快速上手pandas(下)
和上文一样,先导入后面会频繁使用到的模块:
import numpy as np
import pandas as pd
import matplotlib.pyplot as plt
plt.rc('font', family='Arial Unicode MS')
plt.rc('axes', unicode_minus='False')
pd.__version__
'1.1.3'
注意:我这里是Mac系统,用matplotlib
画图时设置字体为Arial Unicode MS
支持中文显示,如果是deepin系统可以设置字体为WenQuanYi Micro Hei
,即:
# import numpy as np
# import pandas as pd
# import matplotlib.pyplot as plt
# plt.rc('font', family='WenQuanYi Micro Hei')
# plt.rc('axes', unicode_minus='False')
如果其他系统画图时中文乱码,可以用以下几行代码查看系统字体,然后自行寻找支持中文的字体:
# from matplotlib.font_manager import FontManager
# fonts = set([x.name for x in FontManager().ttflist])
# print(fonts)
话不多说,继续pandas的学习。
在实际的业务处理中,往往需要将多个数据集、文档合并后再进行分析。
Signature:
pd.concat(
objs: Union[Iterable[~FrameOrSeries], Mapping[Union[Hashable, NoneType], ~FrameOrSeries]],
axis=0,
join='outer',
ignore_index: bool = False,
keys=None,
levels=None,
names=None,
verify_integrity: bool = False,
sort: bool = False,
copy: bool = True,
) -> Union[ForwardRef('DataFrame'), ForwardRef('Series')]
Docstring:
Concatenate pandas objects along a particular axis with optional set logic
along the other axes.
Can also add a layer of hierarchical indexing on the concatenation axis,
which may be useful if the labels are the same (or overlapping) on
the passed axis number.
数据准备:
df1 = pd.DataFrame({'A': ['A0', 'A1', 'A2', 'A3'],
'B': ['B0', 'B1', 'B2', 'B3'],
'C': ['C0', 'C1', 'C2', 'C3'],
'D': ['D0', 'D1', 'D2', 'D3']},
index=[0, 1, 2, 3])
df1
A | B | C | D | |
---|---|---|---|---|
0 | A0 | B0 | C0 | D0 |
1 | A1 | B1 | C1 | D1 |
2 | A2 | B2 | C2 | D2 |
3 | A3 | B3 | C3 | D3 |
df2 = pd.DataFrame({'A': ['A4', 'A5', 'A6', 'A7'],
'B': ['B4', 'B5', 'B6', 'B7'],
'C': ['C4', 'C5', 'C6', 'C7'],
'D': ['D4', 'D5', 'D6', 'D7']},
index=[4, 5, 6, 7])
df2
A | B | C | D | |
---|---|---|---|---|
4 | A4 | B4 | C4 | D4 |
5 | A5 | B5 | C5 | D5 |
6 | A6 | B6 | C6 | D6 |
7 | A7 | B7 | C7 | D7 |
df3 = pd.DataFrame({'A': ['A8', 'A9', 'A10', 'A11'],
'B': ['B8', 'B9', 'B10', 'B11'],
'C': ['C8', 'C9', 'C10', 'C11'],
'D': ['D8', 'D9', 'D10', 'D11']},
index=[8, 9, 10, 11])
df3
A | B | C | D | |
---|---|---|---|---|
8 | A8 | B8 | C8 | D8 |
9 | A9 | B9 | C9 | D9 |
10 | A10 | B10 | C10 | D10 |
11 | A11 | B11 | C11 | D11 |
基本连接:
# 将三个有相同列的表合并到一起
pd.concat([df1, df2, df3])
A | B | C | D | |
---|---|---|---|---|
0 | A0 | B0 | C0 | D0 |
1 | A1 | B1 | C1 | D1 |
2 | A2 | B2 | C2 | D2 |
3 | A3 | B3 | C3 | D3 |
4 | A4 | B4 | C4 | D4 |
5 | A5 | B5 | C5 | D5 |
6 | A6 | B6 | C6 | D6 |
7 | A7 | B7 | C7 | D7 |
8 | A8 | B8 | C8 | D8 |
9 | A9 | B9 | C9 | D9 |
10 | A10 | B10 | C10 | D10 |
11 | A11 | B11 | C11 | D11 |
可以再给每个表给一个一级索引,形成多层索引:
pd.concat([df1, df2, df3], keys=['x', 'y', 'z'])
A | B | C | D | ||
---|---|---|---|---|---|
x | 0 | A0 | B0 | C0 | D0 |
1 | A1 | B1 | C1 | D1 | |
2 | A2 | B2 | C2 | D2 | |
3 | A3 | B3 | C3 | D3 | |
y | 4 | A4 | B4 | C4 | D4 |
5 | A5 | B5 | C5 | D5 | |
6 | A6 | B6 | C6 | D6 | |
7 | A7 | B7 | C7 | D7 | |
z | 8 | A8 | B8 | C8 | D8 |
9 | A9 | B9 | C9 | D9 | |
10 | A10 | B10 | C10 | D10 | |
11 | A11 | B11 | C11 | D11 |
也等同于下面这种方式:
pd.concat({'x': df1, 'y': df2, 'z': df3})
A | B | C | D | ||
---|---|---|---|---|---|
x | 0 | A0 | B0 | C0 | D0 |
1 | A1 | B1 | C1 | D1 | |
2 | A2 | B2 | C2 | D2 | |
3 | A3 | B3 | C3 | D3 | |
y | 4 | A4 | B4 | C4 | D4 |
5 | A5 | B5 | C5 | D5 | |
6 | A6 | B6 | C6 | D6 | |
7 | A7 | B7 | C7 | D7 | |
z | 8 | A8 | B8 | C8 | D8 |
9 | A9 | B9 | C9 | D9 | |
10 | A10 | B10 | C10 | D10 | |
11 | A11 | B11 | C11 | D11 |
合并时不保留原索引,启用新的自然索引:
df4 = pd.DataFrame({'B': ['B2', 'B3', 'B6', 'B7'],
'D': ['D2', 'D3', 'D6', 'D7'],
'F': ['F2', 'F3', 'F6', 'F7']},
index=[2, 3, 6, 7])
df4
B | D | F | |
---|---|---|---|
2 | B2 | D2 | F2 |
3 | B3 | D3 | F3 |
6 | B6 | D6 | F6 |
7 | B7 | D7 | F7 |
pd.concat([df1, df4], ignore_index=True, sort=False)
A | B | C | D | F | |
---|---|---|---|---|---|
0 | A0 | B0 | C0 | D0 | NaN |
1 | A1 | B1 | C1 | D1 | NaN |
2 | A2 | B2 | C2 | D2 | NaN |
3 | A3 | B3 | C3 | D3 | NaN |
4 | NaN | B2 | NaN | D2 | F2 |
5 | NaN | B3 | NaN | D3 | F3 |
6 | NaN | B6 | NaN | D6 | F6 |
7 | NaN | B7 | NaN | D7 | F7 |
有没有类似于数据库中的outer join
呢?
pd.concat([df1, df4], axis=1, sort=False)
A | B | C | D | B | D | F | |
---|---|---|---|---|---|---|---|
0 | A0 | B0 | C0 | D0 | NaN | NaN | NaN |
1 | A1 | B1 | C1 | D1 | NaN | NaN | NaN |
2 | A2 | B2 | C2 | D2 | B2 | D2 | F2 |
3 | A3 | B3 | C3 | D3 | B3 | D3 | F3 |
6 | NaN | NaN | NaN | NaN | B6 | D6 | F6 |
7 | NaN | NaN | NaN | NaN | B7 | D7 | F7 |
很自然联想到inner join
:
pd.concat([df1, df4], axis=1, join='inner')
A | B | C | D | B | D | F | |
---|---|---|---|---|---|---|---|
2 | A2 | B2 | C2 | D2 | B2 | D2 | F2 |
3 | A3 | B3 | C3 | D3 | B3 | D3 | F3 |
join : {'inner', 'outer'}, default 'outer'
How to handle indexes on other axis (or axes).
这里并没有看到left join
或者right join
,那么如何达到left join
的效果呢?
pd.concat([df1, df4.reindex(df1.index)], axis=1)
A | B | C | D | B | D | F | |
---|---|---|---|---|---|---|---|
0 | A0 | B0 | C0 | D0 | NaN | NaN | NaN |
1 | A1 | B1 | C1 | D1 | NaN | NaN | NaN |
2 | A2 | B2 | C2 | D2 | B2 | D2 | F2 |
3 | A3 | B3 | C3 | D3 | B3 | D3 | F3 |
与序列合并:
s1 = pd.Series(['X0', 'X1', 'X2', 'X3'], name='X')
pd.concat([df1, s1], axis=1)
A | B | C | D | X | |
---|---|---|---|---|---|
0 | A0 | B0 | C0 | D0 | X0 |
1 | A1 | B1 | C1 | D1 | X1 |
2 | A2 | B2 | C2 | D2 | X2 |
3 | A3 | B3 | C3 | D3 | X3 |
当然,也是可以使用df.assign()
来定义一个新列。
如果序列没名称,会自动给自然索引名称,如下:
s2 = pd.Series(['_A', '_B', '_C', '_D'])
s3 = pd.Series(['_a', '_b', '_c', '_d'])
pd.concat([df1, s2, s3], axis=1)
A | B | C | D | 0 | 1 | |
---|---|---|---|---|---|---|
0 | A0 | B0 | C0 | D0 | _A | _a |
1 | A1 | B1 | C1 | D1 | _B | _b |
2 | A2 | B2 | C2 | D2 | _C | _c |
3 | A3 | B3 | C3 | D3 | _D | _d |
ignore_index=True
会取消原有列名:
pd.concat([df1, s1], axis=1, ignore_index=True)
0 | 1 | 2 | 3 | 4 | |
---|---|---|---|---|---|
0 | A0 | B0 | C0 | D0 | X0 |
1 | A1 | B1 | C1 | D1 | X1 |
2 | A2 | B2 | C2 | D2 | X2 |
3 | A3 | B3 | C3 | D3 | X3 |
同理,多个Series
也可以合并:
s3 = pd.Series(['李寻欢', '令狐冲', '张无忌', '花无缺'])
s4 = pd.Series(['多情剑客无情剑', '笑傲江湖', '倚天屠龙记', '绝代双骄'])
s5 = pd.Series(['小李飞刀', '独孤九剑', '九阳神功', '移花接玉'])
pd.concat([s3, s4, s5], axis=1)
0 | 1 | 2 | |
---|---|---|---|
0 | 李寻欢 | 多情剑客无情剑 | 小李飞刀 |
1 | 令狐冲 | 笑傲江湖 | 独孤九剑 |
2 | 张无忌 | 倚天屠龙记 | 九阳神功 |
3 | 花无缺 | 绝代双骄 | 移花接玉 |
也可以指定keys
使用新的列名:
pd.concat([s3, s4, s5], axis=1, keys=['name', 'book', 'skill'])
name | book | skill | |
---|---|---|---|
0 | 李寻欢 | 多情剑客无情剑 | 小李飞刀 |
1 | 令狐冲 | 笑傲江湖 | 独孤九剑 |
2 | 张无忌 | 倚天屠龙记 | 九阳神功 |
3 | 花无缺 | 绝代双骄 | 移花接玉 |
Signature:
pd.merge(
left,
right,
how: str = 'inner',
on=None,
left_on=None,
right_on=None,
left_index: bool = False,
right_index: bool = False,
sort: bool = False,
suffixes=('_x', '_y'),
copy: bool = True,
indicator: bool = False,
validate=None,
) -> 'DataFrame'
Docstring:
Merge DataFrame or named Series objects with a database-style join.
