一键Run带你体验扩散模型的魅力
本文分享自华为云社区《爆圈Sora横空出世,AGI通用人工智能时代真的要来了吗?一键Run带你体验扩散模型的魅力!》,作者: 码上开花_Lancer。
Sora这几天的爆炸性新闻,让所有人工智能相关从业者及对应用感兴趣的人群都感到沸腾,震撼到央视也在进行相关的讨论,简直可以和2023年初ChatGPT讨论带来的热潮一般。所以它到底为什么这么火?
一、什么是SORA?
Sora 是OpenAI最新发布的文本生成视频模型,不仅可以生成长达一分钟的视频,且能完全遵照用户的 Prompt 并保持视觉质量。
OpenAI 这个公司的格局非常大,他想要做 World Simulators(世界模拟器),做通用AGI,而不仅仅是文字或者图像视频领域的内容,他希望的是帮助人们解决需要现实世界交互的问题。单从OpenAI 发布的sora模型的论文可以看出来:
图片中文翻译:
视频生成模型作为世界模拟器 我们探讨了在视频数据上对生成模型进行大规模训练。 具体来说,我们共同训练了文本条件扩散模型,这些模型能够处理不同时长、分辨率和宽高比的视频和图像。 我们利用了一种变压器架构,该架构能够处理视频和图像潜在代码的空间时间块。我们最大的模型,Sora,能够生成一分钟的高保真视频。 我们的结果表明,扩展视频生成模型是构建通用物理世界模拟器的有希望的道路。
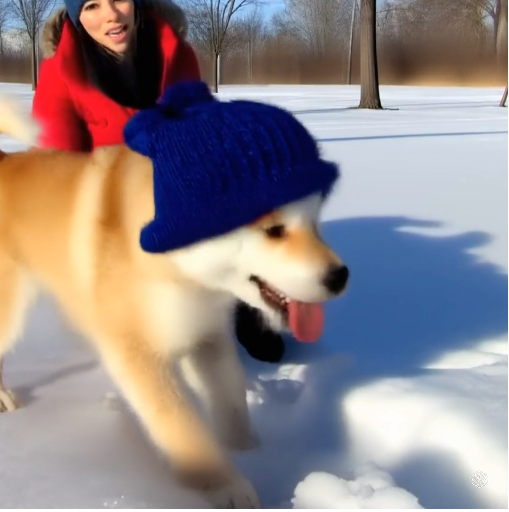
在视频创作领域,画面的稳定性至关重要。如果要呈现出优质的效果,创作者需要具备高超的视频剪辑技能和相关基础。然而,SORA这次的表现真是逆天!通过简单的文字描述,它能生成画面稳定、理解能力强的长视频。
SORA的技术思路与众不同,完全碾压了传统方法。它不再仅关注二维像素的变化,而是专注于语义理解的变化。从以往的视频画面生成,转变为故事逻辑的生成。这种创新思路让人瞠目结舌,展示了技术的无限可能性
二、SORA背后原理的推测
根据OpenAI最新发布的技术报告,Sora背后的“text-to-video”模型基于Diffusion Transformer Model。这种模型结合了Transformer架构和扩散模型,用于生成图像、视频和其他数据。
实际上,Sora是一个基于Transformer的扩散模型。这类模型不仅在理论上具有创新性,而且在实际应用中也显示出了强大的潜力。例如,DiT模型(Sora的基础)和GenTron模型在图像和视频生成等领域都已经取得了巨大的成功,这些创新性的模型为我们展示了技术的无限可能性。目前Sora技术没有公开,大家对它都有不同猜测。DIT提出人谢赛宁:
1)Sora应该是建立在DiT这个扩散Transformer之上的 。
2)Sora可能有大约30亿个参数,(引用论文模型0.13B, 32X算力)。
3)训练数据是Sora 成功的最关键因素。
4)主要的挑战是如何解决错误累积问题并随着时间的推移保持质量/一致 。
DiT模型:Meta提出的完全基于transformer架构的扩散模型,不仅将transformer成功应用在扩散模型,还探究了transformer架构在扩散模型上的scalability能力。
GenTron模型:一种基于Transformer的扩散模型,在针对SDXL的人类评估中,GenTron在视觉质量方面取得了51.1%的胜率(19.8%的平局率),在文本对齐方面取得了42.3%的胜率(42.9%的平局率)。
DiT模型Scalable Diffusion Models with Transformers ---- 基于transformer的扩散模型,称为Diffusion Transformers(DiTs) ,Diffusion Transformer Model(DiT)的设计空间、扩展行为、网络复杂度和样本质量之间的关系。这些研究结果表明,通过简单地扩展DiT并使用高容量的骨干网络,可以在类条件256x256 ImageNet生成基准测试中实现最新的2.27 FID。与像素空间扩散模型相比,DiTs在使用的Gflops只是其一小部分,因此具有较高的计算效率。此外,DiTs还可以应用于像素空间,使得图像生成流程成为混合方法,使用现成的卷积VAEs和基于transformer的DDPMs。
扩散模型中引入了transformer类的标准设计,以取代传统的U-Net设计,从而提供了一种新的架构选择。
引入了潜在扩散模型(LDMs),通过将图像压缩为较小的空间表示,并在这些表示上训练扩散模型,从而解决了在高分辨率像素空间中直接训练扩散模型的计算问题。
那对于我们开发者用户想要强烈体验文生视频的乐趣,那里可以体验呢?今天给大家介绍下Stable Video Diffusion (SVD),一起在华为云一键Run体验其中的乐趣:
三、Stable Video Diffusion (SVD) 扩散模型的图像生成视频的体验
1. 案例简介
Stable Video Diffusion (SVD) 是一种扩散模型,它将静止图像作为条件帧,并从中生成视频。
🔹 本案例需使用 Pytorch-1.8 GPU-V100 及以上规格运行
🔹 点击Run in ModelArts,将会进入到ModelArts CodeLab中,这时需要你登录华为云账号,如果没有账号,则需要注册一个,且要进行实名认证,参考《ModelArts准备工作_简易版》 即可完成账号注册和实名认证。 登录之后,等待片刻,即可进入到CodeLab的运行环境
🔹 出现 Out Of Memory ,请检查是否为您的参数配置过高导致,修改参数配置,重启kernel或更换更高规格资源进行规避❗❗❗
2. 下载代码和模型
!git clone https://github.com/Stability-AI/generative-models.git
Cloning into 'generative-models'... remote: Enumerating objects: 860, done.•[K remote: Counting objects: 100% (489/489), done.•[K remote: Compressing objects: 100% (222/222), done.•[K remote: Total 860 (delta 368), reused 267 (delta 267), pack-reused 371•[K Receiving objects: 100% (860/860), 42.67 MiB | 462.00 KiB/s, done. Resolving deltas: 100% (445/445), done.
