从条码识别中学习到的(来自课程《OpenCV计算机视觉产品实战2》)
零、基本情况
条码(一维码)已经广泛应用于我们日常生产实际,
传统的条码识读方法是使用专用的激光扫描器来扫描条码,从而获取条码中的信息。这个过程人工介入程度较深、一般用于吞吐量较大的专业领域。
近年来随着图像处理技术的发展,特别是终端手持设备性能的增强,广泛出现基于图像进行识别的情况。
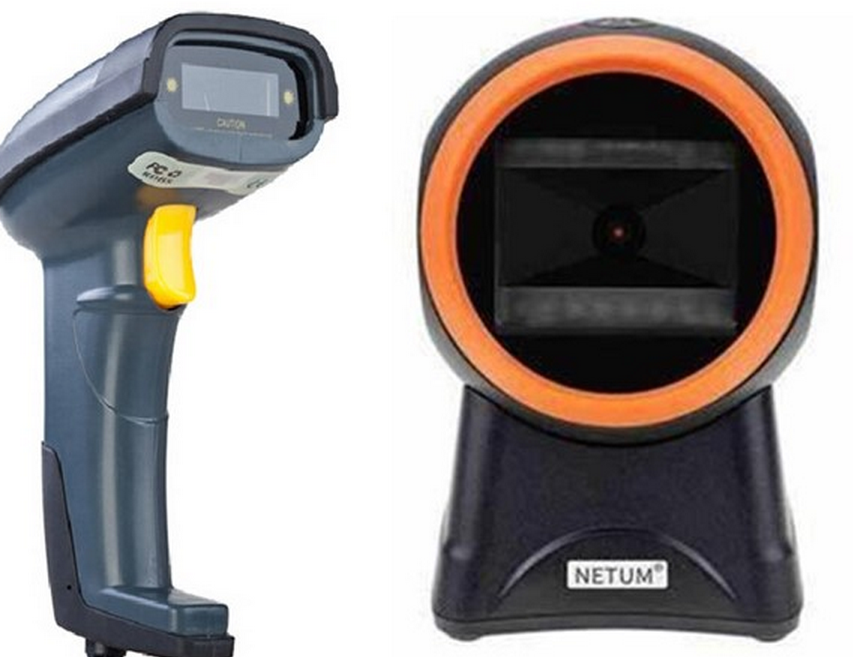
其中非常典型的情况就是在工业控制领域,存在一种专门用来高速读码的设备,用于处理工业场景下的读码需求
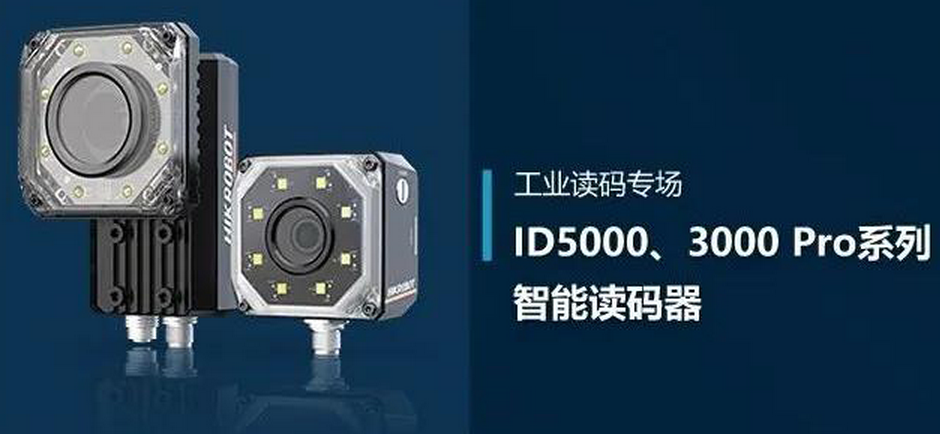
解决的问题是自然环境中,任意条形码的识别问题。作为比较,传统的方法在这里:
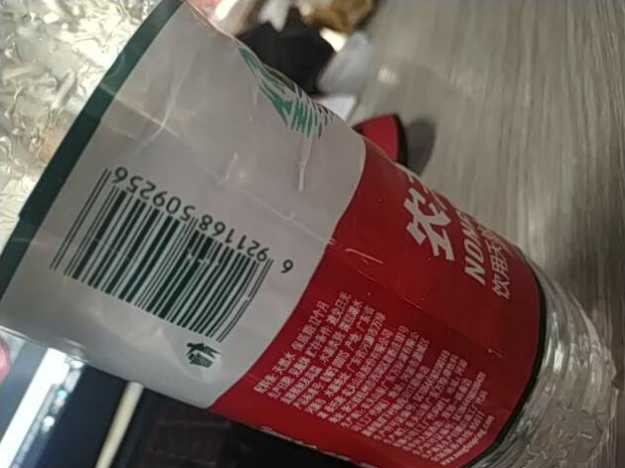
目前能够解码的数量比较有限
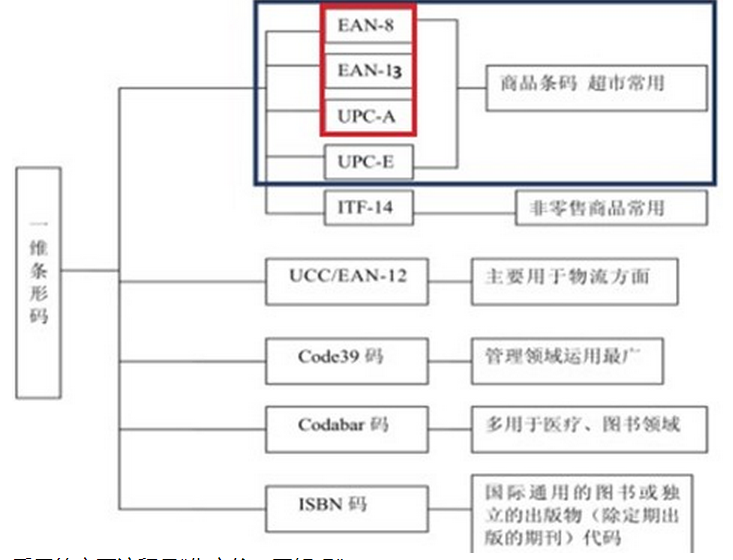
采用的主要流程是“先定位、再解码”
1、首先,基于梯度一致性实现条码定位
2、基于原理实现的条码识别
3、较zxing和 zbar均识别准确率和速度的提高,缺陷是支持的识别码较少 (对比参考:数据集地址:https://github.com/SUSTech-OpenCV/BarcodeTestDataset,共250张条码图片)上进行了测试,我们的识别算法正确率达到了96%,速度为20ms每张图像。作为对比,我们也测试了ZXing在该数据集上的表现,其正确率为64.4%,速度为90ms每张图像。)
opencv中已经出现相关函数
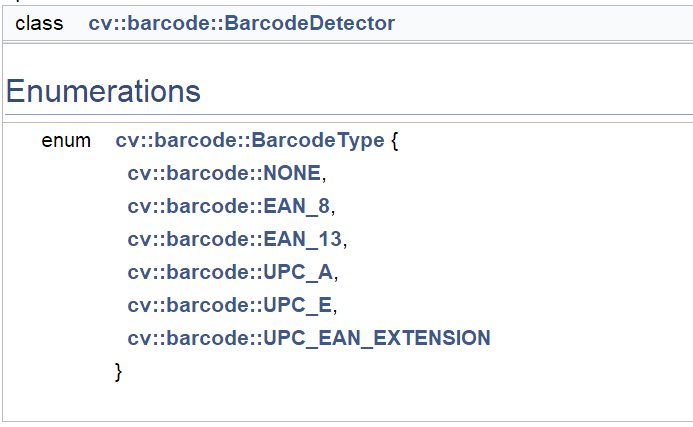
具体的使用说明参考:
#include "opencv2/barcode.hpp"
#include "opencv2/imgproc.hpp"
#include "opencv2/imgproc.hpp"
using namespace cv; Ptr<barcode::BarcodeDetector> bardet = makePtr<barcode::BarcodeDetector>("sr.prototxt", "sr.caffemodel"); //如果不使用超分辨率则可以不指定模型路径 Mat input = imread("your file path"); Mat corners; //返回的检测框的四个角点坐标,如果检测到N个条码,那么维度应该是[N][4][2] std::vector<std::string> decoded_info; //返回的解码结果,如果解码失败,则为空string std::vector<barcode::BarcodeType> decoded_format; //返回的条码类型,如果解码失败,则为BarcodeType::NONE bool ok = bardet->detectAndDecode(input, decoded_info, decoded_format, corners); import cv2 bardet = cv2.barcode_BarcodeDetector() img = cv2.imread("your file path") ok, decoded_info, decoded_type, corners = bardet.detectAndDecode(img)
提交的内容:
barcode目前被安排到contrib库中,交流过程:https://github.com/opencv/opencv_contrib/pull/2757
形码定位部分
一、条形码定位
定位的结果是获得条码的位置
vector<Point2f> points; bool ok = this->detect(img, points);
经过研究,选择了“梯度方向一致性”的解决方法:
• 基于条码区域的纹理信息和形状信息来定位条码
• 基本形态学操作来定位
• Hough变化检测直线来进行条码定位
• 利用条码区域梯度方向的一致性定位条码
• 基于神经网络的条码定位方法
• 基本形态学操作来定位
• Hough变化检测直线来进行条码定位
• 利用条码区域梯度方向的一致性定位条码
• 基于神经网络的条码定位方法

所谓(局部)梯度方向一致性,就是首先对图像进行分块,而后计算每块的梯度大小,最后进行筛选
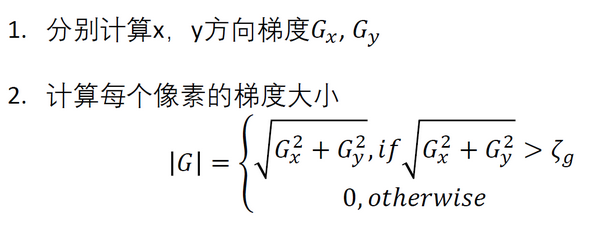
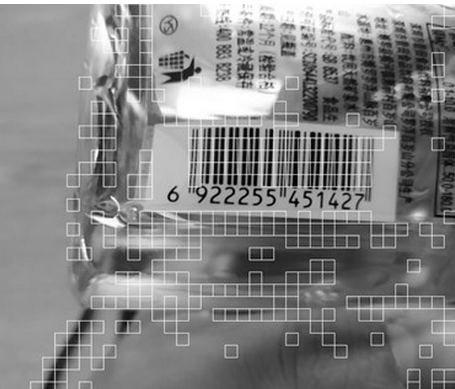
而后基于改进的腐蚀,进一步处理
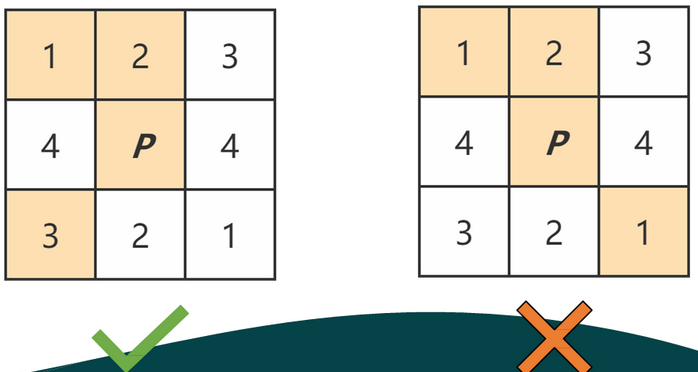
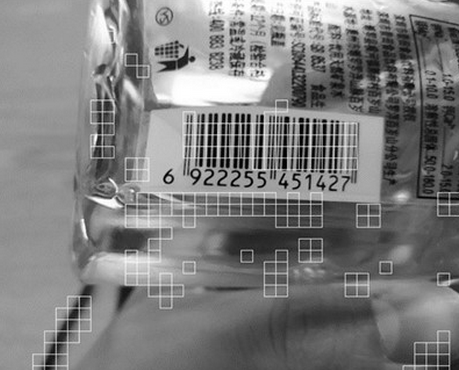
再经过“块联通”“最小外接矩形拟合”“候选区域筛选”“非极大值抑制”的方法定位:
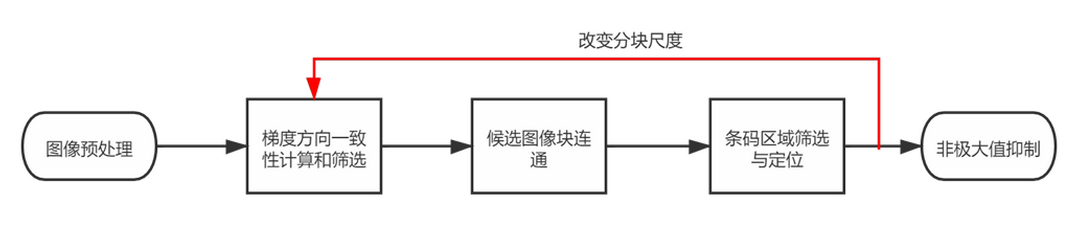
这个Pipline虽然不是非常复杂,但是如果确实能够提供好的效果,非常值得学习。
来看代码:
bool BarcodeDetector::detect(InputArray img, OutputArray points) const { Mat inarr; if (!checkBarInputImage(img, inarr)) //主要是判断图像是否存在 { points.release(); return false; } Detect bardet; bardet.init(inarr); //主要是将图像进行最佳识别尺度缩放 bardet.localization();//主要是用于图像定位 if (!bardet.computeTransformationPoints())//计算出旋转方法 { return false; } vector<vector<Point2f>> pnts2f = bardet.getTransformationPoints(); vector<Point2f> trans_points; for (auto &i : pnts2f) { for (const auto &j : i) { trans_points.push_back(j); } } updatePointsResult(points, trans_points);//输出结果 return true; }
其中,checkBarInputImage实现的是对图像的检测,是值得复用的
static bool checkBarInputImage(InputArray img, Mat &gray) { CV_Assert(!img.empty()); CV_CheckDepthEQ(img.depth(), CV_8U, ""); if (img.cols() <= 40 || img.rows() <= 40) { return false; // image data is not enough for providing reliable results } int incn = img.channels(); CV_Check(incn, incn == 1 || incn == 3 || incn == 4, ""); if (incn == 3 || incn == 4) { cvtColor(img, gray, COLOR_BGR2GRAY); } else { gray = img.getMat(); } return true; }
init主要实现的是缩放,我们知道对于识别来说,过大的图片也是不合适的
