Python数据处理
matplotlib
概念
-
matplotlib能将数据进行可视化,更加直观的呈现
-
使数据更客观,更有说服力
matplotlib是最流行的Python底层绘图库,主要做数据可视化图表,名字取材于matlab,模仿matlab创建
matplotlib官方文档
绘制折线图
from matplotlib import pyplot as plt
x = range(2, 26, 2) # 数据在X轴的位置,是一个可迭代对象
y = [15, 13, 14.5, 17, 20, 25, 26, 26, 24, 22, 18, 15] # 数据在Y轴的数据,是一个可迭代对象
# x轴和y轴的数据一起组成了所有要绘制出的坐标
plt.plot(x, y) # 传入x和y,通过plot绘图
plt.show() # 程序在执行的时候展示图形
设置图片大小
fig = plt.figure(figsize=(20, 8), dpi=80) # 宽20高8,
"""
figure图形图标的意思,在这里指的是我们画的图
通过实例化一个figure并且传递参数,能够在后台自动使用该figure实例
在图像模糊的时候可以传入dpi参数,让图片更加清晰
"""
保存图片
plt.savefig("./sig_size.png") # 保存图片,可以保存为svg格式的矢量图
定义坐标轴的疏密
# 设置x轴的刻度
plt.xticks(range(2, 25, 2)) # 步长为刻度,刻度为2(疏密)
# plt.xticks([i / 2 for i in range(4, 50)]) # 刻度为0.5,即可以使用等差列表来去调整刻度(疏密)
# 设置y轴刻度
plt.yticks(range(min(y), max(y)+1, 2)) # y轴范围为13到26
总代码
from matplotlib import pyplot as plt
fig = plt.figure(figsize=(20, 8), dpi=80) # 宽20高8,
"""
figure图形图标的意思,在这里指的是我们画的图
通过实例化一个figure并且传递参数,能够在后台自动使用该figure实例
在图像模糊的时候可以传入dpi参数,让图片更加清晰
"""
x = range(2, 26, 2) # 数据在X轴的位置,是一个可迭代对象
y = [15, 13, 14.5, 17, 20, 25, 26, 26, 24, 22, 18, 15] # 数据在Y轴的数据,是一个课迭代对象
# x轴和y轴的数据一起组成了所有要绘制出的坐标
plt.plot(x, y) # 传入x和y,通过plot绘图
# 设置x轴的刻度
plt.xticks(range(2, 25, 2)) # 步长为刻度,刻度为2(疏密)
# plt.xticks([i / 2 for i in range(4, 50)]) # 刻度为0.5,即可以使用等差列表来去调整刻度(疏密)
# 设置y轴刻度
plt.yticks(range(min(y), max(y)+1, 2)) # y轴范围为13到26
# plt.savefig("./sig_size.png") # 保存图片,可以保存为svg格式的矢量图
plt.show() # 程序在执行的时候展示图形
案例
import random
from matplotlib import pyplot as plt
"""如果列表a表示10点到12点的每一分钟的气温,如何绘制折线图观察每分钟的气温变化"""
a = [random.randint(20, 35) for i in range(120)]
fig = plt.figure(figsize=(20, 8), dpi=80) # 宽20高8
x = [i for i in range(1, 121)]
y = a
plt.xticks([2 * i for i in range(1, 61)])
plt.yticks([i for i in range(20, 36)])
plt.plot(x, y)
plt.show()
定义坐标轴的刻度
# 在10点时
x_label = [f"10h{i}m" for i in range(1, 61, 2)]
# 在11点时
x_label += [f"11h{i}m" for i in range(1, 61, 2)]
plt.yticks([i for i in range(20, 36)], [f"{i}f" for i in range(20, 36)]) # 后面的参数设置坐标轴显示的样式
plt.xticks([2 * i for i in range(1, 61)], x_label) # 一一对应
设置字体显示中文
为什么无法显示中文
- matplotlib默认不显示中文字符,因为默认的英文字体无法显示汉字
fc-list :lang=zh
- Mac和linux里面查看支持的中文
# windows和linux设置字体的方式
import matplotlib
font = {'family': 'MicroSoft YaHei',
'weight': 'bold',
'size': 12}
matplotlib.rc("font", **font)
from matplotlib import font_manager
my_font = font_manager.FontProperties(fnanme="字体路径") # 后面使用时输入fontproperties=my_font
# 如:plt.ylabel("温度 单位(摄氏度)", fontproperties=my_font)
添加描述信息
# 设置描述信息
plt.xlabel("时间")
plt.ylabel("温度 单位(摄氏度)")
plt.title("10点到12点没分钟内的气温变换情况")
改进后
import random
from matplotlib import pyplot as plt
"""如果列表a表示10点到12点的每一分钟的气温,如何绘制折线图观察每分钟的气温变化"""
a = [random.randint(20, 35) for i in range(120)]
fig = plt.figure(figsize=(20, 8), dpi=80) # 宽20高8
x = [i for i in range(0, 120)]
y = a
# 在10点时
x_label = [f"10h{i}m" for i in range(1, 60)]
# 在11点时
x_label += [f"11h{i}m" for i in range(1, 60)]
import matplotlib
font = {'family': 'MicroSoft YaHei',
'weight': 'bold',
'size': 12}
matplotlib.rc("font", **font)
