Well, I really think u have to write note here, because when I new a word, I always lost.
Get down to business. For decision tree, which is based on rule, also use some statistical method, in other words, heuristic rule.
Error rate
you couldn't use the error rate in training data, what we care about is that the error rate in text data. Consequently, u can use the optimistic and pessimistic statistical method to get the samiliar error rate.
Problems from missing value
Firstly, when the data is not very mess, which means the date don't possess the statistical quality, thus, u couldn't use the statistical method to predict.
Secondly, the decision tree are not unique, consequently, we can use greed or heuristic algorithm to solve the better tree.
Thirdly, for some points, u couldn't separate by using the only attribute, perhaps u should use the expression of the attributes, such as x + y = 1.
Model Evaluation
u know when u create a decision tree, u need a model evalution to know whether it's good. So We introduce two matrices, one is confusion matrix, the other is cost matrix. u know everything have its own environments.
Lastly, we introduce the three index to evaluate the model, which are precision, recall, F.
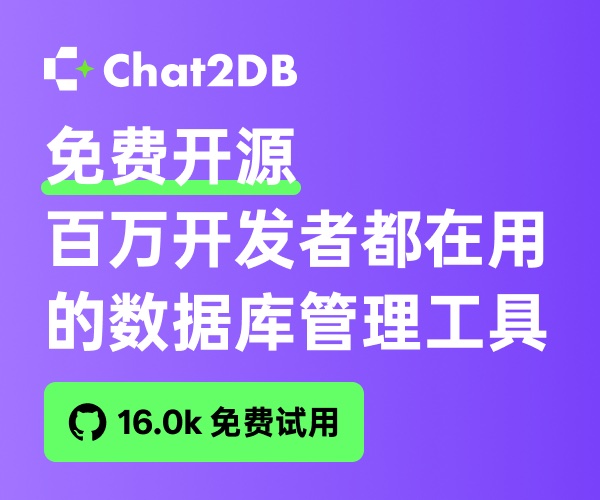
