第九期 写一个自己的AutoGpt
一:先了解AutoGpt https://zhuanlan.zhihu.com/p/629909493?utm_id=0
二:实现自己的AutoGpt之一:prmpt模板处理
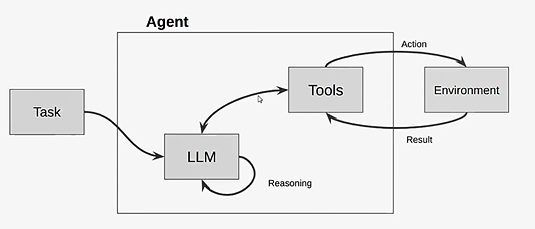
思路
-
prompt与代码分离
-
编写reason(理由) & Act (行为)的prompt模板
-
基于langchain编写AutoGpt框架
-
设计长时和短时Memory
-
封装自己的tools
-
运行AutoGpt
(一)prompt模板编写:与代码分离 --- ./prompts
因为prompt经常需要微调,将prompt抽象成模板,并且可以嵌套,再通过类串联模板
为了方便阅读,下面尽可能写成中文,实践最好还是英文🤔
1.主prompt:main.templ
0.为智能体给一个名字,定义一个角色(可选)
你的名字是{ai_name},你是{ai_role}
1.智能体主要工作描述(reason&act要做/遵守的事情),根据设计/调试过程中发现的不足,可能会经常微调这部分描述,所以进行抽象.其中嵌套的以templ结尾
You must follow the instruction below to complete the ask.
{instructions_templ}
2.描述用户的问题(任务)
你的任务是:
{task_description}
3.限制约束条件(Auto的关键),遵守/避免/不允许
Constraints:
{constraints_templ}
4.告诉可以使用哪些工具/指令(核心),为了对应think and action,为了防止歧义,又加上了“它们又被称为actions”;此外,在每次任务通过添加标识表示完成(后续思考这个是标识工具调用完成还是整体完成?应该是工具调用完成)
你可以使用一下工具或指令,它们又被称为actions:
0. FINISH:任务完成, args:None --- 这里FINISH是一个指令,表示任务完成,后面是工具的描述,FINISH指令占序号0,其他的tool从1往后
{tools}
5.告诉智能体可以操作哪些资源(系统资源),网络搜索/文件操作/...
Resources:
{resources_templ}
6.告诉智能体在每次think时,考虑当前决策的质量,对决策的质量进行描述(宏观角度考虑,不只是针对当前步骤)
Performance Evaluation:
{performance_evalution_templ}
7.描述历史记录,包括长时记忆/短时记忆;这里会实际的执行过程,动态加载长时记忆(跨任务的长时记忆),有对应的机制实现长时记忆(对每一次任务历史进行summary存储,存储到长时记忆,比如向量数据库,用于后面任务类似时查询使用)
相关的历史记录:
{long_term_memory}
8.短时记忆,执行任务过程中(包括思考、推理、执行....等分为多步骤),在执行完一个任务前,会记录每一个步骤(所谓短时记忆,会存储当前任务的历史,存储方式可以是全量存储每一个/部分步骤)
当前任务的执行记录:
{short_term_memory}
9.输出格式的约定(注意:这是每一轮的输出格式约定的指令,thought and action的格式),会根据outputParser定义,先占位模板,后面设计outputParser时会进行填充;补充一个langchain技巧,告诉智能体返回的数据可以被json.load解析,大多数情况可以,针对处理不了的代码中可以修复
You should only respond in JSON format as desctibed below.
Response Format:
{format_instruction}
Ensure the response can be parsed by Python json.loads
10.每一轮中,在前面的变量填充完成后,最后的执行指令。如果需要继续执行,则告诉他思考下一步的动作,选取工具执行;如果是最后一步,则告诉他任务已经完成了,总结前面的内容给一个具体答案。所以可以根据运行状态进行改变
{step_instruction}
补充:这里的长/短时记忆的区别
短时记忆是指记录任务中每一轮的reason和act,设置最大窗口,超过丢弃
长时记忆是跨任务的,将每个任务的记忆存储在可以长期存储的(例如:向量数据库),后面的任务可以根据相似行找到对应的长时记忆,作为参考。
如果长时记忆不进行summary,而是和短时记忆一样全量存储每一个步骤,判断相似后,相当于把相同的两个记忆段落放在prompt,两者等价,浪费token,并且可能误导大模型(因为太相似,格式一样,又很长,所以长时记忆会大于当前任务的短时记忆误导整个任务偏向过去的任务;summary会短很多,并且格式不一样,不会误导)
2.主要工作描述(reason&act要做/遵守的事情):instructions_templ
1.每次你的决策只能使用一种工具,你可以使用任意多次。 --- 一轮任务多个步骤,每个步骤是一次决策
2.确保你调用的指令或使用的工具在下述给定的工具列表中。
3.确保你的回答不会包含违法或有侵犯性的信息。
4.If you have completed all your task, make sure to use "FINISH" command.
5.用中文思考和输出 --- 单纯方便阅读
3.约束条件:constraints_templ (Auto的关键)
1.如果执行某个指令或者工具失败,尝试改变参数或参数格式再次调用。
2.如果通过互联网无法搜索到需要的信息,尝试更换关键字或在关键字中添加空格。
3.如果搜索得到的结果不正确,尝试更换搜索关键字或表达方式。
4.已经告诉你的信息,不要再通过工具或互联网去查询。 --- 防止重复查询,比如在记忆力/背景信息里面存在的不要再去互联网搜索了
5.不要向用户提问。 --- 这个就是Auto关键,希望Gpt独立完成,不与用户交互。我们可以删除,带交互的情况(需要代码逻辑)
4.资源定义:resources_templ(系统资源,不同于tool)
1.Internet access for searches and information gathering. --- 接入互联网进行搜索和信息收集
2.File input and output. --- 文件操作;如果有其他资源,可以接着写
5.思考过程中要考虑质量问题,需要注意的东西:performance_evalution_templ(宏观角度)
1. Continuously review and analyze your actions to ensure you are performing to the best of your abilities. --- 尽你的全力去审查和分析你的actions
2. Constructively self-criticize your big-picture behavior constantly. --- 反思宏观行为对不对(全局角度思考)
3. Reflect on past decisions and strategies to refine your approach. --- 反思过去的决定和策略,以改进你的方法。
4. If you get repeated feedback on a certain action, refine your thoughts or plans to resolve the issue. --- 如果在某个action上陷入死循环,请完善你的想法或计划来解决这个问题
5. Consider location, date, time and other factors (such as weather, transportations) when making decisions. --- 考虑一下时间、地点、这些因素
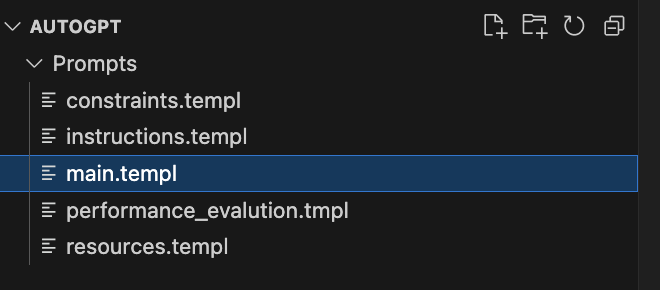
(二)将prompt模板文件解析成langchain需要的promptTemplate --- ./Utils
主要思路:加载文件、填充数据,获取完整的promptTemplate
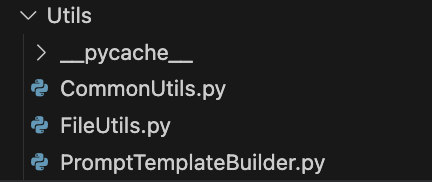
PromptTemplateBuilder.py
from typing import List, Optional
