OpenMMLab项目的困惑点
OpenMMLab
本随笔规划
- 前言
- 安装
- MMEngine相关
- MMCV相关
————————————————————————————————————
必看内容,建议重复阅读,从而加深理解:
————————————————————————————————————
前言
安装
mim
mim是一种管理工具,比较推荐用mim去安装相关库|包
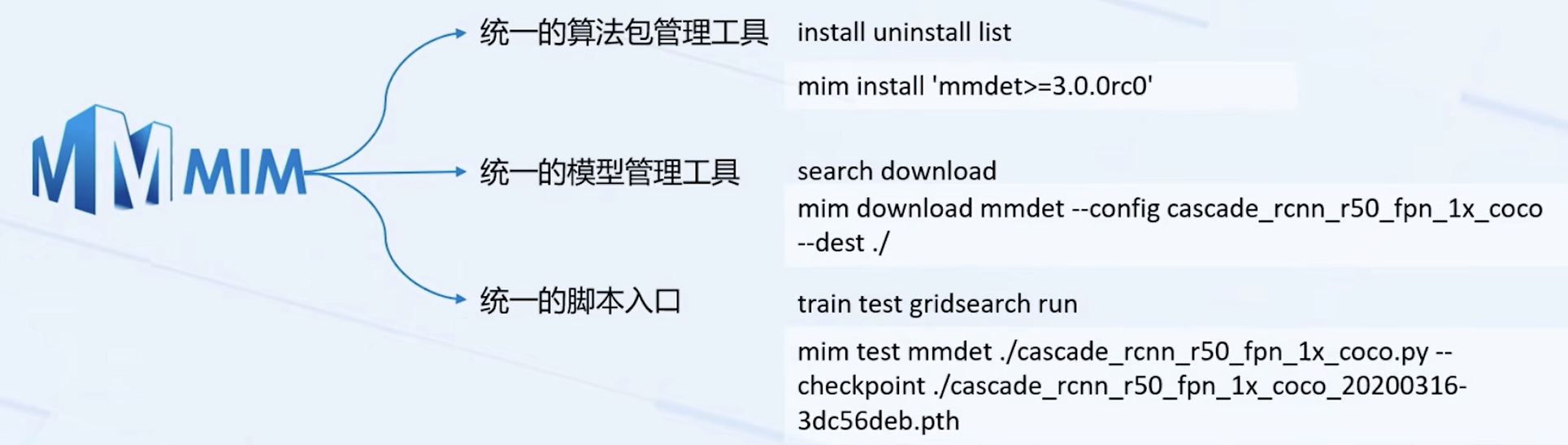
mim 提供了许多命令供User使用,包括install,uninstall,list,search,download,train,gridsearch,run
比如,
mim install使用方式与pip install 完全一致
相比于pip install有两个额外功能:
- 自动为mmcv寻找预编译包,不需要再查cuda,torch版本
- 自动的处理算法库之间的依赖关系
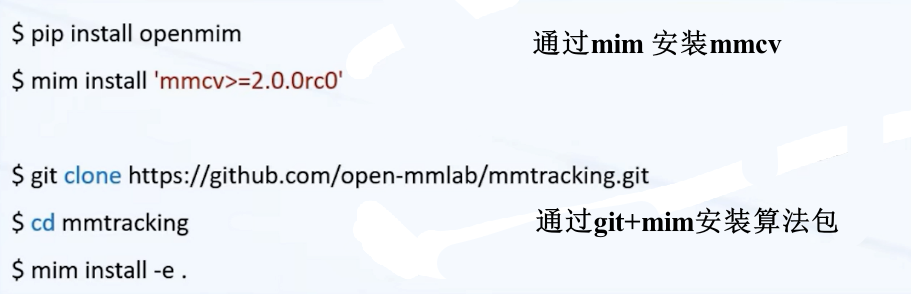
MMEngine
MMEngine 是一个基于 PyTorch 实现的,用于训练深度学习模型的基础库,支持在 Linux、Windows、macOS 上运行。
MMEngine 实现了 OpenMMLab 算法库的新一代训练架构,为 OpenMMLab 中的 30 多个算法库提供了统一的执行基座。其核心组件包含训练引擎、评测引擎和模块管理等
。
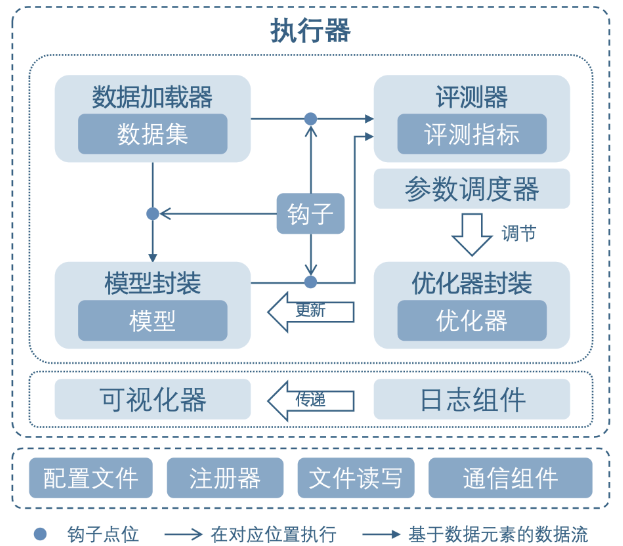
MMEngine 将训练过程中涉及的组件和它们的关系进行了抽象,如上图所示。不同算法库中的同类型组件具有相同的接口定义。
以下将说明核心模块和相关组件:
执行器Runner
训练引擎的核心模块是执行器(Runner)
。执行器负责执行训练
、测试
和推理
任务并管理这些过程中所需要的各个组件。在训练、测试、推理任务执行过程中的特定位置,执行器设置了钩子(Hook)
来允许用户拓展、插入和执行自定义逻辑。执行器主要调用如下组件来完成训练和推理过程中的循环:
-
数据集(Dataset)
:负责在训练、测试、推理任务中构建数据集,并将数据送给模型。实际使用过程中会被数据加载器(DataLoader)封装一层,数据加载器会启动多个子进程来加载数据。 -
模型(Model)
:在训练过程中接受数据并输出 loss;在测试、推理任务中接受数据,并进行预测。分布式训练等情况下会被模型的封装器(Model Wrapper,如 MMDistributedDataParallel)封装一层。 -
优化器封装(Optimizer)
:优化器封装负责在训练过程中执行反向传播优化模型,并且以统一的接口支持了混合精度训练和梯度累加。 -
参数调度器(Parameter Scheduler)
:训练过程中,对学习率、动量等优化器超参数进行动态调整。
在训练间隙或者测试阶段,评测指标与评测器(Metrics & Evaluator)
会负责对模型性能进行评测。其中评测器负责基于数据集对模型的预测进行评估。评测器内还有一层抽象是评测指标,负责计算具体的一个或多个评测指标(如召回率、正确率等)。
为了统一接口,OpenMMLab 2.0 中各个算法库的评测器,模型和数据之间交流的接口都使用了数据元素(Data Element)
来进行封装。
可视化和日志组件
在训练、推理执行过程中,上述各个组件都可以调用日志管理模块和可视化器进行结构化和非结构化日志的存储与展示。
日志管理(Logging Modules)
:负责管理执行器运行过程中产生的各种日志信息。其中消息枢纽(MessageHub)负责实现组件与组件、执行器与执行器之间的数据共享,日志处理器(Log Processor)负责对日志信息进行处理,处理后的日志会分别发送给执行器的日志器(Logger)和可视化器(Visualizer)进行日志的管理与展示。可视化器(Visualizer)
