如何理解K-L散度(相对熵)
K-L散度
Kullback-Leibler Divergence
,即K-L散度
,是一种量化两种概率分布P和Q之间差异的方式,又叫相对熵
。更简单的、近似的分布
来替代观察数据
或太复杂的分布
。K-L散度能帮助我们度量使用一个分布来近似另一个分布时所损失的信息。Cross Entropy
而非K-L Divergence
。我们从下面这个问题出发思考K-L散度。
假设我们是一群太空科学家,经过遥远的旅行,来到了一颗新发现的星球。在这个星球上,生存着一种长有牙齿的蠕虫,引起了我们的研究兴趣。我们发现这种蠕虫生有10颗牙齿,但是因为不注意口腔卫生,又喜欢嚼东西,许多蠕虫会掉牙。收集大量样本之后,我们得到关于蠕虫牙齿数量的经验分布,如下图所示
均分布
,因为蠕虫牙齿不会超过10颗,所以有11个可能值,那蠕虫的牙齿数量概率都为 1/11
。分布图如下: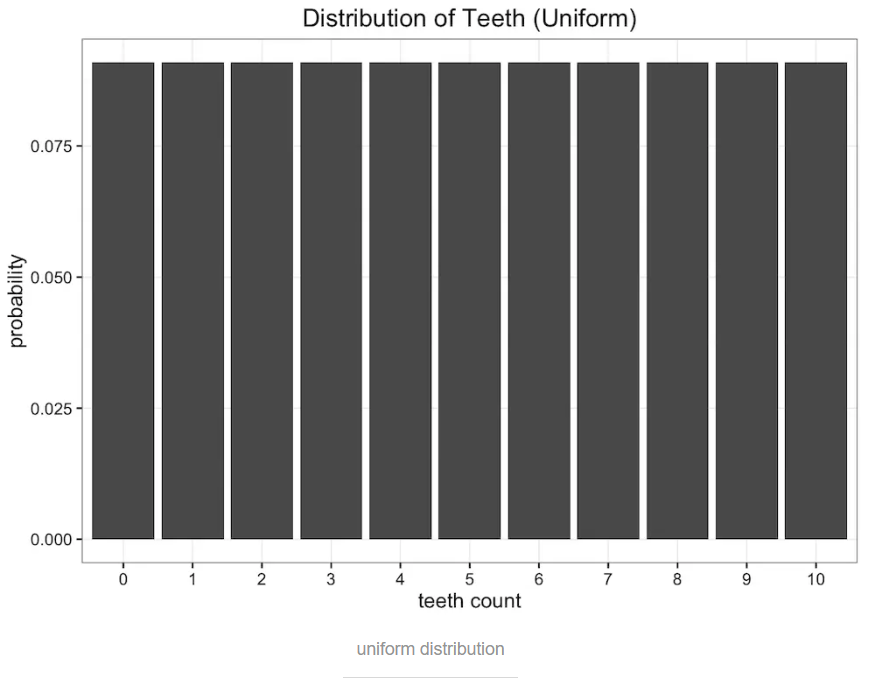
二项式分布binomial distribution
。蠕虫嘴里面共有n=10
个牙槽,每个牙槽出现牙齿与否为独立事件,且概率均为p
。则蠕虫牙齿数量即为期望值E[x]=n*p
,真实期望值即为观察数据的平均值,比如说5.7
,则p=0.57
,得到如下图所示的二项式分布: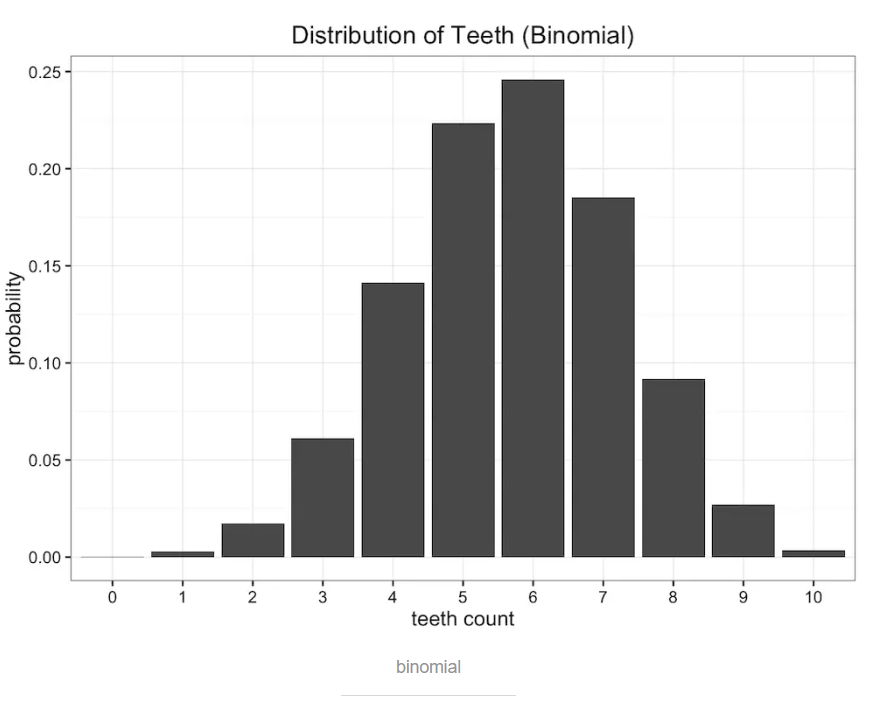
对比一下原始数据,可以看出均分布和二项分布都不能完全描述原始分布。
已经有许多度量误差的方式存在,但是我们所要考虑的是减小发送的信息量。上面讨论的均分布和二项式分布都把问题规约到只需要两个参数,牙齿数量和概率值(均分布只需要牙齿数量即可)。那么哪个分布保留了更多的原始数据分布的信息呢?这个时候就需要K-L散度登场了。
数据的熵
K-L散度源于信息论。信息论主要研究如何量化数据中的信息。最重要的信息度量单位是熵
