大模型与KG(一)——大模型的前世今生(发展脉络与基本知识扫盲)
已经好久好久好久没有写博客了,快一年了,学术进展在最近的一年也接近停滞。因为选择职业/人生方向花费了很多的时间,在新环境中心安定下来才决定继续走学术研究的道路。最近的整体状态还算不错的,在各方面都算顺利,因为逐渐从内心接纳了自己,无论是优秀还是菜。在读研的时候看到Bert之类的词汇就本能地抗拒,对于学习和学术自始至终无法正视,所以也计划去了解一下这个东西,看看是什么样子,克服长久以来的恐惧和抗拒。从做一些副业的过程中,就感觉到自己并非讨厌研究问题、debug、只是不认可、不接受自己的方式和节奏,但是根本无需去管别人此时跑的有多快、做得有多好,不去刻意关注差距、不去习惯性否定自己,接纳自己达到目标的方式,在自己的世界里,按自己的想法来,最终会发现,自己做得也还不错,已经远远超过了自己最初顶下的目标。
最近看大模型的论文,感觉效率不是很高,其实很多东西还是应该放一放的,避免对着论文无意识走神(在看自己看不懂、不感兴趣的东西时,是很容易走神的)。一篇论文,只要抓住最核心的一个点就够了。需要用到的时候再细读。在实践过程中永远是最快、效率最高的,这也是我为什么而今不抗拒做工程、不认为是做学术的阻拦的原因。而今,无论是对NLP、KG等AI,还是无安全的一些东西,都还是抱有兴趣的,愿意去学习和了解。后面的方向是打算做时序知识图谱的,也打算用语言模型来做,符合一下工程需求和蹭蹭热度。从上上周就开始找资料,大概理出来三块需要先了解的背景知识:大模型的基础知识和发展脉络、大模型做图尤其是KG的现状(据了解,主要是Transformer做的),以及时序图现状。核心和目标是时序图,手段可能会用到大模型,应用可以尝试网安的实时攻击研判什么的。接下来的博客也分三篇介绍这三块的内容。
大模型最初的起源就是神经语言模型的 word embedding,后来到 Transformer、Bert,然后就是 GPT,到现在的 GPT4,一个 GPT 火了,各单位纷纷跟进,到现在的啥啥都大模型,确实有炒作的成分。昨天下午的大模型安全讨论,也印证了我之前的感觉,大模型其实并没有那么神,只不过是把很多基础功能集成、意图理解准确、信息检索的质量提高、并以问答形式呈现的结果。通用的对话,还有在信息收集的效率方面可以提供很大的辅助作用、节省人力的。但终究还是一个辅助工具,给出的返回信息也只是人类提升认知和决策时的参考。
一些基础问题
大模型的叫法已经满天飞了,在真正进行调研了解之前,有很多疑问,慢慢了解中进行解答。先找的综述,刚好今年人民大学高瓴人工智能研究院出了一个非常详细的大模型综述:http://ai.ruc.edu.cn/research/science/20230605100.html ,名字就叫《A Survey of Large Language Models》论文在arxiv和github上都有,把模型列的还是比较全的。而我自己的学习,主要是挑几个比较常见的标志性模型,大概了解一下这个发展过程。
-
大模型的“大”如何界定?
大模型最初不叫“大模型”,叫预训练语言模型。ChatGPT火了之后,大家都通俗地叫起了“大模型”。这个分界线,大概是出现在Bert前后。综述里面给出的定义是:参数超过100亿,就视为大模型。 -
常说的大模型有哪些?
大模型以GPT为代表,很多单位都发布自己的大模型,有很多,但最有名的还是OpenAI的GPT。 -
大模型的发展脉络?
按照人大的综述,语言模型的发展分为四个阶段:统计语言模型,就是n-gram;神经语言模型,就是word2vec;预训练语言模型PLM(Pretrained Language Model),GloVe、ELMo、Transformer、Bert;发展到现在就是LLM(Large Language Model),以GPT为代表。 -
大模型有没有做图序列预测的?
这块后面会专门调研一下。目前的感觉,只有同质图,做异质图的不多。浙大陈华钧老师团队有用Transformer做KGE,不知道有没有用别的大模型做的。目前也没有发现有做图结构序列预测的(关键词:图演化),后面也会再调研一下。 -
大模型做知识图谱的?
主要是浙大的工作:Relphormer: Relational Graph Transformer for Knowledge Graph Representations、KGTransformer: Structure Pretraining and Prompt Tuning for Knowledge Graph Transfer 等。浙大做知识图谱还是做得非常好的。 -
大模型做KG可尝试哪些模型?
打算还是先尝试Transformer,bertKG之前尝试过还是比较慢的、GPT什么的也不好尝试。Transformer和Bert是开源的,GPT1和2应该也是,3和4就不开源了。因为是个人研究,所以还是先尝试Transformer,Bert之后的大模型对算力还是要求比较高的,一般是公司和团队做,个人还是有难度。要选对算力要求低的、开源的。 -
经典模型的原理结构、和代码?
