ViT简述【Transformer】
Transformer在NLP任务中表现很好,但是在CV任务中应用还很有限,基本都是作为CNN的一个辅助,Vit尝试使用纯Transformer结构解决CV的任务,并成功将其应用到了CV的基本任务--图像分类中。
因此,简单而言,这篇论文的主旨就是,用Transformer结构完成图像分类任务。
图像分类
图像分类,给定一张图片,输出一个概率向量p
,如下图所示,p
的每一个值为某个类别的概率值,如下图预测该图片为dog
的概率为40%。
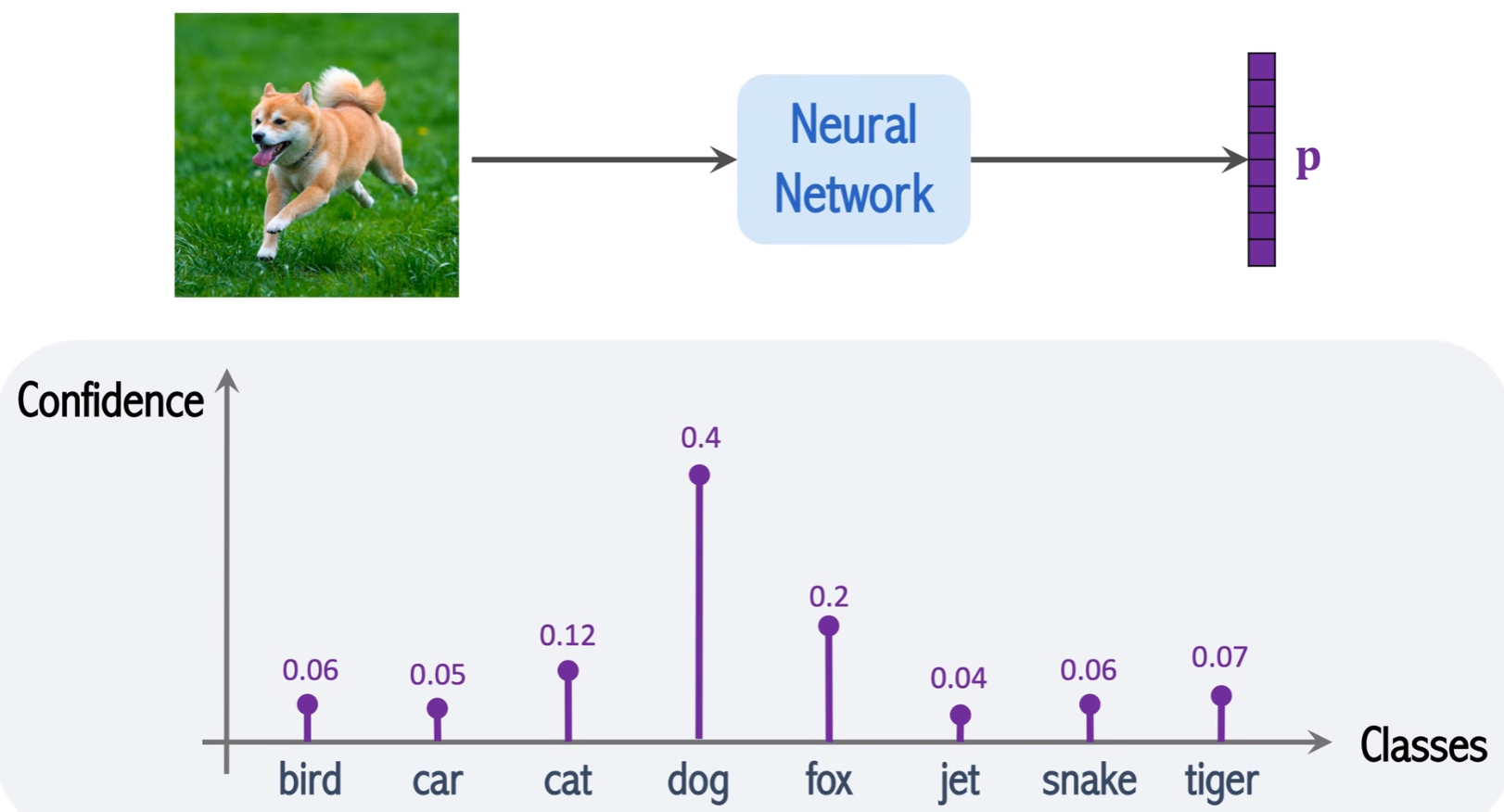
结构概述
基本结构如下:
核心要点:
- 图像切patch
- Patch0
- Position Embedding
- Multi-Head Attention
图像切patch
在NLP任务中,将自然语言使用Word2Vec转为向量(Embedding)送入模型进行处理,在CV中没有对应的序列化token,因此作者采用将原始图像切分为多个小块,然后将每个小块儿内的信息展平的方式。
- 切块:
需要指定两个参数:- patch_size:切小块,每个小块儿的尺寸
- stride:切小块儿的步长
假设输入的shape为:(1, 3, 288, 288)
,stride=32, patch_size=32,
则上述图片被切分为9个小块,则每个小块的shape为:(1, 3, 32, 32)
- flatten:然后将每个小块展平,则每个小块为
(1, 3072)
,有9个小块 - Linear Projection of Flattened Patched:将shape为
(1, 9, 3072)
向量线性映射,输出shape为(1, 9, 1024)
, - 加Position Embedding,得到
Transformer Encoder
的输入,shape为(1, 10, 1024)
,也就是图中Embedded Patches
的shape。
Patch0
为什么需要有Patch0?
这是因为需要对1-9个patches信息的整合,最后送入MLP Head的只有Patch0。
Position Embedding
图像被切分和展开后,丢失了位置信息,对于图像处理任务来说,这是很怪异的,因此,作者这里采用在每个Patch上增加一个位置信息的方式,将位置信息纳入考虑。
Multi-Head Attention
参考Attention的基本结构。
王树森Attention与Self-Attention学习笔记
王树森Transformer学习笔记
代码[Pytorch]
import torch
from vit_pytorch import ViT
v = ViT(
image_size = 256,
patch_size = 32,
num_classes = 1000,
dim = 1024,
depth = 6,
heads = 16,
mlp_dim = 2048,
dropout = 0.1,
emb_dropout = 0.1
)
img = torch.randn(1, 3, 256, 256)
preds = v(img)
print(preds.shape) # 1000,与ViT定义的num_classes一致