void Detect::init(const Mat &src) { const double min_side = std::min(src.size().width, src.size().height); if (min_side > 512.0) { purpose = SHRINKING; coeff_expansion = min_side / 512.0; width = cvRound(src.size().width / coeff_expansion); height = cvRound(src.size().height / coeff_expansion); Size new_size(width, height); resize(src, resized_barcode, new_size, 0, 0, INTER_AREA); } else { purpose = UNCHANGED; coeff_expansion = 1.0; width = src.size().width; height = src.size().height; resized_barcode = src.clone(); } }
localization才是实际上PipleLine的主体。但是在具体实现上显然是没有一致对应的。
void Detect::localization() { localization_bbox.clear(); bbox_scores.clear(); preprocess(); //获得积分图像 //基于经验的缩放设定 static constexpr float SCALE_LIST[] = {0.01f, 0.03f, 0.06f, 0.08f}; const auto min_side = static_cast<float>(std::min(width, height)); int window_size; for (const float scale:SCALE_LIST) { window_size = cvRound(min_side * scale); calCoherence(window_size); //计算连贯性 barcodeErode(); //专用腐蚀 regionGrowing(window_size); //区域增长 } }
积分图像:作为类的实现,这些过程变量都作为类的参数,这里的作用主要是计算积分图
Mat resized_barcode, gradient_magnitude, coherence, orientation, edge_nums, integral_x_sq, integral_y_sq, integral_xy, integral_edges; void Detect::preprocess() { Mat scharr_x, scharr_y, temp; static constexpr double THRESHOLD_MAGNITUDE = 64.; Scharr(resized_barcode, scharr_x, CV_32F, 1, 0); //实现梯度 Scharr(resized_barcode, scharr_y, CV_32F, 0, 1); // calculate magnitude of gradient and truncate magnitude(scharr_x, scharr_y, temp); //计算平方和 threshold(temp, temp, THRESHOLD_MAGNITUDE, 1, THRESH_BINARY); //根据经验过滤 temp.convertTo(gradient_magnitude, CV_8U); integral(gradient_magnitude, integral_edges, CV_32F); //计算出积分,用于边缘? for (int y = 0; y < height; y++) { auto *const x_row = scharr_x.ptr<float_t>(y); auto *const y_row = scharr_y.ptr<float_t>(y); auto *const magnitude_row = gradient_magnitude.ptr<uint8_t>(y); for (int pos = 0; pos < width; pos++) { if (magnitude_row[pos] == 0)//根据平方和反馈原始图像 { x_row[pos] = 0; y_row[pos] = 0; continue; } if (x_row[pos] < 0)//全部翻转? { x_row[pos] *= -1; y_row[pos] *= -1; } } } integral(scharr_x, temp, integral_x_sq, CV_32F, CV_32F); //X的平方 integral(scharr_y, temp, integral_y_sq, CV_32F, CV_32F); //Y的平方 integral(scharr_x.mul(scharr_y), integral_xy, temp, CV_32F, CV_32F);//X乘以Y }
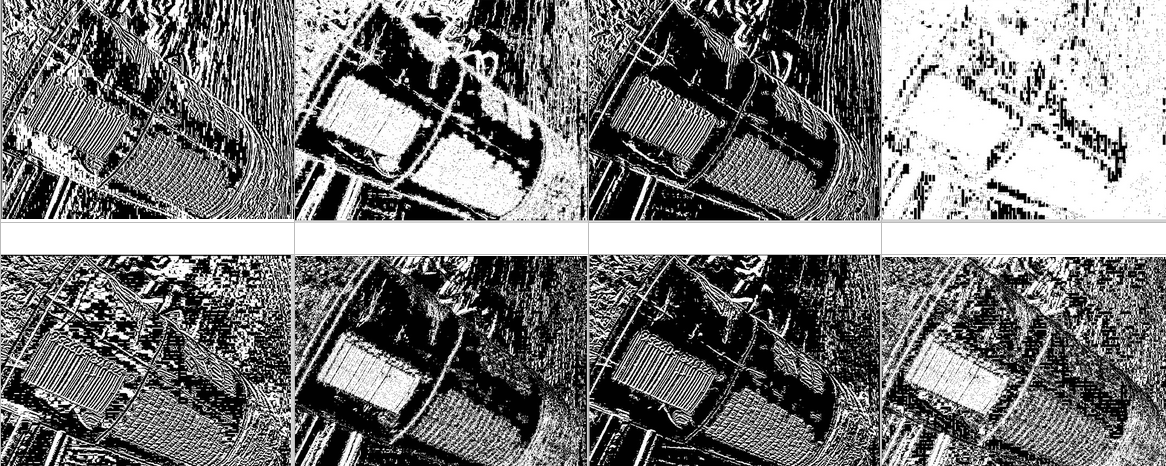
if (magnitude_row[pos] == 0)//根据平方和反馈原始图像 { x_row[pos] = 0; y_row[pos] = 0; continue; } if (x_row[pos] < 0)//全部翻转? { x_row[pos] *= -1; y_row[pos] *= -1; } if (magnitude_row[pos] == 0) { x_row[pos] = 0; y_row[pos] = 0; continue; } if (x_row[pos] < 0)//全部翻转? { x_row[pos] *= -1; y_row[pos] *= -1; }
计算连通性,这里实际上为计算“区域一致性”在做准备
//连贯性计算 // Change coherence orientation edge_nums // depend on width height integral_edges integral_x_sq integral_y_sq integral_xy void Detect::calCoherence(int window_size) { static constexpr float THRESHOLD_COHERENCE = 0.9f; int right_col, left_col, top_row, bottom_row; float xy, x_sq, y_sq, d, rect_area; const float THRESHOLD_AREA = float(window_size * window_size) * 0.42f; Size new_size(width / window_size, height / window_size); coherence = Mat(new_size, CV_8U), orientation = Mat(new_size, CV_32F), edge_nums = Mat(new_size, CV_32F); float top_left, top_right, bottom_left, bottom_right; int integral_cols = width + 1; const auto *edges_ptr = integral_edges.ptr<float_t>(), *x_sq_ptr = integral_x_sq.ptr<float_t>(), *y_sq_ptr = integral_y_sq.ptr<float_t>(), *xy_ptr = integral_xy.ptr<float_t>(); for (int y = 0; y < new_size.height; y++) { auto *coherence_row = coherence.ptr<uint8_t>(y); auto *orientation_row = orientation.ptr<float_t>(y); auto *edge_nums_row = edge_nums.ptr<float_t>(y); if (y * window_size >= height) { continue; } top_row = y * window_size; bottom_row = min(height, (y + 1) * window_size); for (int pos = 0; pos < new_size.width; pos++) { // then calculate the column locations of the rectangle and set them to -1 // if they are outside the matrix bounds if (pos * window_size >= width) { continue; } left_col = pos * window_size; right_col = min(width, (pos + 1) * window_size); //we had an integral image to count non-zero elements CALCULATE_SUM(edges_ptr, rect_area) if (rect_area < THRESHOLD_AREA) { // smooth region coherence_row[pos] = 0; continue; } CALCULATE_SUM(x_sq_ptr, x_sq) CALCULATE_SUM(y_sq_ptr, y_sq) CALCULATE_SUM(xy_ptr, xy) // get the values of the rectangle corners from the integral image - 0 if outside bounds d = sqrt((x_sq - y_sq) * (x_sq - y_sq) + 4 * xy * xy) / (x_sq + y_sq); if (d > THRESHOLD_COHERENCE) //根据梯度一致性的条码检测 { coherence_row[pos] = 255; orientation_row[pos] = atan2(x_sq - y_sq, 2 * xy) / 2.0f; edge_nums_row[pos] = rect_area; } else { coherence_row[pos] = 0; } } } }
专用腐蚀,我是第一次见到:
关于腐蚀内核,是人为确定的
255, 0, 0,
0, 0, 0,
0, 0, 255
0, 0, 255,
0, 0, 0,
255, 0, 0
0, 0, 0,
255, 0, 255,
0, 0, 0
0, 255, 0,
0, 0, 0,
0, 255, 0
inline const std::array<Mat, 4> &getStructuringElement() { static const std::array<Mat, 4> structuringElement{ Mat_<uint8_t>{{3, 3}, {255, 0, 0, 0, 0, 0, 0, 0, 255}}, Mat_<uint8_t>{{3, 3}, {0, 0, 255, 0, 0, 0, 255, 0, 0}}, Mat_<uint8_t>{{3, 3}, {0, 0, 0, 255, 0, 255, 0, 0, 0}}, Mat_<uint8_t>{{3, 3}, {0, 255, 0, 0, 0, 0, 0, 255, 0}}}; return structuringElement; }
最后把这几个核加起来
void Detect::barcodeErode() { static const std::array<Mat, 4> &structuringElement = getStructuringElement(); Mat m0, m1, m2, m3; dilate(coherence, m0, structuringElement[0]); dilate(coherence, m1, structuringElement[1]); dilate(coherence, m2, structuringElement[2]); dilate(coherence, m3, structuringElement[3]); int sum; for (int y = 0; y < coherence.rows; y++) { auto coherence_row = coherence.ptr<uint8_t>(y); auto m0_row = m0.ptr<uint8_t>(y); auto m1_row = m1.ptr<uint8_t>(y); auto m2_row = m2.ptr<uint8_t>(y); auto m3_row = m3.ptr<uint8_t>(y); for (int pos = 0; pos < coherence.cols; pos++) { if (coherence_row[pos] != 0) { sum = m0_row[pos] + m1_row[pos] + m2_row[pos] + m3_row[pos]; //more than 2 group coherence_row[pos] = sum > 600 ? 255 : 0; } } }
虽然比较复杂,但是在包边性上,好像真得有点意思:关于这些东西,我都是可以专门来写一些的,不要急。
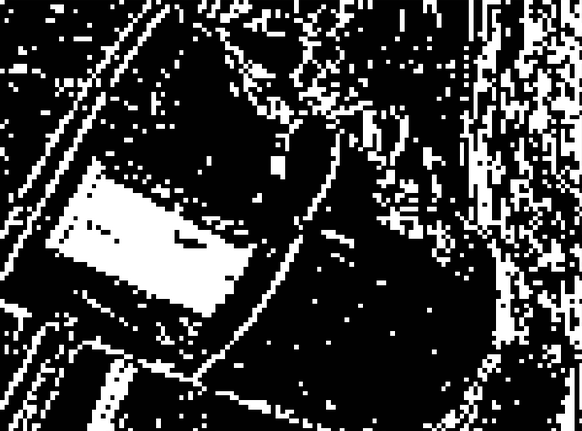
// will change localization_bbox bbox_scores // will change coherence, // depend on coherence orientation edge_nums void Detect::regionGrowing(int window_size) { static constexpr float LOCAL_THRESHOLD_COHERENCE = 0.95f, THRESHOLD_RADIAN = PI / 30, LOCAL_RATIO = 0.5f, EXPANSION_FACTOR = 1.2f; static constexpr uint THRESHOLD_BLOCK_NUM = 35; Point pt_to_grow, pt; //point to grow float src_value; float cur_value; float edge_num; float rect_orientation; float sin_sum, cos_sum; uint counter; //grow direction static constexpr int DIR[8][2] = {{-1, -1}, {0, -1}, {1, -1}, {1, 0}, {1, 1}, {0, 1}, {-1, 1}, {-1, 0}}; vector<Point2f> growingPoints, growingImgPoints; for (int y = 0; y < coherence.rows; y++) { auto *coherence_row = coherence.ptr<uint8_t>(y); for (int x = 0; x < coherence.cols; x++) { if (coherence_row[x] == 0) { continue; } // flag coherence_row[x] = 0; growingPoints.clear(); growingImgPoints.clear(); pt = Point(x, y); cur_value = orientation.at<float_t>(pt); sin_sum = sin(2 * cur_value); cos_sum = cos(2 * cur_value); counter = 1; edge_num = edge_nums.at<float_t>(pt); growingPoints.push_back(pt); growingImgPoints.push_back(Point(pt)); while (!growingPoints.empty()) { pt = growingPoints.back(); growingPoints.pop_back(); src_value = orientation.at<float_t>(pt); //growing in eight directions for (auto i : DIR) { pt_to_grow = Point(pt.x + i[0], pt.y + i[1]); //check if out of boundary if (!isValidCoord(pt_to_grow, coherence.size())) { continue; } if (coherence.at<uint8_t>(pt_to_grow) == 0) { continue; } cur_value = orientation.at<float_t>(pt_to_grow); if (abs(cur_value - src_value) < THRESHOLD_RADIAN || abs(cur_value - src_value) > PI - THRESHOLD_RADIAN) { coherence.at<uint8_t>(pt_to_grow) = 0; sin_sum += sin(2 * cur_value); cos_sum += cos(2 * cur_value); counter += 1; edge_num += edge_nums.at<float_t>(pt_to_grow); growingPoints.push_back(pt_to_grow); //push next point to grow back to stack growingImgPoints.push_back(pt_to_grow); } } } //minimum block num if (counter < THRESHOLD_BLOCK_NUM) { continue; } float local_coherence = (sin_sum * sin_sum + cos_sum * cos_sum) / static_cast<float>(counter * counter); // minimum local gradient orientation_arg coherence_arg if (local_coherence < LOCAL_THRESHOLD_COHERENCE) { continue; } RotatedRect minRect = minAreaRect(growingImgPoints); if (edge_num < minRect.size.area() * float(window_size * window_size) * LOCAL_RATIO || static_cast<float>(counter) < minRect.size.area() * LOCAL_RATIO) { continue; } const float local_orientation = atan2(cos_sum, sin_sum) / 2.0f; // only orientation_arg is approximately equal to the rectangle orientation_arg rect_orientation = (minRect.angle) * PI / 180.f; if (minRect.size.width < minRect.size.height) { rect_orientation += (rect_orientation <= 0.f ? HALF_PI : -HALF_PI); std::swap(minRect.size.width, minRect.size.height); } if (abs(local_orientation - rect_orientation) > THRESHOLD_RADIAN && abs(local_orientation - rect_orientation) < PI - THRESHOLD_RADIAN) { continue; } minRect.angle = local_orientation * 180.f / PI; minRect.size.width *= static_cast<float>(window_size) * EXPANSION_FACTOR; minRect.size.height *= static_cast<float>(window_size); minRect.center.x = (minRect.center.x + 0.5f) * static_cast<float>(window_size); minRect.center.y = (minRect.center.y + 0.5f) * static_cast<float>(window_size); localization_bbox.push_back(minRect); bbox_scores.push_back(edge_num); } }
关于区域增长的一些代码,我也是一直比较缺乏有效积累。所以还是首先要从实验开始,对比现有传统方法和这里的比较复杂的方法的差异,而后找到这里存在的问题,最后获得较好的收获。
bool Detect::computeTransformationPoints() { bbox_indices.clear(); transformation_points.clear(); transformation_points.reserve(bbox_indices.size()); RotatedRect rect; Point2f temp[4]; const float THRESHOLD_SCORE = float(width * height) / 300.f; dnn::NMSBoxes(localization_bbox, bbox_scores, THRESHOLD_SCORE, 0.1f, bbox_indices);//非最大值抑制 for (const auto &bbox_index : bbox_indices) { rect = localization_bbox[bbox_index]; if (purpose == ZOOMING) { rect.center /= coeff_expansion; rect.size.height /= static_cast<float>(coeff_expansion); rect.size.width /= static_cast<float>(coeff_expansion); } else if (purpose == SHRINKING) { rect.center *= coeff_expansion; rect.size.height *= static_cast<float>(coeff_expansion); rect.size.width *= static_cast<float>(coeff_expansion); } rect.points(temp); transformation_points.emplace_back(vector<Point2f>{temp[0], temp[1], temp[2], temp[3]}); } return !transformation_points.empty(); }
这个函数主要实现的就是一个非最大值的抑制,并且将结果填入到可以被调用的地方。
以目标检测为例:目标检测的过程中在同一目标的位置上会产生大量的候选框,这些候选框相互之间可能会有重叠,此时我们需要利用非极大值抑制找到最佳的目标边界框,消除冗余的边界框。Demo如