:可视化器负责对模型的特征图、预测结果和训练过程中产生的结构化日志进行可视化,支持 Tensorboard 和 WanDB 等多种可视化后端。
公共基础模块
MMEngine 中还实现了
各种算法模型执行
过程中需要用到的公共基础模块
,包括
-
配置类(Config)
:在 OpenMMLab 算法库中,用户可以通过编写 config 来配置训练、测试过程以及相关的组件。 -
注册器(Registry)
:负责管理算法库中具有相同功能的模块。MMEngine 根据对算法库模块的抽象,定义了一套根注册器,算法库中的注册器可以继承自这套根注册器,实现模块的跨算法库调用
。 -
文件读写(File I/O)
:为各个模块的文件读写提供了统一的接口,以统一的形式支持了多种文件读写后端和多种文件格式
,并具备扩展性。 -
分布式通信原语(Distributed Communication Primitives)
:负责在程序分布式运行过程中不同进程间的通信。这套接口屏蔽了分布式和非分布式环境的区别,同时也自动处理了数据的设备和通信后端。 -
其他工具(Utils)
:还有一些工具性的模块,如 ManagerMixin,它实现了一种全局变量的创建和获取方式,执行器内很多全局可见对象的基类就是 ManagerMixin。
使用示例:
完整的利用 MMEngine 执行器进行训练和验证的脚本:
import torch.nn.functional as F
import torchvision
import torchvision.transforms as transforms
from torch.optim import SGD
from torch.utils.data import DataLoader
from mmengine.evaluator import BaseMetric
from mmengine.model import BaseModel
from mmengine.runner import Runner
class MMResNet50(BaseModel):
def __init__(self):
super().__init__()
self.resnet = torchvision.models.resnet50()
def forward(self, imgs, labels, mode):
x = self.resnet(imgs)
if mode == 'loss':
return {'loss': F.cross_entropy(x, labels)}
elif mode == 'predict':
return x, labels
class Accuracy(BaseMetric):
def process(self, data_batch, data_samples):
score, gt = data_samples
self.results.append({
'batch_size': len(gt),
'correct': (score.argmax(dim=1) == gt).sum().cpu(),
})
def compute_metrics(self, results):
total_correct = sum(item['correct'] for item in results)
total_size = sum(item['batch_size'] for item in results)
return dict(accuracy=100 * total_correct / total_size)
norm_cfg = dict(mean=[0.491, 0.482, 0.447], std=[0.202, 0.199, 0.201])
train_dataloader = DataLoader(batch_size=32,
shuffle=True,
dataset=torchvision.datasets.CIFAR10(
'data/cifar10',
train=True,
