocity_params', 'velocity_graph', 'velocity_graph_neg', 'clusters_gradients_colors'
obsm: 'X_pca', 'X_umap', 'velocity_umap'
varm: 'loss'
layers: 'Ms', 'Mu', 'fit_t', 'fit_tau', 'fit_tau_', 'spliced', 'unspliced', 'velocity', 'velocity_u'
obsp: 'connectivities', 'distances'
#latent_time
scv.tl.latent_time(adata)
computing latent time using root_cells as prior
finished (0:00:02) --> added
'latent_time', shared time (adata.obs)
scv.pl.scatter(adata, color='latent_time', color_map='gnuplot', size=80)
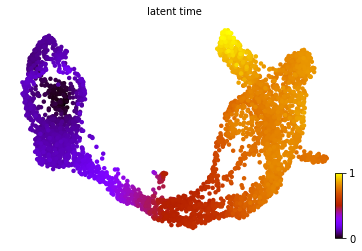
top_genes = adata.var['fit_likelihood'].sort_values(ascending=False).index[:300]
top_genes
Index(['Pcsk2', 'Dcdc2a', 'Ank', 'Gng12', 'Top2a', 'Pak3', 'Tmem163', 'Nfib',
'Pim2', 'Smoc1',
...
'Cyr61', 'Ptprn2', 'Rpa2', 'Nit2', 'Cdc20', 'Hmmr', 'Ankrd12', 'Itpr1',
'Auts2', 'Mdfi'],
dtype='object', name='index', length=300)
scv.pl.heatmap(adata, var_names=top_genes, sortby='latent_time', col_color='clusters', n_convolve=100)
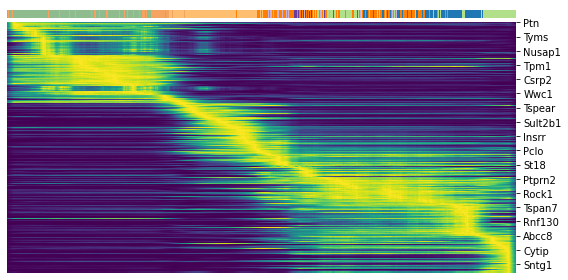
top = df['fit_likelihood'].sort_values(ascending=False).index[:300]
top
Index(['Pcsk2', 'Dcdc2a', 'Ank', 'Gng12', 'Top2a', 'Pak3', 'Tmem163', 'Nfib',
'Pim2', 'Smoc1',
...
'Trp53inp2', 'Ccnb1', 'Atp2a2', 'Dtl', 'Kmt2a', 'Emb', 'Rcan2',
'Gm28172', 'Rab3b', 'Gk'],
dtype='object', name='index', length=300)
scv.pl.heatmap(adata, var_names=top, sortby='latent_time', col_color='clusters', n_convolve=100)
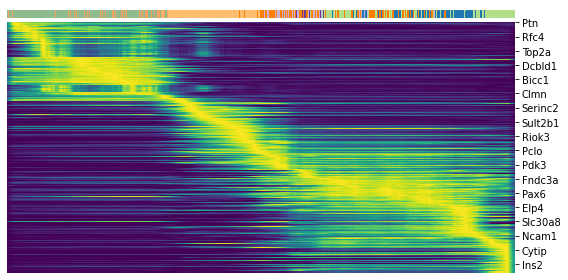
# 驱动基因
top_genes = adata.var['fit_likelihood'].sort_values(ascending=False).index
scv.pl.scatter(adata, basis=top_genes[:15], ncols=5, frameon=False)