# 设置描述信息
plt.xlabel("时间")
plt.ylabel("温度 单位(摄氏度)")
plt.title("10点到12点每分钟内的气温变换情况")
plt.yticks([i for i in range(20, 36)], [f"{i}度" for i in range(20, 36)]) # 后面的参数设置坐标轴显示的样式
plt.xticks(x[::3], x_label[::3], rotation=90) # 要保证前后的一一对应,rotation旋转度数
plt.plot(x, y)
plt.show()
绘制网格线
# 绘制网格
plt.grid(alpha=0.4) # alpha设置透明度
案例
# 表示出从一个月11号到最后一天30号内每天做的题数如列表a,请绘制折线图,以便分析每天做题的走势
a = [1, 0, 1, 1, 2, 4, 3, 2, 3, 4, 4, 5, 6, 5, 4, 3, 3, 1, 1, 1]
# 要求:y轴表示个数、x轴表示日期
from matplotlib import pyplot as plt
import matplotlib
# 自己
y = a
x = [i for i in range(11, 31)]
plt.figure(figsize=(20, 8), dpi=80)
# 但是,半路杀出了一个同桌,请设计一个折线图,表示两者做题数量
y_c = [1, 0, 3, 1, 2, 2, 3, 3, 2, 1, 2, 1, 1, 1, 1, 1, 1, 1, 1, 1]
# 设置轴参数
plt.yticks([i for i in range(min(a), max(a)+1)], [f"{i}人" for i in range(min(a), max(a)+1)])
plt.xticks(x[::4], [f"{i}号" for i in range(11, 31, 4)], rotation=90)
plt.xlabel("日期(号)")
plt.ylabel("数量(题)")
plt.title("每天的做题数量情况")
# 绘制网格
plt.grid(alpha=0.4) # 设置透明度
# 设置字体
font = {'family': 'MicroSoft YaHei',
'weight': 'bold',
'size': 12}
matplotlib.rc("font", **font)
# 自己
plt.plot(x, y, label="自己", color="red") # color添加颜色
# 同桌
plt.plot(x, y_c, label="同桌", color="blue", linestyle='--') # linestyle设置线条样式
# 添加图例
plt.legend(loc="upper left") # loc设置位置
plt.show()
绘制散点图
from matplotlib import pyplot as plt # 其他元素可以直接使用折线图来表示
# 使用scatter绘制散点图和绘制折线图的代码区别
plt.scatter(x, y)
案例
from matplotlib import pyplot as plt
import matplotlib, random
font = {'family': 'MicroSoft YaHei',
'weight': 'bold',
'size': 12}
matplotlib.rc("font", **font)
plt.figure(figsize=(20, 8), dpi=80)
y_1 = [random.randint(1, 1000) for i in range(1, 31)] # 定义y轴
y_2 = [random.randint(1, 100) for j in range(1, 31)]
x = range(1, 31) # 定义x轴
plt.scatter(x, y_2, label="自己") # 注意x和y的值要一一对应
plt.scatter(x, y_1, label="同学")
plt.xlabel("日期(号)")
plt.ylabel("数量(题)")
plt.title("每天的做题数量情况")
plt.legend() # 设置图例
plt.show()
绘制条形图
from matplotlib import pyplot as plt
plt.bar(x, y) # 使用bar绘制条形图
plt.barh(x, y) # 绘制横方向的条形图图
案例
from matplotlib import pyplot as plt
import matplotlib, random
font = {'family': 'MicroSoft YaHei',
'weight': 'bold',
'size': 12}
matplotlib.rc("font", **font)
plt.figure(figsize=(20, 8), dpi=80)
y_1 = [random.randint(1, 1000) for i in range(1, 31)]
y_2 = [random.randint(1, 100) for j in range(1, 31)]
x_1 = range(1, 31)
x_2 = [i+0.3 for i in range(1, 31)]
plt.xticks(list(x), [f"{i}日" for i in range(1, 31)])
plt.bar(x_2, y_2, label="自己", width=0.3) # 竖状图,宽度可以传入列表
plt.bar(x_1, y_1, label="同学", width=0.3) # 利用数据的偏移使其呈现出对比度,即两列图标
# plt.barh(x, y_1, label="同学", height=0.3) # 使用height
plt.ylabel("日期(号)")
plt.xlabel("数量(题)")
plt.title("每天的做题数量情况")
plt.legend() # 设置图例
plt.show()
绘制直方图
from matplotlib import pyplot as plt
plt.hist(x, bins="组距")
案例
from matplotlib import pyplot as plt
import matplotlib, random
font = {'family': 'MicroSoft YaHei',
'weight': 'bold',
'size': 12}
matplotlib.rc("font", **font)
plt.figure(figsize=(20, 8), dpi=80)
"""有250条数据,其为100分钟到120分钟电影的数量"""
a = [random.randint(100, 121) for i in range(250)] # 模拟250条数据
