会员
周边
众包
新闻
博问
闪存
赞助商
Chat2DB
所有博客
当前博客
我的博客
我的园子
账号设置
简洁模式
...
退出登录
注册
登录
initial_h
https://github.com/initial-h
博客园
首页
新随笔
管理
上一页
1
2
3
4
5
6
7
8
9
···
12
下一页
2022年5月30日
Data-Efficient Hierarchical Reinforcement Learning
摘要: **发表时间:**2018(NIPS 2018) **文章要点:**这篇文章提出了一个分层强化的算法HIRO: Hierarchical Reinforcement learning with Off-policy correction。主要的点在于不用人工设计具体的上层goal,同时用off-po
阅读全文
posted @ 2022-05-30 23:43 initial_h
阅读(101)
评论(0)
推荐(0)
编辑
2022年5月25日
An Investigation of Model-Free Planning
摘要: **发表时间:**2019(ICML 2019) **文章要点:**这篇文章主要是做实验探讨了一下什么形式算planning。之前的planning通常会设置一个具体的planning算法,比如Monte Carlo rollouts,MCTS等等,或者在网络里面嵌入类似planning的结构,比如
阅读全文
posted @ 2022-05-25 23:32 initial_h
阅读(35)
评论(0)
推荐(0)
编辑
2022年5月23日
A0C: Alpha Zero in Continuous Action Space
摘要: **发表时间:**2018 **文章要点:**这篇文章提出A0C算法,把AlphaZero做到连续动作空间上,主要使用的方式是progressive widening和continuous prior(就是continuous policy network)。具体的,progressive wide
阅读全文
posted @ 2022-05-23 23:05 initial_h
阅读(282)
评论(0)
推荐(0)
编辑
2022年5月20日
Decoupling Exploration and Exploitation for Meta-Reinforcement Learning without Sacrifices
摘要:  **发表时间:**2021(ICML 2021) **文章要点:**这篇文章想说,通常强化学习算法explorat
阅读全文
posted @ 2022-05-20 23:43 initial_h
阅读(77)
评论(0)
推荐(0)
编辑
2022年5月18日
Discovering symbolic policies with deep reinforcement learning
摘要: **发表时间:**2021(ICML 2021) **文章要点:**这篇文章想说神经网络的解释性太差,用简单的符号式子来表示策略具有更好的解释性,而且性能也不错。这里符号式子就是一个简单的函数。作者就提出了一个叫deep symbolic policy的算法来搜索symbolic policies。
阅读全文
posted @ 2022-05-18 23:52 initial_h
阅读(143)
评论(0)
推荐(0)
编辑
2022年5月15日
Revisiting Rainbow: Promoting more Insightful and Inclusive Deep Reinforcement Learning Research
摘要: **发表时间:**2021(ICML 2021) **文章要点:**这篇文章就是在小的环境上重新测试了一遍DQN以及一系列变种的效果,得出的结论就是说即使是在简单任务上进行测试,也能得到有价值的结果,呼吁降低研究RL的算力门槛。具体的,作者先说就算是Atari游戏上做研究,对算力的要求也是巨大的,A
阅读全文
posted @ 2022-05-15 23:07 initial_h
阅读(40)
评论(0)
推荐(0)
编辑
2022年5月13日
Planning to Explore via Self-Supervised World Models
摘要: **发表时间:**2020(ICML 2020) **文章要点:**这篇文章提出了一个Plan2Explore的model based方法,通过self-supervised方法来做Task-agnostic的探索,在这个过程中有效学习了world model,然后可以迁移到下游的具体任务上,实现z
阅读全文
posted @ 2022-05-13 22:54 initial_h
阅读(153)
评论(0)
推荐(0)
编辑
EXPLORATION BY RANDOM NETWORK DISTILLATION
