GPU CPU运算时间测试
GPU CPU运算时间测试
本文主要探讨GPU,CPU在做一些复杂运算的时间测试
实验任务
1.向量加法
两个相同维度的向量a,b做加法,分别测试GPU并行时间(包含数据拷贝时间),CPU串行时间。
2.双边滤波
简要介绍:
双边滤波(Bilateral filter)是一种非线性的滤波方法,是结合图像的空间邻近度和像素值相似度的一种折衷处理,同时考虑空域信息和灰度相似性,达到保边去噪的目的。具有简单、非迭代、局部的特点。
双边滤波在计算上是昂贵的,并且具有使优化复杂化的非线性分量。当在中央处理单元(CPU)上顺序运行时,这会花费很多时间,并且双边滤波算法的快速逼近范围很广,使得其算法优化极其困难。
任务描述:
测试\(sigmaColor = 15.0, sigmaSpace = 15.0\),高斯核直径\(d\)变化时, 在大小为 \(800 \times 1068\)图片上的效率。
样例图片:
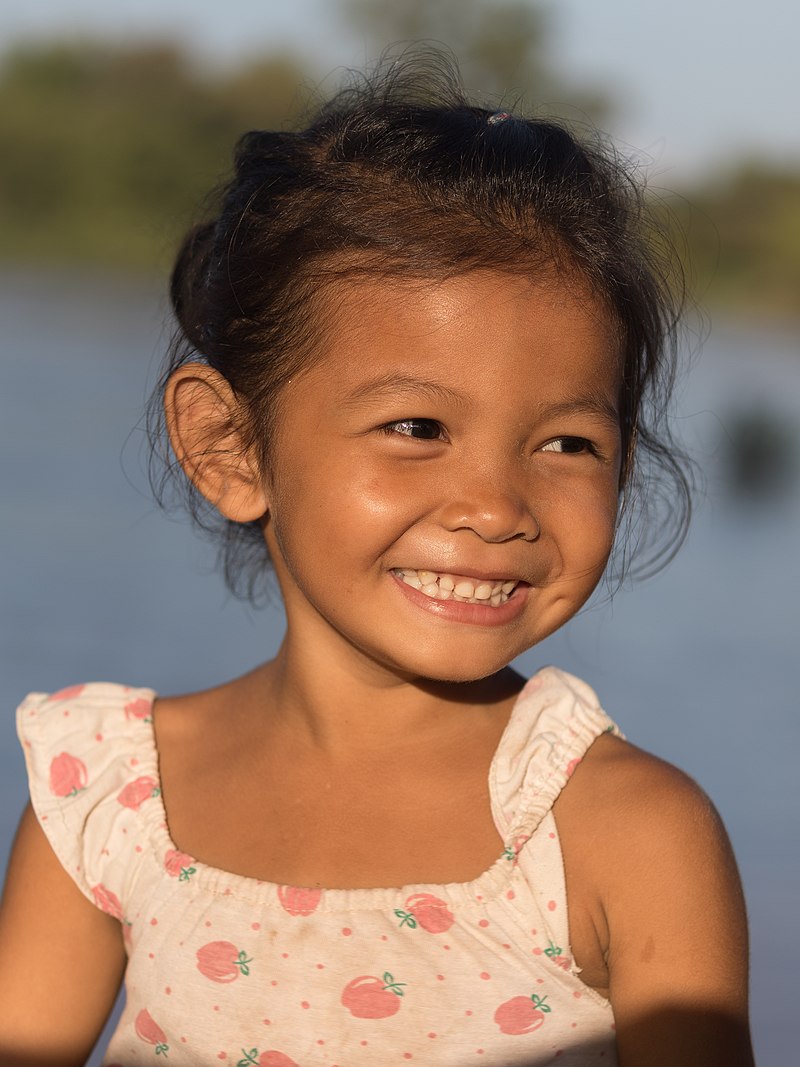
实验环境
- Ubuntu 18.04
- Intel(R) Xeon(R) Silver 4210 CPU @ 2.20GHz
- RTX3090
ps:之前在本地电脑测过向量加法,现在突然放假回家,因此两个任务统一环境,重新测一遍。
实验结果
典型的 CUDA 程序的执行流程如下:
因此对于cuda程序的计时可以分为三部分:数据从内存拷贝到显存
,执行计算
,计算结果从显存拷贝回内存
。
对于CPU的计时只有执行计算
。
1.向量加法
向量a,b的维度 | 262144\((512 \times 512)\) | 1048576\((1024 \times 1024)\) | 4194304\((2048 \times 2048)\) |
---|---|---|---|
实验结果截图 | ![]() |
![]() |
![]() |
内存数据拷贝到GPU时间消耗 | 0.000631 sec | 0.001988 sec | 0.008227sec |
GPU计算时间 | 0.000016 sec | 0.000013 sec | 0.000016 sec |
结果从显存拷贝到内存时间消耗 | 0.000415sec | 0.001169 sec | 0.002168 sec |
显存计算总时间(上述相加) | 0.001062sec | 0.003170 sec | 0.010411 sec |
CPU 计算时间 | 0.001103 sec | 0.003196 sec | 0.017829 sec |
分析:
- 对比GPU和CPU的计算时间,随着数据维度增大,GPU并行计算时间没有明显的增大,CPU计算时间逐渐增大。
- 随着数据维度增大,数据在内存到显存双向拷贝时间逐渐增大。
- 在简单的向量相加任务上,GPU并没有突出的优势
2.双边滤波
d = 2 | d = 4 | d = 8 | d = 64 | |
---|---|---|---|---|
实验截图 | ![]() |
![]() |
![]() |
![]() |
数据拷贝总时间 | 1.532352 ms | 1.566624 ms | 1.563072 ms | 1.494659 ms |
GPU计算时间 | 0.287392 ms | 0.697184 ms | 2.123936 ms | 107.015038 ms |
GPU总时间 | 1.819744 ms | 2.263808 ms | 3.687008 ms | 108.509697 ms |
CPU计算时间 | 376.712 ms | 365.958 ms | 504.757 ms | 7407.72 ms |
可以发现GPU还是很猛的。
放一下d=8的结果,实现了类似于磨皮的效果:
原图 | CPU | GPU |
---|---|---|
![]() |
![]() |
![]() |
实验代码
1.向量加法
-
sum.cu
#include <cuda_runtime.h> #include <stdio.h> #include <time.h> #include "freshman.h" // CPU 加法 void sumArrays(float *a, float *b, float *res, const int size) { for (int i = 0; i < size; i += 1) { res[i] = a[i] + b[i]; } } // GPU 加法 __global__ void sumArraysGPU(float *a, float *b, float *res, int N) { int i = blockIdx.x * blockDim.x + threadIdx.x; if (i < N) res[i] = a[i] + b[i]; } int main(int argc, char **argv) { // set up device initDevice(0); int nElem = 512*512; //int nElem = 1024*1024; //int nElem = 2048*2048; printf("Vector size:%d\n", nElem); // 内存数据申请空间 int nByte = sizeof(float) * nElem; float *a_h = (float *)malloc(nByte); float *b_h = (float *)malloc(nByte); float *res_h = (float *)malloc(nByte); float *res_from_gpu_h = (float *)malloc(nByte); memset(res_h, 0, nByte); memset(res_from_gpu_h, 0, nByte); // 内存数据随机初始化 initialData(a_h, nElem); initialData(b_h, nElem); // 显存申请空间 float *a_d, *b_d, *res_d; CHECK(cudaMalloc((float **)&a_d, nByte)); CHECK(cudaMalloc((float **)&b_d, nByte)); CHECK(cudaMalloc((float **)&res_d, nByte)); // 内存到显存数据拷贝 clock_t start, end; start = clock(); CHECK(cudaMemcpy(a_d, a_h, nByte, cudaMemcpyHostToDevice)); CHECK(cudaMemcpy(b_d, b_h, nByte, cudaMemcpyHostToDevice)); end = clock(); double copytime = (double)(end - start) / CLOCKS_PER_SEC; printf("内存数据拷贝到GPU时间消耗\t %f sec\n", copytime); dim3 block(512); dim3 grid((nElem - 1) / block.x + 1); // GPU 加法 double iStart, iElaps; iStart = cpuSecond(); sumArraysGPU<<<grid, block>>>(a_d, b_d, res_d, nElem); iElaps = cpuSecond() - iStart; double GPU_Cla = iElaps; printf("GPU计算时间 \t\t\t\t %f sec\n", GPU_Cla); //显存到内存数据拷贝 start = clock(); CHECK(cudaMemcpy(res_from_gpu_h, res_d, nByte, cudaMemcpyDeviceToHost)); end = clock(); double copytime2 = (double)(end - start) / CLOCKS_PER_SEC; printf("结果从显存拷贝到内存时间消耗\t %f sec\n", copytime2); printf("GPU时间总消耗\t\t\t\t %f sec\n", copytime2 + GPU_Cla + copytime); // CPU 加法 start = clock(); sumArrays(a_h,b_h,res_h,nElem); end = clock(); printf("CPU 计算时间\t\t\t\t %f sec\n", (double)(end - start) / CLOCKS_PER_SEC); checkResult(res_h, res_from_gpu_h, nElem); cudaFree(a_d); cudaFree(b_d); cudaFree(res_d); free(a_h); free(b_h); free(res_h); free(res_from_gpu_h); return 0; }
-
freshman.h