Entropy,一般用H
表示。分布的熵的公式如下:
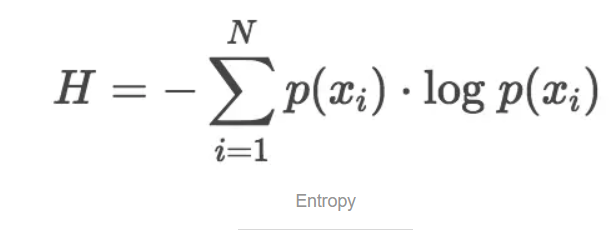
K-L散度度量信息损失
只需要稍加修改熵H
的计算公式就能得到K-L散度
的计算公式。设p
为观察得到的概率分布,q
为另一分布来近似p
,则p
、q
的K-L散度
为: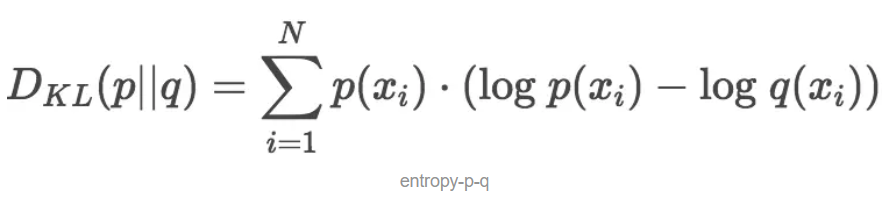
显然,根据上面的公式,K-L散度其实是数据的原始分布p和近似分布q之间的对数差值的期望。如果继续用2
为底的对数计算,则K-L散度值表示信息损失的二进制位数。下面公式以期望表达K-L散度:

一般,K-L散度以下面的书写方式更常见:
OK,现在我们知道当用一个分布来近似另一个分布时如何计算信息损失量了。接下来,让我们重新回到最开始的蠕虫牙齿数量概率分布的问题。
首先是用均分布来近似原始分布的K-L散度:
接下来计算用二项式分布近似原始分布的K-L散度:
通过上面的计算可以看出,使用均分布近似原始分布的信息损失要比用二项式分布近似小。所以,如果要从均分布和二项式分布中选择一个的话,均分布更好些。
散度并非距离
很自然地,一些同学把K-L散度看作是不同分布之间距离的度量。这是不对的,因为从K-L散度的计算公式就可以看出它不符合对称性(距离度量应该满足对称性)。如果用我们上面观察的数据分布来近似二项式分布,得到如下结果:

Dkl (Observed || Binomial) != Dkl (Binomial || Observed)
。也就是说,用p
近似q
和用q
近似p
,二者所损失的信息并不是一样的。使用K-L散度优化模型
前面使用的二项式分布的参数是概率 p=0.57
,是原始数据的均值。p
的值域在 [0, 1] 之间,我们要选择一个p
值,建立二项式分布,目的是最小化近似误差,即K-L散度。那么0.57
是最优的吗?