grou_len = 3
bar_group = int((max(a) - min(a)) / 3) # 表示生成的直方图的组数,数据在100个以内时,按数据最多分为2--12组
# 组距:指每个小组的两个端点的距离,组数 = 极差 / 组距
plt.hist(a, bins=bar_group)
# 设置刻度
plt.xticks(range(min(a), max(a)+1, grou_len)) # 1为组距
plt.show()
绘制饼图
from matplotlib import pyplot as plt
plt.pie(x) # 其余参数大部分与折线图直方图相同
案例
import matplotlib.pyplot as plt
import numpy as np
y = np.array([35, 25, 25, 15])
plt.pie(y,
labels=['A','B','C','D'], # 设置饼图标签
colors=["#d5695d", "#5d8ca8", "#65a479", "#a564c9"], # 设置饼图颜色
)
plt.title("RUNOOB Pie Test") # 设置标题
plt.show()
# -----------------------------------------------------------------------------------------------------------------
import matplotlib.pyplot as plt
import numpy as np
y = np.array([35, 25, 25, 15])
plt.pie(y,
labels=['A','B','C','D'], # 设置饼图标签
colors=["#d5695d", "#5d8ca8", "#65a479", "#a564c9"], # 设置饼图颜色
explode=(0, 0.2, 0, 0), # 第二部分突出显示,值越大,距离中心越远
autopct='%.2f%%', # 格式化输出百分比
)
plt.title("RUNOOB Pie Test")
plt.show()
numpy
一个在Python中做科学计算的基础库,重在数值运算,也是大部分Python科学计算库的基础库,多用于在大型、多维数值上执行数值运算
numpy官方文档
数组基础
创建
import numpy as np
import random
a = np.array([1, 2, 3, 4, 5])
n = np.array(range(1, 6))
c = np.arange(1, 6)
# 上面三种方法内容相同,注意range和arange的区别
print(a)
# 数组的类名
print(type(a))
print(a.dtype) # 查看数据里面存放的数据类型
t = np.array(range(12), dtype="int64").reshape(3, 4)
data = np.zeros((t.shape[0], 1)).astype(int) # 构造与t1数组行数相同,一列全为0的数据
print(data)
data = np.ones((t.shape[0], 1)).astype(int) # 构造与t数组行数相同,一列全为1的数据
print(data)
# numpy里面的bool类型
d = np.array([1111, 0, 0, 1, 11, 1], dtype=bool)
print(d)
# 改变d的数据类型
d = d.astype("int8")
print(d)
f = np.array([random.random() for i in range(10)]) # 生成10个随机小数
print(f)
f = np.round(f, 2) # 保留两位小数
print(f)
形状
a = np.array([[i for i in range(10)], [i for i in range(10)]]) # 使用多维列表创建
print(a)
print(a.shape) # 查看数组的形状(矩阵) 二维数组
a = np.array([[[i for i in range(10)], [i for i in range(10)], [i for i in range(10)]], [[i for i in range(10)], [i for i in range(10)], [i for i in range(10)]]])
print(a)
print(a.shape) # 查看数组的形状(矩阵) 三维数组
t1 = np.arange(12)
t1 = t1.reshape((3, 4)) # 转换成三行四列的二维数组
print("t1:", t1)
t2 = np.arange(48)
t2 = t2.reshape((4, 3, 4) # 转换成四个三行四列的三维数组
print("t2:", t2)
降维
t2 = np.arange(24)
t2 = t2.reshape(2, 3, 4) # 转换成二个三行四列的三维数组
# 降维
t3 = t2.reshape((6, 4)) # 不会对原来的t2数组做任何改变
print("t3:", t3)
print("t2:", t2)
t4 = t3.reshape((24, )) # 变成一维数组,并且赋值给t4
print("t4:", t4)
取值
t2 = np.arange(24)
t2 = t2.reshape(2, 3, 4) # 转换成二个三行四列的三维数组
print("t2", t2)
print(t2[0][0][0]) # 取值顺序为 数组名 [ 第几个] [ 第几行 ] [ 第几列 ]
# 直接把t2降维成一维数组
t3 = t2.reshape((t2.shape[0] * t2.shape[1] * t2.shape[2], ))
for i in range(2): # 使用shape查看数组形状
print(f"{i}:", t2.shape[i])
print("降维后:", t3)
# 展开数组
print(t2.flatten())
使用
数组的广播机制
-
数组与数字相运算
a = np.arange(100, 124).reshape(2, 3, 4) print("before add:\n", a) a += 1 # 加减乘除都一样,都是所有数字都同时进行运算 print("after add int:\n", a)
-
数组与数组相运算
如果两个数组的后缘维度,即从末尾开始算起的维度的轴长度相符,或者其中一方的长度为1,则认为它们时广播兼容的。广播在缺失【或 / 和】长度为1的维度上进行,遵循矩阵运算法则
如:shape为(3, 3, 3)的数组不能和(3, 2)的数组进行运算
shape为(3, 3, 3)的数组可以和(3, 2)的数组进行运算,不能和(3, 3)进行运算