左图是人脸检测的候选框结果,每个边界框有一个置信度得分(confidence score),如果不使用非极大值抑制,就会有多个候选框出现。右图是使用非极大值抑制之后的结果,符合我们人脸检测的预期结果。
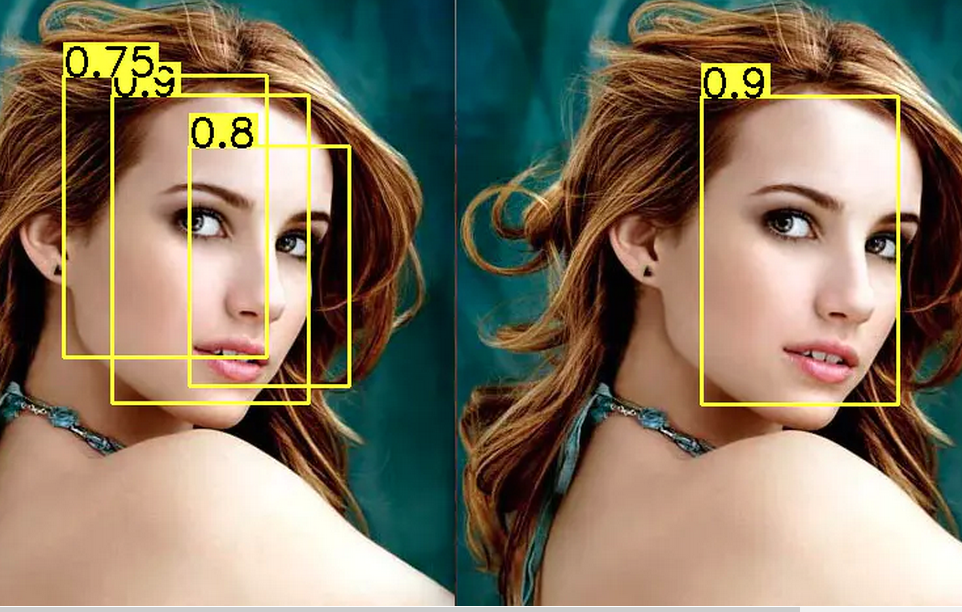
二、条形码识别
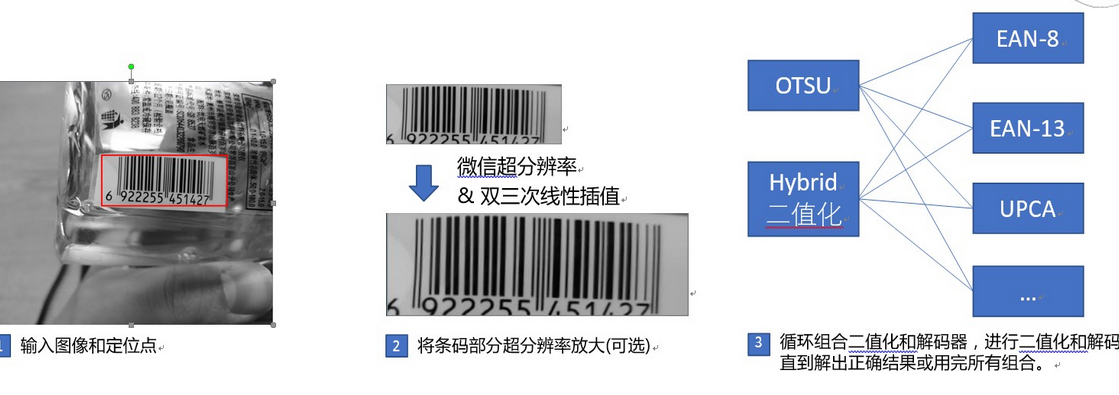
这里,直接调用了较为成熟的“微信超分辨率”模块,也是值得关注的。
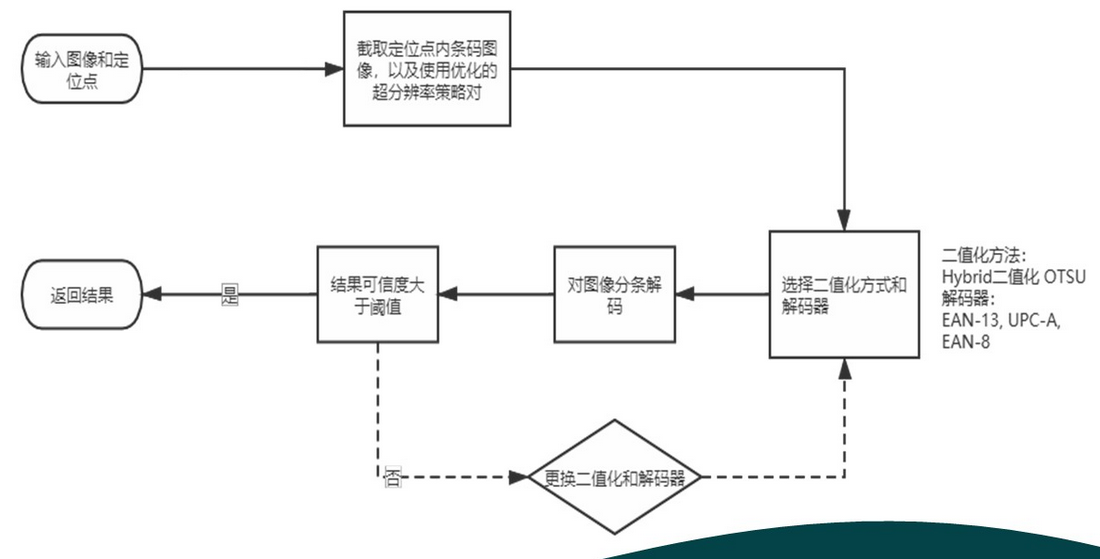
其中:优化的超分辨率策略指的是对较小的条码进行超分辨率放大,不同大小条码做不同处理。
- 解码算法的核心是基于条码编码方式的向量距离计算。因为条码的编码格式为固定的数个"条空",所以可以在约定好"条空"间隔之后。将固定的条空读取为一个向量,接下来与约定好的编码格式向匹配,取匹配程度最高的编码为结果。
- 在解码步骤中,解码的单位为一条线,由于噪点,条空的粘连等原因,单独条码的解码结果存在较大的不确定性,因此我们加入了对多条线的扫码,通过对均匀分布的扫描与解码,能够将二值化过程中的一些不完美之处加以抹除。
具体实现为:首先在检测线上寻找起始符,寻找到起始符之后,对前半部分进行读取与解码,接着寻找中间分割符,接着对后半部分进行读取与解码,最后寻找终结符,并对整个条码进行首位生成与校验(此处以EAN13格式举例,不同格式不尽相同)。最后,每条线都会存在一个解码结果,所以对其进行投票,只将最高且总比例在有效结果50%以上的结果返回。这一部分我们基于ZXing的算法实现做了一些改进(投票等)。 - 更换二值化和解码器指的是在为解码成功遍历使用每种解码器和二值化尝试解码。
- 在检测中识别中,都是有内循环的。这个部分我在之前能够部分实现,但是也缺乏系统梳理。
三、opencv PR经验
1、尽可能早
2、commit少量多次
3、符合规范
4、充分交流
此外,opencv_extra的提交方法上有特别需要注意的地方,这个问题我应该还没有解决。
参考资料:
void cv::magnitude | ( | InputArray | x, |
InputArray | y, | ||
OutputArray | magnitude | ||
) |
Python: | ||||||||||||||||||||||||||||||||||||||||||||||||||||||||||||||||||||||||||||||||||||||||||||||||||||||||||||||||||||||||||||||||||||||||||||||||||||||||||||||||||||||||||||||||||||||||||||||||||||||||||||||||||||||||||||||||||||||||||||||||||||||||||||||||||||||||||||||||||||||||||||||||||||||||||||||||||||||||||||||||||||||||||||||||||||||||||||||||||||||||||||||||||||||||||||||||||||||||||||||||||||||||||||||||||||||||||||||||||||||||||||||||||||||||||||||||||||||||||||||||||||||||||||||||||||||||||||||||||||||||||||||||||||||||||||||||||||||||||||||||||||||||||||||||||||||||||||||||||||||||||||||||||||||||||||||||||||||||||||||||||||||||||||||||||||||||||||||||||||||||||||||||||||||||||||||||||||||||||||||||||||||||||||||||||||||||||||||||||||||||||||||||||||||||||||||||||||||||||||||||||||||||||||||||||||||||||||||||||||||||||||||||||||||||||||||||||||||||||||||||||||||||||||||||||||||||||||||||||||||||||||||||||||||||||||||||||||||||||||||||||||||||||||||||||||||||||||||||||||||
---|---|---|---|---|---|---|---|---|---|---|---|---|---|---|---|---|---|---|---|---|---|---|---|---|---|---|---|---|---|---|---|---|---|---|---|---|---|---|---|---|---|---|---|---|---|---|---|---|---|---|---|---|---|---|---|---|---|---|---|---|---|---|---|---|---|---|---|---|---|---|---|---|---|---|---|---|---|---|---|---|---|---|---|---|---|---|---|---|---|---|---|---|---|---|---|---|---|---|---|---|---|---|---|---|---|---|---|---|---|---|---|---|---|---|---|---|---|---|---|---|---|---|---|---|---|---|---|---|---|---|---|---|---|---|---|---|---|---|---|---|---|---|---|---|---|---|---|---|---|---|---|---|---|---|---|---|---|---|---|---|---|---|---|---|---|---|---|---|---|---|---|---|---|---|---|---|---|---|---|---|---|---|---|---|---|---|---|---|---|---|---|---|---|---|---|---|---|---|---|---|---|---|---|---|---|---|---|---|---|---|---|---|---|---|---|---|---|---|---|---|---|---|---|---|---|---|---|---|---|---|---|---|---|---|---|---|---|---|---|---|---|---|---|---|---|---|---|---|---|---|---|---|---|---|---|---|---|---|---|---|---|---|---|---|---|---|---|---|---|---|---|---|---|---|---|---|---|---|---|---|---|---|---|---|---|---|---|---|---|---|---|---|---|---|---|---|---|---|---|---|---|---|---|---|---|---|---|---|---|---|---|---|---|---|---|---|---|---|---|---|---|---|---|---|---|---|---|---|---|---|---|---|---|---|---|---|---|---|---|---|---|---|---|---|---|---|---|---|---|---|---|---|---|---|---|---|---|---|---|---|---|---