【WPC】2020-DAC-Non-uniform DNN Structured Subnets Sampling for Dynamic Inference-论文阅读
Non-uniform DNN Structured Subnets Sampling for Dynamic Inference
2020-DAC-Non-uniform DNN Structured Subnets Sampling for Dynamic Inference
2020 57th ACM/IEEE Design Automation Conference (DAC) CCF-A
来源:ChenBong 博客园
- Institute:Arizona State University
- Author:Li Yang, Zhezhi He(SJTU AP), Yu Cao(IEEE Fellow, H56), Deliang Fan
- GitHub:/
- Citation:4
Introduction
- 设计了一种 L2 filter剪枝的变式, 先对模型进行k=4次剪枝, 获得k个不同大小的剪枝结构(no-uniform width), 再根据k个剪枝结构, 构造参数共享的嵌套超网, 进行one-shot训练
- Non-uniform宽度的动态子网的性能会略低于独立训练的子网(im1000 差距2个点以内), 但超过了s-net
Motivation
- 动态资源自适应的DNN, 达到 FLOPs/acc 的tradeoff
- 之前已经有了S-Net一类的工作, 使用uniform宽度, 本文使用Non-uniform宽度, 需要解决2个问题
- 子网结构的确定 Subnets generation (?)
- 子网联合训练 Fused subnets training (√)
Contribution
- 通过剪枝方法来确定不同大小的non-uniform宽度的子网结构
- 训练包含多个non-uniform宽度的子网的动态网络
Method
Subnets generation
基于 L2-norm 的 Group Lasso 稀疏约束 filter 剪枝
\(\hat{\mathcal{L}}=\mathcal{L}\left(f\left(\boldsymbol{x} ;\left\{\boldsymbol{W}_{l}\right\}_{l=1}^{L}\right), \boldsymbol{t}\right)+\lambda \underbrace{\sum_{l=1}^{L} \sum_{i=1}^{G_{l}} \overbrace{\mathcal{P}\left(\boldsymbol{W}_{l, i}\right)}^{\text {Intra-group} \ {L_2\text{-norm}}} }_{\text {Inter-group } L_{1} \text { -norm }} \qquad(1)\)