对经典模型按照实践顺序进行了依次阅读,Attention-->Transformer-->Bert-->GPT1-->GPT2-->GPT3-->GPT4,下面对各个模型的关键创新点和核心代码进行介绍。
大模型的基本构建是Transformer,Transformer的创新点是注意力机制。后面Bert、GPT等模型,都是基于Transformer来的,不过是把模型参数量和数据量加大了而已,所以了解注意力机制和Transformer是基础。
注意力机制
看了B站上解惑者的视频:https://www.bilibili.com/video/BV1Tt411V7WE/
处理语言关键的是处理词语序列,在Attention出现之前,是用LSTM、RNN这些带能处理前后信息的神经网络去单向地处理句子中的词语序列,但是Attention机制出现的效果远超过LSTM等序列网络,所以之后就是Attention的天下了。
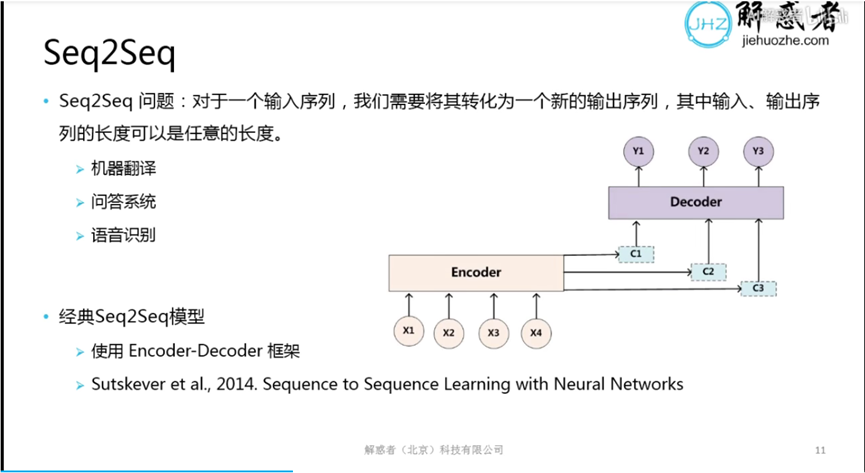
Attention机制之前一直不明白,看到那个QKV的公式就发怵,但是核心思想就是一个赋权重的操作:重要的/相关的东西,给大的权重,不重要/不相关的,给小的权重。对序列加权聚合,得到输出的表示。这个idea吧,最初看到的时候,感觉平平无奇,像是聚了个寂寞,因为给了好听的名字,听上去还觉得很复杂。但是本质似乎并没有太复杂。今天想一想这个思想之所以好,可能是因为,“把钱用在了刀刃上”,关注重要的信息,忽略不重要的信息,也是人类认知时很重要的一点。但这只是猜想和感性的认识。
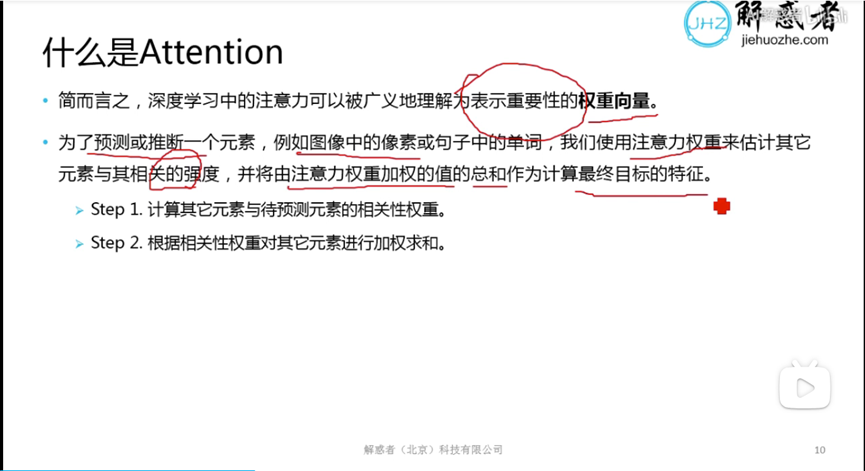
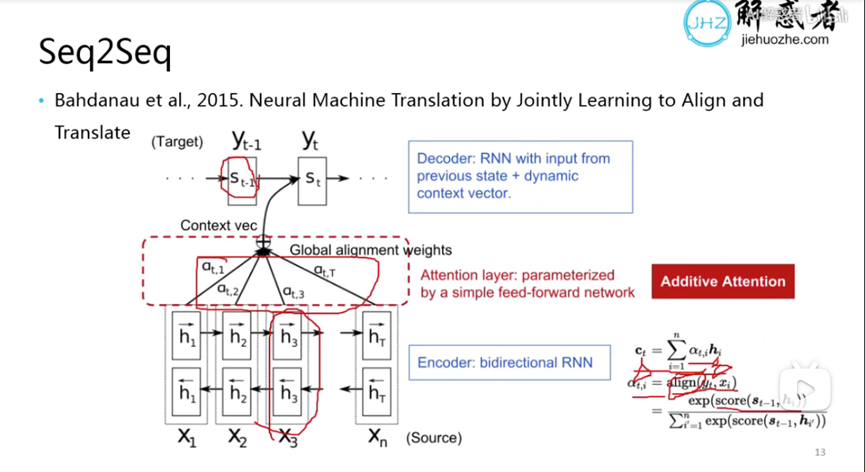
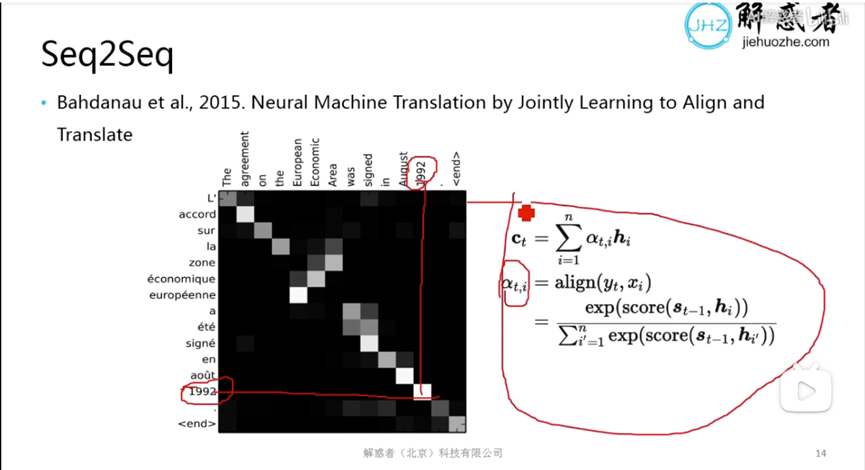
Attention 其实就是seq2seq机器翻译中,对相关的词给比较大的权重。
代码实现:
import torch
import torch.nn as nn
class Attention(nn.Module):
def __init__(self, query_dim, key_dim, value_dim):
super(Attention, self).__init__()
self.query_dim = query_dim
self.key_dim = key_dim
self.value_dim = value_dim
self.query_transform = nn.Linear(query_dim, query_dim)
self.key_transform = nn.Linear(key_dim, query_dim)
self.value_transform = nn.Linear(value_dim, value_dim)
self.softmax = nn.Softmax(dim=-1)
def forward(self, query, key, value):
query = self.query_transform(query)
key = self.key_transform(key)
value = self.value_transform(value)
scores = torch.matmul(query, key.transpose(-2, -1))
attention_weights = self.softmax(scores)
attended_values = torch.matmul(attention_weights, value)
return attended_values, attention_weights
这个Attention类接受三个输入:query(查询向量)、key(键向量)和value(值向量)。在前向传播过程中,通过线性变换将query、key和value映射到指定维度。然后,计算query和key之间的点积得分,应用softmax函数得到注意力权重。最后,通过权重对value进行加权求和,得到经过注意力机制后的向量attended_values。
Transformer
看的上个视频的第二集:https://www.bilibili.com/video/BV13t411j7PM/
Transformer 是2017年google提出来的,最早提出self-attention机制,论文名称就是《attention is all you need》。其架构图很经典,乍一看上去非常复杂,但是有一些NN基础再来看的话,就知道没什么,都是简单的东西重复堆叠,让它看起来复杂了而已。