from langchain.prompts import PromptTemplate
from langchain_core.output_parsers.base import BaseOutputParser
from langchain_core.tools import BaseTool
from Utils.FileUtils import load_file
from Utils.CommonUtils import *
import json
class PromptTemplateBuilder:
def __init__(self,
prompt_path: str,
prompt_file: str = "main.templ",
):
self.prompt_path = prompt_path
self.prompt_file = prompt_file
def build(
self,
tools: Optional[List[BaseTool]] = None,
output_parser: Optional[BaseOutputParser] = None,
) -> PromptTemplate:
main_templ_str = load_file(self.prompt_path, self.prompt_file)
main_templ = PromptTemplate.from_template(main_templ_str)
#使用了langchain的from_template方法,可以直接从字符串中构建PromptTemplate对象,字符串里面的占位会被解析为变量,然后通过partial_variables参数指定变量名和对应的值
"""
print(main_templ)
input_variables=['ai_name', 'ai_role', 'constraints_templ', 'format_instruction', 'instructions_templ', 'long_term_memory', 'performance_evalution_tmpl', 'resources_templ', 'short_term_memory', 'step_instruction', 'task_desctription', 'tools']
template='你的名字是{ai_name},你是{ai_role}\n\nYou must follow the instruction below to complete the ask.\n{instructions_templ}\n\n你的任务是:\n{task_desctription}\n\nConstraints:\n{constraints_templ}\n\n你可以使用一下工具或指令,它们又被称为actions:\n0. FINISH:任务完成, args:None\n{tools}\n\nResources:\n{resources_templ}\n\nPerformance Evaluation:\n{performance_evalution_tmpl}\n\n相关的历史记录:\n{long_term_memory}\n\n当前任务的执行记录:\n{short_term_memory}\n\nYou should only respond in JSON format as desctibed below.\nResponse Format:\n{format_instruction}\n\nEnsure the response can be parsed by Python json.loads\n\n{step_instruction}'
"""
partial_variables = {}
for var in main_templ.input_variables:
if var.endswith("_templ"):
var_file = var[:-6] + ".templ"
var_str = self._get_prompt(var_file)
partial_variables[var] = var_str
if tools is not None:
tools_prompt = self._get_tools_prompt(tools)
partial_variables["tools"] = tools_prompt
if output_parser is not None:
# 为了避免ascii码转入我们的prompt导致问题,我们调用该函数进行转换
partial_variables["format_instruction"] = ChinsesFriendly(output_parser.get_format_instructions())
return main_templ.partial(**partial_variables) #返回填充了templ文件、tools、output_parser的prompt,其他变量用户单独传递
# 加载模板,返回给partial_variables使用
def _get_prompt(self, prompt_file): #没有破环
builder = PromptTemplateBuilder(self.prompt_path,prompt_file=prompt_file)
return builder.build().format()
# 获取工具提示:根据工具集里面每个工具的提示生成对应的prompt
def _get_tools_prompt(self, tools):
tools_prompt = ""
for i,tool in enumerate(tools):
tools_prompt += f"{i+1}. {tool.name} : {tool.description},\
args json schema: {json.dumps(tool.args,ensure_ascii=False)}\n"
return tools_prompt
FileUtils.py
import os
def load_file(file_path,file_name):
with open(os.path.join(file_path, file_name), 'r',encoding='utf-8') as file:
return file.read()
CommonUtils.py
import json
# https://q0tozme54s7.feishu.cn/wiki/YkGpwAlo2iD0h3kZ0TgczWJNnqb#FyXGdCg72oy2I3xDPqjc2WE5nNe
def ChinsesFriendly(string):
"""
langchain的outputparser返回的描述是压缩后的,并且中文被转成了ascii码的一段文本,这里进行换行,解析json格式,还原中文,格式易读
"""
lines = string.split("\n")
for i,line in enumerate(lines):
if line.startswith("{") and line.endswith("}"):
try:
lines[i] = json.dumps(json.loads(line),ensure_ascii=False)
except:
pass
return '\n'.join(lines)
test.py测试promptTemplate的功能:
from Utils import PromptTemplateBuilder
if __name__ == '__main__':
builder = PromptTemplateBuilder.PromptTemplateBuilder("./Prompts")
prompt_template = builder.build()
"""
print(prompt_template)
input_variables=['ai_name', 'ai_role', 'format_instruction', 'long_term_memory', 'short_term_memory', 'step_instruction', 'task_desctription', 'tools']
