第一章 规划问题
第一章 规划问题
1.1 线性规划模型
1.1.1 基础numpy操作
import numpy as np
a = np.array([[1,2,3],[4,5,6]])
b = np.array([[1,2],[3,4],[5,6]])
c = np.array([[1,2,3]])
d = np.array([[9,8,7],[3,2,1]])
# 矩阵加法
sum = a + d
print(sum)
输出:[[10 10 10]
[ 7 7 7]]
# 放缩
e = 3*a
print(e)
输出:[[ 3 6 9]
[12 15 18]]
# 数乘、矩阵乘
e = np.dot(a,b)
print(e)
输出:[[22 28]
[49 64]]
# 元素乘
e = a*d
print(e)
输出:[[ 9 16 21]
[12 10 6]]
# 转置
e = c.T
print(e)
输出:
[[1]
[2]
[3]]
e = np.array([[1,2],[3,4]])
# 逆矩阵
result = np.linalg.inv(e)
print(result)
输出:
[[-2. 1. ]
[ 1.5 -0.5]]
# 行列式
result = np.linalg.det(e)
print(result)
输出:-2.0000000000000004
# 矩阵的秩
e = np.linalg.matrix_rank(d)
print(e)
输出;2
1.1.2 例题1(标准形式)
以上为基础的矩阵操作功能,接下来我们队一道题目进行解答.
例题:
\(\left\{\begin{aligned} 10 x-y-2 z &=72 \\-x+10 y-2 z &=83 \\-x-y+5 z &=42 \end{aligned}\right.\)
import numpy as np
A = np.array([[10,-1,-2],[-1,10,-2],[-1,-1,5]]) # A为系数矩阵
b = np.array([72,83,42]) # b为常数列
inv_A = np.linalg.inv(A) #A的逆矩阵
x = np.dot(A,b) # A的逆矩阵与b做点积运算
x = np.linalg.solve(A,b) # 5,6两行也可用本行替代
print(x)
[11. 12. 13.]
使用 sympy 进行解答
from sympy import symbols,Eq,solve
x,y,z = symbols('x,y,z')
eqs = [Eq(10*x - y - 2*z, 72),
Eq(-x + 10*y - 2*z, 83),
Eq(-x - y + 5*z, 42)]
print(solve(eqs,[x,y,z]))
{x: 11, y: 12, z: 13}
sympy 主要用于符号解,numpy 和 scipy 主要用于数值解
1.1.3 例题2(不等式形式)
\(\max \quad z=2 x_{1}+3 x_{2}-5 x_{3}\)
s.t. \(\left\{\begin{array}{l}x_{1}+x_{2}+x_{3}=7 \\ 2 x_{1}-5 x_{2}+x_{3}>=10 \\ x_{1}+3 x_{2}+x_{3}<=12 \\ x_{1}, x_{2}, x_{3}>=0\end{array}\right.\)
from scipy import optimize
import numpy as np
c = np.array([-2,-3,5])
A = np.array([[-2,5,-1],[1,3,1]])
b = np.array([-10,12])
Aeq = np.array([[1,1,1]])
beq = np.array([7])
x1 = (0,None)
x2 = (0,None)
x3 = (0,None) # 指的是x1,x2,x3的范围为 0 到无穷大
res = optimize.linprog(-c,A,b,Aeq,beq,bounds=(x1,x2,x3))
print(res)
print(res.x)
con: array([1.52631917e-07])
fun: -14.000000657683213
message: 'Optimization terminated successfully.'
nit: 5
slack: array([-4.30601379e-07, 5.00000013e+00])
status: 0
success: True
x: array([2.99999979e+00, 1.04988686e-08, 4.00000005e+00])
[2.99999979e+00 1.04988686e-08 4.00000005e+00]
fun 为最小值, slack 为精度, x 为对应的变量值,可以通过 res.xxx 来显示对应的内容。
1.2 非线性规划
例题
【列 3-1】三台火电机组的运行成本 (单位: \(t / h\) ) 与出力限㓡 (单位:MW) 分别如下:
\[\left\{\begin{array}{l}
F_{G 1}=4+0.3 P_{G 1}+0.0007 P_{G 1}^{2}, 100 \leqslant P_{G 1} \leqslant 200 \\
F_{G 2}=3+0.32 P_{G 2}+0.0004 P_{G 2}^{2}, 120 \leqslant P_{G 2} \leqslant \leqslant 250 \\
F_{G 3}=3.5+0.3 P_{G 3}+0.00045 P_{G 3}^{2}, 150 \leqslant P_{G 3} \leqslant 300
\end{array}\right.