#ifndef FRESHMAN_H #define FRESHMAN_H #define CHECK(call)\ {\ const cudaError_t error=call;\ if(error!=cudaSuccess)\ {\ printf("ERROR: %s:%d,",__FILE__,__LINE__);\ printf("code:%d,reason:%s\n",error,cudaGetErrorString(error));\ exit(1);\ }\ } #include <time.h> #ifdef _WIN32 # include <windows.h> #else # include <sys/time.h> #endif #ifdef _WIN32 int gettimeofday(struct timeval *tp, void *tzp) { time_t clock; struct tm tm; SYSTEMTIME wtm; GetLocalTime(&wtm); tm.tm_year = wtm.wYear - 1900; tm.tm_mon = wtm.wMonth - 1; tm.tm_mday = wtm.wDay; tm.tm_hour = wtm.wHour; tm.tm_min = wtm.wMinute; tm.tm_sec = wtm.wSecond; tm. tm_isdst = -1; clock = mktime(&tm); tp->tv_sec = clock; tp->tv_usec = wtm.wMilliseconds * 1000; return (0); } #endif double cpuSecond() { struct timeval tp; gettimeofday(&tp,NULL); return((double)tp.tv_sec+(double)tp.tv_usec*1e-6); } void initialData(float* ip,int size) { time_t t; srand((unsigned )time(&t)); for(int i=0;i<size;i++) { ip[i]=(float)(rand()&0xffff)/1000.0f; } } void initialData_int(int* ip, int size) { time_t t; srand((unsigned)time(&t)); for (int i = 0; i<size; i++) { ip[i] = int(rand()&0xff); } } void printMatrix(float * C,const int nx,const int ny) { float *ic=C; printf("Matrix<%d,%d>:",ny,nx); for(int i=0;i<ny;i++) { for(int j=0;j<nx;j++) { printf("%6f ",C[j]); } ic+=nx; printf("\n"); } } void initDevice(int devNum) { int dev = devNum; cudaDeviceProp deviceProp; CHECK(cudaGetDeviceProperties(&deviceProp,dev)); printf("Using device %d: %s\n",dev,deviceProp.name); CHECK(cudaSetDevice(dev)); } void checkResult(float * hostRef,float * gpuRef,const int N) { double epsilon=1.0E-8; for(int i=0;i<N;i++) { if(abs(hostRef[i]-gpuRef[i])>epsilon) { printf("Results don\'t match!\n"); printf("%f(hostRef[%d] )!= %f(gpuRef[%d])\n",hostRef[i],i,gpuRef[i],i); return; } } printf("Check result success!\n"); } #endif//FRESHMAN_H
2. \(双边滤波^{[1]}\)
-
kernel.cu