- 其中: \(f\left(\boldsymbol{x} ;\left\{\boldsymbol{W}_{l}\right\}_{l=1}^{L}\right)\) 是网络的输出, \(\boldsymbol t\) 是target
- 网络一共 \(L\) 层, 每一层的权重划分为 \(G_l\) 个gruop, \(\mathcal{P}\left(\boldsymbol{W}_{l, i}\right)=||W_{l,i}||_2\) , 表示第 \(l\) 层第 \(i\) 个 group 的所有权重的 L2 norm
- 组内的权重是L2 norm, 组间是L1 norm(即求和); 所以总的稀疏约束项就是将所有 group 的 norm 求和
- 这里按照 output channel 划分 group, 即 filter 剪枝
clipped Group Lasso with adaptive Weight Penalty Clipping (Group Lasso+WPC)
我们发现, 基于上面 group lasso 的filter剪枝, 精度会下降得比较多
观察: 剪枝率高的网络 weight 的 L1 norm 会比剪枝率低的网络来的大
我们假设: 精度下降是由于 group lasso 对非零权重持续的施加惩罚, 导致本该变得更大的权重无法变得足够大
对 Eq(1) reformatted:
\(\tilde{\mathcal{L}}=\mathcal{L}\left(f\left(\boldsymbol{x} ;\left\{\boldsymbol{W}_{l}\right\}_{l=1}^{L}\right), \boldsymbol{t}\right)+\lambda \sum_{l=1}^{L} \sum_{i=1}^{C_{l}} \underbrace{\min \left(\left\|\boldsymbol{W}_{l, i}\right\|_{2} ; \delta_{l}\right)}_{\text {Weight Penalty Clipping }} \\s.t. \quad \delta_{l}=a \cdot \frac{1}{C_{l}} \sum_{i=1}^{C_{l}}\left\|\boldsymbol{W}_{l, i}\right\|_{2} \qquad (3)\)
对本来就很大的权重, 不施加惩罚, 只对低于阈值的权重施加惩罚:
- 如果一个权重不重要, 即使它初始的norm很大, 但由于它的梯度是向norm减小的方向, 该权重最终也会降到阈值以下并被惩罚
- 如果一个权重重要, 即使它初始的norm很小, 惩罚项不足以对抗它norm变大的梯度, 该权重最终也会上升到阈值以上, 不再被惩罚
- 如果一个权重不重要, 初始norm也很小, 很快会被就decay到0; 如果一个权重重要, 初始也很大, 不会受到惩罚