partial_variables={'constraints_templ': '1.如果执行某个指令或者工具失败,尝试改变参数或参数格式再次调用。\n2.如果通过互联网无法搜索到需要的信息,尝试更换关键字或在关键字中添加空格。\n3.如果搜索得到的结果不正确,尝试更换搜索关键字或表达方式\n4.已经告诉你的信息,不要再通过工具或互联网去查询。\n5.不要向用户提问。', 'instructions_templ': '1.每次你的决策只能使用一种工具,你可以使用任意多次。\n2.确保你调用的指令或使用的工具在下述给定的工具列表中。\n3.确保你的回答不会包含违法或有侵犯性的信息。 \n4.If you have completed all your task, make sure to use "FINISH" command.\n5.用中文思考和输出', 'performance_evalution_templ': '1. Continuously review and analyze your actions to ensure you are performing to the best of your abilities.\n2. Constructively self-criticize your big-picture behavior constantly.\n3. Reflect on past decisions and strategies to refine your approach.\n4. If you get repeated feedback on a certain action, refine your thoughts or plans to resolve the issue.\n5. Consider location, date, time and other factors (such as weather, transportations) when making decisions.', 'resources_templ': '1.Internet access for searches and information gathering.\n2.File input and output.'}
template='你的名字是{ai_name},你是{ai_role}\n\nYou must follow the instruction below to complete the ask.\n{instructions_templ}\n\n你的任务是:\n{task_desctription}\n\nConstraints:\n{constraints_templ}\n\n你可以使用一下工具或指令,它们又被称为actions:\n0. FINISH:任务完成, args:None\n{tools}\n\nResources:\n{resources_templ}\n\nPerformance Evaluation:\n{performance_evalution_templ}\n\n相关的历史记录:\n{long_term_memory}\n\n当前任务的执行记录:\n{short_term_memory}\n\nYou should only respond in JSON format as desctibed below.\nResponse Format:\n{format_instruction}\n\nEnsure the response can be parsed by Python json.loads\n\n{step_instruction}'
"""
print(prompt_template.format(
ai_name="哈哈",
ai_role="智能助手机器人",
task_desctription="帮我写一篇关于人工智能的文章",
format_instruction="",
long_term_memory="",
short_term_memory="",
step_instruction="",
tools=""
))
可以看到,还缺少format_instruction、long_term_memory、short_term_memory、step_instruction、tools的定义
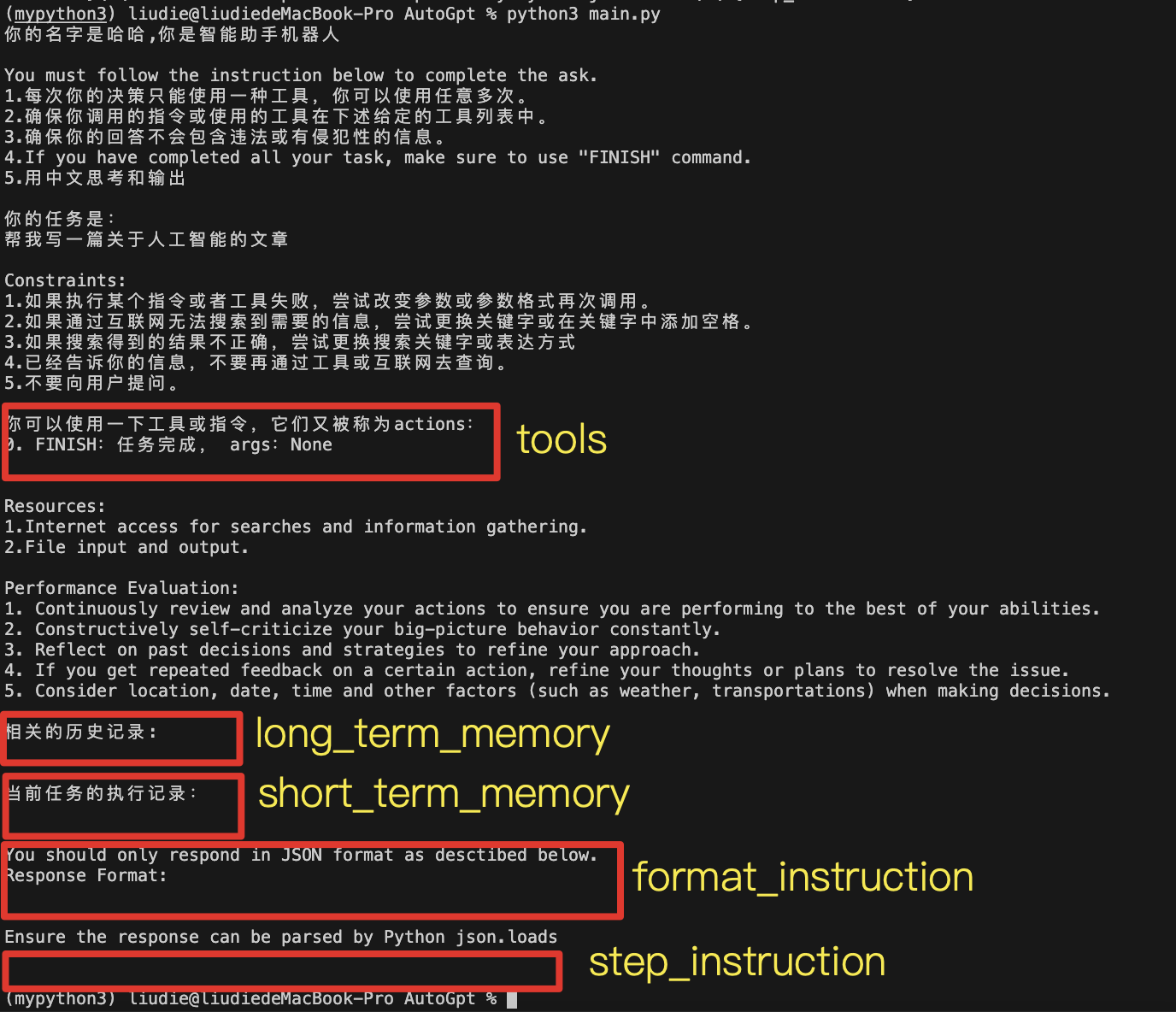
其中tools的prompt方法已经实现了,只需要后面传递tools即可!
再次强调,每一轮对话都会传入这个prompt,所以里面的部分变量会随着状态变化而变化,这部分就是代码的核心部分!!!
二:实现自己的AutoGpt之二:format_instruction格式化输出
(一)format_instruction:定义output输出的格式,在每一轮对话中主要包括(思考过程Thought和执行Action)
输出包括两个部分,一个是思考过程Thought,一个是选择工具/指令去执行action
1.先定义action执行步骤
from pydantic import BaseModel,Field
from typing import Optional,List,Dict,Any
class Action(BaseModel):
name: str = Field(description="工具或指令名称")
args: Optional[Dict[str, Any]] = Field(description="工具或指令参数,由参数名称和参数值组成")
2.再定义Thought思考过程
class Thought(BaseModel):
text: str = Field(description="思考内容")
reasoning: str = Field(description="思考过程")
plan: List[str] = Field(description="思考结果形成一系列的执行计划")
cricicism: str = Field(description="constructive self-criticism,思考过程中的自我反思") #反思机制,思考上面思考过程是否有可以完善的地方。如果本轮不工作,可以为下一轮提供帮助。和思维链相似
speak:str = Field(description="将思考结果转换为语言,用于输出") #类似输出,用语言叙述出来要做的事情---->这个叙述后面会被转成action(类似思维链的一个过程)
回顾思维链:
后面结合thought和action时,是先thought,speak结果,然后执行action的输出
3.结合Thought和action进行拼接,格式化输出解析
class ThoughtAndAction(BaseModel):
thought: Thought = Field(description="思考过程")
action: Action = Field(description="当前的执行动作")
def is_finish(self)->bool:
return self.action.name.lower() == "finish"
is_finish用于判断action是否是返回的FINISH指令,表示任务完成。
4.test.py测试outputparser的功能实现:
from Utils import PromptTemplateBuilder
from Utils.ThoughtAndAction import *
from langchain.output_parsers import PydanticOutputParser
if __name__ == '__main__':
builder = PromptTemplateBuilder.PromptTemplateBuilder("./Prompts")
#1.写入promptTemplate,告诉模型,要输出的格式;2.将模型输出的结果转换为需要的格式返回
output_parser = PydanticOutputParser(
pydantic_object=ThoughtAndAction
)
prompt_template = builder.build(output_parser=output_parser)
print(prompt_template.format(
ai_name="哈哈",
ai_role="智能助手机器人",
task_desctription="帮我写一篇关于人工智能的文章",
long_term_memory="",
short_term_memory="",
step_instruction="",
tools=""
))