import moxing as mox mox.file.copy_parallel('obs://modelarts-labs-bj4-v2/case_zoo/Stable_Video_Diffusion/file/modify_file/generative-models/sgm/modules/encoders','generative-models/sgm/modules/encoders') mox.file.copy_parallel('obs://modelarts-labs-bj4-v2/case_zoo/Stable_Video_Diffusion/file/models','generative-models/models') mox.file.copy_parallel(,'obs://modelarts-labs-bj4-v2/case_zoo/Stable_Video_Diffusion/file/checkpoints','generative-models/checkpoints')
INFO:root:Using MoXing-v2.1.0.5d9c87c8-5d9c87c8 INFO:root:Using OBS-Python-SDK-3.20.9.1
3. 配置运行环境
本案例依赖Python3.10.10及以上环境,因此我们首先创建虚拟环境:
!/home/ma-user/anaconda3/bin/conda create -n python-3.10.10 python=3.10.10 -y --override-channels --channel https://mirrors.tuna.tsinghua.edu.cn/anaconda/pkgs/main !/home/ma-user/anaconda3/envs/python-3.10.10/bin/pip install ipykernel
/home/ma-user/anaconda3/lib/python3.7/site-packages/requests/__init__.py:91: RequestsDependencyWarning: urllib3 (1.26.12) or chardet (3.0.4) doesn't match a supported version! RequestsDependencyWarning) Collecting package metadata (current_repodata.json): done Solving environment: failed with repodata from current_repodata.json, will retry with next repodata source. Collecting package metadata (repodata.json): done Solving environment: done
import json import os data = { "display_name": "python-3.10.10", "env": { "PATH": "/home/ma-user/anaconda3/envs/python-3.10.10/bin:/home/ma-user/anaconda3/envs/python-3.7.10/bin:/modelarts/authoring/notebook-conda/bin:/opt/conda/bin:/usr/local/nvidia/bin:/usr/local/cuda/bin:/usr/local/sbin:/usr/local/bin:/usr/sbin:/usr/bin:/sbin:/bin:/home/ma-user/modelarts/ma-cli/bin:/home/ma-user/modelarts/ma-cli/bin:/home/ma-user/anaconda3/envs/PyTorch-1.8/bin" }, "language": "python", "argv": [ "/home/ma-user/anaconda3/envs/python-3.10.10/bin/python", "-m", "ipykernel", "-f", "{connection_file}" ] } if not os.path.exists("/home/ma-user/anaconda3/share/jupyter/kernels/python-3.10.10/"): os.mkdir("/home/ma-user/anaconda3/share/jupyter/kernels/python-3.10.10/") with open('/home/ma-user/anaconda3/share/jupyter/kernels/python-3.10.10/kernel.json', 'w') as f: json.dump(data, f, indent=4)
创建完成后,稍等片刻,或刷新页面,点击右上角kernel选择python-3.10.10
!pip install torch==2.0.1 torchvision==0.15.2 !pip install MoviePy