The join is done on columns or indexes. If joining columns on
columns, the DataFrame indexes *will be ignored*. Otherwise if joining indexes
on indexes or indexes on a column or columns, the index will be passed on.
Parameters
----------
left : DataFrame
right : DataFrame or named Series
Object to merge with.
how : {'left', 'right', 'outer', 'inner'}, default 'inner'
Type of merge to be performed.
* left: use only keys from left frame, similar to a SQL left outer join;
preserve key order.
* right: use only keys from right frame, similar to a SQL right outer join;
preserve key order.
* outer: use union of keys from both frames, similar to a SQL full outer
join; sort keys lexicographically.
* inner: use intersection of keys from both frames, similar to a SQL inner
join; preserve the order of the left keys.
on : label or list
Column or index level names to join on. These must be found in both
DataFrames. If `on` is None and not merging on indexes then this defaults
to the intersection of the columns in both DataFrames.
left_on : label or list, or array-like
Column or index level names to join on in the left DataFrame. Can also
be an array or list of arrays of the length of the left DataFrame.
These arrays are treated as if they are columns.
right_on : label or list, or array-like
Column or index level names to join on in the right DataFrame. Can also
be an array or list of arrays of the length of the right DataFrame.
These arrays are treated as if they are columns.
left_index : bool, default False
Use the index from the left DataFrame as the join key(s). If it is a
MultiIndex, the number of keys in the other DataFrame (either the index
or a number of columns) must match the number of levels.
right_index : bool, default False
Use the index from the right DataFrame as the join key. Same caveats as
left_index.
sort : bool, default False
Sort the join keys lexicographically in the result DataFrame. If False,
the order of the join keys depends on the join type (how keyword).
suffixes : list-like, default is ("_x", "_y")
A length-2 sequence where each element is optionally a string
indicating the suffix to add to overlapping column names in
`left` and `right` respectively. Pass a value of `None` instead
of a string to indicate that the column name from `left` or
`right` should be left as-is, with no suffix. At least one of the
values must not be None.
copy : bool, default True
If False, avoid copy if possible.
indicator : bool or str, default False
If True, adds a column to the output DataFrame called "_merge" with
information on the source of each row. The column can be given a different
name by providing a string argument. The column will have a Categorical
type with the value of "left_only" for observations whose merge key only
appears in the left DataFrame, "right_only" for observations
whose merge key only appears in the right DataFrame, and "both"
if the observation's merge key is found in both DataFrames.
validate : str, optional
If specified, checks if merge is of specified type.
* "one_to_one" or "1:1": check if merge keys are unique in both
left and right datasets.
* "one_to_many" or "1:m": check if merge keys are unique in left
dataset.
* "many_to_one" or "m:1": check if merge keys are unique in right
dataset.
* "many_to_many" or "m:m": allowed, but does not result in checks.
这里的merge
与关系型数据库中的join
非常类似。下面根据实例看看如何使用。
on
:根据某个字段进行连接,必须存在于两个DateFrame中(若未同时存在,则需要分别使用left_on
和right_on
来设置)
left = pd.DataFrame({'key': ['K0', 'K1', 'K2', 'K3'],
'A': ['A0', 'A1', 'A2', 'A3'],
'B': ['B0', 'B1', 'B2', 'B3']})
right = pd.DataFrame({'key': ['K0', 'K1', 'K2', 'K3'],
'C': ['C0', 'C1', 'C2', 'C3'],
'D': ['D0', 'D1', 'D2', 'D3']})
pd.merge(left, right, on='key')
key | A | B | C | D | |
---|---|---|---|---|---|
0 | K0 | A0 | B0 | C0 | D0 |
1 | K1 | A1 | B1 | C1 | D1 |
2 | K2 | A2 | B2 | C2 | D2 |
3 | K3 | A3 | B3 | C3 | D3 |
也可以有多个连接键:
left = pd.DataFrame({'key1': ['K0', 'K0', 'K1', 'K2'],
'key2': ['K0', 'K1', 'K0', 'K1'],
'A': ['A0', 'A1', 'A2', 'A3'],
'B': ['B0', 'B1', 'B2', 'B3']})
right = pd.DataFrame({'key1': ['K0', 'K1', 'K1', 'K2'],
'key2': ['K0', 'K0', 'K0', 'K0'],
'C': ['C0', 'C1', 'C2', 'C3'],
'D': ['D0', 'D1', 'D2', 'D3']})
pd.merge(left, right, on=['key1', 'key2'])
key1 | key2 | A | B | C | D | |
---|---|---|---|---|---|---|
0 | K0 | K0 | A0 | B0 | C0 | D0 |
1 | K1 | K0 | A2 | B2 | C1 | D1 |
2 | K1 | K0 | A2 | B2 | C2 | D2 |
how
: 可以指定数据用哪种方式进行合并,没有的内容会为NaN
,默认值inner
上面没有指定how
,默认就是inner
,下面分别看看left
, right
, outer
的效果。
左外连接:
pd.merge(left, right, how='left', on=['key1', 'key2'])
key1 | key2 | A | B | C | D | |
---|---|---|---|---|---|---|
0 | K0 | K0 | A0 | B0 | C0 | D0 |
1 | K0 | K1 | A1 | B1 | NaN | NaN |
2 | K1 | K0 | A2 | B2 | C1 | D1 |
3 | K1 | K0 | A2 | B2 | C2 | D2 |
4 | K2 | K1 | A3 | B3 | NaN | NaN |
右外连接:
pd.merge(left, right, how='right', on=['key1', 'key2'])
key1 | key2 | A | B | C | D | |
---|---|---|---|---|---|---|
0 | K0 | K0 | A0 | B0 | C0 | D0 |
1 | K1 | K0 | A2 | B2 | C1 | D1 |
2 | K1 | K0 | A2 | B2 | C2 | D2 |
3 | K2 | K0 | NaN | NaN | C3 | D3 |
全外连接:
pd.merge(left, right, how='outer', on=['key1', 'key2'])
key1 | key2 | A | B | C | D | |
---|---|---|---|---|---|---|
0 | K0 | K0 | A0 | B0 | C0 | D0 |
1 | K0 | K1 | A1 | B1 | NaN | NaN |
2 | K1 | K0 | A2 | B2 | C1 | D1 |
3 | K1 | K0 | A2 | B2 | C2 | D2 |
4 | K2 | K1 | A3 | B3 | NaN | NaN |
5 | K2 | K0 | NaN | NaN | C3 | D3 |
如果设置indicator
为True
, 则会增加名为_merge
的一列,显示这列是如何而来,其中left_only
表示只在左表中, right_only
表示只在右表中, both
表示两个表中都有:
pd.merge(left, right, how='outer', on=['key1', 'key2'], indicator=True)
key1 | key2 | A | B | C | D | _merge | |
---|---|---|---|---|---|---|---|
0 | K0 | K0 | A0 | B0 | C0 | D0 | both |
1 | K0 | K1 | A1 | B1 | NaN | NaN | left_only |
2 | K1 | K0 | A2 | B2 | C1 | D1 | both |
3 | K1 | K0 | A2 | B2 | C2 | D2 | both |
4 | K2 | K1 | A3 | B3 | NaN | NaN | left_only |
5 | K2 | K0 | NaN | NaN | C3 | D3 | right_only |
如果左、右两边连接的字段名称不同时,可以分别设置left_on
、right_on
:
left = pd.DataFrame({'key1': ['K0', 'K1', 'K2', 'K3'],
'A': ['A0', 'A1', 'A2', 'A3'],
'B': ['B0', 'B1', 'B2', 'B3']})
right = pd.DataFrame({'key2': ['K0', 'K1', 'K2', 'K3'],
'C': ['C0', 'C1', 'C2', 'C3'],
'D': ['D0', 'D1', 'D2', 'D3']})
pd.merge(left, right, left_on='key1', right_on='key2')
key1 | A | B | key2 | C | D | |
---|---|---|---|---|---|---|
0 | K0 | A0 | B0 | K0 | C0 | D0 |
1 | K1 | A1 | B1 | K1 | C1 | D1 |
2 | K2 | A2 | B2 | K2 | C2 | D2 |
3 | K3 | A3 | B3 | K3 | C3 | D3 |
非关联字段名称相同时,会怎样?
