Referred to https://www.bilibili.com/video/BV17X4y1H7dK/?spm_id_from=333.337.search-card.all.click&vd_source=d312c66700fc64b66258a994f0a117ad
Referred to https://www.bilibili.com/video/BV17X4y1H7dK/?spm_id_from=333.337.search-card.all.click&vd_source=d312c66700fc64b66258a994f0a117ad
import torch
import numpy as np
torch.cuda.is_available()
True
1 Basic Operation
1.1 create tensor
# from list
torch.tensor([1,2]),torch.tensor([[1,2],[3,4]]),torch.tensor([1,2],dtype=float)
(tensor([1, 2]),
tensor([[1, 2],
[3, 4]]),
tensor([1., 2.], dtype=torch.float64))
# from numpy
torch.from_numpy(np.array([1,2])),torch.from_numpy(np.array([1.0,2.0]))
(tensor([1, 2], dtype=torch.int32), tensor([1., 2.], dtype=torch.float64))
print(torch.ones(2,3))
print(torch.zeros(2, 3))
print(torch.eye(3))
print(torch.full((2, 3), 3.14))
tensor([[1., 1., 1.],
[1., 1., 1.]])
tensor([[0., 0., 0.],
[0., 0., 0.]])
tensor([[1., 0., 0.],
[0., 1., 0.],
[0., 0., 1.]])
tensor([[3.1400, 3.1400, 3.1400],
[3.1400, 3.1400, 3.1400]])
print(torch.empty(2, 3))
print(torch.rand(2, 3))
print(torch.randn(2, 3))
print(torch.randint(1, 100, (2, 3)))
tensor([[3.1400, 3.1400, 3.1400],
[3.1400, 3.1400, 3.1400]])
tensor([[0.6569, 0.8155, 0.0479],
[0.6782, 0.2530, 0.6569]])
tensor([[-1.2733, 0.2047, 0.5430],
[-0.2978, -1.0526, 0.6567]])
tensor([[80, 59, 75],
[95, 48, 94]])
print(torch.arange(0, 10, 2))
print(torch.arange(0, 10, 2).dtype)
print(torch.arange(0.0, 10.0, 2).dtype)
print(torch.linspace(0, 10, 3))
print(torch.linspace(0, 10, 3).dtype)
tensor([0, 2, 4, 6, 8])
torch.int64
torch.float32
tensor([ 0., 5., 10.])
torch.float32
print(torch.arange(0, 10, 2).to(torch.float64).dtype)
torch.float64
1.2 tensor index
# Create test data a
# 4 images, 3 channels, 28*28 pixels
a = torch.zeros(4,3,28,28)
a.size(),a.shape
(torch.Size([4, 3, 28, 28]), torch.Size([4, 3, 28, 28]))
# View 0th picture
print(a[0].shape)
# View the 0th image,the 0th channel
print(a[0, 0].shape)
# View the 0th image,the 0th channel,the 2nd row
print(a[0, 0, 2].shape)
# View the 0th image,the 0th channel,the 2nd row and 4th column
print(a[0, 0, 2, 4].shape)
torch.Size([3, 28, 28])
torch.Size([28, 28])
torch.Size([28])
torch.Size([])
#Slice
#View 0-1 images
print(a[:2].shape)
#View 0-1 channels of 0-1 images
print(a[:2, :2].shape)
#View the inverse 5-positive 24 rows of all channels of 0-1 images
print(a[:2, :, -5:25].shape)
#Interval indexed
print(a[:, :, :, ::2].shape)
print(a[:, :, ::6, ::2].shape)
torch.Size([2, 3, 28, 28])
torch.Size([2, 2, 28, 28])
torch.Size([2, 3, 2, 28])
torch.Size([4, 3, 28, 14])
torch.Size([4, 3, 5, 14])
# Use ... to indicate multiple omitted
#Fetch columns 0-2 of all images
print(a[... , :2].shape)
#fetch rows 0-2 of all images
print(a[... , :2, :].shape)
# take the 2nd image
print(a[2, ...] .shape)
torch.Size([4, 3, 28, 2])
torch.Size([4, 3, 2, 28])
torch.Size([3, 28, 28])
a = torch.randn(4,1,28,28)
print(a.shape)
torch.Size([4, 1, 28, 28])
# view == reshape
print(a.reshape(4,1*28*28).shape)
print(a.reshape(4,784).reshape(4, 1, 28, 28).shape)
torch.Size([4, 784])
torch.Size([4, 1, 28, 28])
print(a.unsqueeze(0).shape)
print(a.unsqueeze(-1).shape)
torch.Size([1, 4, 1, 28, 28])
torch.Size([4, 1, 28, 28, 1])
print(a.squeeze(0).shape)
print(a.squeeze(1).shape)
torch.Size([4, 1, 28, 28])
torch.Size([4, 28, 28])
print(a.unsqueeze(-1).squeeze().shape)
torch.Size([4, 28, 28])
# repeat
print(torch.randn(11,2,1).repeat(3, 3, 3).shape)
torch.Size([33, 6, 3])
# Dimensional exchange
b = torch.randn(3,4)
print(b.t().shape)
c = b.unsqueeze(-1).repeat(1,1,5)
print(c.shape)
print(c.transpose(0,1).shape)
print(c.transpose(-1,-2).shape)
print(c.permute(2,1,0).shape)
torch.Size([4, 3])
torch.Size([3, 4, 5])
torch.Size([4, 3, 5])
torch.Size([3, 5, 4])
torch.Size([5, 4, 3])
1.4 broadcast
#broadcast
a = torch.zeros(2, 3)
b = torch.ones(1, 3)
b[0][1] = 0
c = torch.randn(1)
print(a)
print(b)
print(a+b)
print((a + b).shape)
print((a + c).shape)
print(b.expand_as(a).shape)
print(c.expand_as(a).shape)
tensor([[0., 0., 0.],
[0., 0., 0.]])