摘要: 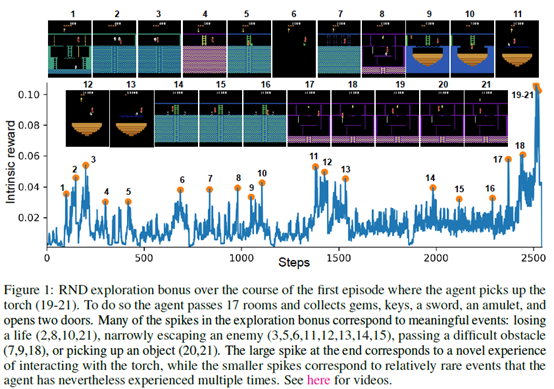 **发表时间:**2018(ICLR 2019) **文章要点:**文章提出了一个random network
阅读全文
posted @ 2022-05-13 22:50 initial_h
阅读(357)
评论(0)
推荐(0)
编辑
2022年5月8日
NEVER GIVE UP: LEARNING DIRECTED EXPLORATION STRATEGIES
摘要: **发表时间:**2020(ICLR 2020) **文章要点:**这篇文章的思路是运用distributed RL的形式,基于intrinsic reward,并行多个agent,将exploration策略和exploitation策略分开单独训练,这样可以设置一族探索程度不同的explorat
阅读全文
posted @ 2022-05-08 23:59 initial_h
阅读(255)
评论(0)
推荐(0)
编辑
2022年5月4日
Discovering and Achieving Goals via World Models
摘要: **发表时间:**2021(NeurIPS 2021) **文章要点:**这篇文章提出Latent Explorer Achiever (LEXA)算法,通过学习world model的imagined rollouts来训练一个explorer策略和一个achiever策略,通过unsupervi
阅读全文
posted @ 2022-05-04 22:23 initial_h
阅读(128)
评论(0)
推荐(0)
编辑
2022年5月2日
Agent57: Outperforming the Atari Human Benchmark
摘要: **发表时间:**2020(ICML) **文章要点:**这篇文章提出Agent57算法,是第一个在所有57个Atari游戏上超过人类表现的算法。主要的思路就是基于Never Give Up (NGU)和R2D2((RECURRENT EXPERIENCE REPLAY IN DISTRIBUTED
阅读全文
posted @ 2022-05-02 23:08 initial_h
阅读(443)
评论(0)
推荐(0)
编辑
2022年4月29日
Efficient Deep Reinforcement Learning via Adaptive Policy Transfer
摘要: **发表时间:**2020(IJCAI 2020) **文章要点:**这篇文章提出Policy Transfer Framework (PTF)算法来做policy transfer。主要思路就是自动去学什么时候用哪一个source policy用来作为target policy的学习目标,以及什么
阅读全文
posted @ 2022-04-29 23:23 initial_h
阅读(176)
评论(0)
推荐(0)
编辑
2022年4月27日
Think Too Fast Nor Too Slow: The Computational Trade-off Between Planning And Reinforcement Learning
摘要: **发表时间:**2020(ICAPS: PRL 2020) **文章要点:**这篇文章主要探究planning和learning的算力的trade-off,得出的结论是既不能planning太多,也不能planning太少。 具体的,作者先指出了一类叫multi-step approximate
阅读全文
posted @ 2022-04-27 23:44 initial_h
阅读(45)
评论(0)
推荐(0)
编辑
Application of MCTS in Atari Black-box Planning
摘要: **发表时间:**2018(ICAPS 2018 workshop Heuristics and Search for Domain-independent Planning (HSDIP)) **文章要点:**这篇文章主要就是做实验看了看几种tree search方法在Atari上的效果如何,里面
阅读全文
posted @ 2022-04-27 23:40 initial_h
阅读(39)
评论(0)
推荐(0)
编辑
Deep Learning for Real-Time Atari Game Play Using Offline Monte-Carlo Tree Search Planning
摘要: **发表时间:**2014(NIPS 2014) **文章要点:**这篇文章主要是测试了Monte-Carlo Tree Search在Atari上的效果,不过并不是结合强化做的,而是先用tree search收集样本,再用神经网络拟合数据训成一个Q网络或者policy网络。得出的结论是比DQN效果
阅读全文
posted @ 2022-04-27 23:34 initial_h
阅读(124)
评论(0)
推荐(0)
编辑
上一页
1
2
3
4
5
6
7
8
9
···
12
下一页
公告