#include <iostream> #include <algorithm> #include <ctime> #include <opencv2/opencv.hpp> #include "cuda_runtime.h" #include "device_launch_parameters.h" #include <cuda.h> #include <device_functions.h> #define M_PI 3.14159265358979323846 using namespace std; using namespace cv; //一维高斯kernel数组 __constant__ float cGaussian[64]; //声明纹理参照系,以全局变量形式出现 texture<unsigned char, 2, cudaReadModeElementType> inTexture; //计算一维高斯距离权重,二维高斯权重可由一维高斯权重做积得到 void updateGaussian(int r, double sd) { float fGaussian[64]; for (int i = 0; i < 2 * r + 1; i++) { float x = i - r; fGaussian[i] = 1 / (sqrt(2 * M_PI) * sd) * expf(-(x * x) / (2 * sd * sd)); } cudaMemcpyToSymbol(cGaussian, fGaussian, sizeof(float) * (2 * r + 1)); } // 一维高斯函数,计算像素差异权重 __device__ inline double gaussian(float x, double sigma) { return 1 / (sqrt(2 * M_PI) * sigma) * __expf(-(powf(x, 2)) / (2 * powf(sigma, 2))); } __global__ void gpuCalculation(unsigned char* input, unsigned char* output, int width ,int height, int r,double sigmaColor) { int txIndex = blockIdx.x * blockDim.x + threadIdx.x; int tyIndex = blockIdx.y * blockDim.y + threadIdx.y; if ((txIndex < width) && (tyIndex < height)) { double iFiltered = 0; double k = 0; //纹理拾取,得到要计算的中心像素点 unsigned char centrePx = tex2D(inTexture, txIndex, tyIndex); //进行卷积运算 for (int dy = -r; dy <= r; dy++) { for (int dx = -r; dx <= r; dx++) { //得到kernel区域内另一像素点 unsigned char currPx = tex2D(inTexture, txIndex + dx, tyIndex + dy); // Weight = 1D Gaussian(x_axis) * 1D Gaussian(y_axis) * Gaussian(Color difference) double w = (cGaussian[dy + r] * cGaussian[dx + r]) * gaussian(centrePx - currPx, sigmaColor); iFiltered += w * currPx; k += w; } } output[tyIndex * width + txIndex] = iFiltered / k; } } void MyBilateralFilter(const Mat& input, Mat& output, int r, double sigmaColor, double sigmaSpace) { //GPU计时事件 cudaEvent_t start, stop, cal_start, cal_stop; float time_copy, total_time; cudaEventCreate(&start); cudaEventCreate(&stop); cudaEventCreate(&cal_start); cudaEventCreate(&cal_stop); cudaEventRecord(start, 0); //计算图片大小 int gray_size = input.step * input.rows; //在device上开辟2维数据空间保存输入输出数据 unsigned char* d_input = NULL; unsigned char* d_output; updateGaussian(r, sigmaSpace); //分配device内存 cudaMalloc<unsigned char>(&d_output, gray_size); //纹理绑定 size_t pitch; cudaMallocPitch(&d_input, &pitch, sizeof(unsigned