|---|---|---|---|---|---|---|---|---|---|---|---|---|---|---|---|---|---|---|---|---|---|---|---|---|---|---|---|---|---|---|---|---|---|---|---|---|---|---|---|---|---|---|---|---|---|---|---|---|---|---|---|---|---|---|---|---|---|---|---|---|---|---|---|---|---|---|---|---|---|---|---|---|---|---|---|---|---|---|---|---|---|---|---|---|---|---|---|---|---|---|---|---|---|---|---|---|---|---|---|---|---|---|---|---|---|---|---|---|---|---|---|---|---|---|---|---|---|---|---|---|---|---|---|---|---|---|---|---|---|---|---|---|---|---|---|---|---|---|---|---|---|---|---|---|---|---|---|---|---|---|---|---|---|---|---|---|---|---|---|---|---|---|---|---|---|---|---|---|---|---|---|---|---|---|---|---|---|---|---|---|---|---|---|---|---|---|---|---|---|---|---|---|---|---|---|---|---|---|---|---|---|---|---|---|---|---|---|---|---|---|---|---|---|---|---|---|---|---|---|---|---|---|---|---|---|---|---|---|---|---|---|---|---|---|---|---|---|---|---|---|---|---|---|---|---|---|---|---|---|---|---|---|---|---|---|---|---|---|---|---|---|---|---|---|---|---|---|---|---|---|---|---|---|---|---|---|---|---|---|---|---|---|---|---|---|---|---|---|---|---|---|---|---|---|---|---|---|---|---|---|---|---|---|---|---|---|---|---|---|---|---|---|---|---|---|---|---|---|---|---|---|---|---|---|---|---|---|---|---|---|---|---|---|---|---|---|---|---|---|---|---|---|---|---|---|---|---|---|---|---|---|---|---|---|---|---|---|---|---|---|---|---|---|---|---|---|---|---|---|---|---|---|---|---|---|---|---|---|---|---|---|---|---|---|---|---|---|---|---|---|---|---|---|---|---|---|---|---|---|---|---|---|---|---|---|---|---|---|---|---|---|---|---|---|---|---|---|---|---|---|---|---|---|---|---|---|---|---|---|---|---|---|---|---|---|---|---|---|---|---|---|---|---|---|---|---|---|---|---|---|---|---|---|---|---|---|---|---|---|---|---|---|---|---|---|---|---|---|---|---|---|---|---|---|---|---|---|---|---|---|---|---|---|---|---|---|---|---|---|---|---|---|---|---|---|---|---|---|---|---|---|---|---|---|---|---|---|---|---|---|---|---|---|---|---|---|---|---|---|---|---|---|---|---|---|---|---|---|---|---|---|---|---|---|---|---|---|---|---|---|---|---|---|---|---|---|---|---|---|---|---|---|---|---|---|---|---|---|---|---|---|---|---|---|---|---|---|---|---|---|---|---|---|---|---|---|---|---|---|---|---|---|---|---|---|---|---|---|---|---|---|---|---|---|---|---|---|---|---|---|---|---|---|---|---|---|---|---|---|---|---|---|---|---|---|---|---|---|---|---|---|---|---|---|---|---|---|---|---|---|---|---|---|---|---|
cv.magnitude( | x, y[, magnitude] | ) -> | magnitude |
#include <opencv2/core.hpp>
Calculates the magnitude of 2D vectors.
The function cv::magnitude calculates the magnitude of 2D vectors formed from the corresponding elements of x and y arrays:
- Parameters
-
x floating-point array of x-coordinates of the vectors. y floating-point array of y-coordinates of the vectors; it must have the same size as x. magnitude output array of the same size and type as x.
void cv::integral | ( | InputArray | src, |
OutputArray | sum, | ||
OutputArray | sqsum, | ||
OutputArray | tilted, | ||
int | sdepth = -1 , |
||
int | sqdepth = -1 |
||
) |