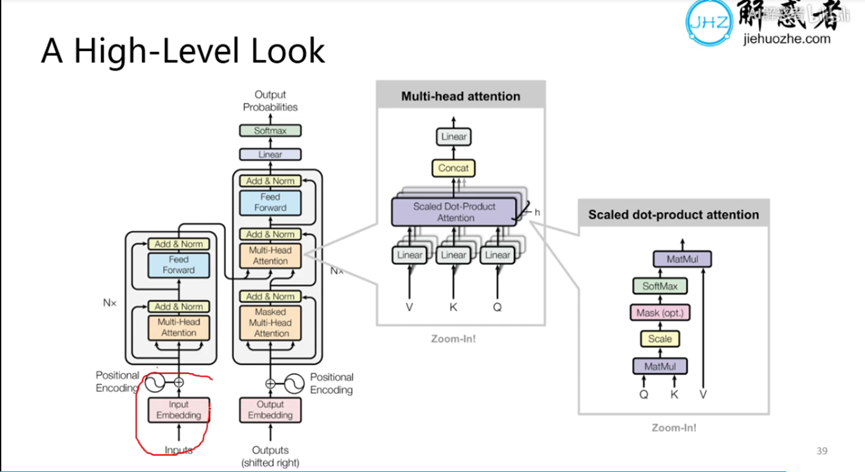
这个图很好,把Transformer的三个图的逻辑关系展示清楚。其实就分为两部分,编码器和解码器。编码器对进来的embedding(比如词的embedding)加一个位置编码(正弦或者余弦),然后过一个多头注意力机制(多头就是多套),然后与残差连接,进行归一化,然后过一个前馈,再加上残差、进行归一化。上面这些重复N次,编码就完成了。右侧是解码的部分,我认为这里输进去的output在训练阶段应该算是标签,也先加一个位置编码(讲真位置编码有些鸡肋,不过是为了让各个词语有个位置标识),然后经过Masked多头注意力、残差和归一化,然后接下来要经过的这个注意力是“编码器-解码器”之间的相互注意力,前面提到的编解码器内部的都是self-attention。self-attention就是句子中的每个词和其他词之间的相关性,之间的注意力的Q和K来源于编码器、V来源于解码器。经过编解码器互注意力之后,再过前馈、然后是残差和归一化,然后再过一个线性层,最后输出softmax的概率。这一套也是要重复N次,形成整个解码器的结构。
对于注意力,就是缩放点积(Scaled Dot-Product),其实就是对点积(原本的相似度)进行了一个\(\sqrt{嵌入维度}\)的缩放。多套注意力输出的结果进行一个拼接。最右边的小图展示了缩放点积是怎么计算的,Q和K的缩放点积算出来softmax作为对每个V的权重,然后对V进行加权求和之后,得到缩放点积的结果。
其实原理不算特别复杂,就是叫了好听的名字,什么Q、K、V、attention、multi-head什么的,就显得很复杂很牛的样子hhh
官方给出的代码内容很多很复杂,没找到核心的,以下是ChatGPT给出的:
import torch
import torch.nn as nn
import torch.nn.functional as F
class SelfAttention(nn.Module):
def __init__(self, input_dim, hidden_dim):
super(SelfAttention, self).__init__()
self.WQ = nn.Linear(input_dim, hidden_dim)
self.WK = nn.Linear(input_dim, hidden_dim)
self.WV = nn.Linear(input_dim, hidden_dim)
self.WO = nn.Linear(hidden_dim, input_dim)
def forward(self, x):
q = self.WQ(x)
k = self.WK(x)
v = self.WV(x)
scores = torch.matmul(q, k.transpose(-2, -1)) / torch.sqrt(torch.tensor(q.size(-1), dtype=torch.float32))
attention_weights = F.softmax(scores, dim=-1)
attended = torch.matmul(attention_weights, v)
output = self.WO(attended)
return output
class FeedForward(nn.Module):
def __init__(self, hidden_dim):
super(FeedForward, self).__init__()
self.linear1 = nn.Linear(hidden_dim, hidden_dim)
self.linear2 = nn.Linear(hidden_dim, hidden_dim)
def forward(self, x):
hidden = self.linear1(x)
hidden = F.relu(hidden)
output = self.linear2(hidden)
return output
class Transformer(nn.Module):
def __init__(self, input_dim, hidden_dim, num_heads, num_layers):
super(Transformer, self).__init__()
self.attention_layers = nn.ModuleList([SelfAttention(input_dim, hidden_dim) for _ in range(num_layers)])