下图是原始数据分布和二项式分布的K-L散度变化随二项式分布参数p
变化情况:
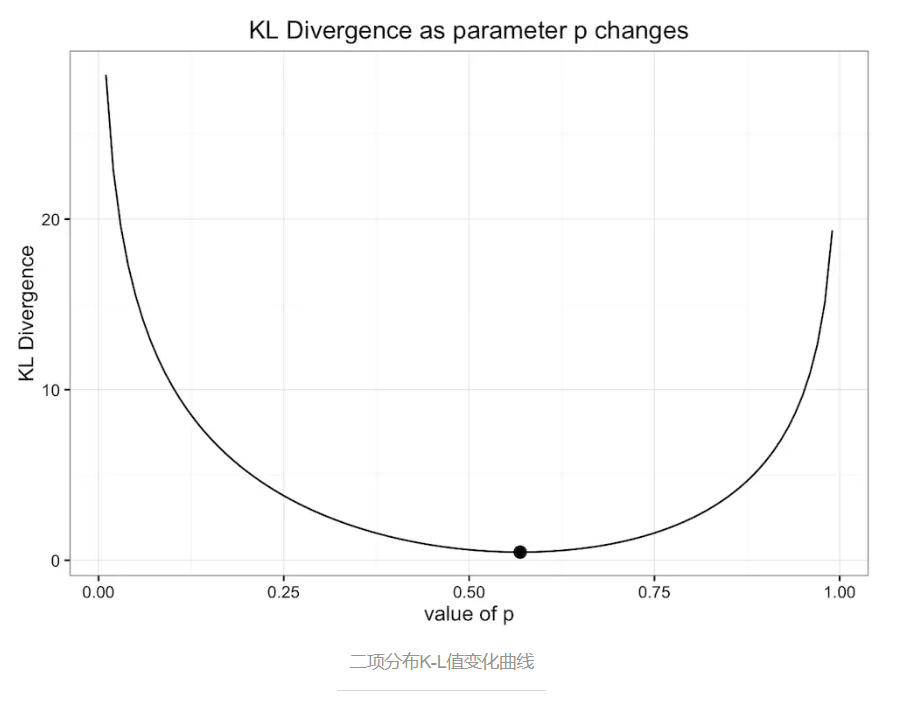
通过上面的曲线图可以看出,K-L散度值在圆点处最小,即p=0.57
。所以我们之前的二项式分布模型已经是最优的二项式模型了。注意,我已经说了,是二项式模型,这里只限定在二项式模型范围内。
前面只考虑了均分布模型和二项式分布模型,接下来我们考虑另外一种模型来近似原始数据。首先把原始数据分成两部分,1)0-5颗牙齿的概率和 2)6-10颗牙齿的概率。概率值如下:
即,一只蠕虫的牙齿数量x=i
的概率为p/5
; x=j
的概率为(1-p) / 6
,i=0,1,2,3,4,5
; j=6,7,8,9,10
。
Aha,我们自己建立了一个新的(奇怪的)模型来近似原始的分布,模型只有一个参数p
,像前面那样优化二项式分布的时候所做的一样,让我们画出K-L散度值随p
变化的情况:
当p=0.47
时,K-L值取最小值0.338
。似曾相识吗?对,这个值和使用均分布的K-L散度值是一样的(这并不能说明什么)!下面我们继续画出这个奇怪模型的概率分布图,看起来确实和均分布的概率分布图相似:
我们自己都说了,这是个奇怪的模型,在K-L值相同的情况下,更倾向于使用更常见的、更简单的均分布模型。
回头看,我们在这一小节中使用K-L散度作为目标方程,分别找到了二项式分布模型的参数p=0.57
和上面这个随手建立的模型的参数p=0.47
。是的,这就是本节的重点:使用K-L散度作为目标方程来优化模型。当然,本节中的模型都只有一个参数,也可以拓展到有更多参数的高维模型中。
变分自编码器VAEs和变分贝叶斯法
f(x)
,通过设定目标函数,可以训练神经网络逼近非常复杂的真实函数g(x)
。训练的关键是要设定目标函数,反馈给神经网络当前的表现如何。训练过程就是不断减小目标函数值的过程。附录
K-L 散度的定义
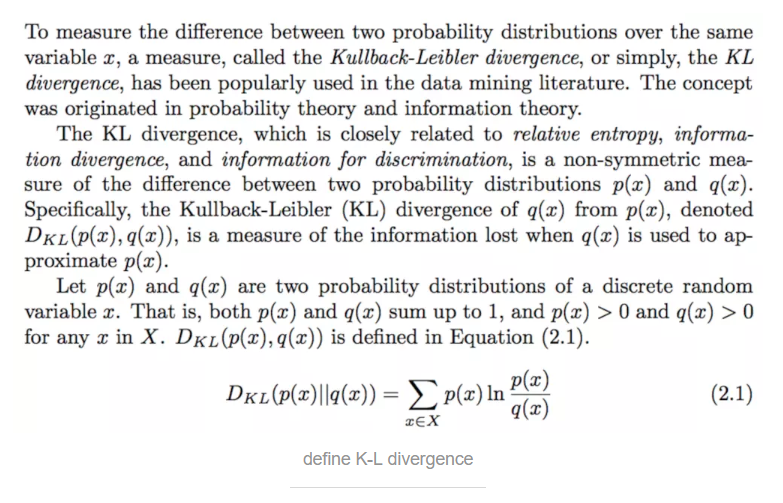
计算K-L的注意事项
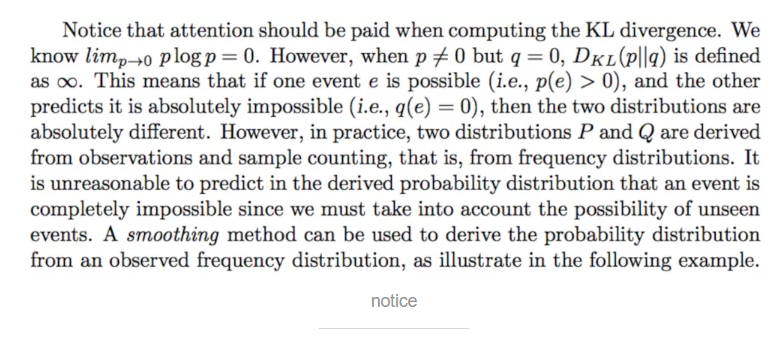