download=True,
transform=transforms.Compose([
transforms.RandomCrop(32, padding=4),
transforms.RandomHorizontalFlip(),
transforms.ToTensor(),
transforms.Normalize(**norm_cfg)
])))
val_dataloader = DataLoader(batch_size=32,
shuffle=False,
dataset=torchvision.datasets.CIFAR10(
'data/cifar10',
train=False,
download=True,
transform=transforms.Compose([
transforms.ToTensor(),
transforms.Normalize(**norm_cfg)
])))
runner = Runner(
model=MMResNet50(),
work_dir='./work_dir',
train_dataloader=train_dataloader,
optim_wrapper=dict(optimizer=dict(type=SGD, lr=0.001, momentum=0.9)),
train_cfg=dict(by_epoch=True, max_epochs=5, val_interval=1),
val_dataloader=val_dataloader,
val_cfg=dict(),
val_evaluator=dict(type=Accuracy),
)
runner.train()
基于 PyTorch 和基于 MMEngine 的训练流程对比如下:
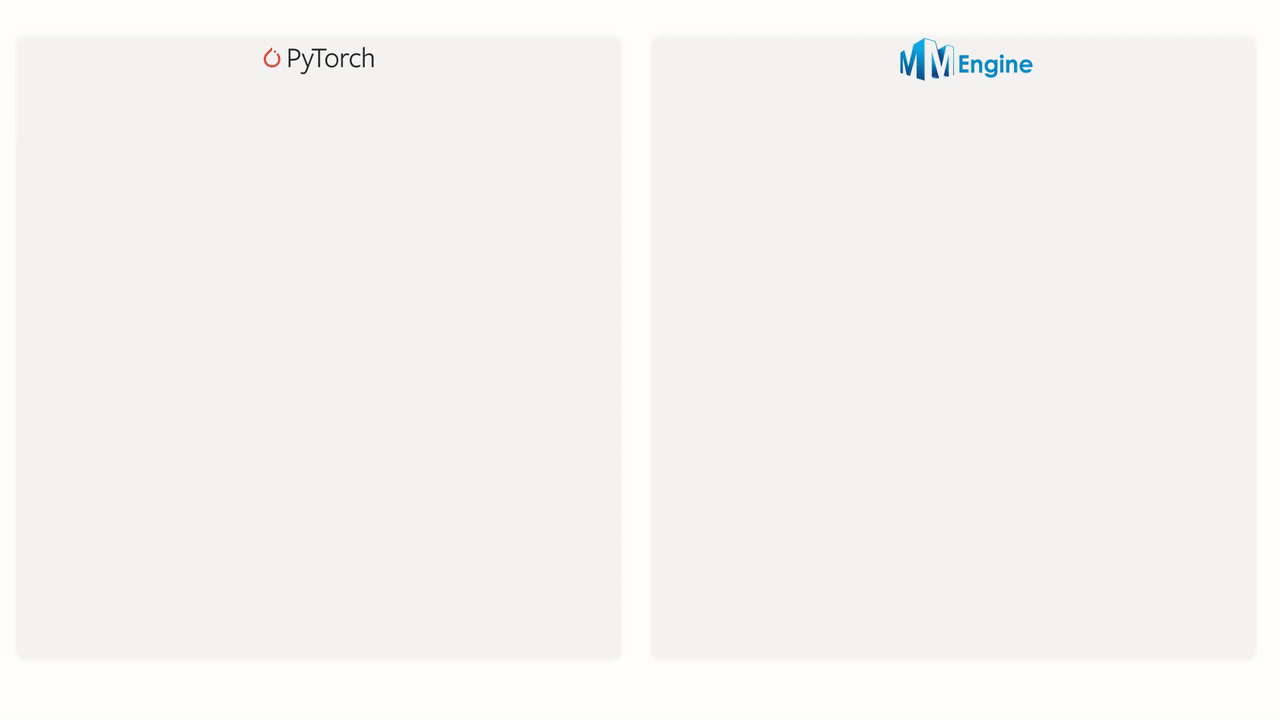
MMCV
MMCV 是计算机视觉研究的基础库,提供以下功能。
- 图像/视频处理
- 图像和注释可视化
- 图像转换
- 各种 CNN 架构
- 高质量实现的通用CUDA操作
It supports the following systems:
- Linux
- Windows
- macOS
It supports many research projects as below:
MMClassification: OpenMMLab image classification toolbox and benchmark.
MMDetection: OpenMMLab detection toolbox and benchmark.
MMDetection3D: OpenMMLab’s next-generation platform for general 3D object detection.
MMRotate: OpenMMLab rotated object detection toolbox and benchmark.
MMYOLO: OpenMMLab YOLO series toolbox and benchmark.
MMSegmentation: OpenMMLab semantic segmentation toolbox and benchmark.
MMOCR: OpenMMLab text detection, recognition, and understanding toolbox.
MMPose: OpenMMLab pose estimation toolbox and benchmark.
MMHuman3D: OpenMMLab 3D human parametric model toolbox and benchmark.
MMSelfSup: OpenMMLab self-supervised learning toolbox and benchmark.
MMRazor: OpenMMLab model compression toolbox and benchmark.