char) * input.step, input.rows); cudaChannelFormatDesc desc = cudaCreateChannelDesc<unsigned char>(); cudaMemcpy2D(d_input, pitch, input.ptr(), sizeof(unsigned char) * input.step, sizeof(unsigned char) * input.step, input.rows, cudaMemcpyHostToDevice); //将纹理参照系绑定到一个CUDA数组 cudaBindTexture2D(0, inTexture, d_input, desc, input.step, input.rows, pitch); dim3 block(16, 16); dim3 grid((input.cols + block.x - 1) / block.x, (input.rows + block.y - 1) / block.y); cudaEventRecord(cal_start, 0); gpuCalculation <<< grid, block >>> (d_input, d_output, input.cols, input.rows, r, sigmaColor); cudaEventRecord(cal_stop, 0); cudaEventSynchronize(cal_stop); cudaEventElapsedTime(&time_copy, cal_start, cal_stop); printf("Cal Time for the GPU: %f ms\n", time_copy); //将device上的运算结果拷贝到host上 cudaMemcpy(output.ptr(), d_output, gray_size, cudaMemcpyDeviceToHost); cudaEventRecord(stop, 0); cudaEventSynchronize(stop); //释放device和host上分配的内存 cudaFree(d_input); cudaFree(d_output); // Calculate and print kernel run time cudaEventElapsedTime(&total_time, start, stop); printf("Copy Time for the GPU: %f ms\n", total_time - time_copy); printf("Toal Time for the GPU: %f ms\n", total_time); }
-
main.cpp
#include <opencv2/opencv.hpp> #include <iostream> using namespace std; using namespace cv; void MyBilateralFilter(const Mat& input, Mat& output, int r, double sI, double sS); int main() { //高斯核直径 int d = 64; double sigmaColor = 15.0, sigmaSpace = 15.0; //将原始图像转化为灰度图像再打开 Mat srcImg = imread("1.jpg", IMREAD_GRAYSCALE); //分配host内存 Mat dstImg(srcImg.rows, srcImg.cols, CV_8UC1); Mat dstImgCV; //在GPU上运行测速 MyBilateralFilter(srcImg, dstImg, d/2, sigmaColor, sigmaSpace); //使用OpenCV bilateral filter在cpu上测速 clock_t start_s = clock(); bilateralFilter(srcImg, dstImgCV, d, sigmaColor, sigmaSpace); clock_t stop_s = clock(); cout << "Time for the CPU: " << (stop_s - start_s) / double(CLOCKS_PER_SEC) * 1000 << " ms" << endl; //展示图片 imshow("原图", srcImg); imwrite("space2.jpg", srcImg); imshow("GPU加速双边滤波", dstImg); imwrite("space2.jpg", dstImg); imshow("CPU双边滤波", dstImgCV); imwrite("space2.jpg", dstImgCV); cv::waitKey(); }