信息熵、交叉熵、相对熵
- 信息熵,即熵,香浓熵。编码方案完美时,最短平均编码长度。
- 交叉熵,cross-entropy。编码方案不一定完美时(由于对概率分布的估计不一定正确),平均编码长度。是神经网络常用的损失函数。
- 相对熵,即K-L散度,relative entropy。编码方案不一定完美时,平均编码长度相对于最小值的增加值。
更详细对比,见知乎如何通俗的解释交叉熵与相对熵?
KL散度可以看做是分布Q和分布P之间远近的一种度量。而交叉熵本身就是这样的一种度量,缺点是自己与自己的交叉熵不为零(等于熵值),因此我们将交叉熵减去熵值,使KL散度更接近距离的定义(不幸的是它依然是不对称的)。
为什么在神经网络中使用交叉熵损失函数,而不是K-L散度?
K-L散度=交叉熵-熵,即 DKL( p||q )=H(p,q)−H(p)
。
在神经网络所涉及到的范围内,H(p)
不变,则DKL( p||q )
等价H(p,q)
。
更多讨论见Why do we use Kullback-Leibler divergence rather than cross entropy in the t-SNE objective function?和Why train with cross-entropy instead of KL divergence in classification?
作者:Will Kurt
If you enjoyed this post please subscribe to keep up to date and follow @willkurt!
If you enjoyed this writing and also like programming languages, you might like the book on Haskell I just finished due in print July 2017 (though nearly all the content is available online today).