Looking in indexes: http://repo.myhuaweicloud.com/repository/pypi/simple Collecting torch==2.0.1 Downloading http://repo.myhuaweicloud.com/repository/pypi/packages/8c/4d/17e07377c9c3d1a0c4eb3fde1c7c16b5a0ce6133ddbabc08ceef6b7f2645/torch-2.0.1-cp310-cp310-manylinux1_x86_64.whl (619.9 MB) •[2K •[90m━━━━━━━━━━━━━━━━━━━━━━━━━━━━━━━━━━━━━━━•[0m •[32m619.9/619.9 MB•[0m •[31m5.6 MB/s•[0m eta •[36m0:00:00•[0m00:01•[0m00:01•[0m ...... Uninstalling decorator-5.1.1: Successfully uninstalled decorator-5.1.1 Successfully installed MoviePy-1.0.3 decorator-4.4.2 imageio-2.34.0 imageio_ffmpeg-0.4.9 proglog-0.1.10 tqdm-4.66.2
%cd generative-models
/home/ma-user/work/stable-video-diffusion/generative-models
/home/ma-user/anaconda3/envs/python-3.10.10/lib/python3.10/site-packages/IPython/core/magics/osm.py:417: UserWarning: using dhist requires you to install the `pickleshare` library. self.shell.db['dhist'] = compress_dhist(dhist)[-100:]
!pip install -r requirements/pt2.txt
Looking in indexes: http://repo.myhuaweicloud.com/repository/pypi/simple Collecting clip@ git+https://github.com/openai/CLIP.git (from -r requirements/pt2.txt (line 3)) Cloning https://github.com/openai/CLIP.git to /tmp/pip-install-_vzv4vq_/clip_4273bc4d2cba4d6486a222a5093fbe4b conda3/envs/python-3.10.10/lib/python3.10/site-packages (from -r requirements/pt2.txt (line 32)) (4.66.2) Collecting transformers==4.19.1 (from -r requirements/pt2.txt (line 33)) Successfully uninstalled urllib3-2.2.1 Successfully installed PyWavelets-1.5.0 aiohttp-3.9.3 aiosignal-1.3.1 altair-5.2.0 antlr4-python3-runtime-4.9.3 appdirs-1.4.4 async-timeout-4.0.3 attrs-23.2.0 black-23.7.0 blinker-1.7.0 braceexpand-0.1.7 cachetools-5.3.2 chardet-5.1.0 click-8.1.7 clip-1.0 contourpy-1.2.0 cycler-0.12.1 docker-pycreds-0.4.0 einops-0.7.0 fairscale-0.4.13 fire-0.5.0 fonttools-4.49.0 frozenlist-1.4.1 fsspec-2024.2.0 ftfy-6.1.3 gitdb-4.0.11 gitpython-3.1.42 huggingface-hub-0.20.3 importlib-metadata-7.0.1 invisible-watermark-0.2.0 jsonschema-4.21.1 jsonschema-specifications-2023.12.1 kiwisolver-1.4.5 kornia-0.6.9 lightning-utilities-0.10.1 markdown-it-py-3.0.0 matplotlib-3.8.3 mdurl-0.1.2 multidict-6.0.5 mypy-extensions-1.0.0 natsort-8.4.0 ninja-1.11.1.1 omegaconf-2.3.0 open-clip-torch-2.24.0 opencv-python-4.6.0.66 pandas-2.2.0 pathspec-0.12.1 protobuf-3.20.3 pudb-2024.1 pyarrow-15.0.0 pydeck-0.8.1b0 pyparsing-3.1.1 pytorch-lightning-2.0.1 pytz-2024.1 pyyaml-6.0.1 referencing-0.33.0 regex-2023.12.25 rich-13.7.0 rpds-py-0.18.0 safetensors-0.4.2 scipy-1.12.0 sentencepiece-0.2.0 sentry-sdk-1.40.5 setproctitle-1.3.3 smmap-5.0.1 streamlit-1.31.1 streamlit-keyup-0.2.0 tenacity-8.2.3 tensorboardx-2.6 termcolor-2.4.0 timm-0.9.16 tokenizers-0.12.1 toml-0.10.2 tomli-2.0.1 toolz-0.12.1 torchaudio-2.0.2 torchdata-0.6.1 torchmetrics-1.3.1 transformers-4.19.1 tzdata-2024.1 tzlocal-5.2 urllib3-1.26.18 urwid-2.6.4 urwid-readline-0.13 validators-0.22.0 wandb-0.16.3 watchdog-4.0.0 webdataset-0.2.86 xformers-0.0.22 yarl-1.9.4 zipp-3.17.0
!pip install .