self.ffn_layers = nn.ModuleList([FeedForward(hidden_dim) for _ in range(num_layers)])
def forward(self, x):
output = x
for attention_layer, ffn_layer in zip(self.attention_layers, self.ffn_layers):
output = attention_layer(output)
output = ffn_layer(output)
return output
# Example usage
input_dim = 512
hidden_dim = 256
num_heads = 4
num_layers = 6
transformer = Transformer(input_dim, hidden_dim, num_heads, num_layers)
input_tensor = torch.randn(10, input_dim) # Example input tensor of shape (batch_size, input_dim)
output_tensor = transformer(input_tensor)
print(output_tensor.shape)
这个示例代码使用PyTorch库实现了Transformer模型,包括自注意力机制和前馈神经网络。SelfAttention类定义了自注意力层,FeedForward类定义了前馈神经网络层,Transformer类整合了这两个组件。在forward方法中,我们逐层应用自注意力和前馈神经网络操作。
Bert
Bert全称是Bidirectional Encoder Representations from Transformers,2019谷歌提出来的,论文名称是:BERT: Pre-training of Deep Bidirectional Transformers for Language Understanding。论文中没有画出Bert架构的细节,只说了是多层双向Transformer编码器。Base版本的Bert堆叠了12个Transformer。核心创新点就是双向、预训练,加掩码。
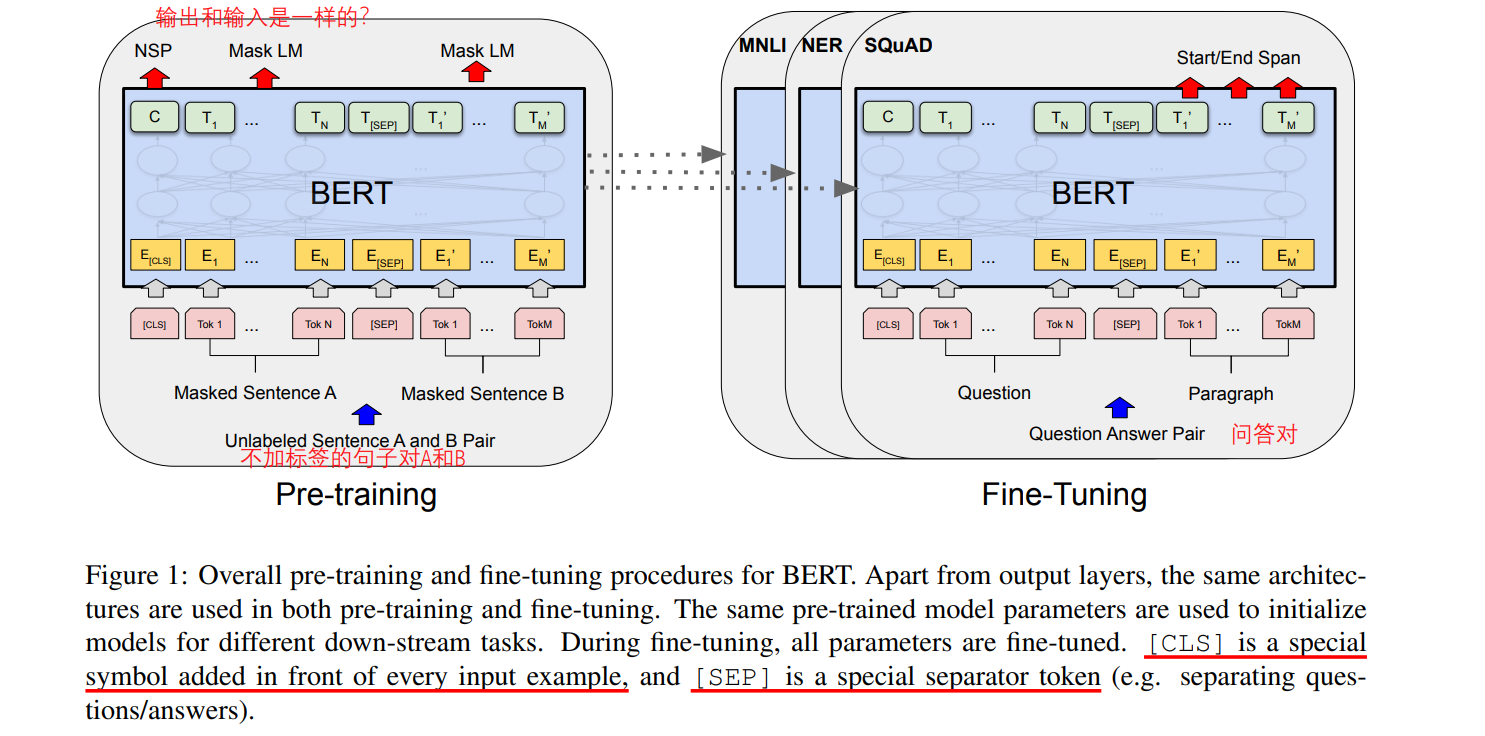
Bert的预训练+微调的方式,奠定了后面模型的发展方向。预训练可能会用单个句子,或者句子对。[cls]是句子开头标识句子所属类别的标识,[sep]用于标识两个句子的分隔。输入的embedding由三个部分相加而来:token embedding、所属句子标识、以及位置编码。
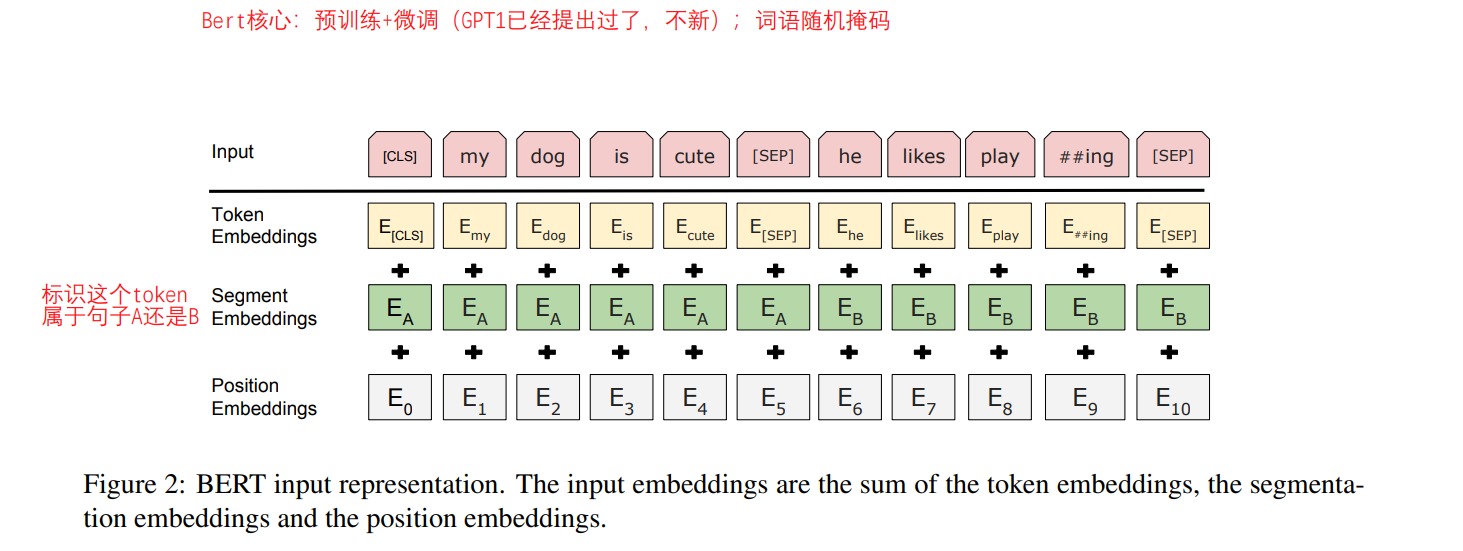
后面就是在各类NLP任务上的实验。
ChatGPT给出的Bert实现
import torch
import torch.nn as nn
import torch.nn.functional as F
class BertEmbedding(nn.Module):
def __init__(self, vocab_size, embedding_dim):
super(BertEmbedding, self).__init__()
self.token_embeddings = nn.Embedding(vocab_size, embedding_dim)
self.position_embeddings = nn.Embedding(max_position_embeddings, embedding_dim)
self.segment_embeddings = nn.Embedding(num_segments, embedding_dim)
def forward(self, input_ids, segment_ids):
token_embeds = self.token_embeddings(input_ids)
position_embeds = self.position_embeddings(torch.arange(input_ids.size(1)).unsqueeze(0).to(input_ids.device))
segment_embeds = self.segment_embeddings(segment_ids)
embeddings = token_embeds + position_embeds + segment_embeds
return embeddings
