Modeling Relational Data with Graph Convolutional Networks
Abstract. Knowledge graphs enable a wide variety of applications, including question answering and information retrieval. Despite the great effort invested in their creation and maintenance, even the largest (e.g., Yago, DBPedia or Wikidata) remain incomplete. We introduce Relational Graph Convolutional Networks (关系图卷积网络) (R-GCNs) and apply them to two standard knowledge base completion tasks: Link prediction (recovery of missing facts, i.e. subject-predicate-object triples) and entity classification (recovery of missing entity attributes). R-GCNs are related to a recent class of neural networks operating on graphs, and are developed specifically to handle the highly multi-relational data characteristic of realistic knowledge bases. We demonstrate the effectiveness of R-GCNs as a stand-alone model for entity classification. We further show that factorization models (分解模型) for link prediction such as DistMult can be significantly improved through the use of an R-GCN encoder model to accumulate evidence over multiple inference steps in the graph, demonstrating a large improvement of 29.8% on FB15k-237 over a decoder-only baseline.
- Introduction
预测知识库中的缺失信息是统计关系学习 (SRL) 的主要焦点。本文主要考虑SRL中的两种任务:链路预测 (Link prediction,恢复缺失的triples) 和实体分类 (Entity classification,为entities指定类型或分类属性)。在这两种情况下,通过领域结构编码的图中可能存在许多缺失信息。出于这个动机,本文在关系图中为实体提出了编码器模型并用在两项任务上。
实体分类模型在图中的每个节点使用softmax分类器。分类器采用R-GCN提供的节点表示并预测标签,通过优化交叉熵损失来学习。链路预测模型视为自动编码器,包括(1)编码器:产生实体潜在特征表示的R-GCN;(2)解码器:利用这些表示预测标记边缘的张量分解模型,本文采用的是DistMult。
- Neural relational modeling
设有向标记图 \(G=\left( V,\varepsilon ,R \right)\);
点(实体) \({{v}_{i}}\in V\);
标记边(关系) \(\left( {{v}_{i}},r,{{v}_{j}} \right)\in \varepsilon\), 其中\(r \in R\)是一个关系类型(canonical direction and inverse direction)。
2.1 Relational graph convolutional networks
本文最初的动机是将本地图邻域上运行的GCN扩展到大规模关系数据。这些及相关方法(如GNN)可以理解为一个简单的可微消息传递框架的特殊情况:
其中,\(h_{i}^{\left( l \right)}\in {{\mathbb{R}}^{{{d}^{\left( l \right)}}}}\) 是点\({v}_{i}\)在第\(l\)层神经网络的隐藏状态,\({{d}^{\left( l \right)}}\)是该层的表征的维度。\({M}_{i}\)表示为节点\({v}_{i}\)的传入消息集并且通常被视为与传入边集相同(\({M}_{i}\) denotes the set of incoming messages for node vi and is often chosen to be identical to the set of incoming edges)。\({{g}_{m}}\left( \cdot ,\cdot \right)\)表示消息传入,通常被选为(特定于消息的)神经网络函数,或简单的线性变换\({{g}_{m}}\left( {{h}_{i}},{{h}_{j}} \right)=W{{h}_{j}}\),\(W\)是一个权重矩阵。这种变换被证明在计算和编码来自本地结构化邻域非常有效,并导致了图分类和图半监督的重大改进。
本文在上式(1)的基础上,定义了一下简单的传播模型:
其中\(N_{i}^{r}\)表示节点\(i\)在关系\(r \in R\)下的邻居节点的集合(\(N_{i}^{\tau }\)the set of neighbor indices of node \(i\) under relation \(r \in R\))。\({{c}_{i,r}}\)是一个特定于问题的归一化常数可以提前学习或给定(例如令\({{c}_{i,r}}=\left| N_{i}^{r} \right|\))。
Remark1: \(r\)就是某种关系,\(i\)就是要关注的那个节点,\({N}^{r}\)就是\(i\)这个节点在关系\(r\)下的邻居节点集合,\(j\)就是集合内的一个点,\({W}_{0}^{l}\)是网络初始化参数,\({h}_{i}^{l}\)是\(i\)这个节点上一层的特征,\({h}_{j}^{l}\)是在\(r\)关联下邻居节点的特征,\({W}_{r}^{l}\)是\(r\)关系下的要学习的参数。
为了确保第\(l+1\)层节点的表示可以通过第\(l\)层的相应表示来informed,本文向数据中的每个节点添加特殊关系类型的单个自连接(自环)。并注意,理论上来说可以选择任何函数来代替线性变换\(W\).