left = pd.DataFrame({'key1': ['K0', 'K1', 'K2', 'K3'],
'A': ['A0', 'A1', 'A2', 'A3'],
'B': ['B0', 'B1', 'B2', 'B3']})
right = pd.DataFrame({'key2': ['K0', 'K1', 'K2', 'K3'],
'C': ['C0', 'C1', 'C2', 'C3'],
'B': [30, 50, 70, 90]})
pd.merge(left, right, left_on='key1', right_on='key2')
key1 | A | B_x | key2 | C | B_y | |
---|---|---|---|---|---|---|
0 | K0 | A0 | B0 | K0 | C0 | 30 |
1 | K1 | A1 | B1 | K1 | C1 | 50 |
2 | K2 | A2 | B2 | K2 | C2 | 70 |
3 | K3 | A3 | B3 | K3 | C3 | 90 |
默认suffixes=('_x', '_y')
,也可以自行修改:
pd.merge(left, right, left_on='key1', right_on='key2',
suffixes=('_left', '_right'))
key1 | A | B_left | key2 | C | B_right | |
---|---|---|---|---|---|---|
0 | K0 | A0 | B0 | K0 | C0 | 30 |
1 | K1 | A1 | B1 | K1 | C1 | 50 |
2 | K2 | A2 | B2 | K2 | C2 | 70 |
3 | K3 | A3 | B3 | K3 | C3 | 90 |
append
可以追加数据,并返回一个新对象,也是一种简单常用的数据合并方式。
Signature:
df.append(other, ignore_index=False, verify_integrity=False, sort=False) -> 'DataFrame'
Docstring:
Append rows of `other` to the end of caller, returning a new object.
参数解释:
other
: 要追加的其他DataFrame
或Series
ignore_index
: 如果为True
则重新进行自然索引verify_integrity
: 如果为True
则遇到重复索引内容时报错sort
: 是否进行排序
追加同结构的数据:
df1.append(df2)
A | B | C | D | |
---|---|---|---|---|
0 | A0 | B0 | C0 | D0 |
1 | A1 | B1 | C1 | D1 |
2 | A2 | B2 | C2 | D2 |
3 | A3 | B3 | C3 | D3 |
4 | A4 | B4 | C4 | D4 |
5 | A5 | B5 | C5 | D5 |
6 | A6 | B6 | C6 | D6 |
7 | A7 | B7 | C7 | D7 |
追加不同结构的数据,没有的列会增加,没有对应内容的会为NAN
:
df1.append(df4, sort=False)
A | B | C | D | F | |
---|---|---|---|---|---|
0 | A0 | B0 | C0 | D0 | NaN |
1 | A1 | B1 | C1 | D1 | NaN |
2 | A2 | B2 | C2 | D2 | NaN |
3 | A3 | B3 | C3 | D3 | NaN |
2 | NaN | B2 | NaN | D2 | F2 |
3 | NaN | B3 | NaN | D3 | F3 |
6 | NaN | B6 | NaN | D6 | F6 |
7 | NaN | B7 | NaN | D7 | F7 |
追加多个DataFrame
:
df1.append([df2, df3])
A | B | C | D | |
---|---|---|---|---|
0 | A0 | B0 | C0 | D0 |
1 | A1 | B1 | C1 | D1 |
2 | A2 | B2 | C2 | D2 |
3 | A3 | B3 | C3 | D3 |
4 | A4 | B4 | C4 | D4 |
5 | A5 | B5 | C5 | D5 |
6 | A6 | B6 | C6 | D6 |
7 | A7 | B7 | C7 | D7 |
8 | A8 | B8 | C8 | D8 |
9 | A9 | B9 | C9 | D9 |
10 | A10 | B10 | C10 | D10 |
11 | A11 | B11 | C11 | D11 |
忽略原索引:
df1.append(df4, ignore_index=True, sort=False)
A | B | C | D | F | |
---|---|---|---|---|---|
0 | A0 | B0 | C0 | D0 | NaN |
1 | A1 | B1 | C1 | D1 | NaN |
2 | A2 | B2 | C2 | D2 | NaN |
3 | A3 | B3 | C3 | D3 | NaN |
4 | NaN | B2 | NaN | D2 | F2 |
5 | NaN | B3 | NaN | D3 | F3 |
6 | NaN | B6 | NaN | D6 | F6 |
7 | NaN | B7 | NaN | D7 | F7 |
追加Series
:
s2 = pd.Series(['X0', 'X1', 'X2', 'X3'],
index=['A', 'B', 'C', 'D'])
df1.append(s2, ignore_index=True)
A | B | C | D | |
---|---|---|---|---|
0 | A0 | B0 | C0 | D0 |
1 | A1 | B1 | C1 | D1 |
2 | A2 | B2 | C2 | D2 |
3 | A3 | B3 | C3 | D3 |
4 | X0 | X1 | X2 | X3 |
追加字典列表:
d = [{'A': 1, 'B': 2, 'C': 3, 'X': 4},
{'A': 5, 'B': 6, 'C': 7, 'Y': 8}]
df1.append(d, ignore_index=True, sort=False)
A | B | C | D | X | Y | |
---|---|---|---|---|---|---|
0 | A0 | B0 | C0 | D0 | NaN | NaN |
1 | A1 | B1 | C1 | D1 | NaN | NaN |
2 | A2 | B2 | C2 | D2 | NaN | NaN |
3 | A3 | B3 | C3 | D3 | NaN | NaN |
4 | 1 | 2 | 3 | NaN | 4.0 | NaN |
5 | 5 | 6 | 7 | NaN | NaN | 8.0 |
来个实战案例。在使用Excel
的时候,常常会在数据最后,增加一行汇总数据,比如求和,求平均值等。现在用Pandas
如何实现呢?
df = pd.DataFrame(np.random.randint(1, 10, size=(3, 4)),
columns=['a', 'b', 'c', 'd'])
df
a | b | c | d | |
---|---|---|---|---|
0 | 8 | 5 | 6 | 1 |
1 | 6 | 2 | 8 | 5 |
2 | 7 | 2 | 2 | 3 |
df.append(pd.Series(df.sum(), name='total'))
a | b | c | d | |
---|---|---|---|---|
0 | 8 | 5 | 6 | 1 |
1 | 6 | 2 | 8 | 5 |
2 | 7 | 2 | 2 | 3 |
total | 21 | 9 | 16 | 9 |
数据清洗是指发现并纠正数据集中可识别的错误的一个过程,包括检查数据一致性,处理无效值、缺失值等。数据清洗是为了最大限度地提高数据集的准确性。
由于数据来源的复杂性、不确定性,数据中难免会存在字段值不全、缺失等情况,下面先介绍如何找出这些缺失的值。
以这里的电影数据举例,其中数据集来自github,为了方便测试,下载压缩后上传到博客园,原始数据链接:
https://github.com/LearnDataSci/articles/blob/master/Python%20Pandas%20Tutorial%20A%20Complete%20Introduction%20for%20Beginners/IMDB-Movie-Data.csv
movies = pd.read_csv('https://files.cnblogs.com/files/blogs/478024/IMDB-Movie-Data.csv.zip')
movies.tail(2)
Rank | Title | Genre | Description | Director | Actors | Year | Runtime (Minutes) | Rating | Votes | Revenue (Millions) | Metascore | |
---|---|---|---|---|---|---|---|---|---|---|---|---|
998 | 999 | Search Party | Adventure,Comedy | A pair of friends embark on a mission to reuni... | Scot Armstrong | Adam Pally, T.J. Miller, Thomas Middleditch,Sh... | 2014 | 93 | 5.6 | 4881 | NaN | 22.0 |
999 | 1000 | Nine Lives | Comedy,Family,Fantasy | A stuffy businessman finds himself trapped ins... | Barry Sonnenfeld | Kevin Spacey, Jennifer Garner, Robbie Amell,Ch... | 2016 | 87 | 5.3 | 12435 | 19.64 | 11.0 |
上面可以看到Revenue (Millions)
列有个NaN
,这里的NaN
是一个缺值标识。
NaN (not a number) is the standard missing data marker used in pandas.