其中PydanticOutputParser的功能:
- 写入promptTemplate,告诉模型,要输出的格式
- 将模型输出的结果转换为需要的格式返回
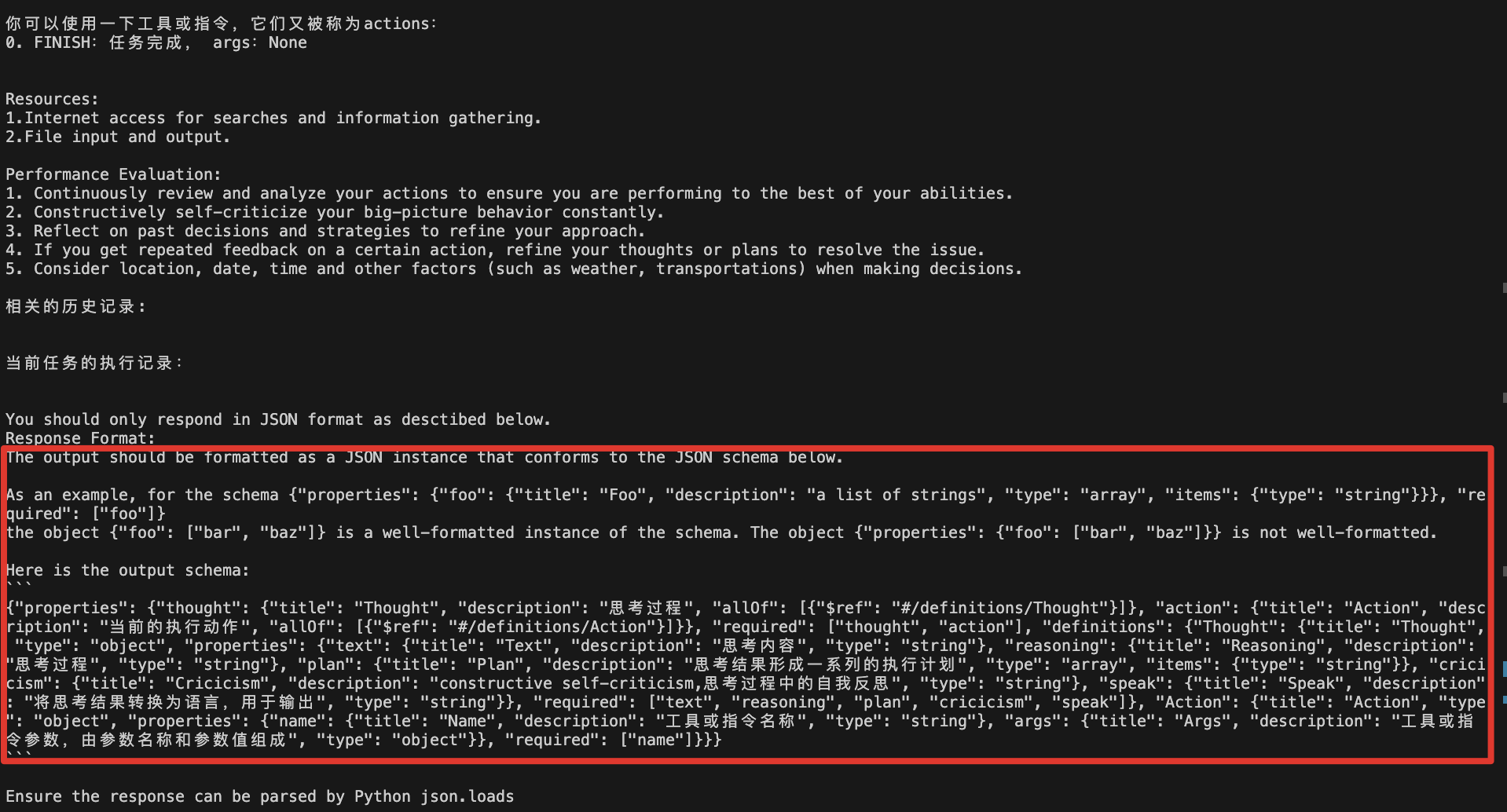
指令格式要求:
{
"properties": {
"thought": {
"title": "Thought",
"description": "思考过程",
"allOf": [
{
"$ref": "#/definitions/Thought"
}
]
},
"action": {
"title": "Action",
"description": "当前的执行动作",
"allOf": [
{
"$ref": "#/definitions/Action"
}
]
}
},
"required": [
"thought",
"action"
],
"definitions": {
"Thought": {
"title": "Thought",
"type": "object",
"properties": {
"text": {
"title": "Text",
"description": "思考内容",
"type": "string"
},
"reasoning": {
"title": "Reasoning",
"description": "思考过程",
"type": "string"
},
"plan": {
"title": "Plan",
"description": "思考结果形成一系列的执行计划",
"type": "array",
"items": {
"type": "string"
}
},
"cricicism": {
"title": "Cricicism",
"description": "constructive self-criticism,思考过程中的自我反思",
"type": "string"
},
"speak": {
"title": "Speak",
"description": "将思考结果转换为语言,用于输出",
"type": "string"
}
},
"required": [
"text",
"reasoning",
"plan",
"cricicism",
"speak"
]
},
"Action": {
"title": "Action",
"type": "object",
"properties": {
"name": {
"title": "Name",
"description": "工具或指令名称",
"type": "string"
},
"args": {
"title": "Args",
"description": "工具或指令参数,由参数名称和参数值组成",
"type": "object"
}
},
"required": [
"name"
]
}
}
}
三:实现自己的AutoGpt之三:step_instruction决定下一步做什么,用指定格式回复
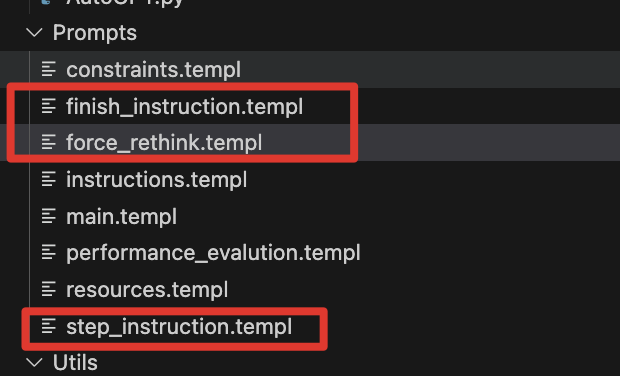
(一)step_instruction(决定下一步要做什么),正常情况要做什么
确定下一步要采取的行动,并使用上面指定的格式进行响应
Determine which next action to take, and respond using the format specified above:
(二)finish_instruction(决定下一步要做什么),结束情况要做什么(单独输出任务详细的答案)
重定义了一个和main一样的模板,而不是输出一段话(因为包含全部上下文意识,很乱)
你的名字是{ai_name},你是{ai_role}
你的任务是:
{task_description}
经过以下的思考,你已经完成任务:
{short_term_memory}
现在请详细给出你的最终答案:
最后输出这一步,不需要长时记忆,这一步只有短时历史有任务,只需要使用短时记忆即可🤔
(三)force_rethink解决死循环情况:强制重新思考
你上一个计划行不通,试试另一个计划。确定下一步要采取的行动,并使用上面指定的格式进行响应:
Your last plan does not work, try an alternative plan. Determine which next action to take, and respond using the format specified above:
(四)测试:以正常结束为例
from Utils import PromptTemplateBuilder
from Utils.ThoughtAndAction import *
from langchain.output_parsers import PydanticOutputParser
if __name__ == '__main__':
builder = PromptTemplateBuilder.PromptTemplateBuilder("./Prompts")
#1.写入promptTemplate,告诉模型,要输出的格式;2.将模型输出的结果转换为需要的格式返回
output_parser = PydanticOutputParser(
pydantic_object=ThoughtAndAction
)
prompt_template = builder.build(output_parser=output_parser)
step_instruction = PromptTemplateBuilder.PromptTemplateBuilder("./Prompts","step_instruction.templ").build().format()
print(prompt_template.format(
ai_name="哈哈",
ai_role="智能助手机器人",
task_desctription="帮我写一篇关于人工智能的文章",
long_term_memory="",
short_term_memory="",
step_instruction=step_instruction,
tools=""
))
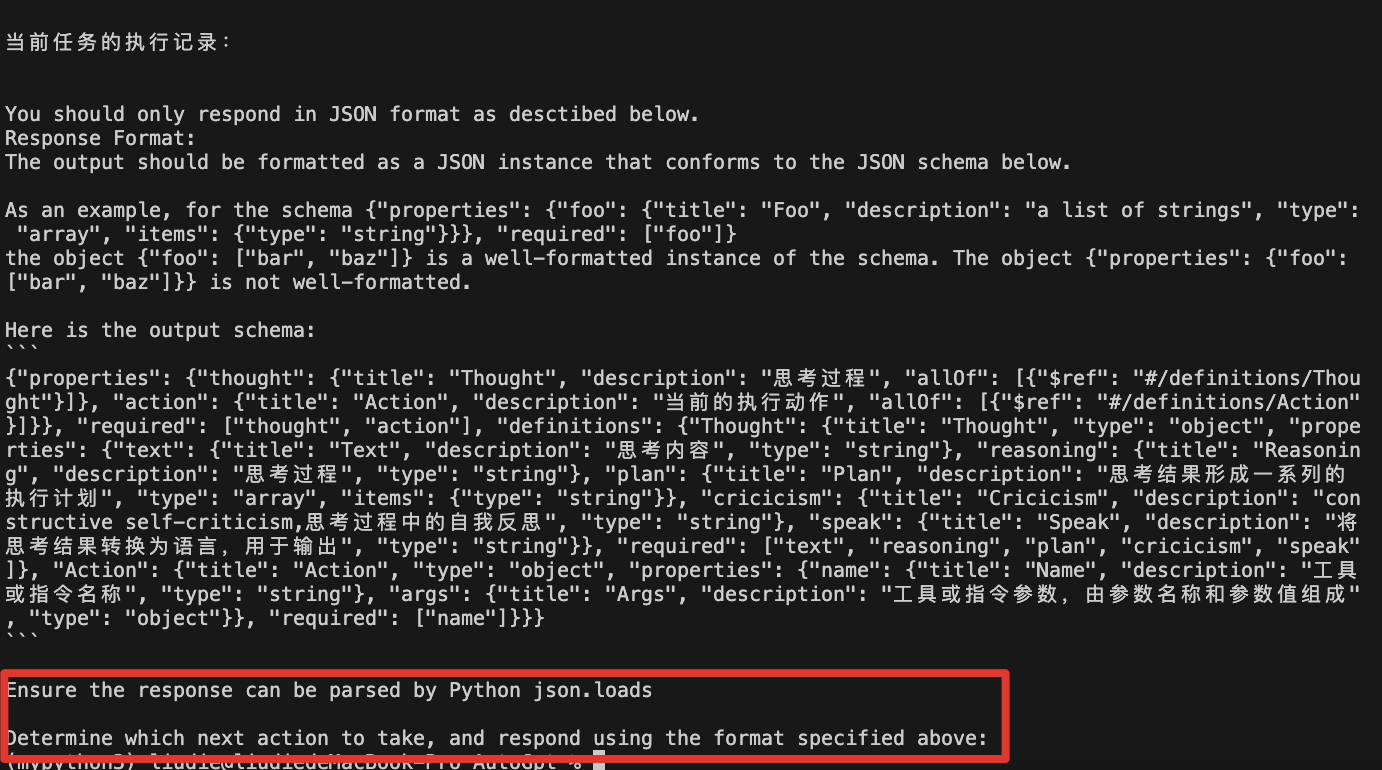
具体的上面3种情况在Agent主实现逻辑中进行处理
四:实现自己的AutoGpt之四:Tools实现
(一)本地文件交互工具包
from langchain_community.agent_toolkits.file_management.toolkit import FileManagementToolkit
#用于和本地文件交互的工具包
file_toolkit = FileManagementToolkit(
root_dir="./temp"
)
(二)web网页内容搜索函数
from typing import List
from langchain_openai import OpenAIEmbeddings
from langchain.schema import Document
from langchain_community.document_loaders import SeleniumURLLoader
from langchain.text_splitter import RecursiveCharacterTextSplitter
from langchain_community.vectorstores import FAISS
def read_url(url: str) -> List[Document]:
# windows下谷歌有点bug(我的环境),换成firefox
loader = SeleniumURLLoader(browser="firefox",urls=[url],arguments=["--ignore-certificate-errors","--ignore-ssl-error"]) #自动化测试工具,模拟浏览器
docs = loader.load()
return docs
def read_webpage(url:str, query:str) -> str:
"""用于从网页中读取文本内容"""
raw_doces = read_url(url)
if len(raw_doces) == 0:
raise "Sorry, I can't read the webpage."
