特征筛选-WOE和IV
背景
在评分卡建模流程中,WOE(Weight of Evidence)常用于特征变换,IV(Information Value)则用来衡量特征的预测能力。
文章取自:风控模型—WOE与IV指标的深入理解应用
代码取自:特征值筛选依据:IV值和WOE的python计算
WOE和IV的应用价值
WOE(Weight of Evidence)叫做证据权重,大家可以思考下为什么会取这个名字?
那么WOE在业务中常有哪些应用呢?
处理缺失值:当数据源没有100%覆盖时,那就会存在缺失值,此时可以把null单独作为一个分箱。这点在分数据源建模时非常有用,可以有效将覆盖率哪怕只有20%的数据源利用起来。
处理异常值:当数据中存在离群点时,可以把其通过分箱离散化处理,从而提高变量的鲁棒性(抗干扰能力)。例如,age若出现200这种异常值,可分入“age > 60”这个分箱里,排除影响。
业务解释性:我们习惯于线性判断变量的作用,当x越来越大,y就越来越大。但实际x与y之间经常存在着非线性关系,此时可经过WOE变换。
IV(Information Value)是与WOE密切相关的一个指标,常用来评估变量的预测能力。因而可用来快速筛选变量。在应用实践中,其评价标准如下:
在此引用一段话来说明两者的区别和联系:
- WOE describes the relationship between a predictive variable and a binary target variable.
- IV measures the strength of that relationship.
WOE和IV的计算步骤
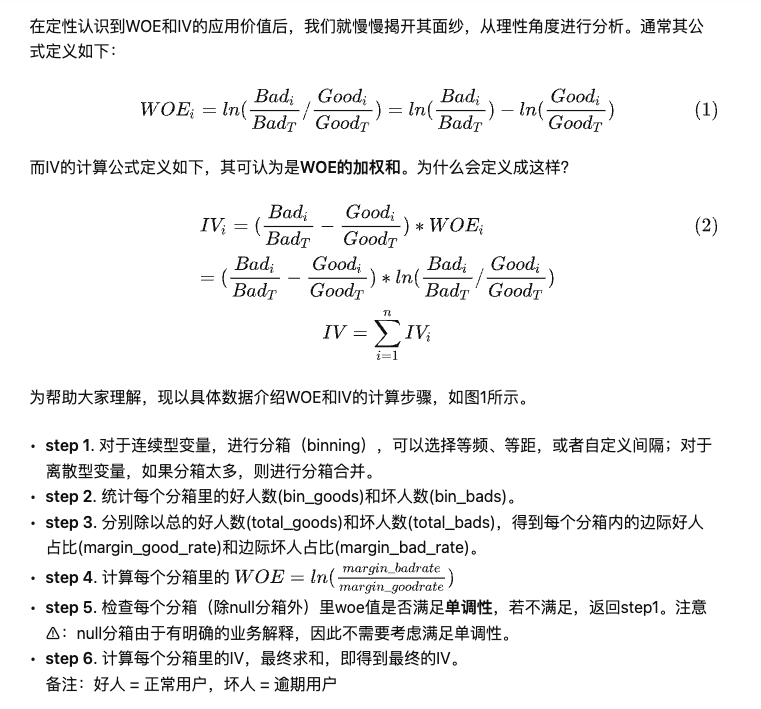

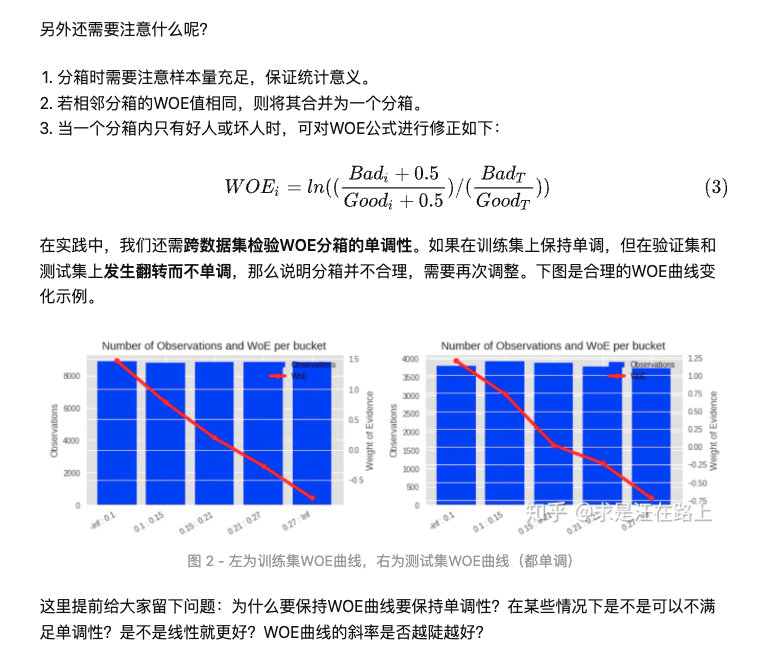
单个特征的IV值计算
import pandas as pd
import numpy as np
# 生成数据集
data = {'age':[6,7,8,11,12,13,23,24,25,37,38,39,44,45,46,51,61,71],
'label':[0,1,0,0,0,1,0,1,1,0,1,1,0,0,1,0,0,1]}
df = pd.DataFrame(data)
# 拆分好坏样本标签
df['good'] = df['label'].map(lambda x: 1 if x == 0 else 0) # 好样本
df['bad'] = df['label'].map(lambda x: 1 if x == 1 else 0) # 坏样本
# 年龄分箱
bins = [0, 10, 20, 30, 40, 50, 100]
df['age_cate'] = pd.cut(df.age, bins, labels=["婴儿","少年","青年","中年","壮年","老年"])
# 统计各箱中好坏比率
woe_iv_df_good = df.groupby('age_cate').agg({'good':'sum'}).reset_index() # 好样本个数
woe_iv_df_bad = df.groupby('age_cate').agg({'bad':'sum'}).reset_index() # 坏样本个数
woe_iv_df = pd.merge(woe_iv_df_good, woe_iv_df_bad, how = 'left', on = 'age_cate')