判断数据集中是否有缺失值:
np.any(movies.isna())
True
或者判断数据集中是否不含缺失值:
np.all(movies.notna())
False
统计 每列有多少个缺失值:
movies.isna().sum(axis=0)
Rank 0 Title 0 Genre 0 Description 0 Director 0 Actors 0 Year 0 Runtime (Minutes) 0 Rating 0 Votes 0 Revenue (Millions) 128 Metascore 64 dtype: int64
统计 每行有多少个缺失值:
movies.isna().sum(axis=1)
0 0 1 0 2 0 3 0 4 0 .. 995 1 996 0 997 0 998 1 999 0 Length: 1000, dtype: int64
统计 一共有多少个缺失值:
movies.isna().sum().sum()
192
筛选出有缺失值的列:
movies.isnull().any(axis=0)
Rank False Title False Genre False Description False Director False Actors False Year False Runtime (Minutes) False Rating False Votes False Revenue (Millions) True Metascore True dtype: bool
统计有缺失值的列的个数:
movies.isnull().any(axis=0).sum()
2
筛选出有缺失值的行:
movies.isnull().any(axis=1)
0 False 1 False 2 False 3 False 4 False ... 995 True 996 False 997 False 998 True 999 False Length: 1000, dtype: bool
统计有缺失值的行的个数:
movies.isnull().any(axis=1).sum()
162
查看Metascore
列缺失的数据:
movies[movies['Metascore'].isna()][['Rank', 'Title', 'Votes', 'Metascore']]
Rank | Title | Votes | Metascore | |
---|---|---|---|---|
25 | 26 | Paris pieds nus | 222 | NaN |
26 | 27 | Bahubali: The Beginning | 76193 | NaN |
27 | 28 | Dead Awake | 523 | NaN |
39 | 40 | 5- 25- 77 | 241 | NaN |
42 | 43 | Don't Fuck in the Woods | 496 | NaN |
... | ... | ... | ... | ... |
967 | 968 | The Walk | 92378 | NaN |
969 | 970 | The Lone Ranger | 190855 | NaN |
971 | 972 | Disturbia | 193491 | NaN |
989 | 990 | Selma | 67637 | NaN |
992 | 993 | Take Me Home Tonight | 45419 | NaN |
64 rows × 4 columns
movies.shape
(1000, 12)
movies.count()
Rank 1000 Title 1000 Genre 1000 Description 1000 Director 1000 Actors 1000 Year 1000 Runtime (Minutes) 1000 Rating 1000 Votes 1000 Revenue (Millions) 872 Metascore 936 dtype: int64
可以看出,每列应该有1000个数据的,count()
时缺失值并没有算进来。
对于缺失值的处理,应根据具体的业务场景以及数据完整性的要求选择较合适的方案。常见的处理方案包括删除存在缺失值的数据(dropna
)、替换缺失值(fillna
)。
某些场景下,有缺失值会认为该样本数据无效,就需要对整行或者整列数据进行删除(dropna
)。
统计无缺失值的行的个数:
movies.notna().all(axis=1).sum()
838
删除所有含缺失值的行:
data = movies.dropna()
data.shape
(838, 12)
movies.shape
(1000, 12)
data
里838行数据都是无缺失值的。但是,值得注意的是,dropna()
默认并不会在原来的数据集上删除,除非指定dropna(inplace=True)
。下面演示一下:
# 复制一份完整的数据做原地删除演示
movies_copy = movies.copy()
print(movies_copy.shape)
# 原地删除
movies_copy.dropna(inplace=True)
# 查看原地删除是否生效
movies_copy.shape
(1000, 12)
(838, 12)
统计无缺失值的列的个数:
movies.notna().all(axis=0).sum()
10
删除含缺失值的列:
data = movies.dropna(axis=1)
data.shape
(1000, 10)
how : {'any', 'all'}, default 'any'. Determine if row or column is removed from DataFrame, when we have at least one NA or all NA.
- 'any' : If any NA values are present, drop that row or column.
- 'all' : If all values are NA, drop that row or column.
删除所有值都缺失的行:
# Drop the rows where all elements are missing
data = movies.dropna(how='all')
data.shape
(1000, 12)
这里的数据不存在所有值都缺失的行,所以how='all'
时dropna()
对此处的数据集无任何影响。
subset: Define in which columns to look for missing values.
subset
参数可以指定在哪些列中寻找缺失值:
# 指定在Title、Metascore两列中寻找缺失值
data = movies.dropna(subset=['Title', 'Metascore'])
data.shape
(936, 12)
统计Title
、Metascore
这两列无缺失值的行数。讲道理,肯定是等于上面删除缺失值后的行数。
movies[['Title', 'Metascore']].notna().all(axis=1).sum()
936
thresh : int, optional. Require that many non-NA values.
# Keep only the rows with at least 2 non-NA values.
data = movies[['Title', 'Metascore', 'Revenue (Millions)']].dropna(thresh=2)
data.shape
(970, 3)
由于Title
列没有缺失值,相当于删除掉Metascore
,Revenue (Millions)
两列都为缺失值的行,如下:
data = movies[['Metascore', 'Revenue (Millions)']].dropna(how='all')
data.shape
(970, 2)
处理缺失值的另外一种常用方法是填充(fillna
)。填充值虽然不绝对准确,但对获得真实结果的影响并不大时,可以尝试一用。
先看个简单的例子,然后再应用到上面的电影数据中去。因为电影数据比较多,演示起来并不直观。
hero = pd.DataFrame(data={'score': [97, np.nan, 96, np.nan, 95],
'wins': [np.nan, 9, np.nan, 11, 10],
'author': ['古龙', '金庸', np.nan, np.nan, np.nan],
'book': ['多情剑客无情剑', '笑傲江湖', '倚天屠龙记', '射雕英雄传', '绝代双骄'],
'skill': ['小李飞刀', '独孤九剑', '九阳神功', '降龙十八掌', '移花接玉'],
'wife': [np.nan, '任盈盈', np.nan, '黄蓉', np.nan],
'child': [np.nan, np.nan, np.nan, '郭襄', np.nan]},
index=['李寻欢', '令狐冲', '张无忌', '郭靖', '花无缺'])
hero
score | wins | author | book | skill | wife | child | |
---|---|---|---|---|---|---|---|
李寻欢 | 97.0 | NaN | 古龙 | 多情剑客无情剑 | 小李飞刀 | NaN | NaN |
令狐冲 | NaN | 9.0 | 金庸 | 笑傲江湖 | 独孤九剑 | 任盈盈 | NaN |
张无忌 | 96.0 | NaN | NaN | 倚天屠龙记 | 九阳神功 | NaN | NaN |
郭靖 | NaN | 11.0 | NaN | 射雕英雄传 | 降龙十八掌 | 黄蓉 | 郭襄 |
花无缺 | 95.0 | 10.0 | NaN | 绝代双骄 | 移花接玉 | NaN | NaN |
全部填充为unknown
:
hero.fillna('unknown')
score | wins | author | book | skill | wife | child | |
---|---|---|---|---|---|---|---|
李寻欢 | 97 | unknown | 古龙 | 多情剑客无情剑 | 小李飞刀 | unknown | unknown |
令狐冲 | unknown | 9 | 金庸 | 笑傲江湖 | 独孤九剑 | 任盈盈 | unknown |
张无忌 | 96 | unknown | unknown | 倚天屠龙记 | 九阳神功 | unknown | unknown |
郭靖 | unknown | 11 | unknown | 射雕英雄传 | 降龙十八掌 | 黄蓉 | 郭襄 |
花无缺 | 95 | 10 | unknown | 绝代双骄 | 移花接玉 | unknown | unknown |
只替换第一个:
hero.fillna('unknown', limit=1)
score | wins | author | book | skill | wife | child | |
---|---|---|---|---|---|---|---|
李寻欢 | 97 | unknown | 古龙 | 多情剑客无情剑 | 小李飞刀 | unknown | unknown |
令狐冲 | unknown | 9 | 金庸 | 笑傲江湖 | 独孤九剑 | 任盈盈 | NaN |
张无忌 | 96 | NaN | unknown | 倚天屠龙记 | 九阳神功 | NaN | NaN |
郭靖 | NaN | 11 | NaN | 射雕英雄传 | 降龙十八掌 | 黄蓉 | 郭襄 |
花无缺 | 95 | 10 | NaN | 绝代双骄 | 移花接玉 | NaN | NaN |
不同列替换不同的值:
hero.fillna(value={'score': 100, 'author': '匿名', 'wife': '保密'})
score | wins | author | book | skill | wife | child | |
---|---|---|---|---|---|---|---|
李寻欢 | 97.0 | NaN | 古龙 | 多情剑客无情剑 | 小李飞刀 | 保密 | NaN |
令狐冲 | 100.0 | 9.0 | 金庸 | 笑傲江湖 | 独孤九剑 | 任盈盈 | NaN |
张无忌 | 96.0 | NaN | 匿名 | 倚天屠龙记 | 九阳神功 | 保密 | NaN |
郭靖 | 100.0 | 11.0 | 匿名 | 射雕英雄传 | 降龙十八掌 | 黄蓉 | 郭襄 |
花无缺 | 95.0 | 10.0 | 匿名 | 绝代双骄 | 移花接玉 | 保密 | NaN |
method : {'backfill', 'bfill', 'pad', 'ffill', None}, default None
上面是填充固定值,此外还能指定填充方法。
pad / ffill: propagate last valid observation forward to next valid
# 使用前一个有效值填充
hero.fillna(method='ffill')
score | wins | author | book | skill | wife | child | |
---|---|---|---|---|---|---|---|
李寻欢 | 97.0 | NaN | 古龙 | 多情剑客无情剑 | 小李飞刀 | NaN | NaN |
令狐冲 | 97.0 | 9.0 | 金庸 | 笑傲江湖 | 独孤九剑 | 任盈盈 | NaN |
张无忌 | 96.0 | 9.0 | 金庸 | 倚天屠龙记 | 九阳神功 | 任盈盈 | NaN |
郭靖 | 96.0 | 11.0 | 金庸 | 射雕英雄传 | 降龙十八掌 | 黄蓉 | 郭襄 |
花无缺 | 95.0 | 10.0 | 金庸 | 绝代双骄 | 移花接玉 | 黄蓉 | 郭襄 |
backfill / bfill: use next valid observation to fill gap.
