Abstract
紧耦合lidar inertial里程计, 用smoothing和mapping.
1. Introduction
紧耦合lidar-inertial里程计.
一般都是用ICP或者是GICP.
在LOAM[1], IMU被引入来de-skew lidar scan, 然后给移动一个先验做scan-匹配.
在[15], 预积分IMU测量被用来 de-skew 点云.
一个robocentric lidar-inertial 状态估计器, R-LINS[16] , 用error-state KF.
LIOM只能 0.6 倍实时
3. LiDAR Inertial Odometry via SAM
A. System Overview
状态是:
\[\mathbf{x}=\left[\mathbf{R}^{\mathbf{T}}, \mathbf{p}^{\mathbf{T}}, \mathbf{v}^{\mathbf{T}}, \mathbf{b}^{\mathbf{T}}\right]^{\mathbf{T}}
\]
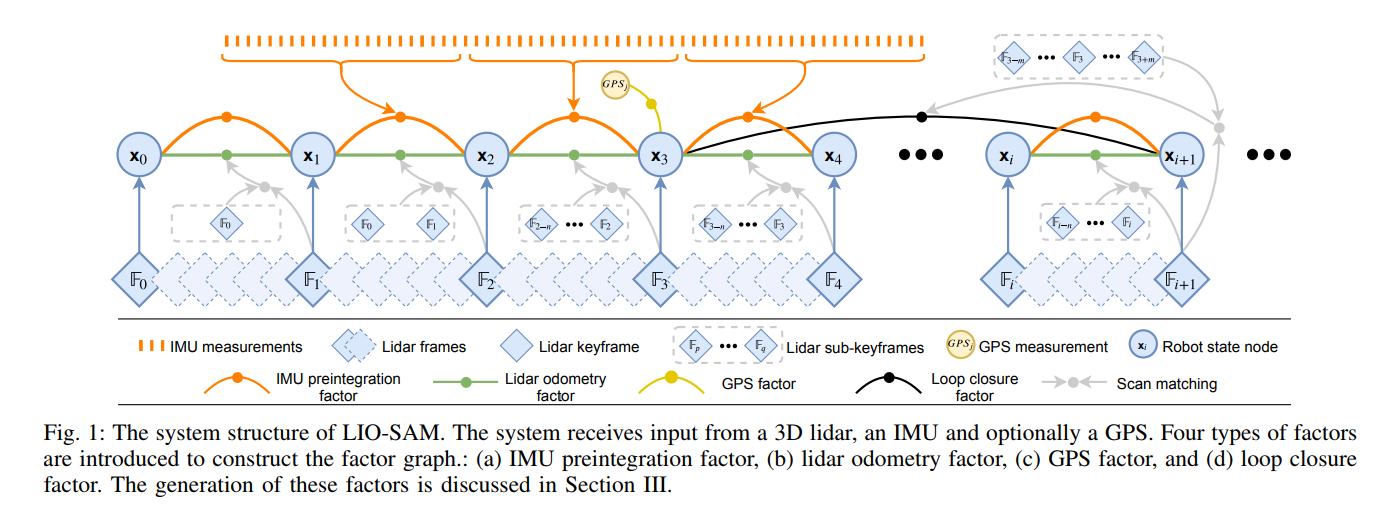
B. IMU Preintegration Factor
角速度, 加速度的测量:
\[\begin{array}{l}
\hat{\boldsymbol{\omega}}_{t}=\boldsymbol{\omega}_{t}+\mathbf{b}_{t}^{\boldsymbol{\omega}}+\mathbf{n}_{t}^{\boldsymbol{\omega}} \\
\hat{\mathbf{a}}_{t}=\mathbf{R}_{t}^{\mathbf{B W}}\left(\mathbf{a}_{t}-\mathbf{g}\right)+\mathbf{b}_{t}^{\mathbf{a}}+\mathbf{n}_{t}^{\mathbf{a}},
\end{array}
\]
这里 \(\hat{\omega}_t\) 和 \(\hat{a}_t\) 是 raw 测量在 \(B\) 系.
