【ARXIV2203】SepViT: Separable Vision Transformer
1、Motivation
作者指出当前视觉Transformer 模型中的痛点在于:huge resource demands。为了解决这个问题,作者提出了 Separable Vision Transformer (SepViT),整体架构如下图所示。
包括下面三个贡献:
- Depthwise separable self-attention. It can achieve local information communication within the windows and global informaiton exchange among the windows in a single Transformer block.
- Window token embedding. Helps to model the attention relationship among windows with negligible computational cost.
2、Depthwise separable self-attention
和 MobileNet 提出的 深度可分离卷积非常类似,包括 Depthwise Self-Attention (DWA) 和 Pointwise Self-Attention (PWA) 两个步骤。一个是逐层计算 attention,一个是逐点计算 attention。
DWA如下图所示,可以看出 attention 是在各个层里计算的,非常简单。但是,如果逐像素计算的话,会出现计算复杂度过高的问题。因此,作者使用了 window token embedding。如图中所示,输入特征是 6x6xC,拆分为2x2=4个window,首先构建windows token 大小为 4xCx1。四个windows 的大小为 4xCx9。把两个特征拼接为 4xCx10,然后在四个 window 里分别计算注意力,最终结果大小为 4xCx10 (包括了新的 winodw 特征和 window token)。
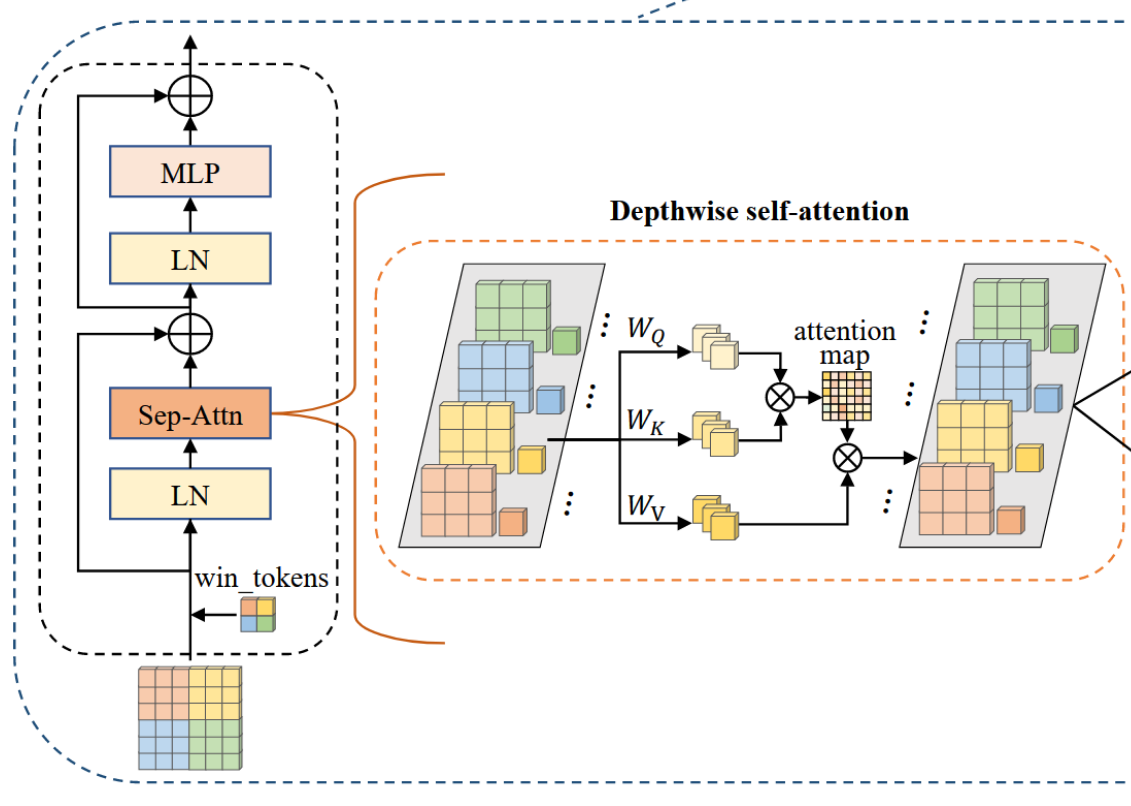
PWA的计算也很有趣,把新的 window token 拿出来进行相似性计算,得到 4x4 的权重矩阵,利用该权重矩阵对四个 window 的特征进行加权,最后得到输出特征。
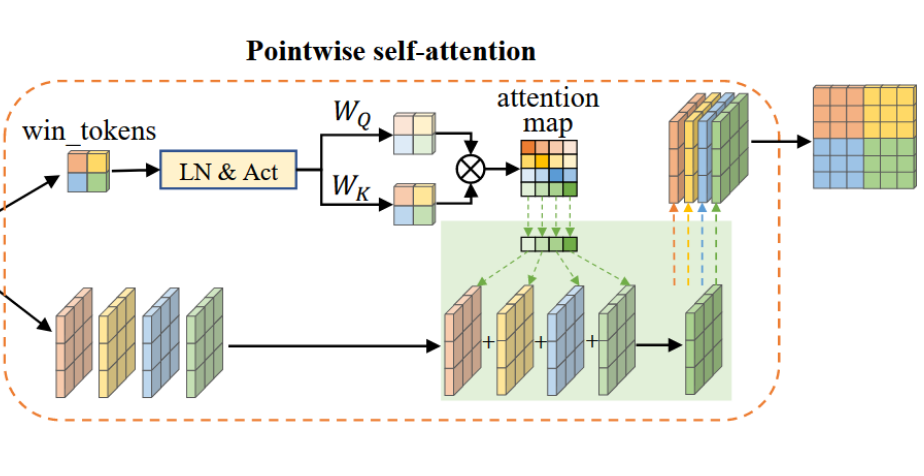
3、Grouped Self-Attention
作者利用组卷积对深度可分离Self-Attention进行了扩展,并提出了分组Self-Attention。如下图所示,将相邻的子Window拼接,形成更大的Window,类似于将Window分成组,在一组Window内进行深度的Self-Attention通信。通过这种方式,Grouped Self-Attention可以捕获多个Window的长期视觉依赖关系。在计算成本和性能增益方面,Grouped Self-Attention比深度可分离Self-Attention具有一定的额外成本,但也具有更好的性能。
实验部分可以参照作者的论文,这里不再过多介绍。