\]
当负荷为 \(700 \mathrm{MW}\) 时, 求经济调度的结果。
下面我们分别用scipy和遗传算法求解。
解法1(scipy)
可以选择对应的插值方式
from scipy.optimize import minimize
import numpy as np
# 目标函数即min(FG1+FG2+FG3)
def fun(x):
return (4+0.3*x[0]+0.0007*x[0]*x[0]+3+0.32*x[1]+0.0004*x[1]*x[1]+3.5+0.3*x[2]+0.00045*x[2]*x[2])
def con():
# 约束条件分别为eq和ineq
# eq表示函数结果等于0;ineq表示表达式大于或等于0
cons = ({'type':'eq','fun':lambda x:x[0]+x[1]+x[2]-700})
# {'type':'ineq','fun':lambda x: -x[2] + x - max} 如果有不等式约束
# cons = ([con1,con2,con3,con4,con5,con6,con7,con8]) 如果与多个约束,最后返回结果是这个
# x[0] 其中的0必须是具体数字,不能是t等参数
return cons
# 上下限约束
b1 = (100,200)
b2 = (120,250)
b3 = (150,300)
bnds = (b1,b2,b3) # 边界约束
if __name__ == "__main__":
cons = con() # 约束
# 设置x初始猜测值
x0 = np.array((150,250,20))
res = minimize(fun,x0,method = "SLSQP",constraints=cons,bounds=bnds) # method可选
print("结果",res.fun)
print(res.success)
print("解",res.x)
print(res)
输出:
结果 305.96739130656937
True
解 [176.08556664 250. 273.91443336]
fun: 305.96739130656937
jac: array([0.54652023, 0.52000046, 0.54652405])
message: 'Optimization terminated successfully'
nfev: 32
nit: 8
njev: 8
status: 0
success: True
x: array([176.08556664, 250. , 273.91443336])
解法2(遗传算法)
通常是一个近似解
from sko.GA import GA
def fun(x):
return(4+0.3*x[0]+0.0007*x[0]*x[0]+3+0.32*x[1]+0.0004*x[1]*x[1]+3.5+0.3*x[2]+0.00045*x[2]*x[2])
def cons1(x):
return [x[0]+x[1]+x[2]-700]
cons = cons1
ga = GA(func=fun,n_dim = 3,size_pop=200,max_iter=800,lb=[100,120,150],ub=[200,250,300],constraint_eq=[cons])
best_x,best_y=ga.run()
print("best_x:",best_x,"\n","best_y:",best_y)
输出:
best_x: [195.39379114 238.51964394 266.08656494]
best_y: [306.61308165]
1.3 整数规划模型
例题
假设有四件事四个人去做,各变量对应的数据假设如表所示
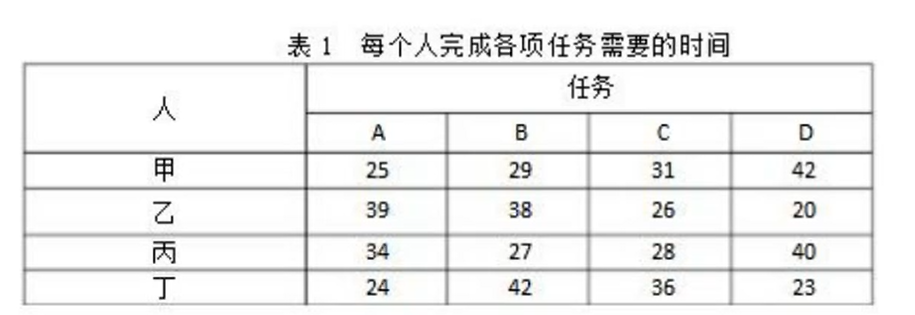
from scipy.optimize import linear_sum_assignment
import numpy as np
cost = np.array([[25,29,31,42],[39,38,26,20],[34,27,28,40],[24,42,36,23]])
row_ind,col_ind = linear_sum_assignment(cost) # 默认求最小,如需求最大可在后添加 True
print(row_ind) # 开销矩阵对应的行索引
print(col_ind) # 对应行索引的郋指派的列索引
print(cost[row_ind,col_ind]) # 提取每个行索引的最有指派列索引的元素,形成数组
print(cost[row_ind,col_ind].sum()) # 数组求和
输出:
[0 1 2 3]
[0 2 1 3]
[25 26 27 23]
101
1.4 动态规划模型
例题
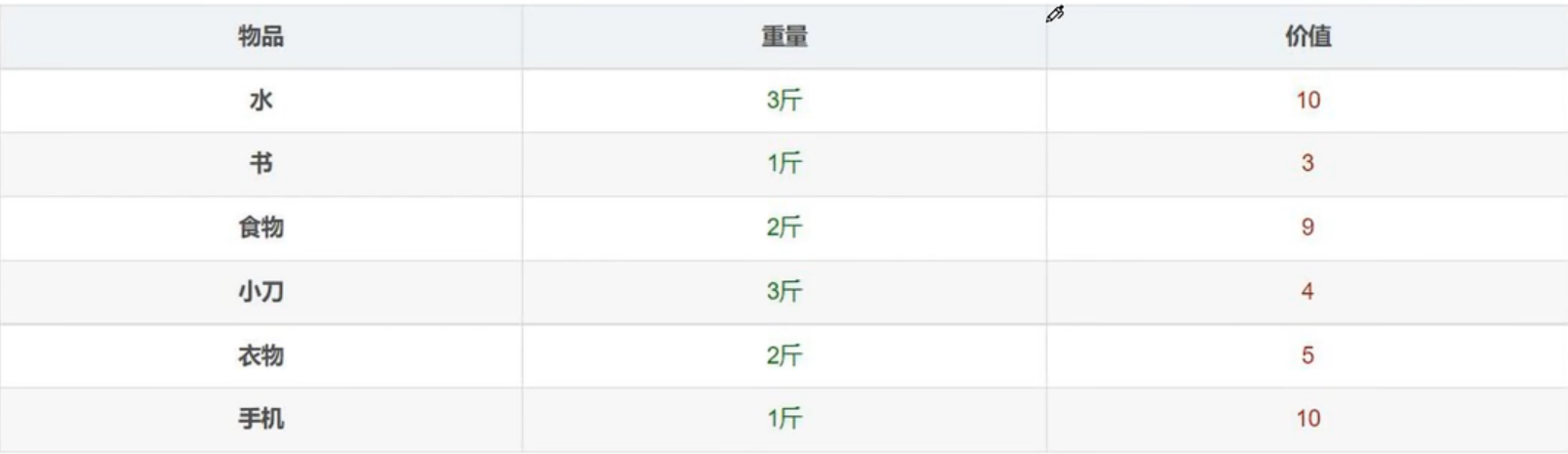
解答:
def dynamic_p() -> list:
items = [
{"name":"水","weight": 3,"value":10},
{"name": "书", "weight": 1, "value": 3},
{"name": "食物", "weight": 2, "value": 9},
{"name": "小刀", "weight": 3, "value": 4},
{"name": "衣物", "weight": 2, "value": 5},
{"name": "手机", "weight": 1, "value": 10}
]
max_capacity = 6
dp = [[0] * (max_capacity + 1) for _ in range(len(items) + 1)]
for row in range(1,len(items) + 1 ):
for col in range(1,max_capacity + 1):
weight = items[row - 1]["weight"]
value = items[row - 1]["value"]
if weight >col:
dp[row][col] = dp[row - 1][col]
else:
dp[row][col] = max(value + dp[row-1][col - weight],dp[row - 1][col])
return dp
dp = dynamic_p()
for i in dp:
print(i)
print(dp[-1][-1])
输出:[0, 0, 0, 0, 0, 0, 0]
[0, 0, 0, 10, 10, 10, 10]
[0, 3, 3, 10, 13, 13, 13]
[0, 3, 9, 12, 13, 19, 22]
[0, 3, 9, 12, 13, 19, 22]
[0, 3, 9, 12, 14, 19, 22]
[0, 10, 13, 19, 22, 24, 29]
P.S. 详细的动态规划内容可以参照博客内leetcode中的动态规划专题