速度, 位置和旋转在 \(t+\Delta t\)时刻如下:
\[\begin{aligned}
\mathbf{v}_{t+\Delta t}=\mathbf{v}_{t}+\mathbf{g} \Delta t+\mathbf{R}_{t}\left(\hat{\mathbf{a}}_{t}-\mathbf{b}_{t}^{\mathbf{a}}-\mathbf{n}_{t}^{\mathbf{a}}\right) \Delta t \\
\mathbf{p}_{t+\Delta t}=\mathbf{p}_{t}+\mathbf{v}_{t} \Delta t+\frac{1}{2} \mathbf{g} \Delta t^{2} \\
&+\frac{1}{2} \mathbf{R}_{t}\left(\hat{\mathbf{a}}_{t}-\mathbf{b}_{t}^{\mathbf{a}}-\mathbf{n}_{t}^{\mathbf{a}}\right) \Delta t^{2} \\
\mathbf{R}_{t+\Delta t}=\mathbf{R}_{t} \exp \left(\left(\hat{\boldsymbol{\omega}}_{t}-\mathbf{b}_{t}^{\omega}-\mathbf{n}_{t}^{\omega}\right) \Delta t\right)
\end{aligned}
\]
这里 \(R_t = R_t^{WB} = R_t^{{BW}^T}\). 这里我们假设 角速度 和 加速度 的\(B\) 保持不变.
C. LiDAR Odometry Factor
当一个新的scan到达时, 我们先做特征提取. Edge / planar 特征被提取来估计局部点的roughness. 有大的 roughness值的实被分类为edge, 值小的就是planar特征.
1. Sub-keyframes for voxel map
2. Scan-matching
3. Relative transform
edge点和平面点对应如下:
\[\begin{array}{r}
\mathbf{d}_{e_{k}}=\frac{\left|\left(\mathbf{p}_{i+1, k}^{e}-\mathbf{p}_{i, u}^{e}\right) \times\left(\mathbf{p}_{i+1, k}^{e}-\mathbf{p}_{i, v}^{e}\right)\right|}{\left|\mathbf{p}_{i, u}^{e}-\mathbf{p}_{i, v}^{e}\right|} \\
\mathbf{d}_{p_{k}}=\frac{\left(\mathbf{p}_{i, u}^{p}-\mathbf{p}_{i, v}^{p}\right) \times\left(\mathbf{p}_{i, u}^{p}-\mathbf{p}_{i, w}^{p}\right) \mid}{\left|\left(\mathbf{p}_{i, u}^{p}-\mathbf{p}_{i, v}^{p}\right) \times\left(\mathbf{p}_{i, u}^{p}-\mathbf{p}_{i, w}^{p}\right)\right|}
\end{array}
\]
D. GPS Factor
当收到GPS测量的时候, 我会先转换到局部笛卡尔坐标系.
一般我们只有在估计的位置协方差大于接受的GPS位置协方差的时候才加入 GPS factor.
E. Loop Closure Factor
...
4. Experiments
我们比较了LIO-SAM, LOAM和LIOM. LIO-SAM和LOAM是专注在实时的输出, 而LIOM是有无限的时间处理的.
A. Rotation Dataset
遇到的最大的旋转速度是 133.7°/s.
B. Walking Dataset
LIOM只跑了0.56x的实时.
C. Campus Dataset
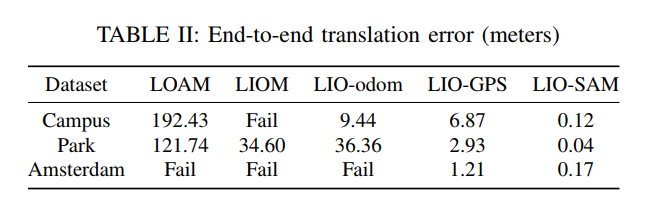
D. Park Dataset
...
E. Amsterdam Dataset
....
F. Benchmarking Results
...
5. Conclusions and Discussion
没啥.