ViT类参数解析:
- dim:Linear Projection的输出维度:1024
- depth:有多少个Transformer Blocks
- heads:Multi-Head的Head数
- mlp_dim:Transformer Encoder内部的MLP的维度
- dropout
- ......
ViT的forward
函数:
def forward(self, img):
x = self.to_patch_embedding(img)
b, n, _ = x.shape
cls_tokens = repeat(self.cls_token, '1 1 d -> b 1 d', b = b)
x = torch.cat((cls_tokens, x), dim=1)
x += self.pos_embedding[:, :(n + 1)]
x = self.dropout(x)
x = self.transformer(x)
x = x.mean(dim = 1) if self.pool == 'mean' else x[:, 0]
x = self.to_latent(x)
return self.mlp_head(x)
输入端的切分主要由下面这句话完成:
x = self.to_patch_embedding(img)
==>
self.to_patch_embedding = nn.Sequential(
Rearrange('b c (h p1) (w p2) -> b (h w) (p1 p2 c)', p1 = patch_height, p2 = patch_width),
nn.LayerNorm(patch_dim),
nn.Linear(patch_dim, dim),
nn.LayerNorm(dim),
)
#由传入参数: image_size = 256, patch_size = 32
# Rearrange完成的shape变换为(b, c, 256, 256) -> (b, 64, 1024*c)
# nn.LayerNorm
# nn.Linear: (b, 64, 1024*c) --> (b, 64, 1024)
Rearrange用更加可理解的方式实现transpose的功能:
We don't write:
y = x.transpose(0, 2, 3, 1)
We write comprehensible code:
y = rearrange(x, 'b c h w -> b h w c')
实验结果
Vit的训练,首先在数据集A上做预训练,然后在数据集B【任务数据集】上做微调,最后在数据集B的测试集上做测试。
Pretrain the model on Dataset A, fine-tune the model on Dataset B, and evaluate the model on Dataset B.
结果显示,预训练的数据集越大Vit的效果越好。
Pretrained on ImageNet(small), Vit is slightly worse than ResNet.
Pretrained on ImageNet-21K(medium), Vit is comparable to ResNet.