MMFewShot: OpenMMLab fewshot learning toolbox and benchmark.
MMAction2: OpenMMLab’s next-generation action understanding toolbox and benchmark.
MMTracking: OpenMMLab video perception toolbox and benchmark.
MMFlow: OpenMMLab optical flow toolbox and benchmark.
MMEditing: OpenMMLab image and video editing toolbox.
MMGeneration: OpenMMLab image and video generative models toolbox.
MMDeploy: OpenMMLab model deployment framework.
具体点,主要功能如下,
数据处理(Data process)
处理类型包括,Image
Image
该模块提供了一些图像处理方法,需要
opencv
先安装。
- 图像的读/写/显示操作(read/write/show):
To read or write images files, useimread or imwrite
.
import mmcv
img = mmcv.imread('test.jpg')
img = mmcv.imread('test.jpg', flag='grayscale')
img_ = mmcv.imread(img) # nothing will happen, img_ = img
mmcv.imwrite(img, 'out.jpg')
To read images from bytes
with open('test.jpg', 'rb') as f:
data = f.read()
img = mmcv.imfrombytes(data)
To show an image file or a loaded image
mmcv.imshow('tests/data/color.jpg')
# this is equivalent to
for i in range(10):
img = np.random.randint(256, size=(100, 100, 3), dtype=np.uint8)
mmcv.imshow(img, win_name='test image', wait_time=200)
- 色彩空间转换(color space conversion)
Supported conversion methods:- bgr2gray
- gray2bgr
- bgr2rgb
- rgb2bgr
- bgr2hsv
- hsv2bgr
img = mmcv.imread('tests/data/color.jpg')
img1 = mmcv.bgr2rgb(img)
img2 = mmcv.rgb2gray(img1)
img3 = mmcv.bgr2hsv(img)
- 重设尺寸 (resize)
有三种调整大小的方法。所有imresize_*
方法都有一个参数return_scale
,如果这个参数是False
,那么返回值只是调整大小的图像,否则就是一个元组(resized_img, scale)
。
# resize to a given size
mmcv.imresize(img, (1000, 600), return_scale=True)
# resize to the same size of another image
mmcv.imresize_like(img, dst_img, return_scale=False)
# resize by a ratio
mmcv.imrescale(img, 0.5)
# resize so that the max edge no longer than 1000, short edge no longer than 800
# without changing the aspect ratio
mmcv.imrescale(img, (1000, 800))
- 旋转(rotate)
要将图像旋转一定角度,请使用imrotate
.可以指定中心,默认为原始图像的中心。旋转有两种模式,一种是保持图像大小不变,以便在旋转后裁剪图像的某些部分,另一种是扩展图像大小以适应旋转的图像。
img = mmcv.imread('tests/data/color.jpg')
# rotate the image clockwise by 30 degrees.
img_ = mmcv.imrotate(img, 30)
# rotate the image counterclockwise by 90 degrees.
img_ = mmcv.imrotate(img, -90)
# rotate the image clockwise by 30 degrees, and rescale it by 1.5x at the same time.
img_ = mmcv.imrotate(img, 30, scale=1.5)
# rotate the image clockwise by 30 degrees, with (100, 100) as the center.
img_ = mmcv.imrotate(img, 30, center=(100, 100))
# rotate the image clockwise by 30 degrees, and extend the image size.
img_ = mmcv.imrotate(img, 30, auto_bound=True)
- 翻转(flip)
要翻转图像,请使用imflip
。
img = mmcv.imread('tests/data/color.jpg')
# flip the image horizontally
mmcv.imflip(img)
# flip the image vertically
mmcv.imflip(img, direction='vertical')
- 裁剪(crop)
imcrop 可以使用一个或多个区域裁剪图像。每个区域由左上角和右下角坐标表示为 (x1,y1, x2,y2)
import mmcv
import numpy as np
img = mmcv.imread('tests/data/color.jpg')
# crop the region (10, 10, 100, 120)
bboxes = np.array([10, 10, 100, 120])
patch = mmcv.imcrop(img, bboxes)
# crop two regions (10, 10, 100, 120) and (0, 0, 50, 50)
bboxes = np.array([[10, 10, 100, 120], [0, 0, 50, 50]])
patches = mmcv.imcrop(img, bboxes)
# crop two regions, and rescale the patches by 1.2x
patches = mmcv.imcrop(img, bboxes, scale=1.2)
- 填充(padding)
有两种方法,
impad
和impad_to_multiple
,使用给定值将图像填充到特定大小。
img = mmcv.imread('tests/data/color.jpg')
# pad the image to (1000, 1200) with all zeros
img_ = mmcv.impad(img, shape=(1000, 1200), pad_val=0)
# pad the image to (1000, 1200) with different values for three channels.
img_ = mmcv.impad(img, shape=(1000, 1200), pad_val=(100, 50, 200))
# pad the image on left, right, top, bottom borders with all zeros
img_ = mmcv.impad(img, padding=(10, 20, 30, 40), pad_val=0)
# pad the image on left, right, top, bottom borders with different values
# for three channels.
img_ = mmcv.impad(img, padding=(10, 20, 30, 40), pad_val=(100, 50, 200))