Remark2:上述中可以得知,R-GCN每层的节点特征都是由上一层节点特征和节点的关系(边)得到;邻居节点特征和自身特征加权求和得到新特征;为保留节点自身信息,考虑自环。
神经网络层更新包括对途中每个节点并行计算(2)。在实践中,上式可以使用稀疏矩阵乘法有效实现,以避免在邻域上显示求和。可以堆叠多个层,以允许跨多个关系步骤的依赖项。(A neural network layer update consists of evaluating (2) in parallel for every node in the graph. In practice, (2) can be implemented efficiently using sparse matrix multiplications to avoid explicit summation over neighborhoods. Multiple layers can be stacked to allow for dependencies across several relational steps.)
R-GCN模型如下:
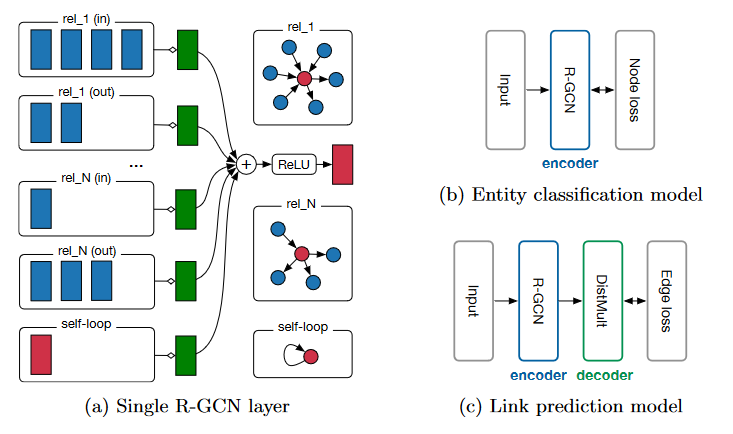
Figure 1. R-GCN模型中单个图节点/实体(红色)更新的计算图。收集来自相邻节点(深蓝色)的激活(d维向量),然后分别对每个关系类型进行变换(对于入边和出边)。结果表示(绿色)以(归一化)和的形式累积,并通过激活函数(如ReLU)。这种每节点更新可以与整个图中的共享参数并行计算。(b)用每个节点损失函数描述实体分类的R-GCN模型。(c)具有R-GCN编码器(散布有完全连接/密集层)和DistMult解码器的链路预测模型。
2.2 Regularization
使用(2)式应用在高维多关系数据上有许多问题:参数数量的迅速增长、过拟合以及模型过于庞大。为解决这个问题,本文提出了两种不同方法来正则化R-GCN层的权重:基分解和块对角分解。
基分解:每个\(W_{r}^{\left(l\right)}\)定义为:
基分解作为基变换\(V_{b}^{\left( l \right)}\in {{\mathbb{R}}^{{{d}^{\left( l+1 \right)}}\times {{d}^{\left( l \right)}}}}\)和系数\(a_{rb}^{\left( l \right)}\)的线性组合,使得系数只依赖于\(r\)。
块对角分解:通过一组低维矩阵的直和来定义每个\(W_{r}^{\left(l\right)}\):
因此,\(W_{r}^{\left(l\right)}\)也是块对角矩阵:
基函数分解(3)可以看作是不同关系类型之间有效权重共享的一种形式,块分解(4)可以看作每个关系类型的权重矩阵上的稀疏性约束,其核心在于潜在的特征可以被分解成一组向量,这些变量在组内的耦合比在组间的耦合更紧密。这两种分解都减少了学习高度多关系数据(如真实知识库)所需的参数数量。
然后整个R-GCN模型采用以下形式:根据(2)中的定义堆叠\(l\)层-上一层的输出是下一层的输入。如果没有其他特征,可以选择第一层的输入作为图中每个节点的one-hot向量。对于块表征,通过单个线性变化将该one-hot向量映射到密集表示。
- 实体分类
对于节点(实体)的(半)监督分类,简单的堆叠形式为(2)的R-GCN层,在最后一层的输出上通过softmax()激活(每个节点)。最小化了所有标记节点上的以下交叉熵损失(同时忽略未标记节点):
其中\(Y\)是具有标签的节点的集合,\(h_{ik}^{\left( L \right)}\)表示输出层有标签的第\(i\)个节点的第\(k\)个实体的预测值,\({{t}_{ik}}\)表示节点的真实标签。在实践中,使用(full-batch)梯度下降训练模型。模型示意图如图1b。
- 链路预测
本文引入图自动编码器模型,模型由实体编码器和评分函数(解码器)组成。编码器将每个实体\({{v}_{i}}\in V\)映射到实值向量\({{e}_{i}}\in {{\mathbb{R}}^{d}}\)。解码器根据顶点表示重建图的边,也就是解码器通过函数\(s:{{\mathbb{R}}^{d}}\times R\times {{\mathbb{R}}^{d}}\to \mathbb{R}\)对三元组(主语,关系,宾语)进行评分。大多数现有的链路预测方法都可以在这个框架下进行解释。
本文与他们的主要区别在于对编码器的依赖。以前的方法对每个\({{v}_{i}}\in V\)使用单一实值向量\({e}_{i}\)直接优化,本文通过\({{e}_{i}}=h_{i}^{\left( L \right)}\)的R-GCN编码器计算表征。链路预测模型如图1c所示。
在实验中使用DistMult因子分解作为评分函数,在DistMult中,每个关系r都与对角矩阵\({{R}_{r}}\in {{\mathbb{R}}^{d\times d}}\)相关联,三元组(\(s,r,o\))的得分为:
通过负采样训练模型。\(\omega\)个负样例,随机破环正例中的主语或者宾语。对交叉熵损失进行了优化:
其中\(T\)是实三元组损坏三元组的总集合,\(l\)是logistic sigmoid函数,\(y\)是示性函数,正三元组\(y=1\),负三元组\(y=0\).
- 代码
5.1 实体分类
# -*- coding: utf-8 -*-
import torch
import torch.nn as nn
import torch.nn.functional as F
from torchmetrics.functional import accuracy
import dgl
from dgl.data.rdf import AIFBDataset, MUTAGDataset, BGSDataset, AMDataset
from dgl.nn.pytorch import RelGraphConv
import argparse
class RGCN(nn.Module):
def __init__(self, num_nodes, h_dim, out_dim, num_rels):
super().__init__()
self.emb = nn.Embedding(num_nodes, h_dim)
# two-layer RGCN
self.conv1 = RelGraphConv(h_dim, h_dim, num_rels, regularizer='basis',
num_bases=num_rels, self_loop=False)
self.conv2 = RelGraphConv(h_dim, out_dim, num_rels, regularizer='basis',
num_bases=num_rels, self_loop=False)
def forward(self, g):
x = self.emb.weight
h = F.relu(self.conv1(g, x, g.edata[dgl.ETYPE], g.edata['norm']))
h = self.conv2(g, h, g.edata[dgl.ETYPE], g.edata['norm'])
return h
def evaluate(g, target_idx, labels, test_mask, model):
test_idx = torch.nonzero(test_mask, as_tuple=False).squeeze()
model.eval()
with torch.no_grad():
logits = model(g)
logits = logits[target_idx]
return accuracy(logits[test_idx].argmax(dim=1), labels[test_idx]).item()
def train(g, target_idx, labels, train_mask, model):
# define train idx, loss function and optimizer
train_idx = torch.nonzero(train_mask, as_tuple=False).squeeze()
loss_fcn = nn.CrossEntropyLoss()
optimizer = torch.optim.Adam(model.parameters(), lr=1e-2, weight_decay=5e-4)
model.train()
for epoch in range(50):
logits = model(g)
logits = logits[target_idx]
loss = loss_fcn(logits[train_idx], labels[train_idx])
optimizer.zero_grad()
loss.backward()
optimizer.step()
acc = accuracy(logits[train_idx].argmax(dim=1), labels[train_idx]).item()
print("Epoch {:05d} | Loss {:.4f} | Train Accuracy {:.4f} "
.format(epoch, loss.item(), acc))
if __name__ == '__main__':
parser = argparse.ArgumentParser(description='RGCN for entity classification')
parser.add_argument("--dataset", type=str, default="aifb",
help="Dataset name ('aifb', 'mutag', 'bgs', 'am').")
args = parser.parse_args()
device = torch.device('cuda' if torch.cuda.is_available() else 'cpu')
print(f'Training with DGL built-in RGCN module.')