# 使用后一个有效值填充
hero.fillna(method='bfill')
score | wins | author | book | skill | wife | child | |
---|---|---|---|---|---|---|---|
李寻欢 | 97.0 | 9.0 | 古龙 | 多情剑客无情剑 | 小李飞刀 | 任盈盈 | 郭襄 |
令狐冲 | 96.0 | 9.0 | 金庸 | 笑傲江湖 | 独孤九剑 | 任盈盈 | 郭襄 |
张无忌 | 96.0 | 11.0 | NaN | 倚天屠龙记 | 九阳神功 | 黄蓉 | 郭襄 |
郭靖 | 95.0 | 11.0 | NaN | 射雕英雄传 | 降龙十八掌 | 黄蓉 | 郭襄 |
花无缺 | 95.0 | 10.0 | NaN | 绝代双骄 | 移花接玉 | NaN | NaN |
对score
、wins
两列的缺失值用平均值来填充:
hero.fillna(hero[['score', 'wins']].mean())
score | wins | author | book | skill | wife | child | |
---|---|---|---|---|---|---|---|
李寻欢 | 97.0 | 10.0 | 古龙 | 多情剑客无情剑 | 小李飞刀 | NaN | NaN |
令狐冲 | 96.0 | 9.0 | 金庸 | 笑傲江湖 | 独孤九剑 | 任盈盈 | NaN |
张无忌 | 96.0 | 10.0 | NaN | 倚天屠龙记 | 九阳神功 | NaN | NaN |
郭靖 | 96.0 | 11.0 | NaN | 射雕英雄传 | 降龙十八掌 | 黄蓉 | 郭襄 |
花无缺 | 95.0 | 10.0 | NaN | 绝代双骄 | 移花接玉 | NaN | NaN |
指定列填充为unknown
,并原地替换:
hero.child.fillna('unknown', inplace=True)
hero
score | wins | author | book | skill | wife | child | |
---|---|---|---|---|---|---|---|
李寻欢 | 97.0 | NaN | 古龙 | 多情剑客无情剑 | 小李飞刀 | NaN | unknown |
令狐冲 | NaN | 9.0 | 金庸 | 笑傲江湖 | 独孤九剑 | 任盈盈 | unknown |
张无忌 | 96.0 | NaN | NaN | 倚天屠龙记 | 九阳神功 | NaN | unknown |
郭靖 | NaN | 11.0 | NaN | 射雕英雄传 | 降龙十八掌 | 黄蓉 | 郭襄 |
花无缺 | 95.0 | 10.0 | NaN | 绝代双骄 | 移花接玉 | NaN | unknown |
再回到上面的实际的电影数据案例。现在用平均值替换缺失值:
filled_movies = movies.fillna(
movies[['Revenue (Millions)', 'Metascore']].mean())
np.any(filled_movies.isna())
False
可见,填充后的电影数据中已经不存在缺失值了。
数据替换常用于数据清洗整理、枚举转换、数据修正等场景。
先看下replace()
方法的介绍:
Signature:
replace(
to_replace=None,
value=None,
inplace=False,
limit=None,
regex=False,
method='pad',
)
Docstring:
Replace values given in `to_replace` with `value`.
再看几个例子:
s = pd.Series(['a', 'b', 'c', 'd', 'e'])
s
0 a 1 b 2 c 3 d 4 e dtype: object
s.replace('a', 'aa')
0 aa 1 b 2 c 3 d 4 e dtype: object
s.replace({'d': 'dd', 'e': 'ee'})
0 a 1 b 2 c 3 dd 4 ee dtype: object
s.replace(['a', 'b', 'c'], ['aa', 'bb', 'cc'])
0 aa 1 bb 2 cc 3 d 4 e dtype: object
# 将c替换为它前一个值
s.replace('c', method='ffill')
0 a 1 b 2 b 3 d 4 e dtype: object
# 将c替换为它后一个值
s.replace('c', method='bfill')
0 a 1 b 2 d 3 d 4 e dtype: object
df = pd.DataFrame({'A': [0, -11, 2, 3, 35],
'B': [0, -20, 2, 5, 16]})
df
A | B | |
---|---|---|
0 | 0 | 0 |
1 | -11 | -20 |
2 | 2 | 2 |
3 | 3 | 5 |
4 | 35 | 16 |
df.replace(0, 5)
A | B | |
---|---|---|
0 | 5 | 5 |
1 | -11 | -20 |
2 | 2 | 2 |
3 | 3 | 5 |
4 | 35 | 16 |
df.replace([0, 2, 3, 5], 10)
A | B | |
---|---|---|
0 | 10 | 10 |
1 | -11 | -20 |
2 | 10 | 10 |
3 | 10 | 10 |
4 | 35 | 16 |
df.replace([0, 2], [100, 200])
A | B | |
---|---|---|
0 | 100 | 100 |
1 | -11 | -20 |
2 | 200 | 200 |
3 | 3 | 5 |
4 | 35 | 16 |
df.replace({0: 10, 2: 22})
A | B | |
---|---|---|
0 | 10 | 10 |
1 | -11 | -20 |
2 | 22 | 22 |
3 | 3 | 5 |
4 | 35 | 16 |
df.replace({'A': 0, 'B': 2}, 100)
A | B | |
---|---|---|
0 | 100 | 0 |
1 | -11 | -20 |
2 | 2 | 100 |
3 | 3 | 5 |
4 | 35 | 16 |
df.replace({'A': {2: 200, 3: 300}})
A | B | |
---|---|---|
0 | 0 | 0 |
1 | -11 | -20 |
2 | 200 | 2 |
3 | 300 | 5 |
4 | 35 | 16 |
对一些极端值,如过大或者过小,可以使用df.clip(lower, upper)
来修剪,当数据大于upper
时,使用upper
的值,小于lower
时用lower
的值,类似numpy.clip
的方法。
在修剪之前,再看一眼原始数据:
df
A | B | |
---|---|---|
0 | 0 | 0 |
1 | -11 | -20 |
2 | 2 | 2 |
3 | 3 | 5 |
4 | 35 | 16 |
# 修剪成最小为2,最大为10
df.clip(2, 10)
A | B | |
---|---|---|
0 | 2 | 2 |
1 | 2 | 2 |
2 | 2 | 2 |
3 | 3 | 5 |
4 | 10 | 10 |
# 对每列元素的最小值和最大值进行不同的限制
# 将A列数值修剪成[-3, 3]之间
# 将B列数值修剪成[-5, 5]之间
df.clip([-3, -5], [3, 5], axis=1)
A | B | |
---|---|---|
0 | 0 | 0 |
1 | -3 | -5 |
2 | 2 | 2 |
3 | 3 | 5 |
4 | 3 | 5 |
# 对每行元素的最小值和最大值进行不同的限制
# 将第1行数值修剪成[5, 10]之间
# 将第2行数值修剪成[-15, -12]之间
# 将第3行数值修剪成[6, 10]之间
# 将第4行数值修剪成[4, 10]之间
# 将第5行数值修剪成[20, 30]之间
df.clip([5, -15, 6, 4, 20],
[10, -12, 10, 10, 30],
axis=0)
A | B | |
---|---|---|
0 | 5 | 5 |
1 | -12 | -15 |
2 | 6 | 6 |
3 | 4 | 5 |
4 | 30 | 20 |
另外,可以将无效值先替换为nan
,再做缺失值处理。这样就能应用上前面讲到的缺失值处理相关的知识。
比如这里的df
,我们认为小于0的数据都是无效数据,可以:
df.replace([-11, -20], np.nan)
A | B | |
---|---|---|
0 | 0.0 | 0.0 |
1 | NaN | NaN |
2 | 2.0 | 2.0 |
3 | 3.0 | 5.0 |
4 | 35.0 | 16.0 |
当然,也可以像下面这样把无效数据变为nan
:
df[df >= 0]
A | B | |
---|---|---|
0 | 0.0 | 0.0 |
1 | NaN | NaN |
2 | 2.0 | 2.0 |
3 | 3.0 | 5.0 |
4 | 35.0 | 16.0 |
此时,上面讲到的缺失值处理就能派上用场了。
文本内容比较复杂时,可以使用正则进行匹配替换。下面看几个例子:
df = pd.DataFrame({'A': ['bat', 'foo', 'bait'],
'B': ['abc', 'bar', 'xyz']})
df
A | B | |
---|---|---|
0 | bat | abc |
1 | foo | bar |
2 | bait | xyz |
# 利用正则将ba开头且总共3个字符的文本替换为new
df.replace(to_replace=r'^ba.$', value='new', regex=True)
A | B | |
---|---|---|
0 | new | abc |
1 | foo | new |
2 | bait | xyz |
# 如果多列正则不同的情况下可以按以下格式对应传入
df.replace({'A': r'^ba.$'}, {'A': 'new'}, regex=True)
A | B | |
---|---|---|
0 | new | abc |
1 | foo | bar |
2 | bait | xyz |
df.replace(regex=r'^ba.$', value='new')
A | B | |
---|---|---|
0 | new | abc |
1 | foo | new |
2 | bait | xyz |
# 不同正则替换不同的值
df.replace(regex={r'^ba.$': 'new', 'foo': 'xyz'})
A | B | |
---|---|---|
0 | new | abc |
1 | xyz | new |
2 | bait | xyz |
# 多个正则替换为同一个值
df.replace(regex=[r'^ba.$', 'foo'], value='new')
A | B | |
---|---|---|
0 | new | abc |
1 | new | new |
2 | bait | xyz |
重复值在数据清洗中可能需要删除。下面介绍Pandas如何识别重复值以及如何删除重复值。
Signature:
df.duplicated(
subset: Union[Hashable, Sequence[Hashable], NoneType] = None,
keep: Union[str, bool] = 'first',
) -> 'Series'
Docstring:
Return boolean Series denoting duplicate rows.
Considering certain columns is optional.
Parameters
----------
subset : column label or sequence of labels, optional
Only consider certain columns for identifying duplicates, by
default use all of the columns.
keep : {'first', 'last', False}, default 'first'
Determines which duplicates (if any) to mark.
- ``first`` : Mark duplicates as ``True`` except for the first occurrence.
- ``last`` : Mark duplicates as ``True`` except for the last occurrence.
- False : Mark all duplicates as ``True``.
看官方给的例子:
df = pd.DataFrame({
'brand': ['Yum Yum', 'Yum Yum', 'Indomie', 'Indomie', 'Indomie'],
'style': ['cup', 'cup', 'cup', 'pack', 'pack'],
'rating': [4, 4, 3.5, 15, 5]
})
df
brand | style | rating | |
---|---|---|---|
0 | Yum Yum | cup | 4.0 |
1 | Yum Yum | cup | 4.0 |
2 | Indomie | cup | 3.5 |
3 | Indomie | pack | 15.0 |
4 | Indomie | pack | 5.0 |
# 默认情况下,对于每行重复的值,第一次出现都设置为False,其他为True
df.duplicated()
0 False 1 True 2 False 3 False 4 False dtype: bool
# 将每行重复值的最后一次出现设置为False,其他为True
df.duplicated(keep='last')
0 True 1 False 2 False 3 False 4 False dtype: bool
# 所有重复行都为True
df.duplicated(keep=False)
0 True 1 True 2 False 3 False 4 False dtype: bool
# 参数subset可以在指定列上查找重复值
df.duplicated(subset=['brand'])
0 False 1 True 2 False 3 True 4 True dtype: bool
再看如何删除重复值:
Signature:
df.drop_duplicates(
subset: Union[Hashable, Sequence[Hashable], NoneType] = None,
keep: Union[str, bool] = 'first',
inplace: bool = False,
ignore_index: bool = False,
) -> Union[ForwardRef('DataFrame'), NoneType]
Docstring:
Return DataFrame with duplicate rows removed.
