聚类--K均值算法:自主实现与sklearn.cluster.KMeans调用
import numpy as np from sklearn.datasets import load_iris iris = load_iris() x=iris.data[:,1] y=np.zeros(150) k=3 def initcent(x,k): return x[0:k].reshape(k) def nearest(kc,i):#数组中的值,与聚类中心最小距离所在类型的索引号 d=(abs(kc-i)) w=np.where(d == np.min(d)) return w[0][0] def kcmean(x,y,kc,k): #计算各聚类新均值 l=list(kc) flag= False for c in range(k): print(c) m=np.where(y == c) # print(m,x[m]) n = np.mean(x[m]) # print(kc[c],n) if l[c] !=n: # kc[c] = n # print(type(kc),kc[c]) l[c] = n flag = True print(l,flag) return (np.array(l),flag) def xclessify(x,y,kc): for i in range(x.shape[0]):# 对数组的每个值分类 y[i] = nearest(kc,x[i]) return y kc=initcent(x,k) flag = True print(x,y,kc,flag) while flag: y = xclessify(x, y, kc) kc,flag=kcmean(x,y,kc,k) print(y,kc) import matplotlib.pyplot as plt plt.scatter(x,x,c=y,s=50,cmap='rainbow',marker='p',alpha=0.5); plt.show()
C:\Users\Administrator\PycharmProjects\untitled1\venv\Scripts\python.exe C:/Users/Administrator/Desktop/kjbfdsaf.py C:\Users\Administrator\PycharmProjects\untitled1\venv\lib\site-packages\sklearn\externals\joblib\externals\cloudpickle\cloudpickle.py:47: DeprecationWarning: the imp module is deprecated in favour of importlib; see the module's documentation for alternative uses import imp [3.5 3. 3.2 3.1 3.6 3.9 3.4 3.4 2.9 3.1 3.7 3.4 3. 3. 4. 4.4 3.9 3.5 3.8 3.8 3.4 3.7 3.6 3.3 3.4 3. 3.4 3.5 3.4 3.2 3.1 3.4 4.1 4.2 3.1 3.2 3.5 3.6 3. 3.4 3.5 2.3 3.2 3.5 3.8 3. 3.8 3.2 3.7 3.3 3.2 3.2 3.1 2.3 2.8 2.8 3.3 2.4 2.9 2.7 2. 3. 2.2 2.9 2.9 3.1 3. 2.7 2.2 2.5 3.2 2.8 2.5 2.8 2.9 3. 2.8 3. 2.9 2.6 2.4 2.4 2.7 2.7 3. 3.4 3.1 2.3 3. 2.5 2.6 3. 2.6 2.3 2.7 3. 2.9 2.9 2.5 2.8 3.3 2.7 3. 2.9 3. 3. 2.5 2.9 2.5 3.6 3.2 2.7 3. 2.5 2.8 3.2 3. 3.8 2.6 2.2 3.2 2.8 2.8 2.7 3.3 3.2 2.8 3. 2.8 3. 2.8 3.8 2.8 2.8 2.6 3. 3.4 3.1 3. 3.1 3.1 3.1 2.7 3.2 3.3 3. 2.5 3. 3.4 3. ] [0. 0. 0. 0. 0. 0. 0. 0. 0. 0. 0. 0. 0. 0. 0. 0. 0. 0. 0. 0. 0. 0. 0. 0. 0. 0. 0. 0. 0. 0. 0. 0. 0. 0. 0. 0. 0. 0. 0. 0. 0. 0. 0. 0. 0. 0. 0. 0. 0. 0. 0. 0. 0. 0. 0. 0. 0. 0. 0. 0. 0. 0. 0. 0. 0. 0. 0. 0. 0. 0. 0. 0. 0. 0. 0. 0. 0. 0. 0. 0. 0. 0. 0. 0. 0. 0. 0. 0. 0. 0. 0. 0. 0. 0. 0. 0. 0. 0. 0. 0. 0. 0. 0. 0. 0. 0. 