Python: | ||||||||||||||||||||||||||||||||||||||||||||||||||||||||||||||||||||||||||||||||||||||||||||||||||||||||||||||||||||||||||||||||||||||||||||||||||||||||||||||||||||||||||||||||||||||||||||||||||||||||||||||||||||||||||||||||||||||||||||||||||||||||||||||||||||||||||||||||||||||||||||||||||||||||||||||||||||||||||||||||||||||||||||||||||||||||||||||||||||||||||||||||||||||||||||||||||||||||||||||||||||||||||||||||||||||||||||||||||||||||||||||||||||||||||||||||||||||||||||||||||||||||||||||||||||||||||||||||||||||||||||||||||||||||||||||||||||||||||||||||||||||||||||||||||||||||||||||||||||||||||||||||||||||||||||||||||||||||||||||||||||||||||||||||||||||||||||||||||||||||||||||||||||||||||||||||||||||||||||||||||||||||||||||||||||||||||||||||||||||||||||||||||||||||||||||||||||||||||||||||||||||||||||||||||||||||||||||||||||||||||||||||||||||||||||||||||||||||||||||||||||||||||||||||||||||||||||||||||||||||||||||||||||||||||||||||||||||||||||||||||||||||||||||||||||||||||||||||||||||
---|---|---|---|---|---|---|---|---|---|---|---|---|---|---|---|---|---|---|---|---|---|---|---|---|---|---|---|---|---|---|---|---|---|---|---|---|---|---|---|---|---|---|---|---|---|---|---|---|---|---|---|---|---|---|---|---|---|---|---|---|---|---|---|---|---|---|---|---|---|---|---|---|---|---|---|---|---|---|---|---|---|---|---|---|---|---|---|---|---|---|---|---|---|---|---|---|---|---|---|---|---|---|---|---|---|---|---|---|---|---|---|---|---|---|---|---|---|---|---|---|---|---|---|---|---|---|---|---|---|---|---|---|---|---|---|---|---|---|---|---|---|---|---|---|---|---|---|---|---|---|---|---|---|---|---|---|---|---|---|---|---|---|---|---|---|---|---|---|---|---|---|---|---|---|---|---|---|---|---|---|---|---|---|---|---|---|---|---|---|---|---|---|---|---|---|---|---|---|---|---|---|---|---|---|---|---|---|---|---|---|---|---|---|---|---|---|---|---|---|---|---|---|---|---|---|---|---|---|---|---|---|---|---|---|---|---|---|---|---|---|---|---|---|---|---|---|---|---|---|---|---|---|---|---|---|---|---|---|---|---|---|---|---|---|---|---|---|---|---|---|---|---|---|---|---|---|---|---|---|---|---|---|---|---|---|---|---|---|---|---|---|---|---|---|---|---|---|---|---|---|---|---|---|---|---|---|---|---|---|---|---|---|---|---|---|---|---|---|---|---|---|---|---|---|---|---|---|---|---|---|---|---|---|---|---|---|---|---|---|---|---|---|---|---|---|---|---|---|---|---|---|---|---|---|---|---|---|---|---|---|---|---|---|---|---|---|---|---|---|---|---|---|---|---|---|---|---|---|---|---|---|---|---|---|---|---|---|---|---|---|---|---|---|---|---|---|---|---|---|---|---|---|---|---|---|---|---|---|---|---|---|---|---|---|---|---|---|---|---|---|---|---|---|---|---|---|---|---|---|---|---|---|---|---|---|---|---|---|---|---|---|---|---|---|---|---|---|---|---|---|---|---|---|---|---|---|---|---|---|---|---|---|---|---|---|---|---|---|---|---|---|---|---|---|---|---|---|---|---|---|---|---|---|---|---|---|---|---|---|---|---|---|---|---|---|---|---|---|---|---|---|---|---|---|---|---|---|---|---|---|---|---|---|---|---|---|---|---|---|---|---|---|---|---|---|---|---|---|---|---|---|---|---|---|---|---|---|---|---|---|---|---|---|---|---|---|---|---|---|---|---|---|---|---|---|---|---|---|---|---|---|---|---|---|---|---|---|---|---|---|---|---|---|---|---|---|---|---|---|---|---|---|---|---|---|---|---|---|---|---|---|---|---|---|---|---|---|---|---|---|---|---|---|---|---|---|---|---|---|---|---|---|---|---|---|---|---|---|---|---|---|---|---|---|---|---|---|---|---|---|---|---|---|---|---|---|---|---|---|---|---|---|---|---|---|---|---|---|---|---|---|---|---|---|---|---|---|---|---|---|---|---|---|---|---|---|---|---|---|---|---|---|---|---|---|---|---|---|---|---|---|---|---|---|---|---