Matlab 代码实现
function KL = kldiv(varValue,pVect1,pVect2,varargin) %KLDIV Kullback-Leibler or Jensen-Shannon divergence between two distributions. % KLDIV(X,P1,P2) returns the Kullback-Leibler divergence between two % distributions specified over the M variable values in vector X. P1 is a % length-M vector of probabilities representing distribution 1, and P2 is a % length-M vector of probabilities representing distribution 2. Thus, the % probability of value X(i) is P1(i) for distribution 1 and P2(i) for % distribution 2. The Kullback-Leibler divergence is given by: % % KL(P1(x),P2(x)) = sum[P1(x).log(P1(x)/P2(x))] % % If X contains duplicate values, there will be an warning message, and these % values will be treated as distinct values. (I.e., the actual values do % not enter into the computation, but the probabilities for the two % duplicate values will be considered as probabilities corresponding to % two unique values.) The elements of probability vectors P1 and P2 must % each sum to 1 +/- .00001. % % A "log of zero" warning will be thrown for zero-valued probabilities. % Handle this however you wish. Adding 'eps' or some other small value % to all probabilities seems reasonable. (Renormalize if necessary.) % % KLDIV(X,P1,P2,'sym') returns a symmetric variant of the Kullback-Leibler % divergence, given by [KL(P1,P2)+KL(P2,P1)]/2. See Johnson and Sinanovic % (2001). % % KLDIV(X,P1,P2,'js') returns the Jensen-Shannon divergence, given by % [KL(P1,Q)+KL(P2,Q)]/2, where Q = (P1+P2)/2. See the Wikipedia article % for "Kullback-Leibler divergence". This is equal to 1/2 the so-called % "Jeffrey divergence." See Rubner et al. (2000). % % EXAMPLE: Let the event set and probability sets be as follow: % X = [1 2 3 3 4]'; % P1 = ones(5,1)/5; % P2 = [0 0 .5 .2 .3]' + eps; % % Note that the event set here has duplicate values (two 3's). These % will be treated as DISTINCT events by KLDIV. If you want these to % be treated as the SAME event, you will need to collapse their % probabilities together before running KLDIV. One way to do this % is to use UNIQUE to find the set of unique events, and then % iterate over that set, summing probabilities for each instance of % each unique event. Here, we just leave the duplicate values to be % treated independently (the default): % KL = kldiv(X,P1,P2); % KL = % 19.4899 % % Note also that we avoided the log-of-zero warning by adding 'eps' % to all probability values in P2. We didn't need to renormalize % because we're still within the sum-to-one tolerance. % % REFERENCES: % 1) Cover, T.M. and J.A. Thomas. "Elements of Information Theory," Wiley, % 1991. % 2) Johnson, D.H. and S. Sinanovic. "Symmetrizing the Kullback-Leibler % distance." IEEE Transactions on Information Theory (Submitted). % 3) Rubner, Y., Tomasi, C., and Guibas, L. J., 2000. "The Earth Mover's % distance as a metric for image retrieval." International Journal of % Computer Vision, 40(2): 99-121. % 4) Kullback-Leibler divergence. Wikipedia, The Free Encyclopedia. % % See also: MUTUALINFO, ENTROPY if ~isequal(unique(varValue),sort(varValue)) warning('KLDIV:duplicates','X contains duplicate values. Treated as distinct values.') end if ~isequal(size(varValue),size(pVect1)) || ~isequal(size(varValue),size(pVect2)) error('All inputs must have same dimension.') end % Check probabilities sum to 1: if (abs(sum(pVect1) - 1) > .00001) || (abs(sum(pVect2) - 1) > .00001) error('Probablities don''t sum to 1.') end if isempty(varargin) varargin{1} = 'kl'; % 默认计算KL散度 % The logarithms in these formulae are taken to base 2 if information is measured in units of bits, % or to base e if information is measured in nats. % Most formulas involving the Kullback–Leibler divergence hold regardless of the base of the logarithm. varargin{2} = '2'; % 默认以2为对数基底(information is measured in units of bits) end if ~isempty(varargin) switch varargin{2} case '2' switch varargin{1} case 'js' logQvect = log2((pVect2+pVect1)/2); KL = .5 * (sum(pVect1.*(log2(pVect1)-logQvect)) + ... sum(pVect2.*(log2(pVect2)-logQvect))); case 'sym' KL1 = sum(pVect1 .* (log2(pVect1)-log2(pVect2))); KL2 = sum(pVect2 .* (log2(pVect2)-log2(pVect1))); KL = (KL1+KL2)/2; case 'kl' KL = sum(pVect1 .* (log2(pVect1)-log2(pVect2))); otherwise error(['Last argument' ' "' varargin{1} '" ' 'not recognized.']) end case 'e' switch varargin{1} case 'js' logQvect = log((pVect2+pVect1)/2); KL = .5 * (sum(pVect1.*(log(pVect1)-logQvect)) + ... sum(pVect2.*(log(pVect2)-logQvect))); case 'sym' KL1 = sum(pVect1 .* (log(pVect1)-log(pVect2))); KL2 = sum(pVect2 .* (log(pVect2)-log(pVect1))); KL = (KL1+KL2)/2; case 'kl' KL = sum(pVect1 .* (log(pVect1)-log(pVect2))); otherwise error(['Last argument' ' "' varargin{1} '" ' 'not recognized.']) end otherwise error(['Last argument' ' "' varargin{2} '" ' 'not recognized.']) end end
参考:https://www.jianshu.com/p/43318a3dc715