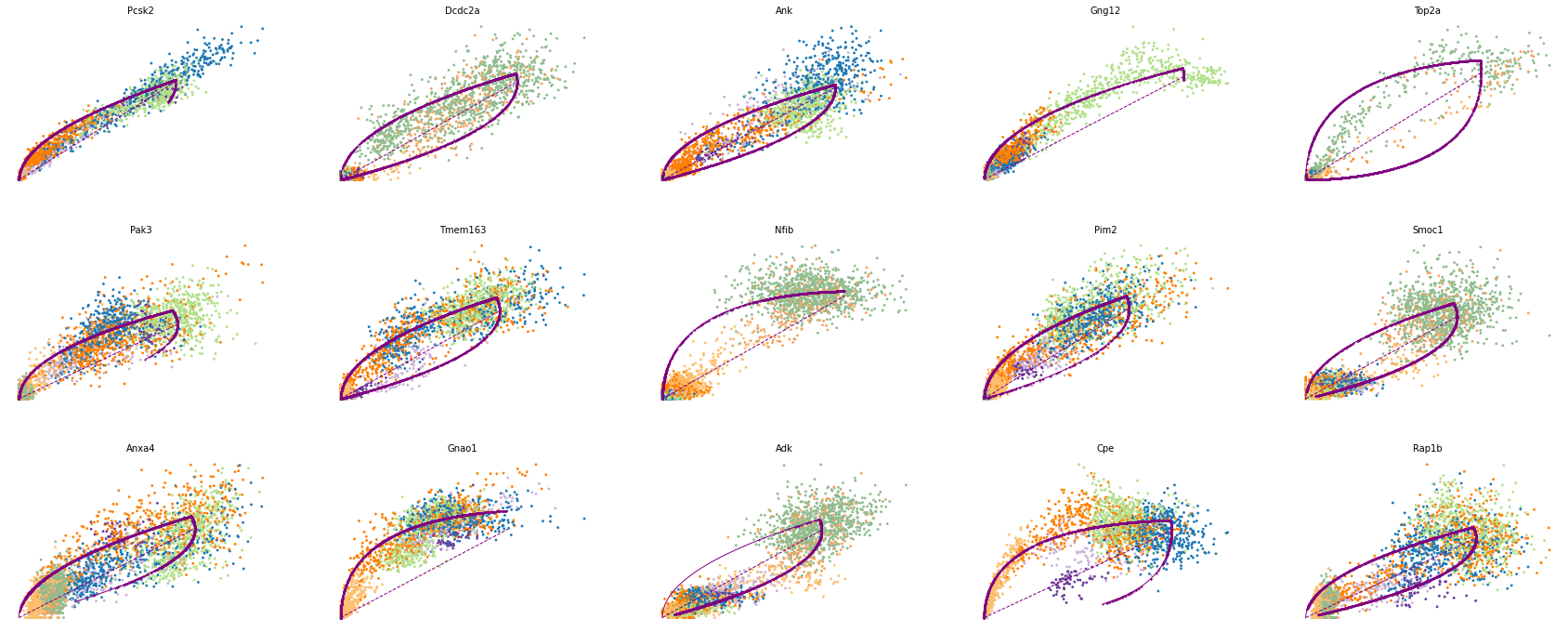
var_names = ['Actn4', 'Ppp3ca', 'Cpe', 'Nnat']
scv.pl.scatter(adata, var_names, frameon=False)
scv.pl.scatter(adata, x='latent_time', y=var_names, frameon=False)


#特定于簇的最高似然基因
scv.tl.rank_dynamical_genes(adata, groupby='clusters')
df = scv.get_df(adata, 'rank_dynamical_genes/names')
df.head(5)
ranking genes by cluster-specific likelihoods
finished (0:00:05) --> added
'rank_dynamical_genes', sorted scores by group ids (adata.uns)
|
Ductal |
Ngn3 low EP |
Ngn3 high EP |
Pre-endocrine |
Beta |
Alpha |
Delta |
Epsilon |
0 |
Dcdc2a |
Dcdc2a |
Rbfox3 |
Abcc8 |
Pcsk2 |
Cpe |
Pcsk2 |
Tox3 |
1 |
Top2a |
Adk |
Mapre3 |
Tmem163 |
Ank |
Gnao1 |
Rap1b |
Rnf130 |
2 |
Nfib |
Mki67 |
Btbd17 |
Gnao1 |
Tmem163 |
Pak3 |
Pak3 |
Meis2 |
3 |
Wfdc15b |
Rap1gap2 |
Sulf2 |
Ank |
Tspan7 |
Pim2 |
Abcc8 |
Adk |
4 |
Cdk1 |
Top2a |
Tcp11 |
Tspan7 |
Map1b |
Map1b |
Slc7a14 |
Rap1gap2 |
for cluster in ['Ductal', 'Ngn3 high EP', 'Pre-endocrine', 'Beta']:
scv.pl.scatter(adata, df[cluster][:5], ylabel=cluster, frameon=False)




df = scv.get_df(adata, 'rank_dynamical_genes/scores')
df.head(5)
|
Ductal |
Ngn3 low EP |
Ngn3 high EP |
Pre-endocrine |
Beta |
Alpha |
Delta |
Epsilon |
0 |
0.59 |
0.59 |
0.53 |
0.73 |
0.90 |
0.61 |
0.96 |
0.61 |
1 |
0.56 |
0.53 |
0.50 |
0.54 |
0.70 |
0.57 |
0.70 |
0.61 |
2 |
0.56 |
0.51 |
0.47 |
0.53 |
0.59 |
0.54 |
0.67 |
0.59 |
3 |
0.50 |
0.50 |
0.46 |
0.53 |
0.59 |
0.54 |
0.62 |
0.57 |
4 |
0.49 |
0.49 |
0.45 |
0.51 |
0.57 |
0.51 |
0.60 |
0.55 |
[参考链接1](https://scvelo.readthedocs.io/DynamicalModeling.html)
[参考链接2](https://www.bilibili.com/read/cv7764833/)