|---|---|---|---|---|---|---|---|---|---|---|---|---|---|---|---|---|---|---|---|---|---|---|---|---|---|---|---|---|---|---|---|---|---|---|---|---|---|---|---|---|---|---|---|---|---|---|---|---|---|---|---|---|---|---|---|---|---|---|---|---|---|---|---|---|---|---|---|---|---|---|---|---|---|---|---|---|---|---|---|---|---|---|---|---|---|---|---|---|---|---|---|---|---|---|---|---|---|---|---|---|---|---|---|---|---|---|---|---|---|---|---|---|---|---|---|---|---|---|---|---|---|---|---|---|---|---|---|---|---|---|---|---|---|---|---|---|---|---|---|---|---|---|---|---|---|---|---|---|---|---|---|---|---|---|---|---|---|---|---|---|---|---|---|---|---|---|---|---|---|---|---|---|---|---|---|---|---|---|---|---|---|---|---|---|---|---|---|---|---|---|---|---|---|---|---|---|---|---|---|---|---|---|---|---|---|---|---|---|---|---|---|---|---|---|---|---|---|---|---|---|---|---|---|---|---|---|---|---|---|---|---|---|---|---|---|---|---|---|---|---|---|---|---|---|---|---|---|---|---|---|---|---|---|---|---|---|---|---|---|---|---|---|---|---|---|---|---|---|---|---|---|---|---|---|---|---|---|---|---|---|---|---|---|---|---|---|---|---|---|---|---|---|---|---|---|---|---|---|---|---|---|---|---|---|---|---|---|---|---|---|---|
cv.integral( | src[, sum[, sdepth]] | ) -> | sum | |||||||||||||||||||||||||||||||||||||||||||||||||||||||||||||||||||||||||||||||||||||||||||||||||||||||||||||||||||||||||||||||||||||||||||||||||||||||||||||||||||||||||||||||||||||||||||||||||||||||||||||||||||||||||||||||||||||||||||||||||||||||||||||||||||||||||||||||||||||||||||||||||||||||||||||||||||||||||||||||||||||||||||||||||||||||||||||||||||||||||||||||||||||||||||||||||||||||||||||||||||||||||||||||||||||||||||||||||||||||||||||||||||||||||||||||||||||||||||||||||||||||||||||||||||||||||||||||||||||||||||||||||||||||||||||||||||||||||||||||||||||||||||||||||||||||||||||||||||||||||||||||||||||||||||||||||||||||||||||||||||||||||||||||||||||||||||||||||||||||||||||||||||||||||||||||||||||||||||||||||||||||||||||||||||||||||||||||||||||||||||||||||||||||||||||||||||||||||||||||||||||||||||||||||||||||||||||||||||||||||||||||||||||||||||||||||||||||||||||||||||||||||||||||||||||||||||||||||||||||||||||||||||||||||||||||||||||||||||||||||||||||||||||||||||||||||||||||||||
cv.integral2( | src[, sum[, sqsum[, sdepth[, sqdepth]]]] | ) -> | sum, sqsum | |||||||||||||||||||||||||||||||||||||||||||||||||||||||||||||||||||||||||||||||||||||||||||||||||||||||||||||||||||||||||||||||||||||||||||||||||||||||||||||||||||||||||||||||||||||||||||||||||||||||||||||||||||||||||||||||||||||||||||||||||||||||||||||||||||||||||||||||||||||||||||||||||||||||||||||||||||||||||||||||||||||||||||||||||||||||||||||||||||||||||||||||||||||||||||||||||||||||||||||||||||||||||||||||||||||||||||||||||||||||||||||||||||||||||||||||||||||||||||||||||||||||||||||||||||||||||||||||||||||||||||||||||||||||||||||||||||||||||||||||||||||||||||||||||||||||||||||||||||||||||||||||||||||||||||||||||||||||||||||||||||||||||||||||||||||||||||||||||||||||||||||||||||||||||||||||||||||||||||||||||||||||||||||||||||||||||||||||||||||||||||||||||||||||||||||||||||||||||||||||||||||||||||||||||||||||||||||||||||||||||||||||||||||||||||||||||||||||||||||||||||||||||||||||||||||||||||||||||||||||||||||||||||||||||||||||||||||||||||||||||||||||||||||||||||||||||||||||||||
cv.integral3( | src[, sum[, sqsum[, tilted[, sdepth[, sqdepth]]]]] | ) -> | sum, sqsum, tilted |