# load and preprocess dataset
if args.dataset == 'aifb':
data = AIFBDataset()
elif args.dataset == 'mutag':
data = MUTAGDataset()
elif args.dataset == 'bgs':
data = BGSDataset()
elif args.dataset == 'am':
data = AMDataset()
else:
raise ValueError('Unknown dataset: {}'.format(args.dataset))
g = data[0]
g = g.int().to(device)
num_rels = len(g.canonical_etypes)
category = data.predict_category
labels = g.nodes[category].data.pop('labels')
train_mask = g.nodes[category].data.pop('train_mask')
test_mask = g.nodes[category].data.pop('test_mask')
# calculate normalization weight for each edge, and find target category and node id
for cetype in g.canonical_etypes:
g.edges[cetype].data['norm'] = dgl.norm_by_dst(g, cetype).unsqueeze(1)
category_id = g.ntypes.index(category)
g = dgl.to_homogeneous(g, edata=['norm'])
node_ids = torch.arange(g.num_nodes()).to(device)
target_idx = node_ids[g.ndata[dgl.NTYPE] == category_id]
# create RGCN model
in_size = g.num_nodes() # featureless with one-hot encoding
out_size = data.num_classes
model = RGCN(in_size, 16, out_size, num_rels).to(device)
train(g, target_idx, labels, train_mask, model)
acc = evaluate(g, target_idx, labels, test_mask, model)
print("Test accuracy {:.4f}".format(acc))
5.2 链路预测(代码报错,有待修改)
# -*- coding: utf-8 -*-
import numpy as np
import torch
import torch.nn as nn
import torch.nn.functional as F
import dgl
from dgl.data.knowledge_graph import FB15k237Dataset
from dgl.dataloading import GraphDataLoader
from dgl.nn.pytorch import RelGraphConv
import tqdm
# for building training/testing graphs
def get_subset_g(g, mask, num_rels, bidirected=False):
src, dst = g.edges()
sub_src = src[mask]
sub_dst = dst[mask]
sub_rel = g.edata['etype'][mask]
if bidirected:
sub_src, sub_dst = torch.cat([sub_src, sub_dst]), torch.cat([sub_dst, sub_src])
sub_rel = torch.cat([sub_rel, sub_rel + num_rels])
sub_g = dgl.graph((sub_src, sub_dst), num_nodes=g.num_nodes())
sub_g.edata[dgl.ETYPE] = sub_rel
return sub_g
class GlobalUniform:
def __init__(self, g, sample_size):
self.sample_size = sample_size
self.eids = np.arange(g.num_edges())
def sample(self):
return torch.from_numpy(np.random.choice(self.eids, self.sample_size))
class NegativeSampler:
def __init__(self, k=10): # negative sampling rate = 10
self.k = k
def sample(self, pos_samples, num_nodes):
batch_size = len(pos_samples)
neg_batch_size = batch_size * self.k
neg_samples = np.tile(pos_samples, (self.k, 1))
values = np.random.randint(num_nodes, size=neg_batch_size)
choices = np.random.uniform(size=neg_batch_size)
subj = choices > 0.5
obj = choices <= 0.5
neg_samples[subj, 0] = values[subj]
neg_samples[obj, 2] = values[obj]
samples = np.concatenate((pos_samples, neg_samples))
# binary labels indicating positive and negative samples
labels = np.zeros(batch_size * (self.k + 1), dtype=np.float32)
labels[:batch_size] = 1
return torch.from_numpy(samples), torch.from_numpy(labels)
class SubgraphIterator:
def __init__(self, g, num_rels, sample_size=30000, num_epochs=6000):
self.g = g
self.num_rels = num_rels
self.sample_size = sample_size
self.num_epochs = num_epochs
self.pos_sampler = GlobalUniform(g, sample_size)
self.neg_sampler = NegativeSampler()
def __len__(self):
return self.num_epochs
def __getitem__(self, i):
eids = self.pos_sampler.sample()
src, dst = self.g.find_edges(eids)
src, dst = src.numpy(), dst.numpy()
rel = self.g.edata[dgl.ETYPE][eids].numpy()
# relabel nodes to have consecutive node IDs
uniq_v, edges = np.unique((src, dst), return_inverse=True)