Pretrained on JFT(large), Vit is slightly better than ResNet.
也就是说,Transformer的模型需要大数据集做预训练,随着预训练数据集的增长,Transformer的准确率会随之增长,ResNet在预训练数据集超过100M之后就不如Transformer了。
Reference
- 王树森Vit介绍
- Dosovitskiy et al. An image is worth 16x16 words: transformers for image recognition at scale. In ICLR, 2021.
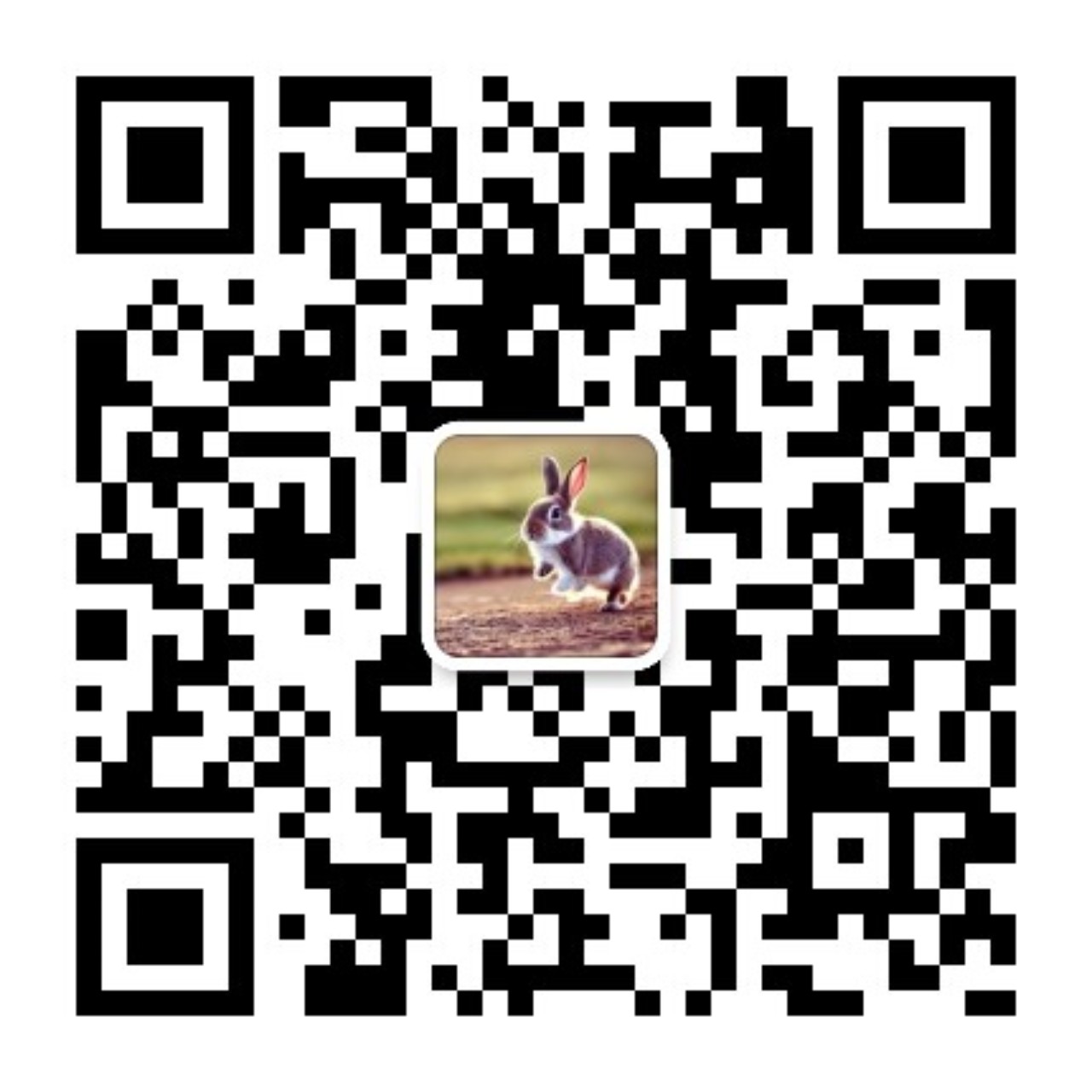
关注我的公众号 不定期推送资讯,接受私信许愿