class BertEncoder(nn.Module):
def __init__(self, hidden_size, num_attention_heads, intermediate_size, hidden_dropout_prob):
super(BertEncoder, self).__init__()
self.attention = BertAttention(hidden_size, num_attention_heads)
self.intermediate = BertIntermediate(hidden_size, intermediate_size)
self.output = BertOutput(hidden_size)
self.dropout = nn.Dropout(hidden_dropout_prob)
def forward(self, hidden_states, attention_mask):
attention_output = self.attention(hidden_states, attention_mask)
intermediate_output = self.intermediate(attention_output)
intermediate_output = self.dropout(intermediate_output)
layer_output = self.output(intermediate_output, attention_output)
return layer_output
class BertAttention(nn.Module):
def __init__(self, hidden_size, num_attention_heads):
super(BertAttention, self).__init__()
self.self = BertSelfAttention(hidden_size, num_attention_heads)
self.output = BertSelfOutput(hidden_size)
def forward(self, hidden_states, attention_mask):
self_output = self.self(hidden_states, attention_mask)
attention_output = self.output(self_output, hidden_states)
return attention_output
class BertSelfAttention(nn.Module):
def __init__(self, hidden_size, num_attention_heads):
super(BertSelfAttention, self).__init__()
self.hidden_size = hidden_size
self.num_attention_heads = num_attention_heads
self.query = nn.Linear(hidden_size, hidden_size)
self.key = nn.Linear(hidden_size, hidden_size)
self.value = nn.Linear(hidden_size, hidden_size)
self.dropout = nn.Dropout(attention_probs_dropout_prob)
def forward(self, hidden_states, attention_mask):
mixed_query_layer = self.query(hidden_states)
mixed_key_layer = self.key(hidden_states)
mixed_value_layer = self.value(hidden_states)
query_layer = self.split_heads(mixed_query_layer)
key_layer = self.split_heads(mixed_key_layer)
value_layer = self.split_heads(mixed_value_layer)
attention_scores = torch.matmul(query_layer, key_layer.transpose(-1, -2))
attention_scores = attention_scores / math.sqrt(self.hidden_size)