Considering certain columns is optional. Indexes, including time indexes
are ignored.
Parameters
----------
subset : column label or sequence of labels, optional
Only consider certain columns for identifying duplicates, by
default use all of the columns.
keep : {'first', 'last', False}, default 'first'
Determines which duplicates (if any) to keep.
- ``first`` : Drop duplicates except for the first occurrence.
- ``last`` : Drop duplicates except for the last occurrence.
- False : Drop all duplicates.
inplace : bool, default False
Whether to drop duplicates in place or to return a copy.
ignore_index : bool, default False
If True, the resulting axis will be labeled 0, 1, …, n - 1.
同样继续官方给的例子:
# By default, it removes duplicate rows based on all columns
df.drop_duplicates()
brand | style | rating | |
---|---|---|---|
0 | Yum Yum | cup | 4.0 |
2 | Indomie | cup | 3.5 |
3 | Indomie | pack | 15.0 |
4 | Indomie | pack | 5.0 |
# To remove duplicates on specific column(s), use `subset`
df.drop_duplicates(subset=['brand'])
brand | style | rating | |
---|---|---|---|
0 | Yum Yum | cup | 4.0 |
2 | Indomie | cup | 3.5 |
# To remove duplicates and keep last occurences, use `keep`
df.drop_duplicates(subset=['brand', 'style'], keep='last')
brand | style | rating | |
---|---|---|---|
1 | Yum Yum | cup | 4.0 |
2 | Indomie | cup | 3.5 |
4 | Indomie | pack | 5.0 |
在数据统计与分析中,分组与聚合非常常见。如果是SQL,对应的就是Group By
和聚合函数(Aggregation Functions
)。下面看看pandas是怎么玩的。
Signature:
df.groupby(
by=None,
axis=0,
level=None,
as_index: bool = True,
sort: bool = True,
group_keys: bool = True,
squeeze: bool = <object object at 0x7f3df810e750>,
observed: bool = False,
dropna: bool = True,
) -> 'DataFrameGroupBy'
Docstring:
Group DataFrame using a mapper or by a Series of columns.
groupby()
方法可以按指定字段对DataFrame
进行分组,生成一个分组器对象,然后再把这个对象的各个字段按一定的聚合方法输出。
其中by
为分组字段,由于是第一个参数可以省略,可以按列表给多个。会返回一个DataFrameGroupBy
对象,如果不给聚合方法,不会返回 DataFrame
。
准备演示数据:
df = pd.read_csv('https://files.cnblogs.com/files/blogs/478024/team.csv.zip')
df
name | team | Q1 | Q2 | Q3 | Q4 | |
---|---|---|---|---|---|---|
0 | Liver | E | 89 | 21 | 24 | 64 |
1 | Arry | C | 36 | 37 | 37 | 57 |
2 | Ack | A | 57 | 60 | 18 | 84 |
3 | Eorge | C | 93 | 96 | 71 | 78 |
4 | Oah | D | 65 | 49 | 61 | 86 |
... | ... | ... | ... | ... | ... | ... |
95 | Gabriel | C | 48 | 59 | 87 | 74 |
96 | Austin7 | C | 21 | 31 | 30 | 43 |
97 | Lincoln4 | C | 98 | 93 | 1 | 20 |
98 | Eli | E | 11 | 74 | 58 | 91 |
99 | Ben | E | 21 | 43 | 41 | 74 |
100 rows × 6 columns
# 按team分组后对应列求和
df.groupby('team').sum()
Q1 | Q2 | Q3 | Q4 | |
---|---|---|---|---|
team | ||||
A | 1066 | 639 | 875 | 783 |
B | 975 | 1218 | 1202 | 1136 |
C | 1056 | 1194 | 1068 | 1127 |
D | 860 | 1191 | 1241 | 1199 |
E | 963 | 1013 | 881 | 1033 |
# 按team分组后对应列求平均值
df.groupby('team').mean()
Q1 | Q2 | Q3 | Q4 | |
---|---|---|---|---|
team | ||||
A | 62.705882 | 37.588235 | 51.470588 | 46.058824 |
B | 44.318182 | 55.363636 | 54.636364 | 51.636364 |
C | 48.000000 | 54.272727 | 48.545455 | 51.227273 |
D | 45.263158 | 62.684211 | 65.315789 | 63.105263 |
E | 48.150000 | 50.650000 | 44.050000 | 51.650000 |
# 按team分组后不同列使用不同的聚合方式
df.groupby('team').agg({'Q1': sum, # 求和
'Q2': 'count', # 计数
'Q3': 'mean', # 求平均值
'Q4': max}) # 求最大值
Q1 | Q2 | Q3 | Q4 | |
---|---|---|---|---|
team | ||||
A | 1066 | 17 | 51.470588 | 97 |
B | 975 | 22 | 54.636364 | 99 |
C | 1056 | 22 | 48.545455 | 98 |
D | 860 | 19 | 65.315789 | 99 |
E | 963 | 20 | 44.050000 | 98 |
If
by
is a function, it's called on each value of the object's index.
# team在C之前(包括C)分为一组,C之后的分为另外一组
df.set_index('team').groupby(lambda team: 'team1' if team <= 'C' else 'team2')['name'].count()
team1 61 team2 39 Name: name, dtype: int64
或者下面这种写法也行:
df.groupby(lambda idx: 'team1' if df.loc[idx]['team'] <= 'C' else 'team2')['name'].count()
team1 61 team2 39 Name: name, dtype: int64
# 按name的长度(length)分组,并取出每组中name的第一个值和最后一个值
df.groupby(df['name'].apply(lambda x: len(x))).agg({'name': ['first', 'last']})
name | ||
---|---|---|
first | last | |
name | ||
3 | Ack | Ben |
4 | Arry | Leon |
5 | Liver | Aiden |
6 | Harlie | Jamie0 |
7 | William | Austin7 |
8 | Harrison | Lincoln4 |
9 | Alexander | Theodore3 |
# 只对部分分组
df.set_index('team').groupby({'A': 'A组', 'B': 'B组'})['name'].count()
A组 17 B组 22 Name: name, dtype: int64
可以将以上方法混合组成列表进行分组:
# 按team,name长度分组,取分组中最后一行
df.groupby(['team', df['name'].apply(lambda x: len(x))]).last()
name | Q1 | Q2 | Q3 | Q4 | ||
---|---|---|---|---|---|---|
team | name | |||||
A | 3 | Ack | 57 | 60 | 18 | 84 |
4 | Toby | 52 | 27 | 17 | 68 | |
5 | Aaron | 96 | 75 | 55 | 8 | |
6 | Nathan | 87 | 77 | 62 | 13 | |
7 | Stanley | 69 | 71 | 39 | 97 | |
B | 3 | Kai | 66 | 45 | 13 | 48 |
4 | Liam | 2 | 80 | 24 | 25 | |
5 | Lewis | 4 | 34 | 77 | 28 | |
6 | Jamie0 | 39 | 97 | 84 | 55 | |
7 | Albert0 | 85 | 38 | 41 | 17 | |
8 | Grayson7 | 59 | 84 | 74 | 33 | |
C | 4 | Adam | 90 | 32 | 47 | 39 |
5 | Calum | 14 | 91 | 16 | 82 | |
6 | Connor | 62 | 38 | 63 | 46 | |
7 | Austin7 | 21 | 31 | 30 | 43 | |
8 | Lincoln4 | 98 | 93 | 1 | 20 | |
9 | Sebastian | 1 | 14 | 68 | 48 | |
D | 3 | Oah | 65 | 49 | 61 | 86 |
4 | Ezra | 16 | 56 | 86 | 61 | |
5 | Aiden | 20 | 31 | 62 | 68 | |
6 | Reuben | 70 | 72 | 76 | 56 | |
7 | Hunter3 | 38 | 80 | 82 | 40 | |
8 | Benjamin | 15 | 88 | 52 | 25 | |
9 | Theodore3 | 43 | 7 | 68 | 80 | |
E | 3 | Ben | 21 | 43 | 41 | 74 |
4 | Leon | 38 | 60 | 31 | 7 | |
5 | Roman | 73 | 1 | 25 | 44 | |
6 | Dexter | 73 | 94 | 53 | 20 | |
7 | Zachary | 12 | 71 | 85 | 93 | |
8 | Jackson5 | 6 | 10 | 15 | 33 |
We can groupby different levels of a hierarchical index using the
level
parameter.
arrays = [['Falcon', 'Falcon', 'Parrot', 'Parrot'],
['Captive', 'Wild', 'Captive', 'Wild']]
index = pd.MultiIndex.from_arrays(arrays, names=('Animal', 'Type'))
df = pd.DataFrame({'Max Speed': [390., 350., 30., 20.]},
index=index)
df
Max Speed | ||
---|---|---|
Animal | Type | |
Falcon | Captive | 390.0 |
Wild | 350.0 | |
Parrot | Captive | 30.0 |
Wild | 20.0 |
# df.groupby(level=0).mean()
df.groupby(level="Animal").mean()
Max Speed | |
---|---|
Animal | |
Falcon | 370.0 |
Parrot | 25.0 |
# df.groupby(level=1).mean()
df.groupby(level="Type").mean()
Max Speed | |
---|---|
Type | |
Captive | 210.0 |
Wild | 185.0 |
We can also choose to include NA in group keys or not by setting
dropna
parameter, the default setting isTrue
.