a_ad = np.arange(24).reshape(2, 3, 4) # 当两数组的形状一样时,其对应位置数字相加 print("after ride array:\n", a+a_ad) # 当两个数组维度不一样时,如果列数相同,同一列的对应位置数字相运算;如果行数相同,同一行的对应位置相互运算
轴
在numpy中可以理解为方向,使用0,1,2···表示,对于一维数组,只有一个0轴;对于二维数组shape(2, 2),有0轴和1轴;对于三维数组(2, 2, 2),有0轴,1轴和2轴
# 一维
z = np.arange(4)
s0 = np.sum(z, axis=0) # 取0轴
print("array:\n", z)
print("axis=0\n", s0)
"""
个人理解
对于一维数组,0轴即为横向
"""
# 二维
z = np.arange(12).reshape((3, 4))
s0 = np.sum(z, axis=0) # 取0轴
s1 = np.sum(z, axis=1) # 取1轴
print("array:\n", z)
print("axis=0:\n", s0)
print("axis=1:\n", s1)
"""
个人理解
对于二维数组,0轴为纵向,1轴为横向
亦可以说,shape((3, 4)) 各部分的和 对应 (1轴, 0轴)
"""
# 三维
z = np.arange(24).reshape((2, 3, 4))
s0 = np.sum(z, axis=0) # 取0轴
s1 = np.sum(z, axis=1) # 取1轴
s2 = np.sum(z, axis=2) # 取2轴
print("array:\n", z)
print("axis=0:\n", s0)
print("axis=1:\n", s1)
print("axis=2:\n", s2)
"""
个人理解
对于三维数组,可以把三维数组假想为一个三维空间,每一块二维数组正对我们排列;同时垂直二维数组空间的轴为0轴,1轴为二维数组所构成空间的纵轴,2轴为二位数组所构成空间的横轴
亦可以说,shape((2, 3, 4)) 各部分的和 对应 (0轴, 1轴, 2轴)
"""
# 还有一种跨行跨列的理解方法
文件
读取数据
csv文件:逗号分隔文件
- 显示:表格状态
- 源文件:换行和逗号分隔行列的格式化文本,每一行的数据表示一条记录
由于csv便于展示,读取和写入,所以很多地方也是用csv的格式存储和传输小型数据
np.loadtxt(frame="文件路径", dtype="返回数据类型", delimit="用什么符号分隔数据", skiprows="跳过第几行,默认为0", usecols="读取指定列,索引,元组类型", unpack=Flase) # 如果unpack为True,读入属性将分别写入不同数组变量,False读入数据只写入一个数组变量,默认False,矩阵的转置效果——按照对角线旋转180°
数组的转置
转置是一种变换,对于numpy中的数组来说,就是在对角线方向交换数据,目的也是为了更方便的去处理数据
t = np.arange(24).reshape((4, 6))
print("转置前:\n", t, "\n--------------------------\n转置后:")
print(t.transpose())
print(t.swapaxes(1, 0)) # 交换轴
print(t.T)
数组高阶
索引和切片
像列表的操作一样,左闭右开
语法:[几个,几行,几列]
每个类型可以使用列表的切片
t2 = np.arange(32)
t2 = t2.reshape(2, 4, 4) # 转换成二个三行四列的三维数组
print("t2", t2)
print("-"*30)
print(t2[0, 0:2])
print(t2[0, 0:2, 0]) # 取得第一块,第一到二行的第一列数据
print(取得不连续的行数据)
print(t2[0, 0:4:2])
t[:,2:4] # 表示取得第二到第三列的值
print(t2[0, 0:4])
print(取得不连续的列)
print(t2[0, 0:4, 0:4:3])
语法:[[存放第几块], [存放第几行], [存放第几列]]
每个类型可以使用列表的切片
t2 = np.arange(24)
t2 = t2.reshape(2, 3, 4) # 转换成二个三行四列的三维数组
print("t2", t2)
print(t2[[0], [0], [0]]) # 取值顺序为 数组名 [[ 第几个] [ 第几行 ] [ 第几列 ]]
print("-"*30)
print(t2[[0], [0: 3]]) # 取第一个,0到三行的数据
print(t2[[0], [0: 3], [0]])
# 取出几个不连续的点
print(t2[[1, 1, 0], [0, 2, 1], [0, 0, 0]])
# 得到三个不连续的点第一个列表代表第几块,第二个列表代表低级含,第三个列表代表第几列,点按照列表的下标一一对应
可以自己尝试使用。类似于列表的切片
修改和布尔索引
使用切片判断
t2 = np.arange(24)
t2 = t2.reshape(2, 3, 4) # 转换成二个三行四列的三维数组
print(t2<10)
print(t2[t2<10]) # 为布尔索引
t2[t2<10] = 0 # 把t2里面所有小于10的数替换成0,赋值操作,把显示为True的赋值为0
print(t2)
t2 = np.arange(24)
t2 = t2.reshape(2, 3, 4) # 转换成二个三行四列的三维数组
# where方法
a = np.where(t2<10, 0, 10) # numpy中的三元运算符,如果t2小于10则换成0,大于10换成10
print(a) # 不会替换数组的值
# clip方法
c = t2.clip(10, 18) # 小于10的替换为10,大于18的替换为18
print(c)
nan和inf
nan:not a number 表示不是一个数字
-
当我们读取本地的文件为float的时候,如果有缺失,就会出现nan
-
当做了一个不合适的计算的时候(比如:无穷大减去无穷大)
inf:infinitly inf表示正无穷,-inf表示负无穷
- 一个数字除以0
那么,如何避免呢
print(np.nan == np.nan) # 可以发现不相等
print(type(np.nan), type(np.inf))
利用两个nan是不相等的,可以判断数组中的nan的个数
t = np.array([1, 2, np.nan])
print(t != t)
print(np.isnan(t))
print(np.count_nonzero(t != t)) # 判断nan的个数
print(np.count_nonzero(np.isnan(t)))