num_nodes = len(uniq_v)
# edges is the concatenation of src, dst with relabeled ID
src, dst = np.reshape(edges, (2, -1))
relabeled_data = np.stack((src, rel, dst)).transpose()
samples, labels = self.neg_sampler.sample(relabeled_data, num_nodes)
# use only half of the positive edges
chosen_ids = np.random.choice(np.arange(self.sample_size),
size=int(self.sample_size / 2),
replace=False)
src = src[chosen_ids]
dst = dst[chosen_ids]
rel = rel[chosen_ids]
src, dst = np.concatenate((src, dst)), np.concatenate((dst, src))
rel = np.concatenate((rel, rel + self.num_rels))
sub_g = dgl.graph((src, dst), num_nodes=num_nodes)
sub_g.edata[dgl.ETYPE] = torch.from_numpy(rel)
sub_g.edata['norm'] = dgl.norm_by_dst(sub_g).unsqueeze(-1)
uniq_v = torch.from_numpy(uniq_v).view(-1).long()
return sub_g, uniq_v, samples, labels
class RGCN(nn.Module):
def __init__(self, num_nodes, h_dim, num_rels):
super().__init__()
# two-layer RGCN
self.emb = nn.Embedding(num_nodes, h_dim)
self.conv1 = RelGraphConv(h_dim, h_dim, num_rels, regularizer='bdd',
num_bases=100, self_loop=True)
self.conv2 = RelGraphConv(h_dim, h_dim, num_rels, regularizer='bdd',
num_bases=100, self_loop=True)
self.dropout = nn.Dropout(0.2)
def forward(self, g, nids):
x = self.emb(nids)
h = F.relu(self.conv1(g, x, g.edata[dgl.ETYPE], g.edata['norm']))
h = self.dropout(h)
h = self.conv2(g, h, g.edata[dgl.ETYPE], g.edata['norm'])
return self.dropout(h)
class LinkPredict(nn.Module):
def __init__(self, num_nodes, num_rels, h_dim = 500, reg_param=0.01):
super().__init__()
self.rgcn = RGCN(num_nodes, h_dim, num_rels * 2)
self.reg_param = reg_param
self.w_relation = nn.Parameter(torch.Tensor(num_rels, h_dim))
nn.init.xavier_uniform_(self.w_relation,
gain=nn.init.calculate_gain('relu'))
def calc_score(self, embedding, triplets):
s = embedding[triplets[:,0]]
r = self.w_relation[triplets[:,1]]
o = embedding[triplets[:,2]]
score = torch.sum(s * r * o, dim=1)
return score
def forward(self, g, nids):
return self.rgcn(g, nids)
def regularization_loss(self, embedding):
return torch.mean(embedding.pow(2)) + torch.mean(self.w_relation.pow(2))
def get_loss(self, embed, triplets, labels):
# each row in the triplets is a 3-tuple of (source, relation, destination)
score = self.calc_score(embed, triplets)
predict_loss = F.binary_cross_entropy_with_logits(score, labels)
reg_loss = self.regularization_loss(embed)
return predict_loss + self.reg_param * reg_loss
def filter(triplets_to_filter, target_s, target_r, target_o, num_nodes, filter_o=True):
"""Get candidate heads or tails to score"""
target_s, target_r, target_o = int(target_s), int(target_r), int(target_o)
# Add the ground truth node first
if filter_o:
candidate_nodes = [target_o]
else:
candidate_nodes = [target_s]
for e in range(num_nodes):
triplet = (target_s, target_r, e) if filter_o else (e, target_r, target_o)
# Do not consider a node if it leads to a real triplet
if triplet not in triplets_to_filter:
candidate_nodes.append(e)
return torch.LongTensor(candidate_nodes)
def perturb_and_get_filtered_rank(emb, w, s, r, o, test_size, triplets_to_filter, filter_o=True):
"""Perturb subject or object in the triplets"""
num_nodes = emb.shape[0]
ranks = []
for idx in tqdm.tqdm(range(test_size), desc="Evaluate"):
target_s = s[idx]
target_r = r[idx]
target_o = o[idx]
candidate_nodes = filter(triplets_to_filter, target_s, target_r,
target_o, num_nodes, filter_o=filter_o)
if filter_o:
emb_s = emb[target_s]