# pad an image so that each edge is a multiple of some value.
img_ = mmcv.impad_to_multiple(img, 32)
Video
此模块提供以下功能:
-
一个具有友好 API 的 VideoReader 类,用于阅读和转换视频。
-
一些编辑(剪切、调整、调整大小)视频的方法。
-
光流读/写/弯曲。
-
VideoReader
该VideoReader
类提供类似 API 的序列来访问视频帧。它将在内部缓存已访问的帧。
video = mmcv.VideoReader('test.mp4')
# obtain basic information
print(len(video))
print(video.width, video.height, video.resolution, video.fps)
# iterate over all frames
for frame in video:
print(frame.shape)
# read the next frame
img = video.read()
# read a frame by index
img = video[100]
# read some frames
img = video[5:10]
将视频转换为图像或从图像目录生成视频
# split a video into frames and save to a folder
video = mmcv.VideoReader('test.mp4')
video.cvt2frames('out_dir')
# generate video from frames
mmcv.frames2video('out_dir', 'test.avi')
- 编辑(剪切,调整)方法
还有一些编辑视频的方法,它包装了 ffmpeg 的命令
# cut a video clip
mmcv.cut_video('test.mp4', 'clip1.mp4', start=3, end=10, vcodec='h264')
# join a list of video clips
mmcv.concat_video(['clip1.mp4', 'clip2.mp4'], 'joined.mp4', log_level='quiet')
# resize a video with the specified size
mmcv.resize_video('test.mp4', 'resized1.mp4', (360, 240))
# resize a video with a scaling ratio of 2
mmcv.resize_video('test.mp4', 'resized2.mp4', ratio=2)
- 光流(optical flow)
mmcv
提供以下操作光流的方法。- IO
- Visualization
- Flow warping
我们提供了两个选项来转储光流文件:未压缩和压缩
。未压缩的方式
只是将浮动数字转储到二进制文件中。它是无损的,但转储的文件大小较大。压缩方式
将光流量化为 0-255,并将其转储为 jpeg 图像。x-dim 和 y-dim 的流将连接成一个图像。
1. IO
:
flow = np.random.rand(800, 600, 2).astype(np.float32)
# dump the flow to a flo file (~3.7M)
mmcv.flowwrite(flow, 'uncompressed.flo')
# dump the flow to a jpeg file (~230K)
# the shape of the dumped image is (800, 1200)
mmcv.flowwrite(flow, 'compressed.jpg', quantize=True, concat_axis=1)
# read the flow file, the shape of loaded flow is (800, 600, 2) for both ways
flow = mmcv.flowread('uncompressed.flo')
flow = mmcv.flowread('compressed.jpg', quantize=True, concat_axis=1)
2. Visualization
可以使用
mmcv.flowshow()
来可视化光流。
mmcv.flowshow(flow)
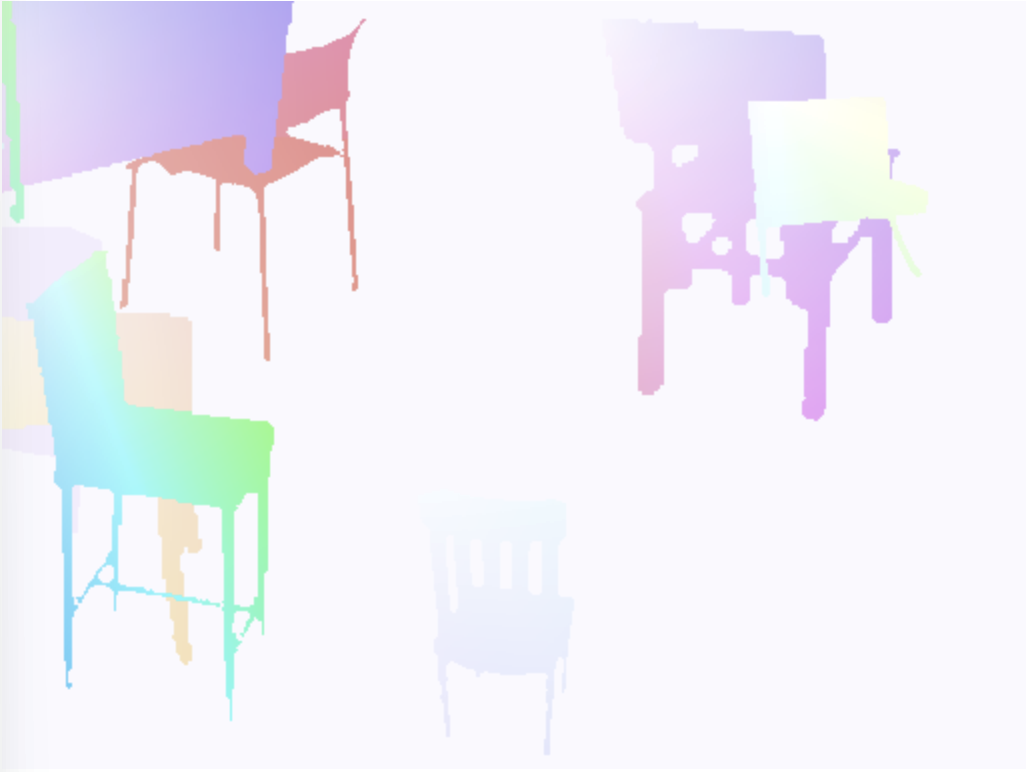
