联邦学习常用数据集
今天开始跑联邦学习论文实验了,这里介绍一下论文的常用benchmark数据集(因为我的研究领域是联邦/分布式学习,所以下面列出的数据集可能偏向这方面,做其他方向的童鞋参考下就好)。
1. CV数据集
(1)FEMINIST
任务:手写字符识别
参数说明: 62种不同的字符类别 (10种数字, 26种小写, 26种大写)的像素(灰度)图片, 图片全为28乘28像素大小 (可以选择将其转为128×128), 总样本数805263,可使用官方给出的代码按3500个client非独立同分布划分。
介绍: FEMNIST数据集全名Federated-MNIST, 属于专门给联邦学习用的基准数据集leaf的成员之一。
官方数据预处理与划分代码:https://github.com/TalwalkarLab/leaf
引用方式:S Caldas, LEAF: A Benchmark for Federated Settings, 2018.
获取方式:采用脚本获取
wget https://s3.amazonaws.com/nist-srd/SD19/by_class.zip
wget https://s3.amazonaws.com/nist-srd/SD19/by_write.zip
(2)EMINIST
任务:手写字符识别
参数说明: 按照byclass方式split的话是62种不同的字符类别(各类别数量不均衡) (10种数字, 26种小写, 26种大写)的像素(灰度)图片, 图片全为28乘28像素大小, 样本数814255,在联邦学习场景下需要自行设计划分算法进行划分。
介绍: EMNIST数据集全名extension of MNIST,是MINIST数据集的扩展版。
官网:https://www.nist.gov/itl/products-and-services/emnist-dataset
引用方式:Cohen G, EMNIST: an extension of MNIST to handwritten letters, 2017
获取方式:可以采用脚本获取
wget https://www.itl.nist.gov/iaui/vip/cs_links/EMNIST/gzip.zip
也可以直接从torchvision中开箱即用
from torchvision.datasets import EMNIST
from torchvision.transforms import Compose, ToTensor, Normalize
RAW_DATA_PATH = './rawdata'
transform = Compose(
[ToTensor(),
Normalize((0.1307,), (0.3081,))
]
)
train_dataset = EMNIST(
root=RAW_DATA_PATH,
split="byclass",
download=True,
train=True, #True加载训练集,False加载测试集
transform=transform
)
一定要注意参数 train