emb_o = emb[candidate_nodes]
else:
emb_s = emb[candidate_nodes]
emb_o = emb[target_o]
target_idx = 0
emb_r = w[target_r]
emb_triplet = emb_s * emb_r * emb_o
scores = torch.sigmoid(torch.sum(emb_triplet, dim=1))
_, indices = torch.sort(scores, descending=True)
rank = int((indices == target_idx).nonzero())
ranks.append(rank)
return torch.LongTensor(ranks)
def calc_mrr(emb, w, test_mask, triplets_to_filter, batch_size=100, filter=True):
with torch.no_grad():
test_triplets = triplets_to_filter[test_mask]
s, r, o = test_triplets[:,0], test_triplets[:,1], test_triplets[:,2]
test_size = len(s)
triplets_to_filter = {tuple(triplet) for triplet in triplets_to_filter.tolist()}
ranks_s = perturb_and_get_filtered_rank(emb, w, s, r, o, test_size,
triplets_to_filter, filter_o=False)
ranks_o = perturb_and_get_filtered_rank(emb, w, s, r, o,
test_size, triplets_to_filter)
ranks = torch.cat([ranks_s, ranks_o])
ranks += 1 # change to 1-indexed
mrr = torch.mean(1.0 / ranks.float()).item()
return mrr
def train(dataloader, test_g, test_nids, test_mask, triplets, device, model_state_file, model):
optimizer = torch.optim.Adam(model.parameters(), lr=1e-2)
best_mrr = 0
for epoch, batch_data in enumerate(dataloader): # single graph batch
model.train()
g, train_nids, edges, labels = batch_data
g = g.to(device)
train_nids = train_nids.to(device)
edges = edges.to(device)
labels = labels.to(device)
embed = model(g, train_nids)
loss = model.get_loss(embed, edges, labels)
optimizer.zero_grad()
loss.backward()
nn.utils.clip_grad_norm_(model.parameters(), max_norm=1.0) # clip gradients
optimizer.step()
print("Epoch {:04d} | Loss {:.4f} | Best MRR {:.4f}".format(epoch, loss.item(), best_mrr))
if (epoch + 1) % 500 == 0:
# perform validation on CPU because full graph is too large
model = model.cpu()
model.eval()
embed = model(test_g, test_nids)
mrr = calc_mrr(embed, model.w_relation, test_mask, triplets,
batch_size=500)
# save best model
if best_mrr < mrr:
best_mrr = mrr
torch.save({'state_dict': model.state_dict(), 'epoch': epoch}, model_state_file)
model = model.to(device)
if __name__ == '__main__':
device = torch.device('cuda' if torch.cuda.is_available() else 'cpu')
print(f'Training with DGL built-in RGCN module')
# load and preprocess dataset
data = FB15k237Dataset(reverse=False)
g = data[0]
num_nodes = g.num_nodes()
num_rels = data.num_rels
train_g = get_subset_g(g, g.edata['train_mask'], num_rels)
test_g = get_subset_g(g, g.edata['train_mask'], num_rels, bidirected=True)
test_g.edata['norm'] = dgl.norm_by_dst(test_g).unsqueeze(-1)
test_nids = torch.arange(0, num_nodes)
test_mask = g.edata['test_mask']
subg_iter = SubgraphIterator(train_g, num_rels) # uniform edge sampling
dataloader = GraphDataLoader(subg_iter, batch_size=1, collate_fn=lambda x: x[0])
# Prepare data for metric computation
src, dst = g.edges()
triplets = torch.stack([src, g.edata['etype'], dst], dim=1)
# create RGCN model
model = LinkPredict(num_nodes, num_rels).to(device)
# train
model_state_file = 'model_state.pth'
train(dataloader, test_g, test_nids, test_mask, triplets, device, model_state_file, model)
# testing
print("Testing...")
checkpoint = torch.load(model_state_file)
model = model.cpu() # test on CPU
model.eval()
model.load_state_dict(checkpoint['state_dict'])
embed = model(test_g, test_nids)
best_mrr = calc_mrr(embed, model.w_relation, test_mask, triplets,
batch_size=500)
print("Best MRR {:.4f} achieved using the epoch {:04d}".format(best_mrr, checkpoint['epoch']))