attention_scores = attention_scores + attention_mask
attention_probs = nn.Softmax(dim=-1)(attention_scores)
attention_probs = self.dropout(attention_probs)
context_layer = torch.matmul(attention_probs, value_layer)
context_layer = self.combine_heads(context_layer)
return context_layer
class BertIntermediate(nn.Module):
def __init__(self, hidden_size, intermediate_size):
super(BertIntermediate, self).__init__()
self.dense = nn.Linear(hidden_size, intermediate_size)
self.intermediate_act_fn = nn.ReLU()
def forward(self, hidden_states):
intermediate_output = self.dense(hidden_states)
intermediate_output = self.intermediate_act_fn(intermediate_output)
return intermediate_output
class BertOutput(nn.Module):
def __init__(self, hidden_size):
super(BertOutput, self).__init__()
self.dense = nn.Linear(hidden_size, hidden_size)
self.LayerNorm = BertLayerNorm(hidden_size, eps=1e-12)
self.dropout = nn.Dropout(hidden_dropout_prob)
def forward(self, hidden_states, input_tensor):
hidden_states = self.dense(hidden_states)
hidden_states = self.dropout(hidden_states)
hidden_states = self.LayerNorm(hidden_states + input_tensor)
return hidden_states
# Example usage
vocab_size = 10000
embedding_dim = 768
hidden_size = 768
num_attention_heads = 12
intermediate_size = 3072
hidden_dropout_prob = 0.1
attention_probs_dropout_prob = 0.1
max_position_embeddings = 200
num_segments = 2
input_ids = torch.tensor([[1, 2, 3, 4, 5], [6, 7, 8, 9, 10]]) # Example input tensor of shape (batch_size, sequence_length)
segment_ids = torch.tensor([[0, 0, 0, 0, 0], [1, 1, 1, 1, 1]]) # Example segment tensor of shape (batch_size, sequence_length)
embedding = BertEmbedding(vocab_size, embedding_dim)
encoder = BertEncoder(hidden_size, num_attention_heads, intermediate_size, hidden_dropout_prob)
embeddings = embedding(input_ids, segment_ids)
encoder_output = encoder(embeddings, attention_mask=None)
print(encoder_output.shape)
这个示例代码展示了如何使用PyTorch构建一个简化的BERT模型。代码中定义了BertEmbedding、BertEncoder、BertAttention等模块,用于实现BERT的不同组件和层。