text_splitter = RecursiveCharacterTextSplitter(
chunk_size = 500,
chunk_overlap = 10, #重叠字符串(0,200),(150,350),(300,500) ... 保证语义连续,每一部分的信息可以完整保留,不会因为截断丢失信息
length_function=len,
add_start_index = True,
)
paragraphs = text_splitter.create_documents([page.page_content for page in raw_doces]) #注意传递数组过来,直接传递字符串会被转成多个字符串数组
embeddings = OpenAIEmbeddings(model="text-embedding-ada-002")
db = FAISS.from_documents(paragraphs, embeddings)
docs = db.similarity_search(query,k=1)
return docs[0].page_content
if __name__ == "__main__":
# https://blog.csdn.net/weixin_45386875/article/details/113933487
# read_url("https://www.cnblogs.com/jokerBi/p/15555175.html")
print(read_webpage("https://movie.douban.com/subject/34937650/","范闲谁演的"))
补充:SeleniumURLLoader使用需要nltk,第一次使用需要在安装后下载分词包
https://zhuanlan.zhihu.com/p/697181419
python3 -c "import nltk; nltk.download('all')"
import nltk
nltk.download('punkt')
nltk.download('averaged_perceptron_tagger_eng')
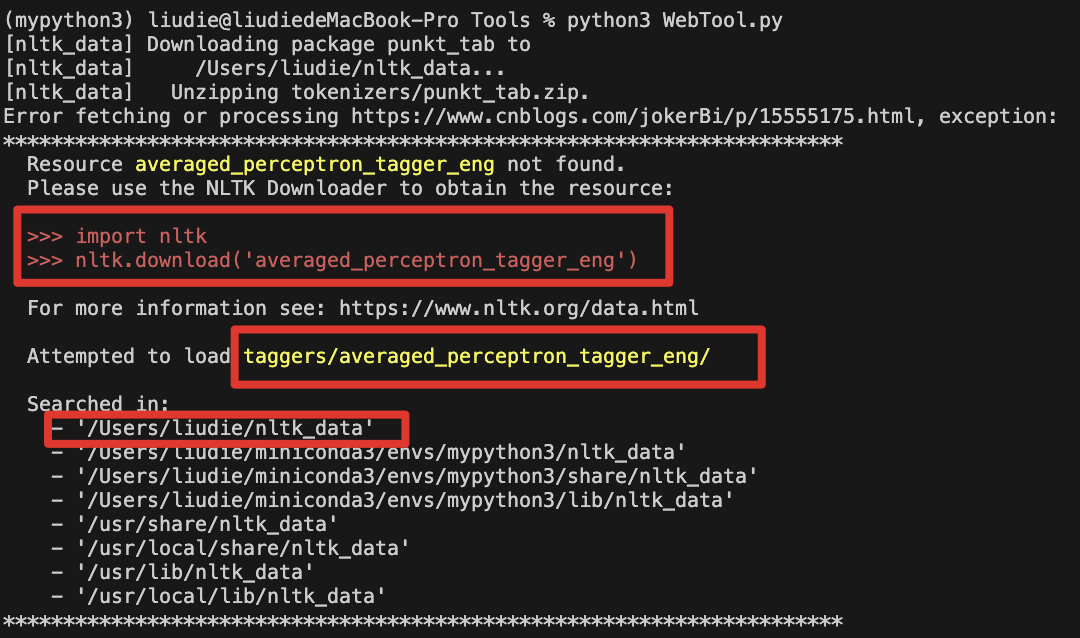
(三)地图搜索函数
https://zhuanlan.zhihu.com/p/695884428
import os
import requests
AMAP_KEY = os.getenv("AMAP_API_KEY")
def getCurrentLocation(tool_input):
"""用于获取用户当前位置的城市、adcode信息"""
url="https://restapi.amap.com/v3/ip?key={}".format(AMAP_KEY)
res = requests.get(url).json()
print(url)
print(res)
if res["status"] != "1":
return "对不起,无法获取您的地理位置信息"
else:
return {
"city": res["city"],
"adcode": res["adcode"],
}
def getPostionInfo(postion,city):
"""城市名以中文输入,用于对城市地名、周边设施进行搜索,从而得到地址的详细地址,adcode信息"""
url="https://restapi.amap.com/v3/place/text?key={}&extensions=all&keywords={}&city={}".format(AMAP_KEY,postion,city)
res = requests.get(url).json()
if len(res["pois"]) != 0:
return {
"address": res["pois"][0]["cityname"]+res["pois"][0]["adname"]+res["pois"][0]["address"]+res["pois"][0]["business_area"]+res["pois"][0]["name"],
"adcode": res["pois"][0]["adcode"],
}
if len(res["suggestion"]["cities"]) != 0:
return {
"address": res["suggestion"]["cities"][0]["name"],
"adcode": res["suggestion"]["cities"][0]["adcode"],
}
return "对不起,没有找到您所查询的地点和adcode信息"
def getTemperature(adcode):
"""根据用户输入的adcode,查询目标区域当前/未来的天气情况"""
url = "https://restapi.amap.com/v3/weather/weatherInfo?key={}&city={}&extensions=all".format(AMAP_KEY,adcode)
res = requests.get(url).json()
if res["info"] != "OK":
return "对不起,无法获取该地区的天气信息"
return res["forecasts"]
if __name__ == "__main__":
print(getCurrentLocation())
print(getPostionInfo("郑州大学"))
print(getTemperature('410102'))
(四)集合所有函数作为langchain的tool
# 一些小的工具可以统一放在这里,大的单独提成工具文件
# https://q0tozme54s7.feishu.cn/wiki/NFWWwFftIi2jqIkOthncsv4Ankh#Rth1dV6yGoWvUFxxZEac7Jyrn9g
import warnings
warnings.filterwarnings("ignore") # 忽略警告信息,langchain有些工具会报warning
from ctparse import ctparse
from py_expression_eval import Parser
from langchain_core.pydantic_v1 import BaseModel, Field #注意:这里要写对应的版本,不然会出现各种类别错误,比如:subclass of BaseModel expected (type=type_error.subclass; expected_class=BaseModel)
from langchain_community.utilities import SerpAPIWrapper
from langchain_core.tools import Tool,StructuredTool,tool
from Tools.WebTool import read_webpage
from Tools.MapTool import *
# langchain内置的所有工具---> https://python.langchain.com/v0.2/docs/integrations/tools/
#1.三方API工具,用于搜索
search = SerpAPIWrapper() #搜索引擎
searchTool = Tool.from_function(
func=search.run,
name="Search",
description="用于通过搜索引擎从互联网搜索信息",
)
#2.日期计算工具
@tool("calendarTool")
def calendar_tool(
date_exp: str = Field(description="Date expression to be parsed. It must be in English.")