# 赋值为0
np.isnan(t) = 0 # 但是在实际中不能够使用
# 为什么要判断呢?
print(np.sum(t)) # 如果有nan,会返回nan;任何值与nan运算,都能返回nan
把全部nan替换成0后,替换之前的平均值如果大于0,替换之后均值肯定减小,所以更一般的方式是把缺失的数值替换为均值(中值)或是直接删除有缺失值的一行
统计函数
求和:np.sum(t, axis=None)
均值:t.mean(a, axis=None)
受高离群点的影响较大
中值:np.median(t, axis=None)
最大值:t.max(axis=None)
最小值:t.min(axis=None)
极值:np.ptp(t, axis=None)
即最大值和最小值的差值
标准差:t.std(axis=None)
- 标准差是一组数据平均值分散程度的一种度量。一个较大的标准差,代表大部分数值和其平均值之间的差异较大;一个较小的标准差,代表这些数值较接近平均值,反映出数据的波动稳定情况,越大代表越不稳定。
默认返回多维数组的全部的统计结果,如果指定axis,则返回一个当前轴上的结果
去除nan
import numpy as np
def change_nan(t1):
for i in range(t1.shape[1]): # 遍历每一列
temp = t1[:,i]
if np.count_nonzero(temp != temp): # 不为0,则说明有nan
temp_not = temp[temp == temp] # 当前一列不为nan的arry。temp == temp用来判断是否有nan,如果有nan,就不传入
temp[np.isnan(temp)] = temp_not.mean() # 将均值赋值给nan
return t1
if __name__ == "__main__":
t1 = np.arange(12).reshape(3, 4).astype("float")
t1[1, 2:] = np.nan
print(change_nan(t1))
数组的拼接
竖直拼接:np.vstack((t1, t2))
水平拼接:np.hstack((t1, t2))
数据的行列交换
t[[2, 1], :] = t[[1, 2], :] # 第一行的数据和第二行数据进行交换
t1[:, [0,2]] = t1[:, [2,0]] # 第零列和第一列进行交换
"""注意和切片不一样,切片内部列表是[:]"""
其他方法
创建一个全为0的数组:np.zeros((3, 4))
三行四列
创建一个全为1的数组:np.ones((3, 4))
三行四列
创建一个个对角线为一的正方形数组(方阵):np.eye(3)
获取最值的位置:np.argmax(t, axis=0)
最大值 np.argmin(t, axis=0)
最小值
生成随机数:np.random.
方法 | 作用 | 参数说明 |
---|---|---|
randn(d0, d1, …, dn) | 产生标准正态分布随机数 | dn为第n维数据的维度 |
randint(low[, high, size, dtype]) | 产生随机整数 | low:最小值;high:最大值;size:数据个数 |
random_sample([size]) | 在[0,1)内产生随机数 | size:随机数的shape,可以为元祖或者列表,[2,3]表示2维随机数,维度为(2,3) |
random([size]) | 同random_sample([size]) | 同random_sample([size]) |
ranf([size]) | 同random_sample([size]) | 同random_sample([size]) |
sample([size])) | 同random_sample([size]) | 同random_sample([size]) |
choice(a[, size, replace, p]) | 从a中随机选择指定数据 | a:1维数组 size:返回数据形状 |
bytes(length) | 返回随机位 | length:位的长度 |
rand(d0, d1, …, dn) | 产生均匀分布的随机数 | dn为第n维数据的维度 |
seed([seed]) | 定义全局种子 | 参数为整数或者矩阵 |
a = b :完全不复制
a = b.copy() :深拷贝
pandas
numpy能够帮助我们处理数据,但是pandas除了处理数值之外(基于numpy),还能够帮助我们处理其他类型的数据
pandas官方文档
常用数据类型
-
series 一维,带标签的一维数组
-
dataframe 二维,series容器
series
创建
import pandas as pd
a = pd.Series([range(10)])
# a = pd.Series([range(10)], index=[range(1, 11)]) # index指定索引值,数量要一致
print(a)
print(type(a))
# 字典创建
temp = {"name": "zansan", "age": 30, "tel": 10086}
ser = pd.Series(temp)
print(ser.dtype) # 查看数据类型
# ser.astype(int) # 如果值全部是数字可以转换
# print(ser.dtype)
print(ser)
print(type(ser))
切片和索引
import pandas as pd
temp = {"name": "zansan", "age": 30, "tel": 10086}
ser = pd.Series(temp)
print(ser[[0, 2]]) # 取得第1条和第3条数据,注意是两个列表
print(ser[0:2:1]) # 切片,直接传入start,end,步长
print(ser[["name", "age"]]) # 通过索引取值