进一步的讨论(双边滤波)
-
gird,block的划分对速度的影响?
要回答这个问题需要一些先验知识,所以最近去学习了一些GPU的组织架构,熟悉了一下相关概念。
由于 SM 的基本执行单元是包含 32 个线程的线程束,所以 block 大小一般要设置为 32 的倍数。
因此在d=8、32的时候,分别设置了以下五种情况:
d = 8 d = 32 只关注第一行,Cal time for GPU就行。
就这个任务总体来看,对RTX3090来说,对于一张分辨率确定的图片,在
dim3 block(64,64)
上发生了改变。我查了一下3090的SM数量是82。
想了一下,还没法回答这个问题,需要以后继续深入探讨。
-
关于使用纹理内存的问题
我看互联网上的描述,差不多就是纹理内存有一些特性(比如可以归一化等等),访问二维矩阵的邻域会获得加速
但是这个访问邻域应该更多地体现在差值上:
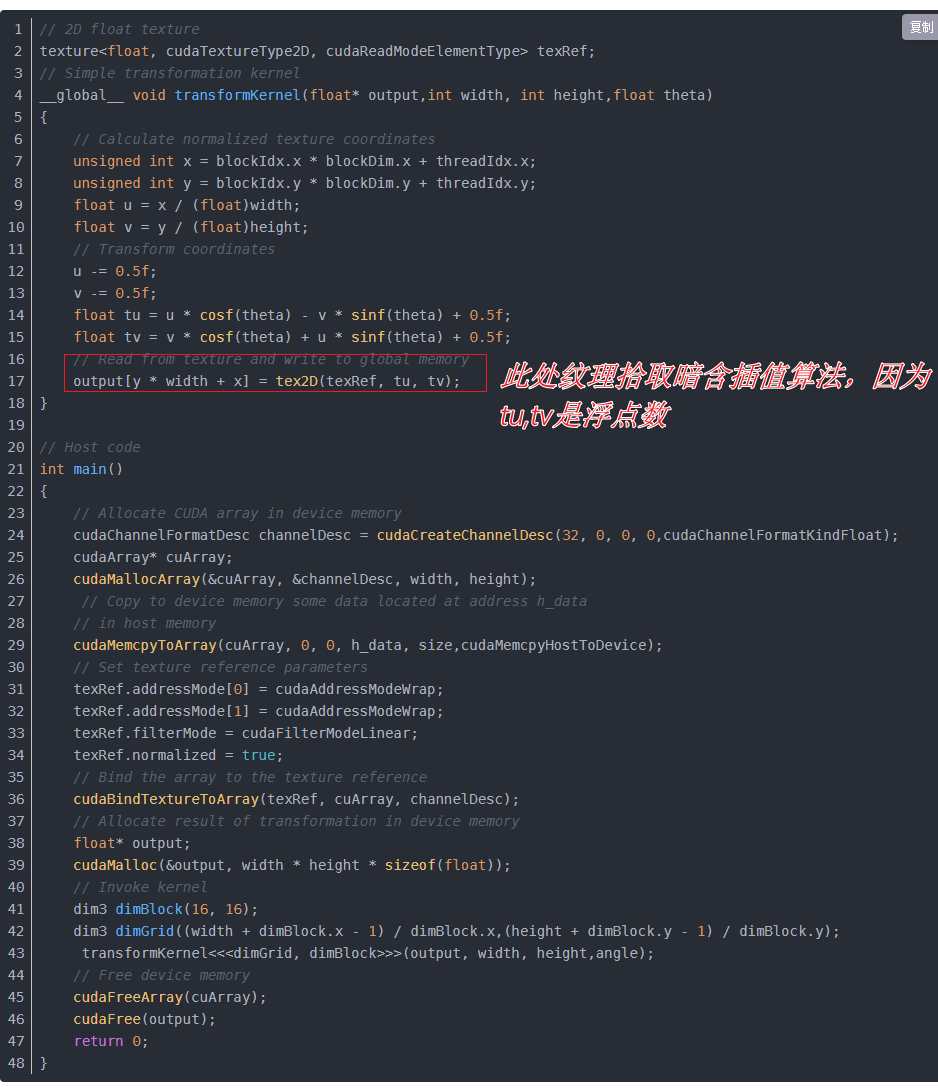
我又回头看了一下作者的代码:
都是整型
,其实也就是没有插值(因为都精确的落在了某个像素上),所以修改为直接从input
读取数据,两种效果应该差不多,果不其然:
texture memory | global memory | |
---|---|---|
d = 8 | ![]() |
![]() |
d = 32 | ![]() |
![]() |
相关代码:
kernel_global_input.cu
#include <iostream>
#include <algorithm>
#include <ctime>
#include <opencv2/opencv.hpp>
#include "cuda_runtime.h"
#include "device_launch_parameters.h"
#include <cuda.h>
#include <device_functions.h>
#define M_PI 3.14159265358979323846
using namespace std;
using namespace cv;
//一维高斯kernel数组
__constant__ float cGaussian[64];
//声明纹理参照系,以全局变量形式出现
texture<unsigned char, 2, cudaReadModeElementType> inTexture;
//计算一维高斯距离权重,二维高斯权重可由一维高斯权重做积得到
void updateGaussian(int r, double sd)
{
float fGaussian[64];
for (int i = 0; i < 2 * r + 1; i++)
{
float x = i - r;
fGaussian[i] = 1 / (sqrt(2 * M_PI) * sd) * expf(-(x * x) / (2 * sd * sd));
}
cudaMemcpyToSymbol(cGaussian, fGaussian, sizeof(float) * (2 * r + 1));
}
// 一维高斯函数,计算像素差异权重
__device__ inline double gaussian(float x, double sigma)
{
return 1 / (sqrt(2 * M_PI) * sigma) * __expf(-(powf(x, 2)) / (2 * powf(sigma, 2)));
}
__global__ void gpuCalculation(unsigned char* input, unsigned char* output, int width ,int height, int r,double sigmaColor)
{
int txIndex = blockIdx.x * blockDim.x + threadIdx.x;
int tyIndex = blockIdx.y * blockDim.y + threadIdx.y;
if ((txIndex < width) && (tyIndex < height))
{
double iFiltered = 0;
double k = 0;
unsigned char centrePx = input[tyIndex * width + txIndex];
//进行卷积运算
for (int dy = -r; dy <= r; dy++) {
for (int dx = -r; dx <= r; dx++) {