的设置,训练集和测试集要分开加载,直接加载出来的不是全部数据集!
该数据集可视化呈现结果如下:
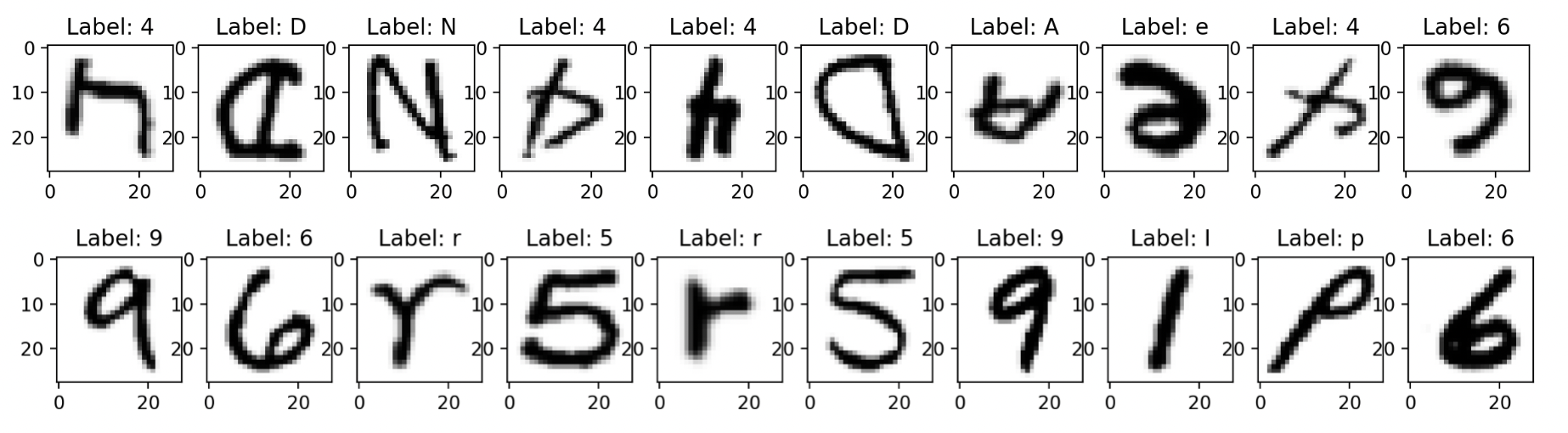
(3)FashionMINIST
任务:时尚物品图像识别
参数说明: 一共10种不同的时尚物品类别 (10种数字, 26种小写, 26种大写)的像素图片, 图片全为28乘28像素大小的像素(灰度)图, 样本数70000(训练集60000测试集10000),在联邦学习场景下需要自行设计划分算法进行划分。
介绍: FashionMNIST数据集取材于Zalando文章中的图片,是MINIST数据集的扩展版。
官网:https://github.com/zalandoresearch/fashion-mnist
引用方式:Xiao H, Rasul K, Vollgraf R. Fashion-mnist: a novel image dataset for benchmarking machine learning algorithms, 2017
获取方式:可以直接git clone 原始项目
git clone https://github.com/zalandoresearch/fashion-mnist.git
或从Kaggle上下载:https://www.kaggle.com/zalando-research/fashionmnist
也可以直接从torchvision中开箱即用
from torchvision.datasets import FashionMNIST
from torchvision.transforms import Compose, ToTensor, Normalize
RAW_DATA_PATH = './rawdata'
transform = Compose(
[ToTensor(),
Normalize((0.1307,), (0.3081,))
]
)
train_dataset = FashionMNIST(
root=RAW_DATA_PATH,
download=True,
train=True, #True加载训练集,False加载测试集
transform=transform
)
一定要注意参数 train
的设置,训练集和测试集要分开加载,直接加载出来的不是全部数据集!
该数据集可视化呈现结果如下:
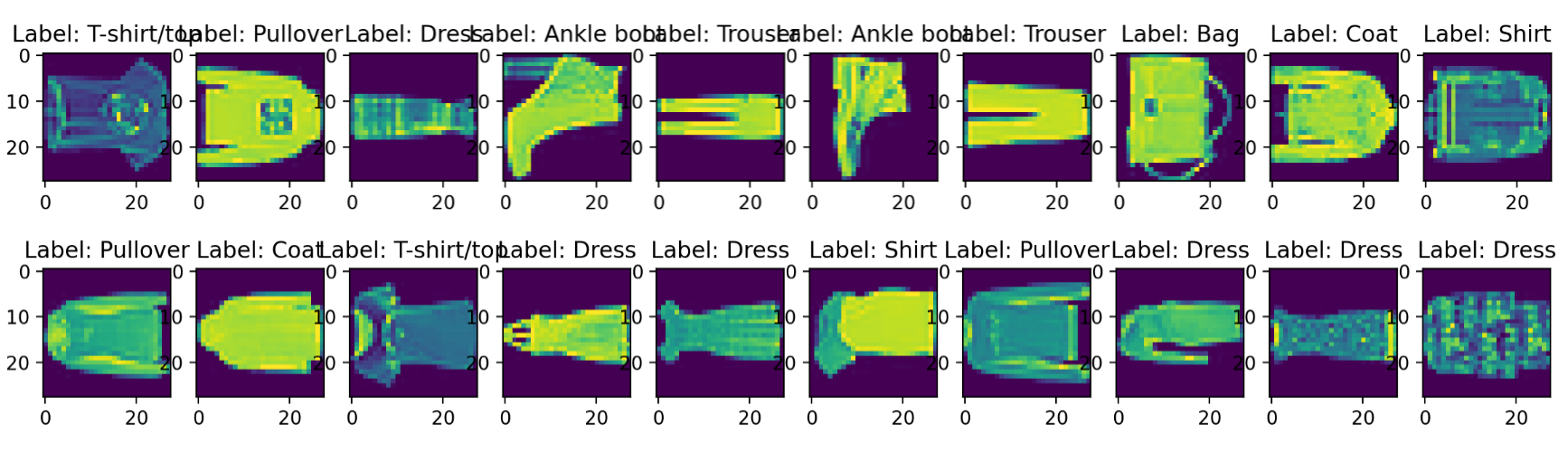
(4)CIFAR10
任务:图像分类
参数说明: 10种32x32的彩色图片(包括人、动物、花、昆虫等), 每种类别都有6000张图片. 50000张训练图片10000张测试图片,在联邦学习场景下需要自行设计划分算法进行划分。
介绍: CIFAR-10 是所谓的8千万张微型图片数据集的有标签子集。
官网:https://www.cs.toronto.edu/~kriz/cifar.html
引用方式:Alex Krizhevsky, Learning Multiple Layers of Features from Tiny Images, 2009.
