Coursera, Machine Learning, SVM
Support Vector Machine (large margin classifiers )
1. cost function and hypothesis
下面那个紫色线就是SVM 的cost function


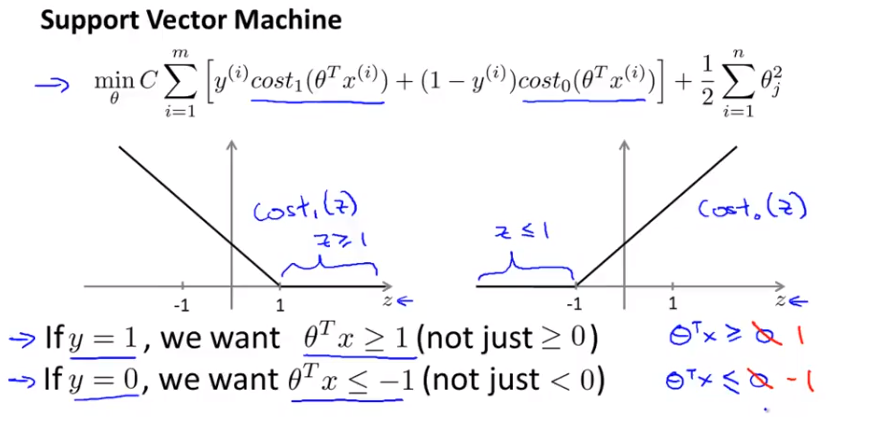
2. SVM 的数学解释


3. SVM with kernel
我的理解是 kernel 的作用就是把低维度的 x 转化成高维的 f, 然后就好分类了
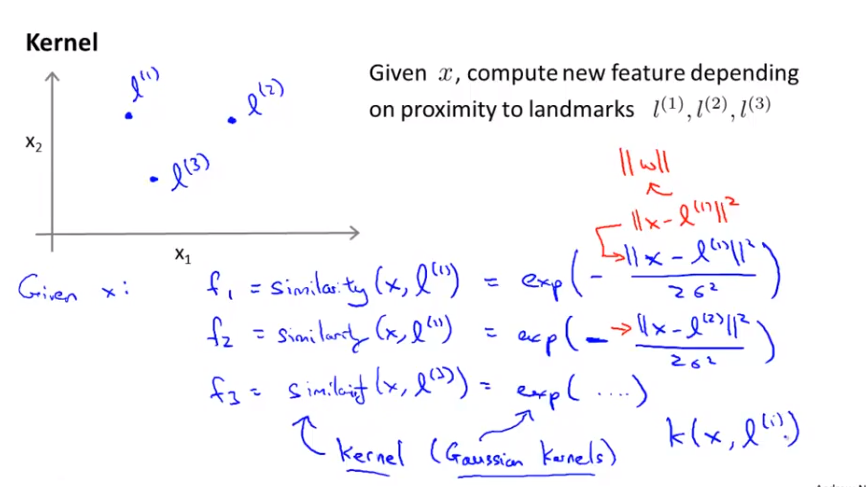
note: 上图就是一个2维(x1, x2)变3维(f1, f2, f3)的例子

4. SVM in practice

想一想,上面的结论也合理,因为SVM+kernel 会把n 个feature变成 m 个feature (m>n 以便放到更高维空间), 所以如果n>m 达不到低维到高维的变换,m 太大又会造成维度太高,最适合的情况是 m 略大于 n.大概相差一个数量级,如上图例子.
note: SVM without kernel 和liner regression 只能 linear 分类, 而SVM with kernel 可以做到non-linear 分类.
Ref:
转载请注明出处 http://www.cnblogs.com/mashuai-191/