3. Flow warping
img1 = mmcv.imread('img1.jpg')
flow = mmcv.flowread('flow.flo')
warped_img2 = mmcv.flow_warp(img1, flow)
img1 (left) and img2 (right)
optical flow (img2 -> img1)
warped image and difference with ground truth(扭曲的图像和与真实的差异)
数据转型(Data transformation)
在 OpenMMLab 算法库中,
数据集构建
和数据准备
是解耦的。通常,数据集的构建只是对数据集进行解析并记录每个样本的基本信息,而数据准备则是一系列的数据转换,包括数据加载、预处理、格式化等操作,这些都是根据样本的基本信息进行的。
Design of data transformation
在 MMCV 中,我们使用各种可调用的数据转换类
来操作数据。这些数据转换类可以接受实例化的多个配置参数,然后按__call__
方法处理输入数据字典。所有数据转换方法都接受字典作为输入
,并将输出生成为字典
。一个简单的例子如下:
import numpy as np
from mmcv.transforms import Resize
transform = Resize(scale=(224, 224))
data_dict = {'img': np.random.rand(256, 256, 3)}
data_dict = transform(data_dict)
print(data_dict['img'].shape)
>>> (224,224,3)
数据转换类读取输入字典的某些字段,并可能添加或更新某些字段。这些字段的键大多是固定的。例如, Resize 将始终读取输入字典 "img" 中的字段。有关输入和输出字段约定的更多信息,请参阅相应类的文档。
注意
,
按照惯例,在数据转换中用作初始化参数的图像形状的顺序(如调整大小、填充)为tuple(宽度、高度)
。在数据转换返回的字典中,与图像相关的形状,如 img_shape
,ori_shape
,pad_shape
等信息,是tuple(高度、宽度)
。
MMCV 为所有数据转换类提供了一个统一的 BaseTransform
基类:
class BaseTransform(metaclass=ABCMeta):
def __call__(self, results: dict) -> dict:
return self.transform(results)
@abstractmethod
def transform(self, results: dict) -> dict:
pass
所有数据转换类都必须继承 BaseTransform
并实现该 transform
方法。 transform
该方法的输入和输出都是字典。在自定义数据转换类部分,我们将更详细地描述如何实现数据转换类。
Data pipeline数据管道
如上所述,所有数据转换的
输入和输出都是字典
。此外,根据 OpenMMLab 中的 [Convention on Datasets] (TODO),数据集中每个样本的基本信息也是字典。这样,我们可以端到端地连接所有数据转换操作,并将它们组合到一个数据管道中
。该流水线输入数据集中样本的信息字典,经过一系列处理后输出信息字典。
以分类任务为例,下图展示了一个典型的数据管道。对于每个样本,数据集中存储的信息都是字典,如图中最左边所示。在蓝色块表示的每个数据转换操作之后,将向数据字典添加一个新字段(标记为绿色)或更新现有字段(标记为橙色)。
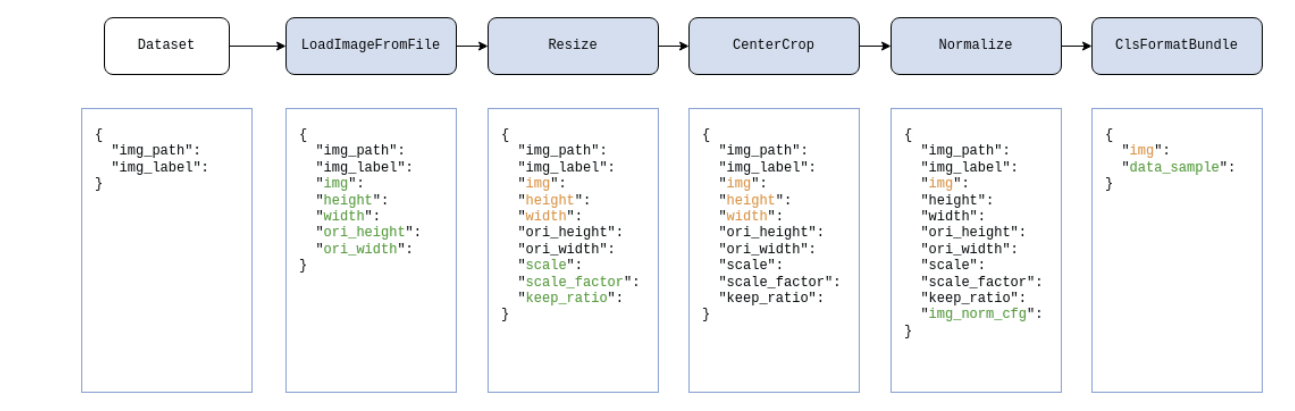
数据管道是配置文件中多个数据转换配置字典的列表。每个数据集都需要设置参数 pipeline 来定义数据集需要执行的数据准备操作。配置文件中上述数据管道的配置如下:
pipeline = [
dict(type='LoadImageFromFile'),
dict(type='Resize', size=256, keep_ratio=True),
dict(type='CenterCrop', crop_size=224),
dict(type='Normalize', mean=[123.675, 116.28, 103.53], std=[58.395, 57.12, 57.375]),
dict(type='ClsFormatBundle')
]
dataset = dict(
...
pipeline=pipeline,
...