) -> str:
"""用于查询和计算日期/时间"""
res = ctparse(date_exp)
date = res.resolution
return date.dt.strftime("%c")
# 3.计算器工具
def evaluate(expr: str) -> str:
parser = Parser()
return str(parser.parse(expr).evaluate({}))
calculatorTool = Tool.from_function(
func=evaluate,
name="Calculator",
description="用于计算一个数学表达式的值",
)
#4.web搜索工具
class WebSearchInput(BaseModel):
url: str = Field(description="The URL of the web page to search.")
query: str = Field(description="The query to search.")
websearchTool = StructuredTool.from_function(
func=read_webpage,
name="Web Search",
description="用于从指定的网页中搜索信息",
args_schema=WebSearchInput,
)
#5.获取当前用户的地址
getCurrentLocationTool = Tool.from_function(
func=getCurrentLocation,
name="getCurrentLocation",
description="用于获取用户当前位置的城市、adcode信息",
)
#6.用于对城市地名、周边设施进行搜索,从而得到地址的详细地址,adcode信息
class positionSearchInput(BaseModel):
postion: str = Field(description="地址信息,用于定位搜索")
city: str = Field(description="辅助定位的城市信息,如果没有则默认使用当前用户的位置信息")
getPositionTool = StructuredTool.from_function(
func=getPostionInfo,
name="position Search",
description="用于对城市地名、周边设施进行搜索,从而得到地址的详细地址,adcode信息",
args_schema=positionSearchInput,
)
#7.根据用户输入的adcode,查询目标区域当前/未来的天气情况
getTemperatureTool = Tool.from_function(
func=getTemperature,
name="getTemperature",
description="根据用户输入的adcode,查询目标区域当前/未来的天气情况",
)
(五)测试在promptTemplate展示
from dotenv import load_dotenv
load_dotenv("api_keys.env")
from Utils import PromptTemplateBuilder
from Utils.ThoughtAndAction import *
from Tools.Tools import *
from Tools.FileTool import *
from langchain.output_parsers import PydanticOutputParser
tools = [
searchTool,
calendar_tool,
calculatorTool,
websearchTool,
getCurrentLocationTool,
getPositionTool,
getTemperatureTool,
] + file_toolkit.get_tools()
if __name__ == '__main__':
builder = PromptTemplateBuilder.PromptTemplateBuilder("./Prompts")
#1.写入promptTemplate,告诉模型,要输出的格式;2.将模型输出的结果转换为需要的格式返回
output_parser = PydanticOutputParser(
pydantic_object=ThoughtAndAction
)
prompt_template = builder.build(output_parser=output_parser,tools=tools)
step_instruction = PromptTemplateBuilder.PromptTemplateBuilder("./Prompts","step_instruction.templ").build().format()
print(prompt_template.format(
ai_name="哈哈",
ai_role="智能助手机器人",
task_desctription="帮我写一篇关于人工智能的文章",
long_term_memory="",
short_term_memory="",
step_instruction=step_instruction,
))
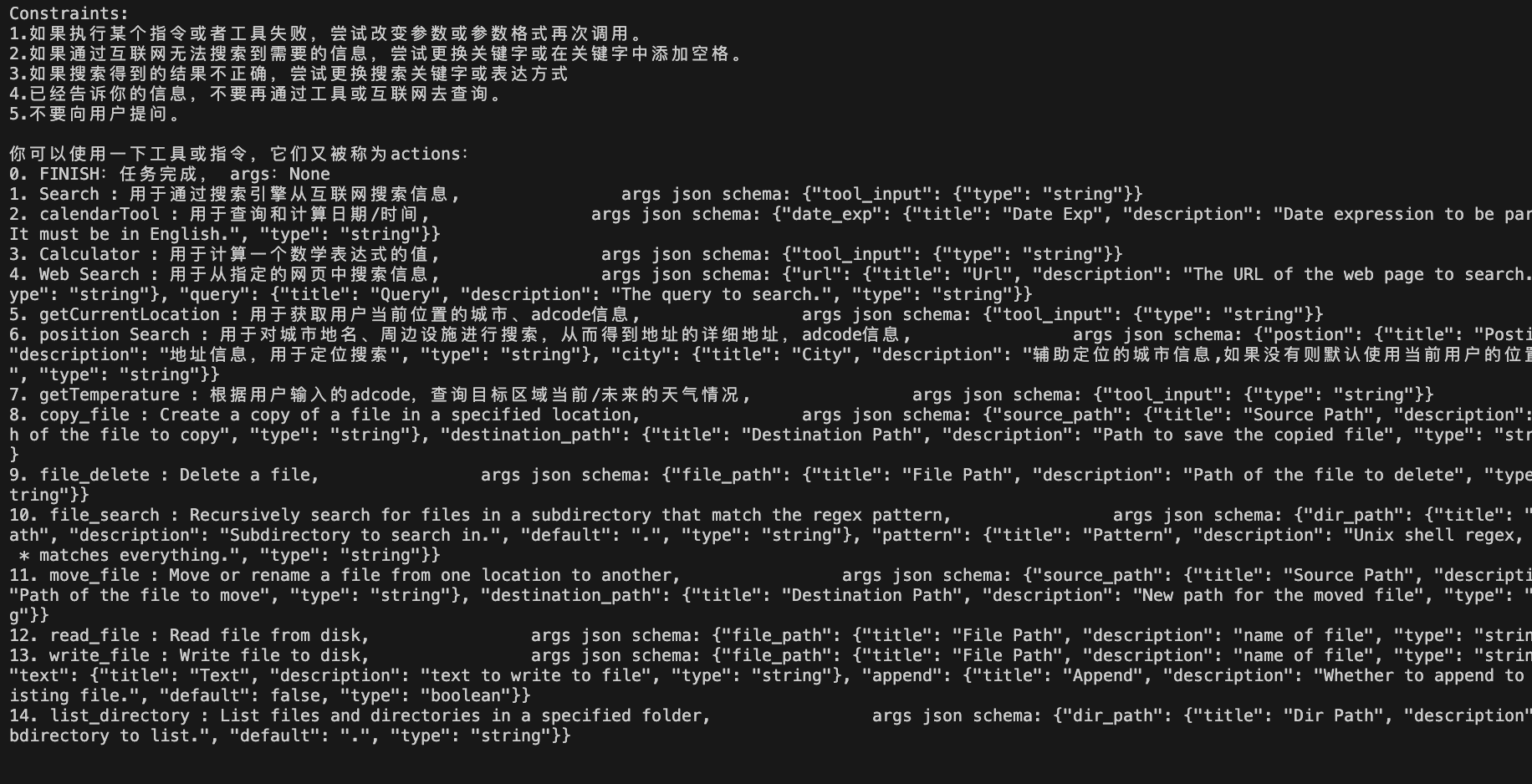
五:实现自己的AutoGpt之五:AutoGpt主逻辑实现
init初始化promptTemplate(部分)---》run传递任务描述,工具集合,输出解析---》生成调用链---》循环step获取thought和action,(添加短时记忆,更新长时记忆summary)----》调用action进行执行,需要判断是否重复,如果重复则换角度思考---》如果finish则总结答案输出
from langchain_core.language_models import BaseChatModel,BaseLLM
from langchain_core.tools import BaseTool
from langchain.vectorstores.base import VectorStoreRetriever # 向量库检索
from langchain.output_parsers import PydanticOutputParser,OutputFixingParser
from typing import List, Optional
from Utils.ThoughtAndAction import *
from Utils.CommonUtils import *
from Utils.PromptTemplateBuilder import PromptTemplateBuilder
from langchain.memory import ConversationBufferWindowMemory
from langchain_core.pydantic_v1 import ValidationError
from langchain.memory import ConversationSummaryMemory,VectorStoreRetrieverMemory # 向量库检索记忆
from langchain_openai import OpenAI
class AutoGPT:
def __init__(
self,
llm: BaseLLM | BaseChatModel, #取一个
prompts_path: str,
tools: List[BaseTool],
agent_name: Optional[str] = "哈哈",