获取方式:
直接从torchvision中开箱即用
from torchvision.datasets import CIFAR10
from torchvision.transforms import Compose, ToTensor, Normalize
RAW_DATA_PATH = './rawdata'
transform = Compose([
ToTensor(),
Normalize((0.4914, 0.4822, 0.4465), (0.2023, 0.1994, 0.2010))
])
dataset = CIFAR10(
root=RAW_DATA_PATH,
download=True,
train=True, #True加载训练集,False加载测试集
transform=transform
)
一定要注意参数 train
的设置,训练集和测试集要分开加载,直接加载出来的不是全部数据集!
该数据集可视化呈现结果如下:
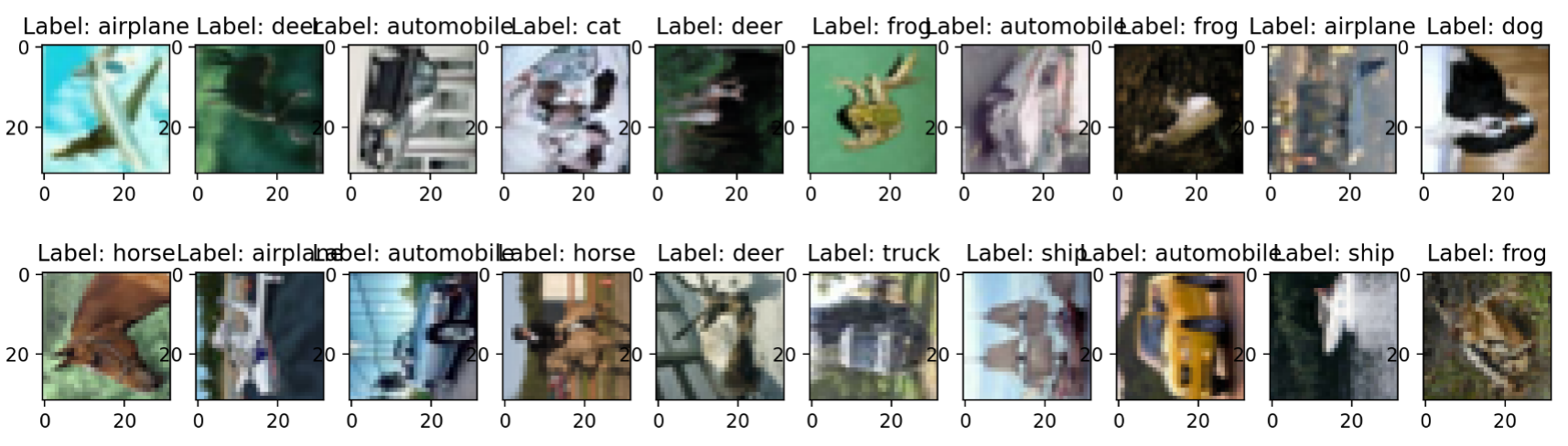
(5)CIFAR100
任务:图像分类
参数说明: 100种32x32的彩色图片(包括人、动物、花、昆虫等), 每种类别都有600张图片. 500张训练图片100张测试图片,在联邦学习场景下需要自行采用划分算法进行划分。
介绍: CIFAR-10的兄弟,也是所谓的8千万张微型图片数据集的有标签子集。
官网:https://www.cs.toronto.edu/~kriz/cifar.html
引用方式:Alex Krizhevsky, Learning Multiple Layers of Features from Tiny Images, 2009.
获取方式:
直接从torchvision中开箱即用
from torchvision.datasets import CIFAR100
from torchvision.transforms import Compose, ToTensor, Normalize
RAW_DATA_PATH = './rawdata'
transform = Compose([
ToTensor(),
Normalize((0.4914, 0.4822, 0.4465), (0.2023, 0.1994, 0.2010))
])
dataset = CIFAR100(
root=RAW_DATA_PATH,
download=True,
train=True, #True加载训练集,False加载测试集
transform=transform
)
一定要注意参数 train
的设置,训练集和测试集要分开加载,直接加载出来的不是全部数据集!