l = [[1, 2, 3], [1, None, 4], [2, 1, 3], [1, 2, 2]]
df = pd.DataFrame(l, columns=["a", "b", "c"])
df
a | b | c | |
---|---|---|---|
0 | 1 | 2.0 | 3 |
1 | 1 | NaN | 4 |
2 | 2 | 1.0 | 3 |
3 | 1 | 2.0 | 2 |
df.groupby(by=["b"]).sum()
a | c | |
---|---|---|
b | ||
1.0 | 2 | 3 |
2.0 | 2 | 5 |
df.groupby(by=["b"], dropna=False).sum()
a | c | |
---|---|---|
b | ||
1.0 | 2 | 3 |
2.0 | 2 | 5 |
NaN | 1 | 4 |
上面体验了一下pandas分组聚合的基本使用后,接下来看看分组聚合的一些过程细节。
有以下动物最大速度数据:
df = pd.DataFrame([('bird', 'Falconiformes', 389.0),
('bird', 'Psittaciformes', 24.0),
('mammal', 'Carnivora', 80.2),
('mammal', 'Primates', np.nan),
('mammal', 'Carnivora', 58)],
index=['falcon', 'parrot', 'lion',
'monkey', 'leopard'],
columns=('class', 'order', 'max_speed'))
df
class | order | max_speed | |
---|---|---|---|
falcon | bird | Falconiformes | 389.0 |
parrot | bird | Psittaciformes | 24.0 |
lion | mammal | Carnivora | 80.2 |
monkey | mammal | Primates | NaN |
leopard | mammal | Carnivora | 58.0 |
# 分组数
df.groupby('class').ngroups
2
# 查看分组
df.groupby('class').groups
{'bird': ['falcon', 'parrot'], 'mammal': ['lion', 'monkey', 'leopard']}
df.groupby('class').size()
class bird 2 mammal 3 dtype: int64
# 查看鸟类分组内容
df.groupby('class').get_group('bird')
class | order | max_speed | |
---|---|---|---|
falcon | bird | Falconiformes | 389.0 |
parrot | bird | Psittaciformes | 24.0 |
获取分组中的第几个值:
# 第一个
df.groupby('class').nth(1)
order | max_speed | |
---|---|---|
class | ||
bird | Psittaciformes | 24.0 |
mammal | Primates | NaN |
# 最后一个
df.groupby('class').nth(-1)
order | max_speed | |
---|---|---|
class | ||
bird | Psittaciformes | 24.0 |
mammal | Carnivora | 58.0 |
# 第一个,第二个
df.groupby('class').nth([1, 2])
order | max_speed | |
---|---|---|
class | ||
bird | Psittaciformes | 24.0 |
mammal | Primates | NaN |
mammal | Carnivora | 58.0 |
# 每组显示前2个
df.groupby('class').head(2)
class | order | max_speed | |
---|---|---|---|
falcon | bird | Falconiformes | 389.0 |
parrot | bird | Psittaciformes | 24.0 |
lion | mammal | Carnivora | 80.2 |
monkey | mammal | Primates | NaN |
# 每组最后2个
df.groupby('class').tail(2)
class | order | max_speed | |
---|---|---|---|
falcon | bird | Falconiformes | 389.0 |
parrot | bird | Psittaciformes | 24.0 |
monkey | mammal | Primates | NaN |
leopard | mammal | Carnivora | 58.0 |
# 分组序号
df.groupby('class').ngroup()
falcon 0 parrot 0 lion 1 monkey 1 leopard 1 dtype: int64
# 返回每个元素在所在组的序号的序列
df.groupby('class').cumcount(ascending=False)
falcon 1 parrot 0 lion 2 monkey 1 leopard 0 dtype: int64
# 按鸟类首字母分组
df.groupby(df['class'].str[0]).groups
{'b': ['falcon', 'parrot'], 'm': ['lion', 'monkey', 'leopard']}
# 按鸟类第一个字母和第二个字母分组
df.groupby([df['class'].str[0], df['class'].str[1]]).groups
{('b', 'i'): ['falcon', 'parrot'], ('m', 'a'): ['lion', 'monkey', 'leopard']}
# 在组内的排名
df.groupby('class').rank()
max_speed | |
---|---|
falcon | 2.0 |
parrot | 1.0 |
lion | 2.0 |
monkey | NaN |
leopard | 1.0 |
对数据进行分组后,接下来就可以收获果实了,给分组给定统计方法,最终得到分组聚合的结果。除了常见的数学统计方法,还可以使用 agg()
和transform()
等函数进行操作。
# 描述性统计
df.groupby('class').describe()
max_speed | ||||||||
---|---|---|---|---|---|---|---|---|
count | mean | std | min | 25% | 50% | 75% | max | |
class | ||||||||
bird | 2.0 | 206.5 | 258.093975 | 24.0 | 115.25 | 206.5 | 297.75 | 389.0 |
mammal | 2.0 | 69.1 | 15.697771 | 58.0 | 63.55 | 69.1 | 74.65 | 80.2 |
# 一列使用多个聚合方法
df.groupby('class').agg({'max_speed': ['min', 'max', 'sum']})
max_speed | |||
---|---|---|---|
min | max | sum | |
class | |||
bird | 24.0 | 389.0 | 413.0 |
mammal | 58.0 | 80.2 | 138.2 |
df.groupby('class')['max_speed'].agg(
Max='max', Min='min', Diff=lambda x: x.max() - x.min())
Max | Min | Diff | |
---|---|---|---|
class | |||
bird | 389.0 | 24.0 | 365.0 |
mammal | 80.2 | 58.0 | 22.2 |
df.groupby('class').agg(max_speed=('max_speed', 'max'),
count_order=('order', 'count'))
max_speed | count_order | |
---|---|---|
class | ||
bird | 389.0 | 2 |
mammal | 80.2 | 3 |
df.groupby('class').agg(
max_speed=pd.NamedAgg(column='max_speed', aggfunc='max'),
count_order=pd.NamedAgg(column='order', aggfunc='count')
)
max_speed | count_order | |
---|---|---|
class | ||
bird | 389.0 | 2 |
mammal | 80.2 | 3 |
transform
类似于agg
,但不同的是它返回的是一个DataFrame
,每个会将原来的值一一替换成统计后的值,比如按组计算平均值,那么返回的新DataFrame
中每个值就是它所在组的平均值。
df.groupby('class').agg(np.mean)
max_speed | |
---|---|
class | |
bird | 206.5 |
mammal | 69.1 |
df.groupby('class').transform(np.mean)
max_speed | |
---|---|
falcon | 206.5 |
parrot | 206.5 |
lion | 69.1 |
monkey | 69.1 |
leopard | 69.1 |
分组后筛选原始数据:
Signature:
DataFrameGroupBy.filter(func, dropna=True, *args, **kwargs)
Docstring:
Return a copy of a DataFrame excluding filtered elements.
Elements from groups are filtered if they do not satisfy the
boolean criterion specified by func.
# 筛选出 按class分组后,分组内max_speed平均值大于100的元素
df.groupby(['class']).filter(lambda x: x['max_speed'].mean() > 100)
class | order | max_speed | |
---|---|---|---|
falcon | bird | Falconiformes | 389.0 |
parrot | bird | Psittaciformes | 24.0 |
# 取出分组后index
df.groupby('class').apply(lambda x: x.index.to_list())
class bird [falcon, parrot] mammal [lion, monkey, leopard] dtype: object
# 取出分组后每组中max_speed最大的前N个
df.groupby('class').apply(lambda x: x.sort_values(
by='max_speed', ascending=False).head(1))
class | order | max_speed | ||
---|---|---|---|---|
class | ||||
bird | falcon | bird | Falconiformes | 389.0 |
mammal | lion | mammal | Carnivora | 80.2 |
df.groupby('class').apply(lambda x: pd.Series({
'speed_max': x['max_speed'].max(),
'speed_min': x['max_speed'].min(),
'speed_mean': x['max_speed'].mean(),
}))
speed_max | speed_min | speed_mean | |
---|---|---|---|
class | |||
bird | 389.0 | 24.0 | 206.5 |
mammal | 80.2 | 58.0 | 69.1 |
按分组导出Excel
文件:
for group, data in df.groupby('class'):
data.to_excel(f'data/{group}.xlsx')
# 每组去重值后数量
df.groupby('class').order.nunique()
class bird 2 mammal 2 Name: order, dtype: int64
# 每组去重后的值
df.groupby("class")['order'].unique()
class bird [Falconiformes, Psittaciformes] mammal [Carnivora, Primates] Name: order, dtype: object
# 统计每组数据值的数量
df.groupby("class")['order'].value_counts()
class order bird Falconiformes 1 Psittaciformes 1 mammal Carnivora 2 Primates 1 Name: order, dtype: int64
# 每组最大的1个
df.groupby("class")['max_speed'].nlargest(1)
class bird falcon 389.0 mammal lion 80.2 Name: max_speed, dtype: float64
# 每组最小的2个
df.groupby("class")['max_speed'].nsmallest(2)
class bird parrot 24.0 falcon 389.0 mammal leopard 58.0 lion 80.2 Name: max_speed, dtype: float64
# 每组值是否单调递增
df.groupby("class")['max_speed'].is_monotonic_increasing
class bird False mammal False Name: max_speed, dtype: bool
# 每组值是否单调递减
df.groupby("class")['max_speed'].is_monotonic_decreasing
class bird True mammal False Name: max_speed, dtype: bool
实际生产中,我们拿到的原始数据的表现形状可能并不符合当前需求,比如说不是期望的维度、数据不够直观、表现力不够等等。此时,可以对原始数据进行适当的变形,比如堆叠、透视、行列转置等。
看个简单的例子就能明白讲的是什么:
df = pd.DataFrame([[19, 136, 180, 98], [21, 122, 178, 96]], index=['令狐冲', '李寻欢'],
columns=['age', 'weight', 'height', 'score'])
df
age | weight | height | score | |
---|---|---|---|---|
令狐冲 | 19 | 136 | 180 | 98 |
李寻欢 | 21 | 122 | 178 | 96 |
# 有点像宽表变高表, 我是这样觉得的
df.stack()
令狐冲 age 19 weight 136 height 180 score 98 李寻欢 age 21 weight 122 height 178 score 96 dtype: int64
# 有点像高表变宽表
df.stack().unstack()
age | weight | height | score | |
---|---|---|---|---|
令狐冲 | 19 | 136 | 180 | 98 |
李寻欢 | 21 | 122 | 178 | 96 |
Signature:
df.pivot(index=None, columns=None, values=None) -> 'DataFrame'
Docstring:
Return reshaped DataFrame organized by given index / column values.