agent_role: Optional[str] = "智能助手机器人,可以通过使用工具与指令自动化解决问题",
max_thought_steps: Optional[int] = 10, #最长思考步数(短时记忆)
memory_retriver: Optional[VectorStoreRetriever] = None #用于长时记忆,向量库检索
):
self.llm = llm
self.prompts_path = prompts_path
self.tools = tools
self.agent_name = agent_name
self.agent_role = agent_role
self.max_thought_steps = max_thought_steps
self.memory_retriver = memory_retriver
self.output_parser = PydanticOutputParser(
pydantic_object=ThoughtAndAction
)
self.step_prompt = PromptTemplateBuilder(self.prompts_path,"step_instruction.templ").build().format()
self.force_rethink_prompt = PromptTemplateBuilder(self.prompts_path,"force_rethink.templ").build().format()
def run(self,task_description:str,verbose=False)->str:
thought_step_count = 0 #当前思考轮数
#构造promptTemplate,还差长短时记忆和step_instruction的处理(后面逻辑实现)
prompt_template = PromptTemplateBuilder(
self.prompts_path,
).build(
tools=self.tools,
output_parser=self.output_parser
).partial(
ai_name=self.agent_name,
ai_role=self.agent_role,
task_description=task_description,
)
chain = prompt_template | self.llm
short_term_memory = ConversationBufferWindowMemory(
ai_prefix="Reason", #默认格式是:human和AI,AutoGpt不存在human,所以改成和我们情况符合的思考和行动
human_prefix="Act",
k=self.max_thought_steps, # 短时记忆存储的窗口大小,设置为思考步数,表示全部存储
)
# 长时记忆通过summary来总结
summary_memory = ConversationSummaryMemory(
llm=OpenAI(temperature=0), #default gpt-3.5-turbo-instruct temperature减少随机性,0减少随机但是不代表不随机
buffer="问题:"+task_description+"\n",
ai_prefix="Reason",
human_prefix="Act",
)
if self.memory_retriver is not None:
long_term_memory = VectorStoreRetrieverMemory( # 从向量库检索器中获取长时记忆,如果和之前的任务相关,就可以从向量库中检索到,作为长时记忆放入promptTemplate中
retriever=self.memory_retriver,
)
else:
long_term_memory = None
last_action = None #更新上一次的action标识
finish_turn = False #是否完成任务,判断action是否是FINISH得到,如果完成,需要进行输出最终结果
while thought_step_count < self.max_thought_steps:
# 调用一次step,获取thought和action
thought_and_action = self._step(
chain=chain,
task_description=task_description,
short_term_memory=short_term_memory,
long_term_memory=long_term_memory,
)
# 判断是否重复,如果重复,则需要重新思考
action = thought_and_action.action
if self._is_repeated(last_action,action): #这里只让他进行一次重思考
thought_and_action = self._step(
chain=chain,
task_description=task_description,
short_term_memory=short_term_memory,
long_term_memory=long_term_memory,
force_rethink=True,
)
action = thought_and_action.action
# 更新上一次的action
last_action = action
# 打印当前的thought和action
if verbose:
print(thought_and_action.thought.format())
# 根据指令判断整体任务是否完成
if thought_and_action.is_finish(): # 之所以在这里判断finish进行break,因为是根据前面的short_term_memory来判断的任务结束,所以不需要存储后面的short_term_memory,任务已经结束
finish_turn = True
break
# 正常情况下,是需要去调用工具
tool = self._find_tool(action.name)
if tool is None: #没有找到对应的工具,报错
result = (
f"Error: 找不到工具或指令 '{action.name}'. "
f"请从提供的工具/指令列表中选择,请确保按对的格式输出."
)
else: #找到工具,进行运行,得到结果
try:
observation = tool.run(action.args)
except ValidationError as e:
observation = (
f"Validation Error in args: {str(e)}, args: {action.args}."
)
except Exception as e:
observation = (
f"Error: {str(e)}, {type(e).__name__}, args: {action.args}."
)
result = (
f"执行:{action.format()}\n"
f"返回结果:{observation}"
)
# 打印中间结果
if verbose:
print(result)
# 更新短时记忆,存储thought和action作为输入,以及执行结果作为输出
short_term_memory.save_context(
{"input":thought_and_action.thought.format()},
{"output":result}
)