woe_iv_df['cnt'] = woe_iv_df['good'] + woe_iv_df['bad']
# 计算各分箱的woe值
woe_iv_df['good'] = woe_iv_df['good']/woe_iv_df['good'].sum()
woe_iv_df['bad'] = woe_iv_df['bad']/woe_iv_df['bad'].sum()
woe_iv_df['woe'] = np.log(woe_iv_df['good']/woe_iv_df['bad'])
woe_iv_df['iv'] = (woe_iv_df['good'] - woe_iv_df['bad']) * woe_iv_df['woe']
woe_iv_df
# 计算iv值
iv = woe_iv_df['iv'].sum()
iv
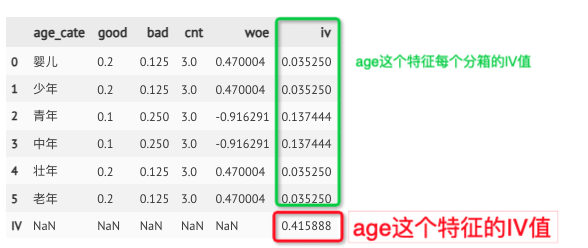
多个特征的IV值计算
data = {'age':[6,7,8,11,12,13,23,24,25,37,38,39,44,45,46,51,61,71],
'gender':['男','女','男','女','男','女','女','女','男','男','女','男','女','男','女','女','女','男'],
'label':[0,1,0,0,0,1,0,1,1,0,1,1,0,0,1,0,0,1]}
df = pd.DataFrame(data)
# 分箱
bins = [0, 10, 20, 30, 40, 50, 100]
df['age_cate'] = pd.cut(df.age, bins, labels=["婴儿","少年","青年","中年","壮年","老年"])
# 正负标签处理
df['label_0'] = df['label'].map(lambda x: 1 if x == 0 else 0)
df['label_1'] = df['label'].map(lambda x: 1 if x == 1 else 0)
# 选择需要计算的指标
dim = ['age_cate','gender']
# 创建空的表格
iv_list = pd.DataFrame([],columns = ['指标','IV值'])
# 写循环计算IV值
for index in dim:
woe_iv_df = df.groupby(index).agg({'label_0':'sum'
,'label_1':'sum'
},).reset_index()
woe_iv_df['ratio_0'] = woe_iv_df['label_0']/woe_iv_df['label_0'].sum()
woe_iv_df['ratio_1'] = woe_iv_df['label_1']/woe_iv_df['label_1'].sum()
woe_iv_df['woe'] = np.log(woe_iv_df['ratio_0']/woe_iv_df['ratio_1'])
woe_iv_df['iv'] = (woe_iv_df['ratio_0'] - woe_iv_df['ratio_1']) * woe_iv_df['woe']
iv = round(woe_iv_df['iv'].sum(),4)
a = pd.DataFrame({'指标':[index],'IV值':[iv]})
iv_list = iv_list.append(a)
# 输出结果
iv_list
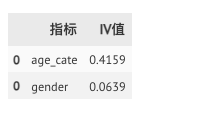