该数据集可视化呈现结果如下:
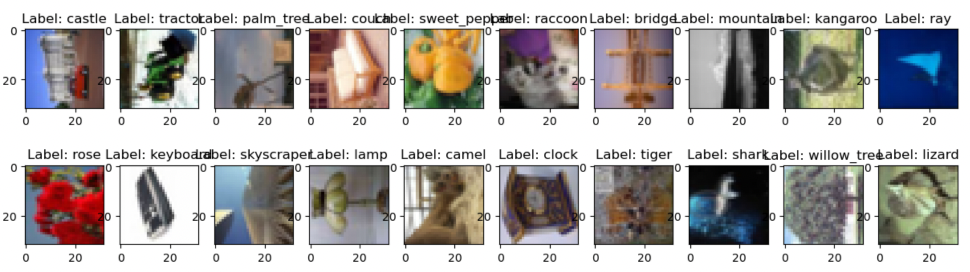
2. NLP数据集
(1)Shakespeare
任务:下一个字符预测
参数说明:总共4,226,15条样本,可使用官方给出的划分代码按照联邦学习场景下1129个client非独立同分布划分。
介绍: 和FEMNST一样,属于专门给联邦学习用的基准数据集leaf的成员之一。
官方数据预处理与划分代码:https://github.com/TalwalkarLab/leaf
引用方式:LEAF: A Benchmark for Federated Settings
获取方式:
用脚本获取
wget http://www.gutenberg.org/files/100/old/1994-01-100.zip
3.普通回归/分类
(1)Synthetic
任务:二分类
参数说明:用户能够自定义分布式节点数量, 类别数量以及维度
介绍: 这个数据集提供了一个生成人工的、但是有挑战性的联邦学习数据集方法, 我们要求的目标是分布式节点上的模型能够尽量有独立性。论文中详细地给出了数据集的生成过程。和FEMNST一样,属于专门给联邦学习用的基准数据集leaf的成员之一,在使用前可使用官方给出的代码按照非独立同分布进行划分(需要先指定client的数目)。
官方数据预处理与划分代码:https://github.com/TalwalkarLab/leaf
引用方式:LEAF: A Benchmark for Federated Settings
获取方式:
需按照下列python代码对数据集进行人工生成(详情见官方数据预处理与划分代码):
from scipy.special import softmax
NUM_DIM = 10
class SyntheticDataset:
def __init__(
self,
num_classes=2,
seed=931231,
num_dim=NUM_DIM,
prob_clusters=[0.5, 0.5]):
np.random.seed(seed)
self.num_classes = num_classes
self.num_dim = num_dim
self.num_clusters = len(prob_clusters)
self.prob_clusters = prob_clusters
self.side_info_dim = self.num_clusters
self.Q = np.random.normal(
loc=0.0, scale=1.0, size=(self.num_dim + 1, self.num_classes, self.side_info_dim))
self.Sigma = np.zeros((self.num_dim, self.num_dim))
for i in range(self.num_dim):
self.Sigma[i, i] = (i + 1)**(-1.2)
self.means = self._generate_clusters()
def get_task(self, num_samples):
cluster_idx = np.random.choice(
range(self.num_clusters), size=None, replace=True, p=self.prob_clusters)
new_task = self._generate_task(self.means[cluster_idx], cluster_idx, num_samples)
return new_task
def _generate_clusters(self):
means = []
for i in range(self.num_clusters):
loc = np.random.normal(loc=0, scale=1., size=None)
mu = np.random.normal(loc=loc, scale=1., size=self.side_info_dim)
means.append(mu)
return means
def _generate_x(self, num_samples):
B = np.random.normal(loc=0.0, scale=1.0, size=None)
loc = np.random.normal(loc=B, scale=1.0, size=self.num_dim)
samples = np.ones((num_samples, self.num_dim + 1))
samples[:, 1:] = np.random.multivariate_normal(
mean=loc, cov=self.Sigma, size=num_samples)
return samples
def _generate_y(self, x, cluster_mean):
model_info = np.random.normal(loc=cluster_mean, scale=0.1, size=cluster_mean.shape)
w = np.matmul(self.Q, model_info)
num_samples = x.shape[0]
prob = softmax(np.matmul(x, w) + np.random.normal(loc=0., scale=0.1, size=(num_samples, self.num_classes)), axis=1)
y = np.argmax(prob, axis=1)
return y, w, model_info
def _generate_task(self, cluster_mean, cluster_id, num_samples):
x = self._generate_x(num_samples)
y, w, model_info = self._generate_y(x, cluster_mean)
# now that we have y, we can remove the bias coeff
x = x[:, 1:]
return {'x': x, 'y': y, 'w': w, 'model_info': model_info, 'cluster': cluster_id}