# 索引,传入序号或者index,多个的时候传入序号或者index的列表
# 布尔索引
print(t[t>10])
for i in ser.index: # 遍历索引
print(i, type(i))
print(len(ser.index)) # 获取长度
print(list(ser.index)) # 得到索引字典
print(ser.values) # 得到值
print(type(ser.values))
series对象本身由两个数组构成,一个数组构成对象的键(index,索引),一个数组构成对象的值(value),键 -> 值
numpy的很多方法都可以运用于series类型,比如:argmax, clip等,可以通过官方文当来查询
读取数据
读取csv文件:pd.read_csv("file_path")
import pandas as pd
from pymongo import MongoClient
client = MongoClient()
# 读取csv文件
f = pd.read_csv(r"D:\programme\Python\Python学习\数据分析\day03\code\youtube_video_data\US_video_data_numbers.csv")
# 从数据库中读取文件
col = client["python"]["book"]
data = list(col.find())
a = pd.Series(data)
print(a)
DataFrame
创建
import pandas as pd
dataframe = pd.DataFrame(np.arange(12).reshape(3, 4))
print(dataframe) # 有行索引也有列索引
import pandas as pd
temp = {"name": ["zansan", "lihua"], "age": [30, 20], "tel": [10086, 10096]} # 第一种,字典传入
dataframe = pd.DataFrame(temp, index=list("ab"))
print(dataframe)
import pandas as pd
temp = [{"name": "lihua", "age": 12}, {"name": "zanshan", "age":2}, {"name":"lisi"}] # 第二种
dataframe = pd.DataFrame(temp, index=list("abc"))
print(dataframe)
DataFrame对象既有行索引,又有列索引
-
行索引,表明不同行,横向索引,叫index,0轴,axis=0
-
列索引,表明不同列,纵向索引,叫columns,1轴,axis=1
import pandas as pd dataframe = pd.DataFrame(np.arange(12).reshape(3, 4), index=list("abc"), columns=list("wxyz")) print(dataframe)
连接mongo读取数据
import pandas as pd
from pymongo import MongoClient
client = MongoClient()
# 从数据库中读取文件
col = client["python"]["book"]
data = list(col.find())
a = pd.DataFrame(data)
print(a)
处理数据
import pandas as pd
from pymongo import MongoClient
client = MongoClient()
# 从数据库中读取文件
col = client["python"]["book"]
data = list(col.find())
data_list = [] # 存放想要的数据
for i in data:
data_list.append({"book_name": i["bookName"], "time": i["time"]})
a = pd.DataFrame(data_list)
print(a)
基本用法
行数:dataframe.shape
列数据类型:dataframe.dtypes
数据维度:df.ndim
行索引:df.index
列索引:df.columns
对象值:df.values
(二维的数组)
显示头部几行:df.head(3)
(默认五行)
显示尾部几行:df.tail(3)
(默认五行)
相关信息概览:df.info()
(列类型等)
快速综合统计结果:df.describe()
(平均值,中位数等)
取行取列
print(df[:20]) # 获取前20行
print(df[:20]["book_name"]) # 同时取行和取列
print(df["book_name"]) # 取列,series类型
loc
df.loc
通过标签索引行数据
df.iloc
通过位置获取行数据
import pandas as pd
df = pd.DataFrame(np.arange(12).reshape(3, 4), index=list("abc"), columns=list("wxyz"))
print(df)
rs = df.loc["a", "z"] # 取特定值,标签取值
print(rs, type(rs))
print(df.loc["a", :]) # 取某一行
print(df.loc[:, "z"]) # 取某一列
print(df.loc[["a", "c"],:]) # 取多行
print(df.loc[:,["x", "z"]]) # 取多列
"""
iloc的用法类似,其是根据索引取值,而不是标签
同时还可以进行赋值操作
"""
布尔索引
print(df[(df["w"]>=0) & (df["w"]<8)]) # &逻辑判断符‘与’,连接,不同条件之间要使用括号括起来
