《算法图解》第七章笔记_迪杰斯特拉算法
软件环境:Python 3.7.0b4
一、迪杰斯特拉(dijkstras)算法介绍
算法目标:找出一个图中最快(耗时最短)的路径。
实现步骤:
- 找出最短时间内前往的节点;
- 对于该节点的邻居,检查是否有前往它们的更短路径,如果有,就更新其开销;
- 重复这个过程,直到对图中的每个节点都重复了以上两个步骤;
- 计算最终路径。
二、迪杰斯特拉算法术语介绍
迪杰斯特拉算法用于每条边都有关联数字的图,这些数字称为权重(weight)。
带权重的图称为加权图(weighted graph),不带权重的图称为非加权图(unweighted graph)
要计算非加权图中的最短路径,可使用广度优先搜索。要计算加权图中的最短路径,可使用狄克斯特拉算法。
三、算法实现
以下图为例
要解决这个问题,需要先画出三个散列表:
随着算法的进行,我们将不断更新散列表costs和parents。
graph = {} #首先需要实现这个图
需要同时存储邻居和前往邻居的开销
graph["start"] = {} graph["start"]["a"] = 6 graph["start"]["b"] = 2
同时还需要用一个散列表来存储每个节点的开销,一个存储父节点的散列表,一个数组。
下面来看看算法的执行过程:
完整代码如下(Python)
# 添加节点和邻居 graph = {} graph["start"] = {} graph["start"]["a"] = 6 graph["start"]["b"] = 2 graph["a"] = {} graph["a"]["fin"] = 1 graph["b"] = {} graph["b"]["a"] = 3 graph["b"]["fin"] = 5 graph["fin"] = {} # 终点没有邻居 # 存储每个节点开销的散列表 infinity = float("inf") costs = {} costs["a"] = 6 costs["b"] = 2 costs["fin"] = infinity # 存储父节点的散列表 parents = {} parents["a"] = "start" parents["b"] = "start" parents["fin"] = None processed = [] # 一个数组,用于记录处理过的节点。因为对于同一个节点,不用处理多次。 def find_lowest_cost_node(costs): lowest_cost = float("inf") lowest_cost_node = None # 遍历所有的节点 for node in costs: cost = costs[node] # 如果当前节点的开销更低且未处理过 if cost < lowest_cost and node not in processed: # 就将其视为开销最低的节点 lowest_cost = cost lowest_cost_node = node return lowest_cost_node # 在未处理的节点中找出开销最小的节点 node = find_lowest_cost_node(costs) # 这个while循环在所有节点都被处理过后结束 while node is not None: cost = costs[node] # 遍历当前节点的所有邻居 neighbors = graph[node] for n in neighbors.keys(): new_cost = cost + neighbors[n] # 如果经当前节点前往该邻居更近 if costs[n] > new_cost: # 就更新该邻居的开销 costs[n] = new_cost # 同时将该邻居的父节点设置为当前节点 parents[n] = node # 将当前节点标记为处理过 processed.append(node) # 找出接下来要处理的节点,并做循环 node = find_lowest_cost_node(costs) print ("Cost from the start to each node:") print (costs)
四、小结
- 广度有限搜索用于在非加权图中查找最短路径。
- 迪杰斯特拉算法用于在加权图中查找最短路径。
- 仅当权重为正时迪杰斯特拉算法才管用。
- 如果图中包含负权边,考虑使用贝尔曼-福德(Bellman-Ford)算法。
版权声明:本文版权归作者和博客园共有,欢迎转载,但未经作者同意必须保留此段声明。
特此声明:所有评论和私信都会在第一时间回复。也欢迎园子里和园子外的大大们指正错误,共同进步。或者直接私信我 (^∀^)
声援博主:如果您觉得文章对您有帮助,可以点击文章右下角【推荐】一下。您的鼓励是作者坚持原创和持续写作的最大动力!
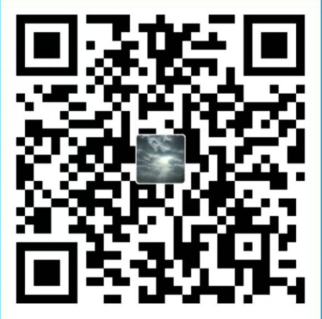
您的资助是我最大的动力!
金额随意,欢迎来赏!
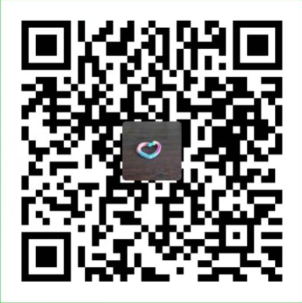
如果,您希望更容易地发现我的新博客,不妨点击一下绿色通道的
本博客的所有打赏均将用于博主女朋友的化妆品购买以及养肥计划O(∩_∩)O。我是【~不会飞的章鱼~】!