实现了只对"不重要"(norm小)的权重进行惩罚的目的
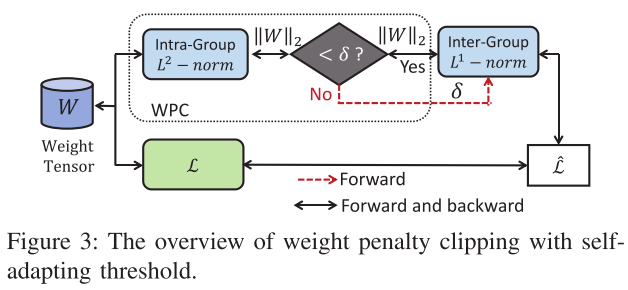
Fused subnets training
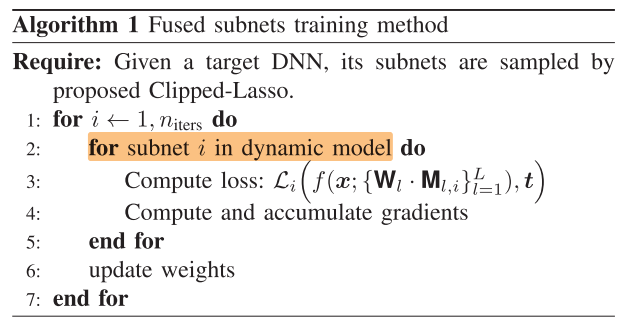
\(\qquad \overbrace{\min _{\left\{\mathbf{W}_{l}\right\}_{l=1}^{L}} \sum_{i=1}^{N} \mathcal{L}_{i}\left(f\left(\boldsymbol{x} ;\left\{\mathbf{W}_{l} \cdot \mathbf{M}_{l, i}\right\}_{l=1}^{L}\right), t\right)}^{\text {training for dynamic inference }} \\ s.t. \underbrace{\left\{\mathbf{M}_{l, i}\right\}_{l=1}^{L}=\arg \min \tilde{\mathcal{L}}\left(f\left(\boldsymbol{x} ;\left\{\mathbf{W}_{l} \cdot \mathbf{M}_{l, i}\right\}\right), \boldsymbol{t}, \lambda_{i}, a_{i}\right)}_{\text {subnets generation/sampling }} \qquad (2)\)
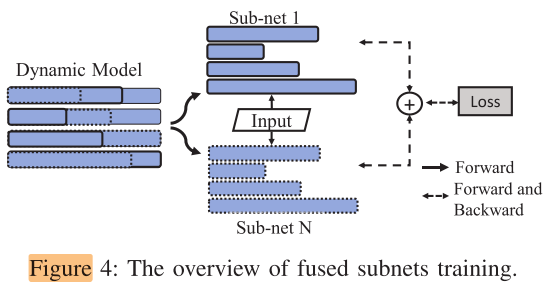
Experiments
ResNet20 on CIFAR-10
FLOPS(10^6), Param(10^4)
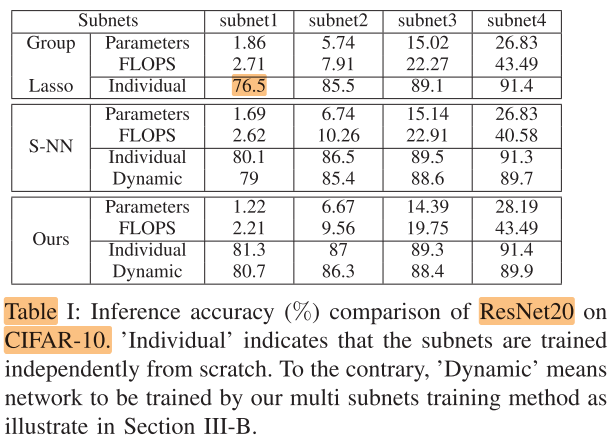
TAS:

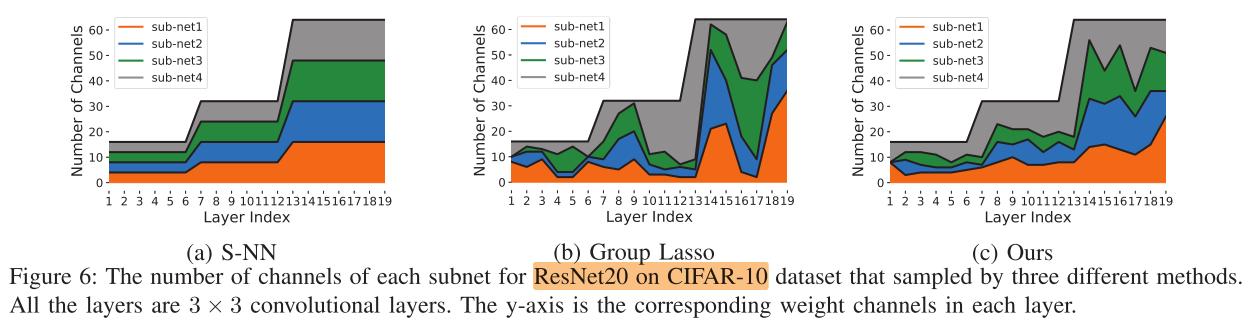
ResNet18 on ImageNet
FLOPS(10^8), Params(10^8)
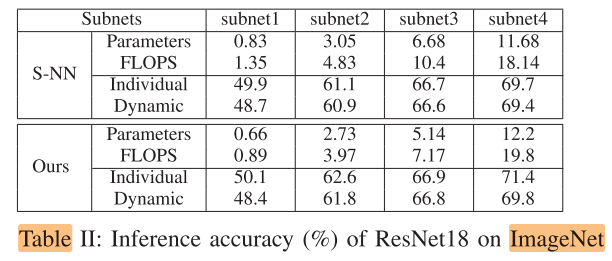
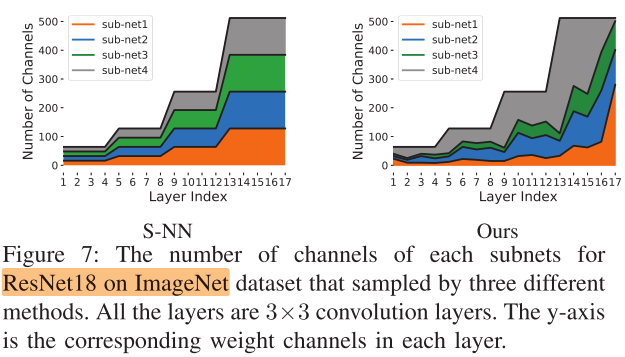
Other
Latency
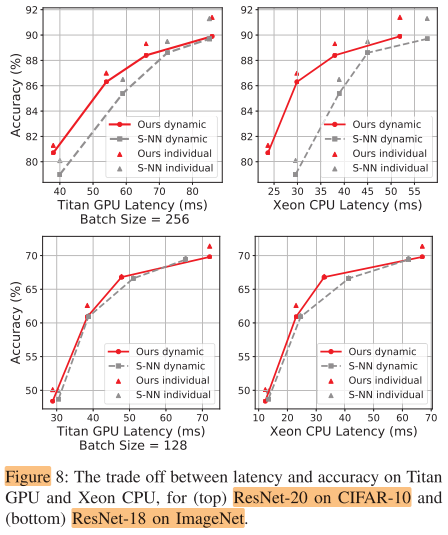
FLOPS-acc trade off
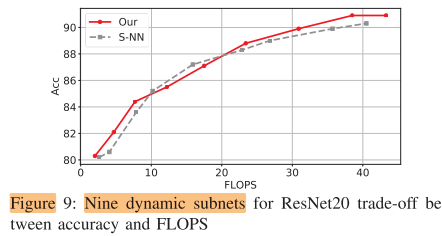
Group Lasso vs +WSP
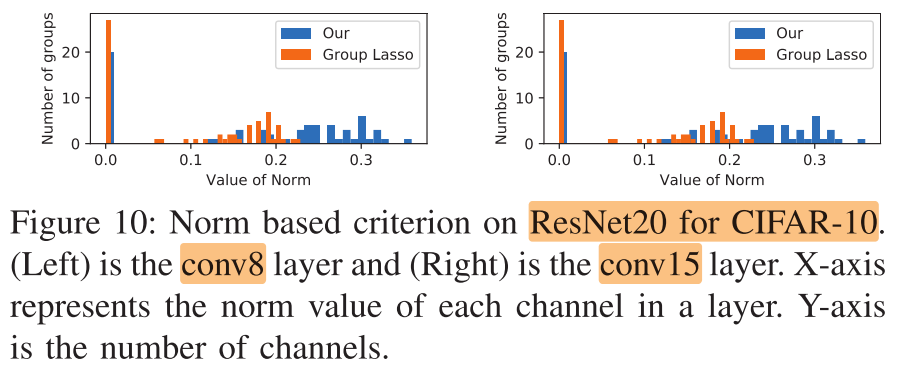
Conclusion
Summary
To Read
Reference
ICRG @ Shanghai Jiao Tong University - Zhezhi (Elliot) He (elliothe.github.io)