Ablation Study
We often come across 'ablation study' in machine learning papers, for example, in this paper with the original R-CNN, it has a section of ablation studies. But what does this means?
Well, we know that when we build a model, we usually have different components of the model. If we remove some component of the model, what's the effect on the model? This is a very coarse definition of ablation study - we want to see the contributions of some proposed components in the model by comparing the model including this component with that without this component.
In the above paper, in order to see the effect of fine-tuning of the CNN, the authors analyzed the performance of the model with the fine-tuning and the performance of it without the fine-tuning. This way, we can easily see the effect of the fine-tuning.
The following I copied from the answer of Jonathan Uesato on Quora, it explains very well:
- An LSTM has 4 gates: feature, input, output, forget. We might ask: are all 4 necessary? What if I remove one? Indeed, lots of experimentation has gone into LSTM variants, the GRU being a notable example (which is simpler).
- If certain tricks are used to get an algorithm to work, it’s useful to know whether the algorithm is robust to removing these tricks. For example, DeepMind’s original DQN paper reports using (1) only periodically updating the reference network and (2) using a replay buffer rather than updating online. It’s very useful for the research community to know that both these tricks are necessary, in order to build on top of these results.
- If an algorithm is a modification of a previous work, and has multiple differences, researchers want to know what the key difference is.
- Simpler is better (inductive prior towards simpler model classes). If you can get the same performance with two models, prefer the simpler one.
如果这篇文章帮助到了你,你可以请作者喝一杯咖啡
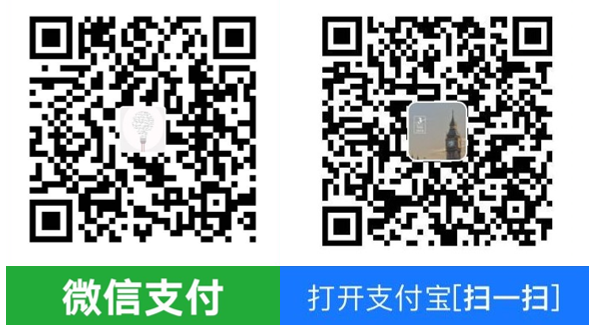