字符串索引
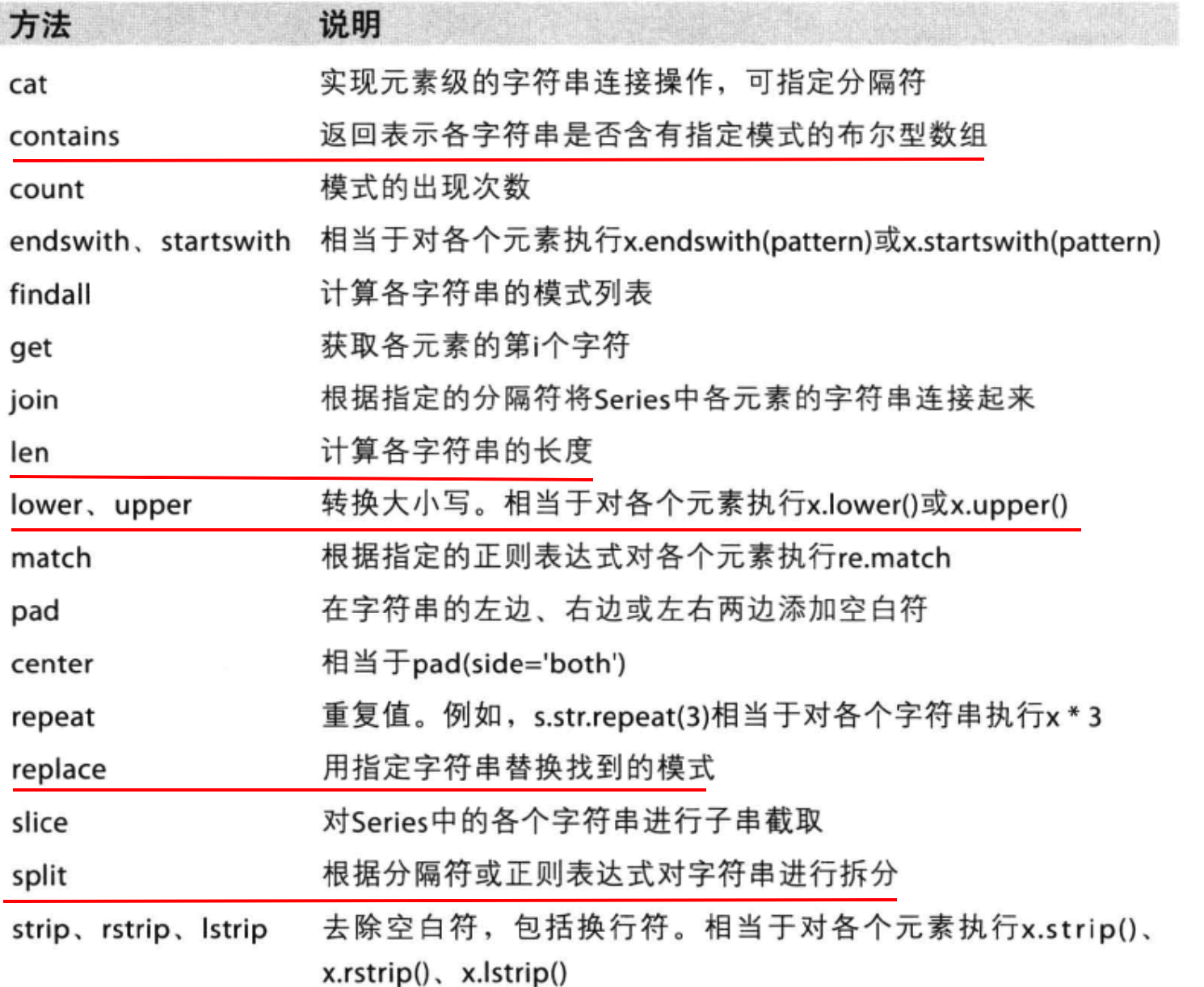
print(df['book_name'].str.split("《").tolist())
数据处理
缺失数据处理
判断数据是否为NaN:pd.isnul(df) / pd.notnull(df)
NaN
处理方式
-
删除NaN所在的行列
dropna(axis=0, how="any", inplace=False)
t3[pd.notnull(ts["w"])] # 取t3第w列,没有nan的行,数组的布尔索引:为True则选择;反之则抛弃 t3.dropna(axis=0, how="any") # 删除行,how—— = any时,只要有一个nan就删除
-
填充数据
t.fillna()
t3 = t3.fillna(t3.mean()) # 填充均值括号内部还可以为其他任意数字 t3["age"] = t3["age"].fillna(t3["age"].mean()) # 填充一列数据
如果计算均值的那一列有nan,pandas不会把那个数字计算在里面
案例一
import pandas as pd
from matplotlib import pyplot as plt
df = pd.read_csv(r"D:\programme\Python\Python学习\数据分析\day05\code\IMDB-Movie-Data.csv") # 直接时DataFrame框架
# runtime的分布情况
# 选择图型,直方图
# 准备数据
runtime_data = df["Runtime (Minutes)"].values # 取出runtime
max_runtime = runtime_data.max()
min_runtime = runtime_data.min()
# 计算组数
num_bin = (max_runtime - min_runtime) // 5
plt.figure(figsize=(20, 8), dpi=80)
plt.hist(runtime_data, bins=num_bin)
plt.xticks(range(min_runtime, max_runtime+5, 5)) # 调整组距
plt.show()
案例二
import pandas as pd
import numpy as np
from concurrent.futures import ThreadPoolExecutor
from matplotlib import pyplot as plt
# 设置字体
import matplotlib
df = pd.read_csv(r"D:\programme\Python\Python学习\数据分析\day05\code\IMDB-Movie-Data.csv") # 直接是DataFrame框架
# runtime的分布情况
# 选择图型,直方图
# 准备数据
temp_data = df["Genre"].str.split(",").tolist() # 取出runtime
genre = list(set([i for j in temp_data for i in j]))
# 构造全部为0的数组
zero = pd.DataFrame(np.zeros((df.shape[0], len(genre))), columns=genre)
# 给每个电影出现分类的位置赋值为一
for i in range(df.shape[0]):
zero.loc[i, temp_data[i]] = 1 # 出现名字就赋值为一
def draw(genre, zero):
font = {'family': 'MicroSoft YaHei',
'weight': 'bold',
'size': 12}
matplotlib.rc("font", **font)
# 准备画图
plt.figure(figsize=(20, 8), dpi=80)
for i in range(len(genre)):
# 得到数量统计
arr = np.array([0, 0]) # 第一个是含有这部分的数量
for j in list(zero[genre[i]]):
if j == 1.0:
arr[0] += 1
else:
arr[1] += 1
# 绘制饼图
plt.pie(arr, labels=["有该类型", "无该类型"])
print(genre[int(i)])
print(i)
print(int(i))
plt.title(f"电影类型{genre[int(i)]}")
plt.savefig(f"./{i}类型.svg")
# plt.show()
# 画条形图
genre_count = zero.sum(axis=0) # 竖向求和
y = genre_count.values
x = genre_count.index
plt.bar(x, y)
plt.savefig("./bar.svg")
if __name__ == '__main__':
with ThreadPoolExecutor(50) as pool: # 使用线程,加快分析速度
pool.submit(draw, args=(genre, zero))
常用统计方法
# 构造全部为0的数组
zero = pd.DataFrame(np.zeros((df.shape[0], len(genre))), columns=genre)
# 给每个电影出现分类的位置赋值为一,便于统计数量
for i in range(df.shape[0]):
zero.loc[i, temp_data[i]] = 1 # 出现名字就赋值为一
数据合并
join
默认情况下,它是把行索引相同的数据合并到一起
import pandas as pd
import numpy as np