Looking in indexes: http://repo.myhuaweicloud.com/repository/pypi/simple Processing /home/ma-user/work/stable-video-diffusion/generative-models Installing build dependencies ... •[?25ldone •[?25h Getting requirements to build wheel ... •[?25ldone •[?25h Preparing metadata (pyproject.toml) ... •[?25ldone •[?25hBuilding wheels for collected packages: sgm Building wheel for sgm (pyproject.toml) ... •[?25ldone •[?25h Created wheel for sgm: filename=sgm-0.1.0-py3-none-any.whl size=127368 sha256=0f9ff6913b03b2e0354cd1962ecb2fc03df36dea90d14b27dc46620e6eafc9a0 Stored in directory: /home/ma-user/.cache/pip/wheels/a9/b8/f4/e84140beaf1762b37f5268788964d58d91394ee17de04b3f9a Successfully built sgm Installing collected packages: sgm Successfully installed sgm-0.1.0
4. 生成视频
视频默认生成到outputs文件夹内
!python scripts/sampling/simple_video_sample.py --decoding_t 1 --input_path 'assets/test_image.png'
/home/ma-user/work/stable-video-diffusion/generative-models VideoTransformerBlock is using checkpointing VideoTransformerBlock is using checkpointing VideoTransformerBlock is using checkpointing VideoTransformerBlock is using checkpointing VideoTransformerBlock is using checkpointing VideoTransformerBlock is using checkpointing VideoTransformerBlock is using checkpointing VideoTransformerBlock is using checkpointing VideoTransformerBlock is using checkpointing VideoTransformerBlock is using checkpointing VideoTransformerBlock is using checkpointing VideoTransformerBlock is using checkpointing VideoTransformerBlock is using checkpointing VideoTransformerBlock is using checkpointing VideoTransformerBlock is using checkpointing VideoTransformerBlock is using checkpointing Initialized embedder #0: FrozenOpenCLIPImagePredictionEmbedder with 683800065 params. Trainable: False Initialized embedder #1: ConcatTimestepEmbedderND with 0 params. Trainable: False Initialized embedder #2: ConcatTimestepEmbedderND with 0 params. Trainable: False Initialized embedder #3: VideoPredictionEmbedderWithEncoder with 83653863 params. Trainable: False Initialized embedder #4: ConcatTimestepEmbedderND with 0 params. Trainable: False Restored from checkpoints/svd.safetensors with 0 missing and 0 unexpected keys 100%|███████████████████████████████████████| 890M/890M [00:50<00:00, 18.5MiB/s] /home/ma-user/anaconda3/envs/python-3.10.10/lib/python3.10/site-packages/torch/utils/checkpoint.py:31: UserWarning: None of the inputs have requires_grad=True. Gradients will be None warnings.warn("None of the inputs have requires_grad=True. Gradients will be None")
#将视频文件转成动图显示 from moviepy.editor import * # 指定输入视频路径 video_path = "outputs/simple_video_sample/svd/000000.mp4" # 加载视频 clip = VideoFileClip(video_path) # 设置保存GIF的参数(如分辨率、持续时间等) output_file = "output_animation.gif" fps = 10 # GIF每秒显示的帧数 # 生成并保存GIF clip.write_gif(output_file, fps=fps)
MoviePy - Building file output_animation.gif with imageio.
from IPython.display import Image Image(open('output_animation.gif','rb').read())
大家赶紧来体验文生视频的乐趣吧!