)
常见数据转换类
常用的数据转换类大致可以分为数据加载
、数据预处理
和增强以及数据格式化
。在 MMCV 中,我们提供了一些常用的类,如下所示:
Data loading 数据加载
为了支持大规模数据集的加载,初始化时
Dataset
通常不会加载数据。仅加载相应的路径。因此,有必要在数据管道中加载特定数据。
Class | Feature |
---|---|
LoadImageFromFile | Load from file path从文件路径加载 |
LoadAnnotations | Load and organize the annotations (bbox, etc.)加载和组织注释(bbox 等) |
Data preprocessing and enhancement 数据预处理和增强
数据预处理和增强通常涉及转换图像本身,例如裁剪、填充、缩放等。
Class | Feature |
---|---|
Pad | Padding |
CenterCrop | Center crop 中心裁剪 |
Normalize | Image normalization 图像归一化 |
Resize | 调整大小到指定的大小或比例 |
RandomResize | 在指定范围内随机缩放图像 |
RandomMultiscaleResize | 从多个选项中将图像缩放为随机大小 |
RandomGrayscale | 随机灰度 |
RandomFlip | 随机翻转 |
MultiScaleFlipAug | 支持测试过程中的缩放和翻转 |
Data formatting 数据格式
数据格式化操作是对数据执行的类型转换。
Class | Feature |
---|---|
ToTensor | 将指定数据转换为 torch.Tensor |
ImageToTensor | 将图像转换为 torch.Tensor |
自定义数据转换类
若要实现新的数据转换类,必须继承 BaseTransform 并实现该 transform 方法。在这里,我们以一个简单的翻转变换 ( MyFlip ) 为例:
import random
import mmcv
from mmcv.transforms import BaseTransform, TRANSFORMS
@TRANSFORMS.register_module()
class MyFlip(BaseTransform):
def __init__(self, direction: str):
super().__init__()
self.direction = direction
def transform(self, results: dict) -> dict:
img = results['img']
results['img'] = mmcv.imflip(img, direction=self.direction)
return results
现在,我们可以 MyFlip
实例化为可调用对象来处理我们的数据字典。
import numpy as np
transform = MyFlip(direction='horizontal')
data_dict = {'img': np.random.rand(224, 224, 3)}
data_dict = transform(data_dict)
processed_img = data_dict['img']
或者,在 pipeline
配置文件中使用 MyFlip
transform。
pipeline = [
...
dict(type='MyFlip', direction='horizontal'),
...
]
需要注意的是,如果要在配置文件中使用它,则必须确保在运行时可以导入 MyFlip
类所在的文件。
Transform wrapper 转换包装器
转换包装器是一类特殊的数据转换。它们本身不会对数据字典中的图像、标签或其他信息进行操作。相反,它们
增强了其中定义的数据转换的行为
。
KeyMapper
KeyMapper
用于映射数据字典中的字段。例如,图像处理转换通常从数据字典中的 "img"
字段获取其值。但有时我们希望这些转换能够处理数据字典中其他字段中的图像,例如字段 "gt_img"
。
与注册表和配置文件一起使用时,应按如下方式使用字段映射包装器:
pipeline = [
...
dict(type='KeyMapper',
mapping={
'img': 'gt_img', # map "gt_img" to "img"
'mask': ..., # The "mask" field in the raw data is not used. That is, for wrapped data transformations, the "mask" field is not included in the data
},
auto_remap=True, # remap "img" back to "gt_img" after the transformation
transforms=[
# only need to specify "img" in `RandomFlip`
dict(type='RandomFlip'),
])
...
]
有了 KeyMapper
,我们在实现数据转换类时不需要考虑 transform
方法中各种可能的输入字段名称。我们只需要处理默认字段。
RandomChoice 和 RandomApply
RandomChoice
用于从给定选项中随机选择数据转换管道。有了这个包装器,我们可以轻松实现一些数据增强功能,比如 AutoAugment。
在配置文件中,您可以按如下方式使用 RandomChoice
:
pipeline = [
...
dict(type='RandomChoice',
transforms=[
[
dict(type='Posterize', bits=4),
dict(type='Rotate', angle=30.)
], # the first combo option
[
dict(type='Equalize'),
dict(type='Rotate', angle=30)
], # the second combo option
],
prob=[0.4, 0.6] # the prob of each combo
)
...
]
RandomApply
用于随机执行具有指定概率的数据转换组合。例如:
pipeline = [
...
dict(type='RandomApply',
transforms=[dict(type='Rotate', angle=30.)],
prob=0.3) # perform the transformation with prob as 0.3
...