if(txIndex+dx >= 0 && tyIndex+dy >=0 && txIndex+dx <= width && tyIndex+dy <= height)
{
//得到kernel区域内另一像素点
unsigned char currPx = input[(tyIndex+dy) * width + txIndex+dx];
// Weight = 1D Gaussian(x_axis) * 1D Gaussian(y_axis) * Gaussian(Color difference)
double w = (cGaussian[dy + r] * cGaussian[dx + r]) * gaussian(centrePx - currPx, sigmaColor);
iFiltered += w * currPx;
k += w;
}
}
}
output[tyIndex * width + txIndex] = iFiltered / k;
}
}
void MyBilateralFilter(const Mat& input, Mat& output, int r, double sigmaColor, double sigmaSpace)
{
//GPU计时事件
cudaEvent_t start, stop, cal_start, cal_stop;
float time_copy, total_time;
cudaEventCreate(&start);
cudaEventCreate(&stop);
cudaEventCreate(&cal_start);
cudaEventCreate(&cal_stop);
cudaEventRecord(start, 0);
//计算图片大小
int gray_size = input.step * input.rows;
//在device上开辟2维数据空间保存输入输出数据
unsigned char* d_input;
unsigned char* d_output;
updateGaussian(r, sigmaSpace);
//分配device内存
cudaMalloc<unsigned char>(&d_input, gray_size);
cudaMalloc<unsigned char>(&d_output, gray_size);
// global memory 图片数据拷贝
cudaMemcpy(d_input, input.ptr(), gray_size, cudaMemcpyHostToDevice);
dim3 block(16, 16);
dim3 grid((input.cols + block.x - 1) / block.x, (input.rows + block.y - 1) / block.y);
cudaEventRecord(cal_start, 0);
gpuCalculation <<< grid, block >>> (d_input, d_output, input.cols, input.rows, r, sigmaColor);
cudaEventRecord(cal_stop, 0);
cudaEventSynchronize(cal_stop);
cudaEventElapsedTime(&time_copy, cal_start, cal_stop);
printf("Cal Time for the GPU: %f ms\n", time_copy);
//将device上的运算结果拷贝到host上
cudaMemcpy(output.ptr(), d_output, gray_size, cudaMemcpyDeviceToHost);
cudaEventRecord(stop, 0);
cudaEventSynchronize(stop);
//释放device和host上分配的内存
cudaFree(d_input);
cudaFree(d_output);
// Calculate and print kernel run time
cudaEventElapsedTime(&total_time, start, stop);
printf("Copy Time for the GPU: %f ms\n", total_time - time_copy);
printf("Toal Time for the GPU: %f ms\n", total_time);
}
引用
本文来自博客园,作者:CuriosityWang,转载请注明原文链接:https://www.cnblogs.com/curiositywang/p/16974393.html