Reshape data (produce a "pivot" table) based on column values. Uses
unique values from specified `index` / `columns` to form axes of the
resulting DataFrame. This function does not support data
aggregation, multiple values will result in a MultiIndex in the
columns.
df = pd.DataFrame({'name': ['江小鱼', '江小鱼', '江小鱼', '花无缺', '花无缺',
'花无缺'],
'bug_level': ['A', 'B', 'C', 'A', 'B', 'C'],
'bug_count': [2, 3, 5, 1, 5, 6]})
df
name | bug_level | bug_count | |
---|---|---|---|
0 | 江小鱼 | A | 2 |
1 | 江小鱼 | B | 3 |
2 | 江小鱼 | C | 5 |
3 | 花无缺 | A | 1 |
4 | 花无缺 | B | 5 |
5 | 花无缺 | C | 6 |
把上面的bug等级与bug数统计表变形如下,还是原来的数据,但是不是更加直观呢?
df.pivot(index='name', columns='bug_level', values='bug_count')
bug_level | A | B | C |
---|---|---|---|
name | |||
江小鱼 | 2 | 3 | 5 |
花无缺 | 1 | 5 | 6 |
如果原始数据中有重复的统计呢?就比如说上面的例子中来自不同产品线的bug统计,就可能出现两行这样的数据['江小鱼','B',3]、['江小鱼','B',4]
,先试下用pivot
会怎样?
df = pd.DataFrame({'name': ['江小鱼', '江小鱼', '江小鱼', '江小鱼', '江小鱼', '花无缺', '花无缺',
'花无缺', '花无缺', '花无缺', ],
'bug_level': ['A', 'B', 'C', 'B', 'C', 'A', 'B', 'C', 'A', 'B'],
'bug_count': [2, 3, 5, 4, 6, 1, 5, 6, 3, 1],
'score': [70, 80, 90, 76, 86, 72, 82, 88, 68, 92]})
df
name | bug_level | bug_count | score | |
---|---|---|---|---|
0 | 江小鱼 | A | 2 | 70 |
1 | 江小鱼 | B | 3 | 80 |
2 | 江小鱼 | C | 5 | 90 |
3 | 江小鱼 | B | 4 | 76 |
4 | 江小鱼 | C | 6 | 86 |
5 | 花无缺 | A | 1 | 72 |
6 | 花无缺 | B | 5 | 82 |
7 | 花无缺 | C | 6 | 88 |
8 | 花无缺 | A | 3 | 68 |
9 | 花无缺 | B | 1 | 92 |
try:
df.pivot(index='name', columns='bug_level', values='bug_count')
except ValueError as e:
print(e)
Index contains duplicate entries, cannot reshape
原来,pivot()
只能将数据进行reshape
,不支持聚合。遇到上面这种含重复值需进行聚合计算,应使用pivot_table()
。它能实现类似Excel
那样的高级数据透视功能。
# 统计员工来自不同产品线不同级别的bug总数
df.pivot_table(index=['name'], columns=['bug_level'],
values='bug_count', aggfunc=np.sum)
bug_level | A | B | C |
---|---|---|---|
name | |||
江小鱼 | 2 | 7 | 11 |
花无缺 | 4 | 6 | 6 |
当然,这里的聚合可以非常灵活:
df.pivot_table(index=['name'], columns=['bug_level'], aggfunc={
'bug_count': np.sum, 'score': [max, np.mean]})
bug_count | score | ||||||||
---|---|---|---|---|---|---|---|---|---|
sum | max | mean | |||||||
bug_level | A | B | C | A | B | C | A | B | C |
name | |||||||||
江小鱼 | 2 | 7 | 11 | 70 | 80 | 90 | 70 | 78 | 88 |
花无缺 | 4 | 6 | 6 | 72 | 92 | 88 | 70 | 87 | 88 |
还可以给每列每行加个汇总,如下所示:
df.pivot_table(index=['name'], columns=['bug_level'],
values='bug_count', aggfunc=np.sum, margins=True, margins_name='汇总')
bug_level | A | B | C | 汇总 |
---|---|---|---|---|
name | ||||
江小鱼 | 2 | 7 | 11 | 20 |
花无缺 | 4 | 6 | 6 | 16 |
汇总 | 6 | 13 | 17 | 36 |
交叉表是用于统计分组频率的特殊透视表。简单来说,就是将两个或者多个列重中不重复的元素组成一个新的 DataFrame,新数据的行和列交叉的部分值为其组合在原数据中的数量。
还是来个例子比较直观。有如下学生选专业数据:
df = pd.DataFrame({'name': ['杨过', '小龙女', '郭靖', '黄蓉', '李寻欢', '孙小红', '张无忌',
'赵敏', '令狐冲', '任盈盈'],
'gender': ['男', '女', '男', '女', '男', '女', '男', '女', '男', '女'],
'major': ['机械工程', '软件工程', '金融工程', '工商管理', '机械工程', '金融工程', '软件工程', '工商管理', '软件工程', '工商管理']})
df
name | gender | major | |
---|---|---|---|
0 | 杨过 | 男 | 机械工程 |
1 | 小龙女 | 女 | 软件工程 |
2 | 郭靖 | 男 | 金融工程 |
3 | 黄蓉 | 女 | 工商管理 |
4 | 李寻欢 | 男 | 机械工程 |
5 | 孙小红 | 女 | 金融工程 |
6 | 张无忌 | 男 | 软件工程 |
7 | 赵敏 | 女 | 工商管理 |
8 | 令狐冲 | 男 | 软件工程 |
9 | 任盈盈 | 女 | 工商管理 |
若想了解学生选专业是否与性别有关,可以做如下统计:
pd.crosstab(df['gender'], df['major'])
major | 工商管理 | 机械工程 | 软件工程 | 金融工程 |
---|---|---|---|---|
gender | ||||
女 | 3 | 0 | 1 | 1 |
男 | 0 | 2 | 2 | 1 |
同时,回忆一下上篇讲到的 https://www.cnblogs.com/bytesfly/p/pandas-1.html#画图
# 男、女生填报专业比例饼状图
pd.crosstab(df['gender'], df['major']).T.plot(
kind='pie', subplots=True, figsize=(12, 8), autopct="%.0f%%")
plt.show()
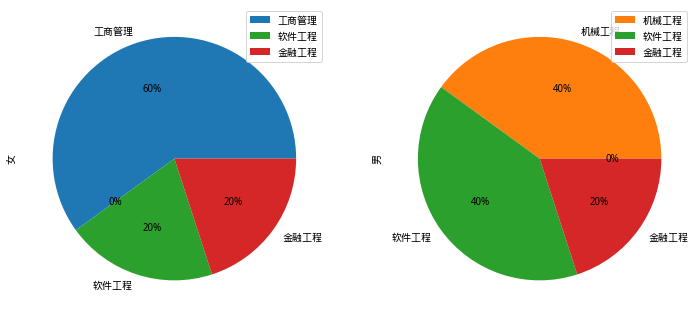
换个角度看下:
# 各专业男女生填报人数柱状图
pd.crosstab(df['gender'], df['major']).T.plot(
kind='bar', stacked=True, rot=0, title='各专业男女生填报人数柱状图', xlabel='', figsize=(10, 6))
plt.show()
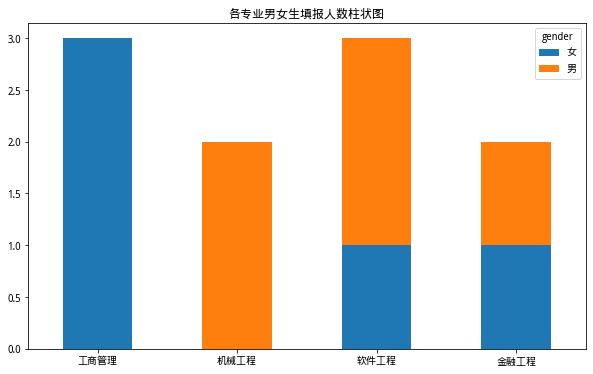
再回到上面所讲的交叉表相关知识。
# 对交叉结果进行归一化
pd.crosstab(df['gender'], df['major'], normalize=True)
major | 工商管理 | 机械工程 | 软件工程 | 金融工程 |
---|---|---|---|---|
gender | ||||
女 | 0.3 | 0.0 | 0.1 | 0.1 |
男 | 0.0 | 0.2 | 0.2 | 0.1 |
# 对交叉结果按行进行归一化
pd.crosstab(df['gender'], df['major'], normalize='index')
major | 工商管理 | 机械工程 | 软件工程 | 金融工程 |
---|---|---|---|---|
gender | ||||
女 | 0.6 | 0.0 | 0.2 | 0.2 |
男 | 0.0 | 0.4 | 0.4 | 0.2 |
# 对交叉结果按列进行归一化
pd.crosstab(df['gender'], df['major'], normalize='columns')
major | 工商管理 | 机械工程 | 软件工程 | 金融工程 |
---|---|---|---|---|
gender | ||||
女 | 1.0 | 0.0 | 0.333333 | 0.5 |
男 | 0.0 | 1.0 | 0.666667 | 0.5 |
同样,也可以给每列每行加个汇总,如下:
pd.crosstab(df['gender'], df['major'], margins=True, margins_name='汇总')
major | 工商管理 | 机械工程 | 软件工程 | 金融工程 | 汇总 |
---|---|---|---|---|---|
gender | |||||
女 | 3 | 0 | 1 | 1 | 5 |
男 | 0 | 2 | 2 | 1 | 5 |
汇总 | 3 | 2 | 3 | 2 | 10 |