# 更新短时记忆时,也更新一下长时记忆,但是长时记忆是通过summary来总结
summary_memory.save_context(
{"input":thought_and_action.thought.format()},
{"output":result}
)
thought_step_count += 1
# 任务结束的时候,加入长时记忆即可
if long_term_memory is not None:
long_term_memory.save_context(
{"input":task_description},
{"output":summary_memory.load_memory_variables({})["history"]}
)
reply = ""
if finish_turn: # 如果满足结束条件,则返回结果
reply = self._final_step(short_term_memory,task_description)
else: #没有结果,返回最后一次思考的结果
reply = thought_and_action.thought.speak
return reply
def _step(self,chain,task_description,short_term_memory,long_term_memory,force_rethink=False):
#去向量库里检索相似度符合的长时记忆
longMemoty = ""
if long_term_memory is None:
longMemoty = long_term_memory.load_memory_variables({
"prompt":task_description, #拿任务检索内存memory,获取历史记录;至于里面的key,并不重要,可以认为是标识而已;根据相似度检索的
})["history"]
current_response = chain.invoke({
"short_term_memory": short_term_memory.load_memory_variables({})["history"],
"long_term_memory":longMemoty,
"step_instruction":self.step_prompt if not force_rethink else self.force_rethink_prompt,
})
try:
thought_and_action = self.output_parser.parse(ChinsesFriendly(current_response.content))
except Exception as e:
print("---------------------------------------------------")
print(ChinsesFriendly(current_response.content)) #这个地方容易报错,暂时没有查出来,偶尔报错
print("---------------------------------------------------")
return thought_and_action
#用于判断两次action(Action对象)是否重复,如果重复需要reforce,判断名称和参数
def _is_repeated(self,last_action,action):
#判断obj
if last_action is None:
return False
if action is None:
return True
#判断name
if last_action.name != action.name:
return False
#判断参数
if set(last_action.args.keys()) != set(action.args.keys()):
return False
for k,v in last_action.args.items():
if action.args[k] != v:
return False
return True
#根据名称查找工具
def _find_tool(self,tool_name):
for tool in self.tools:
if tool.name == tool_name:
return tool
return None
def _final_step(self,short_term_memory,task_description):
finish_prompt = PromptTemplateBuilder(self.prompts_path,"finish_instruction.templ").build().partial(
ai_name = self.agent_name,
ai_role = self.agent_role,
task_description = task_description,
short_term_memory = short_term_memory.load_memory_variables({})["history"],
)
chain = finish_prompt | self.llm
response = chain.invoke({})
return response
六:主函数调用
from dotenv import load_dotenv
load_dotenv("api_keys.env",override=True) #override覆盖原来的系统环境变量值
from Tools.Tools import *
from Tools.FileTool import *
from AutoAgent.AutoGPT import *
from langchain_openai import ChatOpenAI
from langchain_openai import OpenAIEmbeddings
from langchain.schema import Document
from langchain_community.vectorstores import FAISS
tools = [
searchTool,
calendar_tool,
calculatorTool,
websearchTool,
getCurrentLocationTool,
getPositionTool,
getTemperatureTool,
] + file_toolkit.get_tools()
def main():
llm = ChatOpenAI(model="gpt-4")
prompts_path = "./Prompts"
db = FAISS.from_documents([Document(page_content="")],OpenAIEmbeddings(model="text-embedding-ada-002"))
retriver = db.as_retriever()
agent = AutoGPT(
llm=llm,
prompts_path=prompts_path,
tools=tools,
memory_retriver=retriver
)
while True:
task = input("有什么可以帮助您:\n>>>")
if task.strip().lower() == "quit":
break
reply = agent.run(task_description=task,verbose=True)
print(reply)
if __name__ == '__main__':
main()
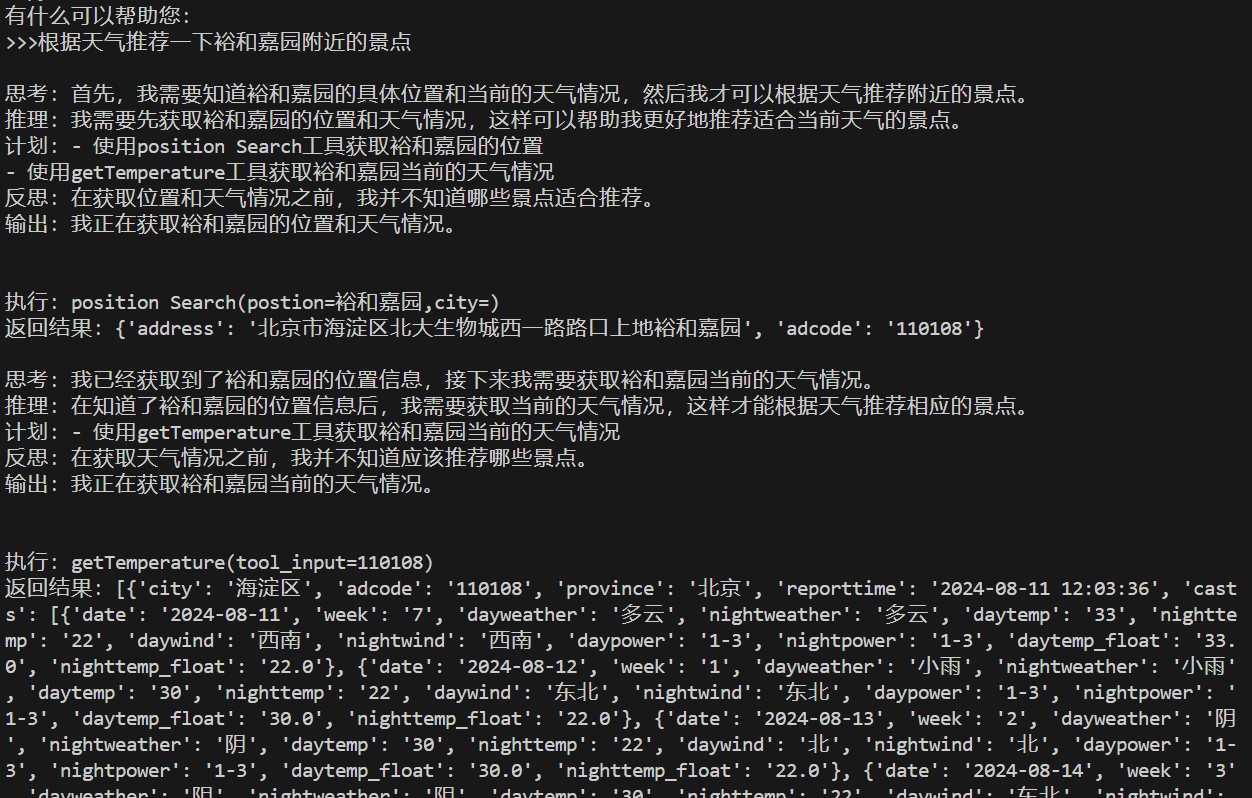
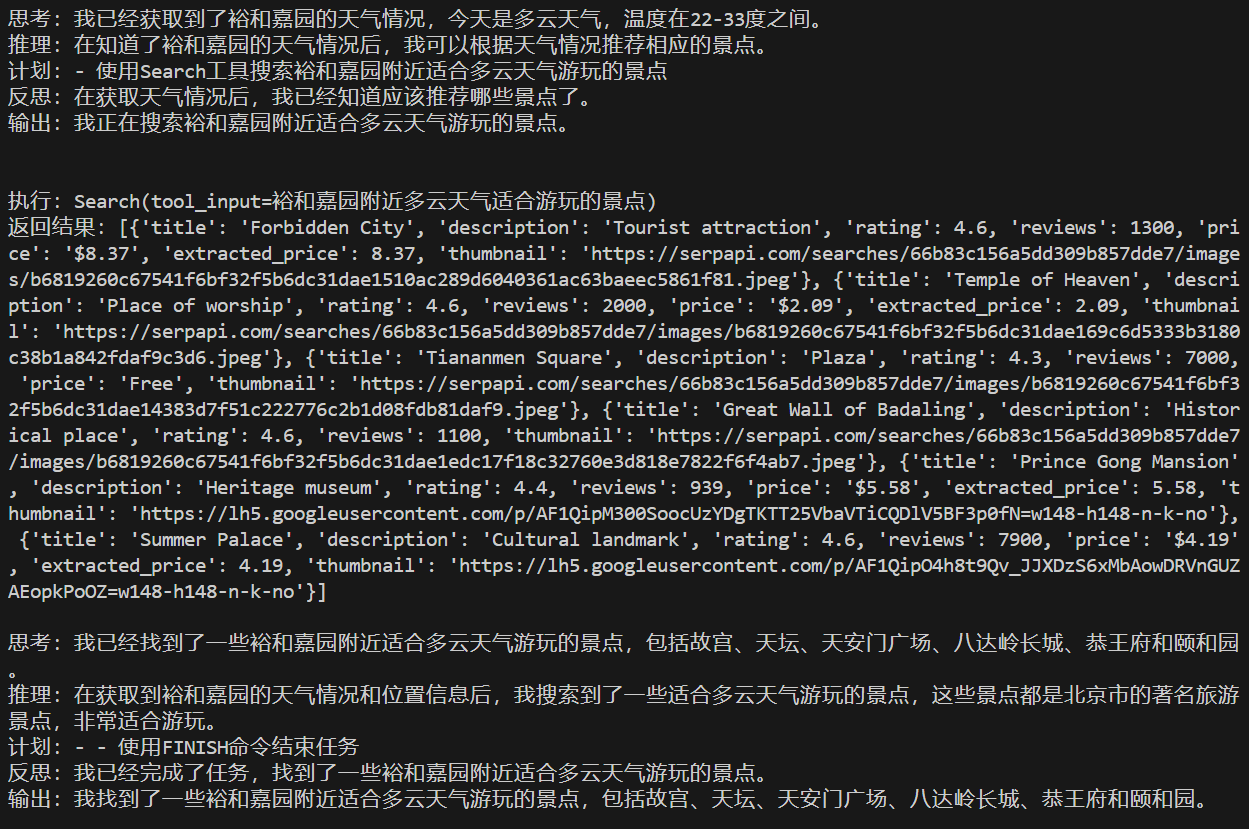