GPT 系列
GPT是OpenAI公司发布的,目前已经发展到GPT4。GPT系列的论文中都没有架构图,只说也是用Transformer做的。
GPT1
论文是Improving Language Understanding by Generative Pre-Training,比Bert出现得早,只是因为效果没有Bert那么好,所以没有引起轰动。
GPT全称是Generative Pre Training,生成式预训练。预训练用的是最基本的n-gram语言模型。
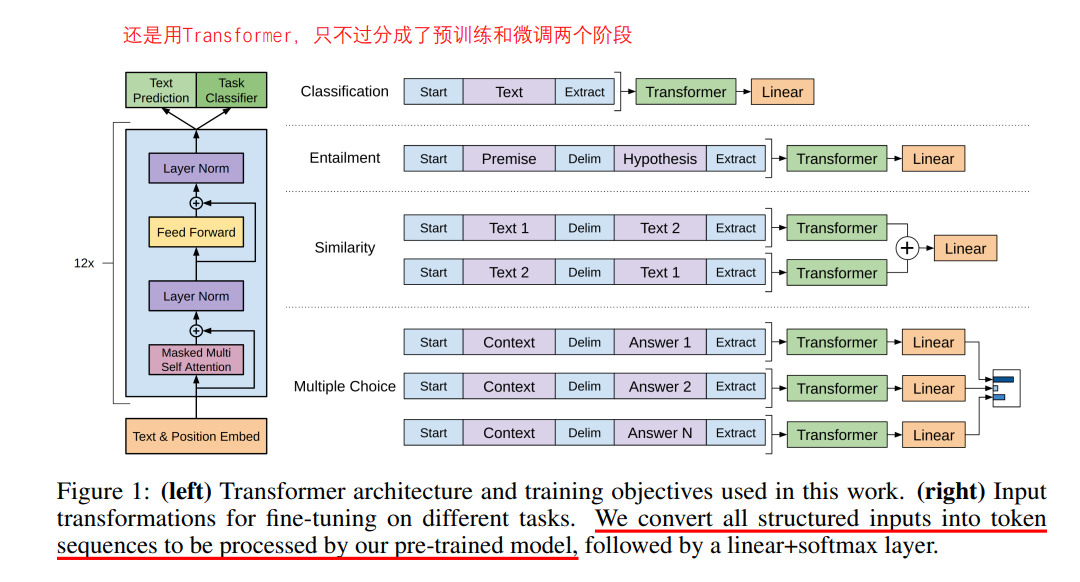
一个小细节,Bert和GPT都是用的Tansformer的编码器(因为编解码器的结构差不多)。如图所示,GPT把编码器重复了12遍。这么看来,Bert和GPT的结构应该是相同的,并且都是预训练+微调,但为什么Bert效果更好?是因为Bert是双向的,并且用了masked。
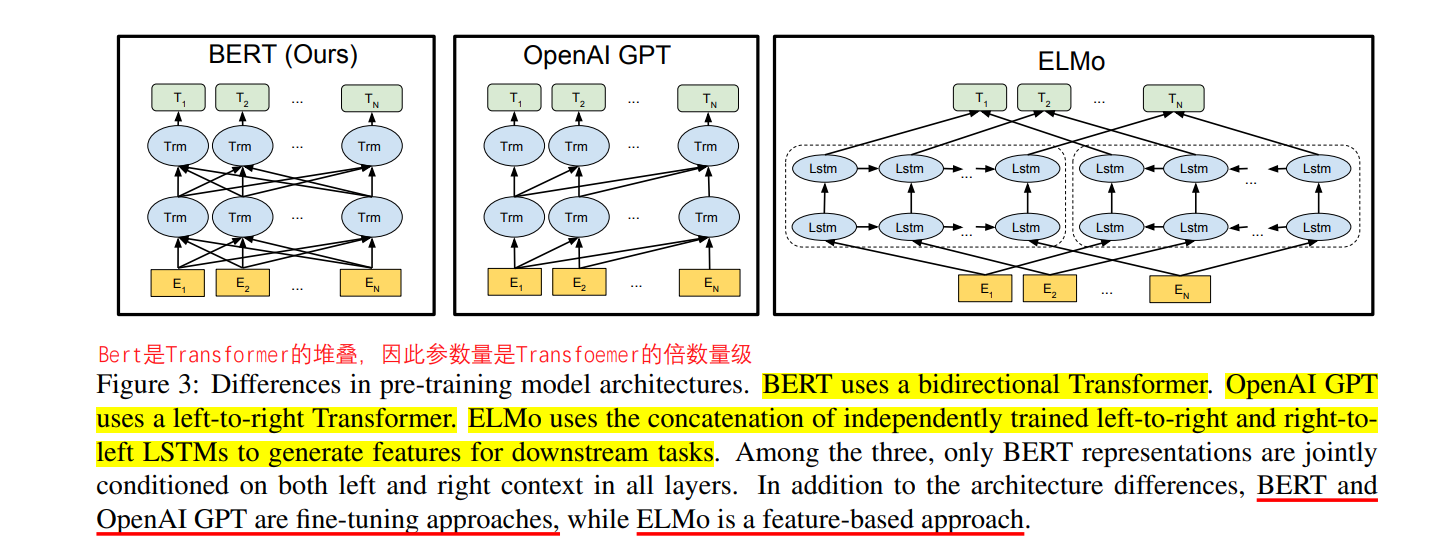
ChatGPT 给出的简化版本的 GPT 的示例代码,用于生成文本:
import torch
import torch.nn as nn
import torch.nn.functional as F
class GPTBlock(nn.Module):
def __init__(self, hidden_dim, num_heads, dropout_rate=0.1):
super(GPTBlock, self).__init__()
self.attention = nn.MultiheadAttention(hidden_dim, num_heads, dropout=dropout_rate)
self.layer_norm1 = nn.LayerNorm(hidden_dim)
self.feed_forward = nn.Sequential(
nn.Linear(hidden_dim, 4 * hidden_dim),
nn.ReLU(),
nn.Linear(4 * hidden_dim, hidden_dim)
)
self.layer_norm2 = nn.LayerNorm(hidden_dim)
self.dropout = nn.Dropout(dropout_rate)
def forward(self, x):
attention_output, _ = self.attention(x, x, x)
x = x + self.dropout(attention_output)
x = self.layer_norm1(x)
feed_forward_output = self.feed_forward(x)
x = x + self.dropout(feed_forward_output)
x = self.layer_norm2(x)
return x
class GPT(nn.Module):
def __init__(self, vocab_size, embedding_dim, hidden_dim, num_heads, num_layers):
super(GPT, self).__init__()
self.token_embeddings = nn.Embedding(vocab_size, embedding_dim)
self.position_embeddings = nn.Embedding(1000, embedding_dim) # 1000 is the maximum sequence length
self.blocks = nn.ModuleList([GPTBlock(hidden_dim, num_heads) for _ in range(num_layers)])
self.fc = nn.Linear(embedding_dim, vocab_size)
def forward(self, input_ids):
positions = torch.arange(input_ids.size(1)).unsqueeze(0).to(input_ids.device)
token_embeds = self.token_embeddings(input_ids)
position_embeds = self.position_embeddings(positions)
x = token_embeds + position_embeds
for block in self.blocks:
x = block(x)
logits = self.fc(x)
return logits
# Example usage
vocab_size = 10000
embedding_dim = 512
hidden_dim = 512
num_heads = 8
num_layers = 6
gpt = GPT(vocab_size, embedding_dim, hidden_dim, num_heads, num_layers)
input_ids = torch.tensor([[1, 2, 3, 4, 5]]) # Example input tensor of shape (batch_size, sequence_length)
output_logits = gpt(input_ids)
print(output_logits.shape)
这个示例代码展示了如何使用PyTorch构建了一个简化的GPT模型。代码中定义了GPTBlock和GPT类,GPTBlock表示GPT模型中的一个块(block),由多头自注意力和前馈神经网络组成,GPT类整合了多个块。
请注意,这只是一个简化的示例,并且省略了一些重要的细节,如位置编码和残差连接等。实际上,GPT模型包含更多的层和组件,并且通常在预训练阶段使用了更多的技巧,如遮蔽语言建模(Masked Language Modeling)等。
GPT2
论文名称:Language Models are Unsupervised Multitask Learners。2和1一样,还是用语言模型n-gram进行无监督学习,进行zero-shot task。架构还是用的Transformer。
让给出GPT2的代码,但是给出的和GPT一样:
GPT3
论文名称:Language Models are Few-Shot Learners。架构上相比GPT2没有创新,主要还是体量变大,参数量1750亿。测试是在few-shot下进行的。
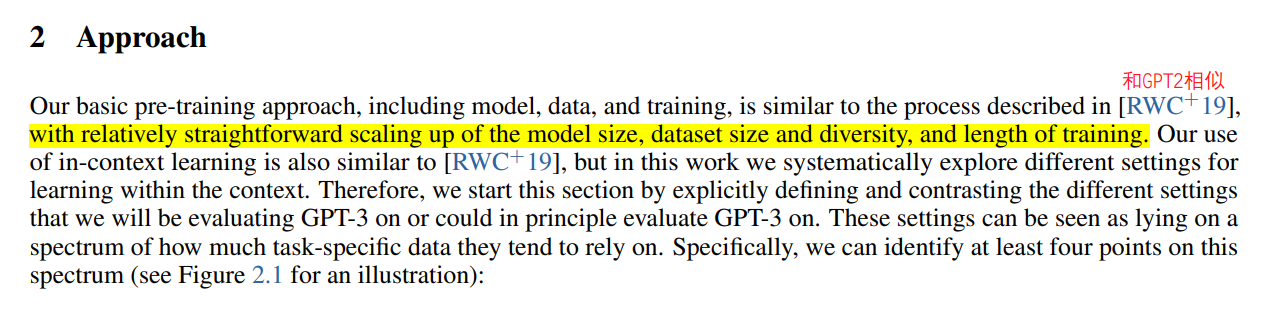
论文最初介绍了各种任务,算术、纠错、翻译等,感觉应该是各种基本能力有专门进行训练调优。各种能力是分开的,就像人会学习数学、语文等。并详细介绍了 zero-shot、one-shot和few-shot是怎么回事。xx-shot是说的在任务/微调阶段。
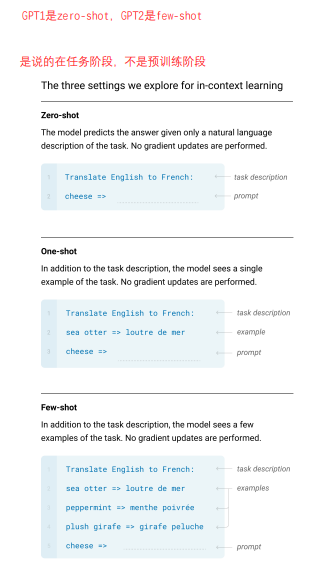
文中对于GPT3的模型架构没有过多的解释,只说了
We use the same model and architecture as GPT-2 [RWC+19]
Our basic pre-training approach, including model, data, and training, is similar to the process described in [RWC+19],
with relatively straightforward scaling up of the model size, dataset size and diversity, and length of training.
GPT4
GPT4 没有论文,只有一个技术报告:GPT-4 Technical Report
GPT-4 is a Transformer-based model pre-trained to predict the next token in a document
A core component of this project was developing infrastructure and optimization methods that behave predictably across a wide
range of scales.
反正就是说越来越大,然后就主要介绍性能和效果了。
3 和 4 都是没有开源的。
总结
除了上面介绍的这些模型,还有很多很多大模型,比如百度的文心一言、Meta(原来的Facebook)的LLaMA、东北大学的TechGPT等。人大的LLM综述里面有详细的总结。本文介绍的是比较火的、有代表性的几个,用于梳理各模型的技术发展脉络。
核心的底层结构就是Transformer,就是规模(包括模型参数和语料的规模)越来越大,集成了很多通用的功能,呈现出来让人感觉效果好。还是偏通用AI(General AI),本质是精准的信息检索代替一些繁琐低脑力成本的劳动,集成一些通用功能。到每个行业的话,还是有行业壁垒的,无论是行业知识,还是行业经验和能力。