0. 0. 0. 0. 0. 0. 0. 0. 0. 0. 0. 0. 0. 0. 0. 0. 0. 0. 0. 0. 0. 0. 0. 0. 0. 0. 0. 0. 0. 0. 0. 0. 0. 0. 0. 0. 0. 0. 0. 0. 0. 0. 0. 0.] [3.5 3. 3.2] True 0 [3.6378378378378375, 3.0, 3.2] True 1 [3.6378378378378375, 2.793617021276596, 3.2] True 2 [3.6378378378378375, 2.793617021276596, 3.2315789473684213] True 0 [3.752, 2.793617021276596, 3.2315789473684213] True 1 [3.752, 2.753012048192771, 3.2315789473684213] True 2 [3.752, 2.753012048192771, 3.245238095238096] True 0 1 [3.752, 2.6403508771929824, 3.245238095238096] True 2 [3.752, 2.6403508771929824, 3.151470588235294] True 0 1 [3.752, 2.585106382978723, 3.151470588235294] True 2 [3.752, 2.585106382978723, 3.119230769230769] True 0 1 2 [0. 2. 2. 2. 0. 0. 2. 2. 2. 2. 0. 2. 2. 2. 0. 0. 0. 0. 0. 0. 2. 0. 0. 2. 2. 2. 2. 0. 2. 2. 2. 2. 0. 0. 2. 2. 0. 0. 2. 2. 0. 1. 2. 0. 0. 2. 0. 2. 0. 2. 2. 2. 2. 1. 1. 1. 2. 1. 2. 1. 1. 2. 1. 2. 2. 2. 2. 1. 1. 1. 2. 1. 1. 1. 2. 2. 1. 2. 2. 1. 1. 1. 1. 1. 2. 2. 2. 1. 2. 1. 1. 2. 1. 1. 1. 2. 2. 2. 1. 1. 2. 1. 2. 2. 2. 2. 1. 2. 1. 0. 2. 1. 2. 1. 1. 2. 2. 0. 1. 1. 2. 1. 1. 1. 2. 2. 1. 2. 1. 2. 1. 0. 1. 1. 1. 2. 2. 2. 2. 2. 2. 2. 1. 2. 2. 2. 1. 2. 2. 2.] [3.752 2.58510638 3.11923077] 进程已结束,退出代码0
#3.用sklearn.cluster.KMeans,鸢尾花花瓣长度数据做聚类并用散点图显示 import numpy as np from sklearn.cluster import KMeans from sklearn.datasets import load_iris import matplotlib.pyplot as plt iris_data = load_iris() X=iris_data.data petal_length = X[:, 2:3] x= petal_length print(x) k_means = KMeans(n_clusters=3) est = k_means.fit(x) kc = est.cluster_centers_ y_kmeans = k_means.predict(x)
plt.scatter(x,np.linspace(1,150,150),c=y_kmeans,marker='o',cmap='rainbow',linewidths=4) plt.show plt(1,150150),c=y_kmeans,marker='o',cmap='rainbow',linewidths=4) plt.show()
import matplotlib.pyplot as plt import numpy as np from sklearn.datasets import load_iris iris = load_iris() X = iris.data X from sklearn.cluster import KMeans est = KMeans(n_clusters = 3) est.fit(X) kc = est.cluster_centers_ y_kmeans = est.predict(X) #预测每个样本的聚类索引 print(y_kmeans,kc) print(kc.shape,y_kmeans.shape,np.shape) plt.scatter(X[:,0],X[:,1],c=y_kmeans,s=50,cmap='rainbow'); plt.show()
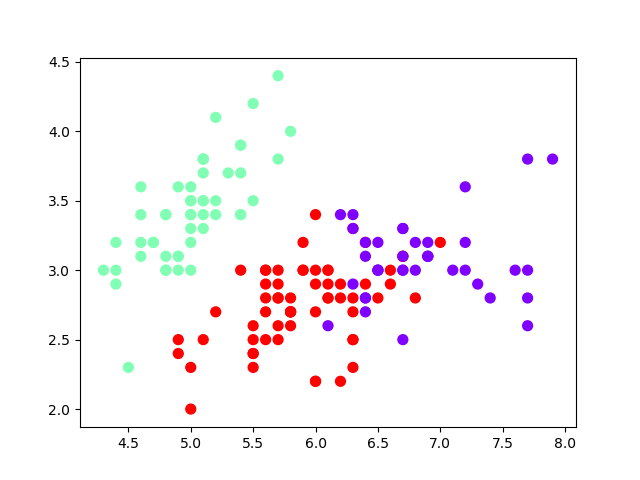