pf1 = pd.DataFrame(np.ones((2, 4)), index=["A", "B"], columns=list("abcd"))
print(pf1)
pf2 = pd.DataFrame(np.zeros((3, 3)), index=["A", "B", "C"], columns=list("xyz"))
print(pf2)
print(pf1.join(pf2), "\n", pf2.join(pf1)) # 哪个在前面就以哪个为主要
merge
按照指定的列把数据按照一定方式合并到一起,与数据库mysql的合并方式类似
pf3 = pd.DataFrame(np.arange(9).reshape((3, 3)), columns=list("fax"))
# 内链接
print("-----------------------------")
print(pf1)
print("-----------------------------")
print(pf3)
print(pf1.merge(pf3, on="a", how="inner")) # 内链接,取交集
print("-----------------------------")
pf3.loc[1, "a"] = 1
print(pf3)
print(pf1.merge(pf3, on="a", how="inner")) # 取交集
# 外链接
print("-----------------------------")
print(pf1)
print("-----------------------------")
print(pf3)
print(pf1.merge(pf3, on="a", how="outer")) # 外链接,取并集
print("-----------------------------")
pf3.loc[1, "a"] = 1
print(pf3)
print(pf1.merge(pf3, on="a", how="outer")) # 取并集
# 左链接
print(pf1.merge(pf3, on="a", how="left")) # 左边为准,NaN补全,即以pf1为准
# 右链接
print(pf1.merge(pf3, on="a", how="right")) # 右边为准,NaN补全,即以pf3为准
分组聚合
grouped = groupby(by="columns name")
grouped是一个DataFrameGroupBy对象,是可迭代的
grouped中的每一个元素是一个元组
元组里面是(索引(分组的值), 分组之后的DataFrame)
DataFrameGroupBy对象有很多经过优化的方法
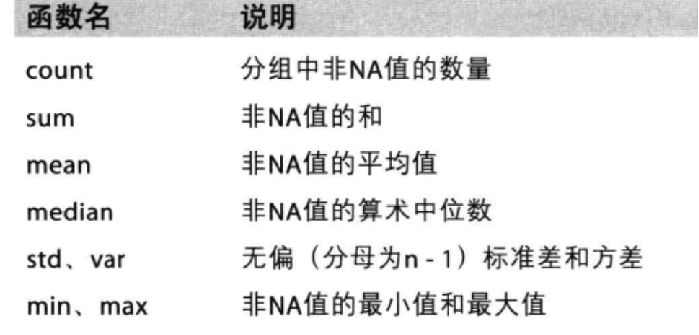
df = pd.read_csv("D:\programme\Python\Python学习\数据分析\day05\code\starbucks_store_worldwide.csv")
# print(df.info())
group = df.groupby(by="Country")
# print(group) # 可以进行遍历,调用聚合方法
# for i, j in group:
# print(i)
# print("----------------"*30)
# print(j)
# print("*"*60)
# 使用聚合方法,比较
country_count = group["Brand"].count()
print(country_count["US"])
print(country_count["CN"])
# 统计每个省
cn = df[df["Country"] == "CN"]
# print(cn)
group = cn.groupby(by="State/Province").count()["Brand"]
print(group)
# 数据按照多个条件进行分组如果为df[["Store Name"]],返回类型为DataFrame
group = df["Store Name"].groupby(by=[df["Country"], df["State/Province"]])
# for i, j in group:
# print(i)
# print("----------------"*30)
# print(j)
# print("*"*60)
print(group.count()) # series类型,两个索引
索引的方法和属性
简单的索引操作:
-
获取index:
df.index
-
指定index :
df.index = ['x','y']
-
重新设置index :
df.reindex(list("abcedf"))
-
指定某一列作为index :
df.set_index("Country",drop=False)
-
返回index的唯一值:
df.set_index("Country").index.unique()
df = pd.read_csv("D:\programme\Python\Python学习\数据分析\day05\code\starbucks_store_worldwide.csv")
# 使用matplotlib呈现出店铺总数排名前十的国家
# 准备数据
detail = df.groupby(by="Country").count()["Brand"].sort_values(ascending=False)[:10]
x = detail.index
y = detail.values
# 画图
plt.figure(figsize=(20, 8) ,dpi=80)
plt.bar(x, y)
plt.show()
本文来自博客园,作者:Kenny_LZK,转载请注明原文链接:https://www.cnblogs.com/liuzhongkun/p/15813700.html