]
TransformBroadcaster
通常,数据转换类仅从一个字段读取操作的目标。虽然我们也可以使用 KeyMapper
来更改读取的字段,但无法同时对多个字段的数据进行转换。为了实现这一点,我们需要使用 多目标扩展包装器 TransformBroadcaster
。
TransformBroadcaster
有两种用途,一种是将数据转换应用于多个指定字段,另一种是将数据转换应用于一个字段下的一组目标。
- 应用于多个领域
假设我们需要对两个字段 "lq" (低质量)和 "gt" (真实)中的图像应用数据转换。
pipeline = [
dict(type='TransformBroadcaster',
# apply to the "lq" and "gt" fields respectively, and set the "img" field to both
mapping={'img': ['lq', 'gt']},
# remap the "img" field back to the original field after the transformation
auto_remap=True,
# whether to share random variables in the transformation of each target
# more introduction will be referred in the following chapters (random variable sharing)
share_random_params=True,
transforms=[
# only need to manipulate the "img" field in the `RandomFlip` class
dict(type='RandomFlip'),
])
]
在多目标扩展的 mapping
设置中,我们也可以用来 ...
忽略指定的原始字段。如以下示例所示,换行 RandomCrop 将裁剪字段中的图像, "img" 并更新裁剪图像的大小(如果该字段 "img_shape" 存在)。如果我们想同时对两个图像字段 "lq" "gt" 进行相同的随机裁剪,但只更新 "img_shape" 一次字段,我们可以按照示例中的方式进行:
pipeline = [
dict(type='TransformBroadcaster',
mapping={
'img': ['lq', 'gt'],
'img_shape': ['img_shape', ...],
},
# remap the "img" and "img_shape" fields back to their original fields after the transformation
auto_remap=True,
# whether to share random variables in the transformation of each target
# more introduction will be referred in the following chapters (random variable sharing)
share_random_params=True,
transforms=[
# "img" and "img_shape" fields are manipulated in the `RandomCrop` class
# if "img_shape" is missing, only operate on "img"
dict(type='RandomCrop'),
])
]
- 应用于字段的一组目标
假设我们需要对 "images" 字段应用数据转换,该字段是图像列表。
pipeline = [
dict(type='TransformBroadcaster',
# map each image under the "images" field to the "img" field
mapping={'img': 'images'},
# remap the images under the "img" field back to the list in the "images" field after the transformation
auto_remap=True,
# whether to share random variables in the transformation of each target
share_random_params=True,
transforms=[
# in the `RandomFlip` transformation class, we only need to manipulate the "img" field
dict(type='RandomFlip'),
])
]
Decorator cache_randomness
在TransformBroadcaster
,我们提供了支持跨多个数据转换共享随机状态 share_random_params
的选项。例如,在超分辨率任务中,我们希望同时对低分辨率图像和原始图像应用相同的随机变换。如果我们在自定义数据转换类中使用此函数,则需要标记哪些随机变量支持在类中共享。这可以通过装饰器 cache_randomness
来实现。
从 MyFlip 上面的例子来看,我们想以一定的概率随机执行翻转:
from mmcv.transforms.utils import cache_randomness
@TRANSFORMS.register_module()
class MyRandomFlip(BaseTransform):
def __init__(self, prob: float, direction: str):
super().__init__()
self.prob = prob
self.direction = direction
@cache_randomness # label the output of the method as a shareable random variable
def do_flip(self):
flip = True if random.random() > self.prob else False
return flip
def transform(self, results: dict) -> dict:
img = results['img']
if self.do_flip():
results['img'] = mmcv.imflip(img, direction=self.direction)
return results
在上面的例子中,我们用 cache_randomness 来修饰 do_flip
方法,将方法返回值 flip
标记为支持共享的随机变量。因此,在 TransformBroadcaster 转换为多个目标时,此变量的值将保持不变。
Decorator avoid_cache_randomness
在某些情况下,我们无法将数据转换中生成随机变量的过程分离到类方法中。例如,数据转换中使用的第三方库中的模块将随机变量的相关部分封装在其中,因此无法将其提取为数据转换的类方法。此类数据转换无法通过装饰器 cache_randomness 注释支持共享随机变量,因此在多目标扩展期间无法共享随机变量。
为了避免在多对象扩展中滥用此类数据转换,我们提供了另一个装饰器 avoid_cache_randomness
,用于标记此类数据转换:
from mmcv.transforms.utils import avoid_cache_randomness
@TRANSFORMS.register_module()
@avoid_cache_randomness
class MyRandomTransform(BaseTransform):
def transform(self, results: dict) -> dict:
...
当标有的数据 avoid_cache_randomness
转换类的实例包装为 TransformBroadcaster 且参数 share_random_params 设置为 True
时,将引发异常。这提醒用户不要以这种方式使用它。
使用 avoid_cache_randomness 时需要牢记以下几点:
avoid_cache_randomness
仅用于修饰数据转换类(BaseTransfrom
的子类),不能用于修饰其他常规类、类方法或函数- 当修饰的数据
avoid_cache_randomness
转换用作基类时,其子类将不会继承其功能。如果子类仍然无法共享随机变量,avoid_cache_randomness
则应再次使用。 - 仅当数据转换是随机的并且不能共享其随机参数时,才需要修改
avoid_cache_randomness
数据转换。没有随机性的数据转换不需要修饰