tensor([[1., 0., 1.]])
tensor([[1., 0., 1.],
[1., 0., 1.]])
torch.Size([2, 3])
torch.Size([2, 3])
torch.Size([2, 3])
torch.Size([2, 3])
1.5 Splice and Split
a = torch.rand(4,2,3)
b = torch.rand(1,2,3)
torch.cat((a,b),dim=0).shape
torch.Size([5, 2, 3])
a = torch.rand(4,2,3)
b = torch.rand(4,2,3)
torch.stack((a, b), dim=0).shape
torch.Size([2, 4, 2, 3])
a = torch.rand(12, 32, 8)
_1, _2, _3,_4 = a.split(3, dim=0)
print(_1.shape)
print(_2.shape)
_1, _2, _3 = a.split([4, 2, 6], dim=0)
print(_1.shape)
print(_2.shape)
print(_3.shape)
torch.Size([3, 32, 8])
torch.Size([3, 32, 8])
torch.Size([4, 32, 8])
torch.Size([2, 32, 8])
torch.Size([6, 32, 8])
1.6 math
a = torch.FloatTensor([[0, 1, 2], [3, 4, 5]])
b = torch.FloatTensor([[0, 1, 2], [3, 4, 5],[6, 7, 8]])
a, b
(tensor([[0., 1., 2.],
[3., 4., 5.]]),
tensor([[0., 1., 2.],
[3., 4., 5.],
[6., 7., 8.]]))
print(a @ b)
print(a.matmul(b))
tensor([[15., 18., 21.],
[42., 54., 66.]])
tensor([[15., 18., 21.],
[42., 54., 66.]])
a = torch.FloatTensor([[0, 1, 2], [3, 4, 5]])
b = torch.FloatTensor([0, 1, 2])
print(a + b)
print(a - b)
print(a * b)
print(a / b)
tensor([[0., 2., 4.],
[3., 5., 7.]])
tensor([[0., 0., 0.],
[3., 3., 3.]])
tensor([[ 0., 1., 4.],
[ 0., 4., 10.]])
tensor([[ nan, 1.0000, 1.0000],
[ inf, 4.0000, 2.5000]])
print(a**2)
print(a**0.5)
print(a.exp())
print(a.log())
print(a.log2())
tensor([[ 0., 1., 4.],
[ 9., 16., 25.]])
tensor([[0.0000, 1.0000, 1.4142],
[1.7321, 2.0000, 2.2361]])
tensor([[ 1.0000, 2.7183, 7.3891],
[ 20.0855, 54.5981, 148.4132]])
tensor([[ -inf, 0.0000, 0.6931],
[1.0986, 1.3863, 1.6094]])
tensor([[ -inf, 0.0000, 1.0000],
[1.5850, 2.0000, 2.3219]])
# limit the upper and lower limits
a.clamp(2, 4)
tensor([[2., 2., 2.],
[3., 4., 4.]])
print(a > b)
print(a < b)
print(a == b)
print(a != b)
tensor([[False, False, False],
[ True, True, True]])
tensor([[False, False, False],
[False, False, False]])
tensor([[ True, True, True],
[False, False, False]])
tensor([[False, False, False],
[ True, True, True]])
c = torch.FloatTensor([3.14])
print(c.floor())
print(c.ceil())
print(c.round())
tensor([3.])
tensor([4.])
tensor([3.])
1.7 statistics
a = torch.FloatTensor([[0., 1., 2.], [3., 4., 5.]])
a
tensor([[0., 1., 2.],
[3., 4., 5.]])
print(a.min())
print(a.max())
print(a.mean())
#product
print(a.prod())
print(a.sum())
print(a.argmax())
print(a.argmin())
tensor(0.)
tensor(5.)
tensor(2.5000)
tensor(0.)
tensor(15.)
tensor(5)
tensor(0)
b = torch.FloatTensor([[0., 1., 2.], [3., 4., 5.], [6., 7., 8.]])
b.max(dim=0),b.max(dim=1)
(torch.return_types.max(
values=tensor([6., 7., 8.]),
indices=tensor([2, 2, 2])),
torch.return_types.max(
values=tensor([2., 5., 8.]),
indices=tensor([2, 2, 2])))
b.argmax(dim=0),b.argmax(dim=1)
(tensor([2, 2, 2]), tensor([2, 2, 2]))
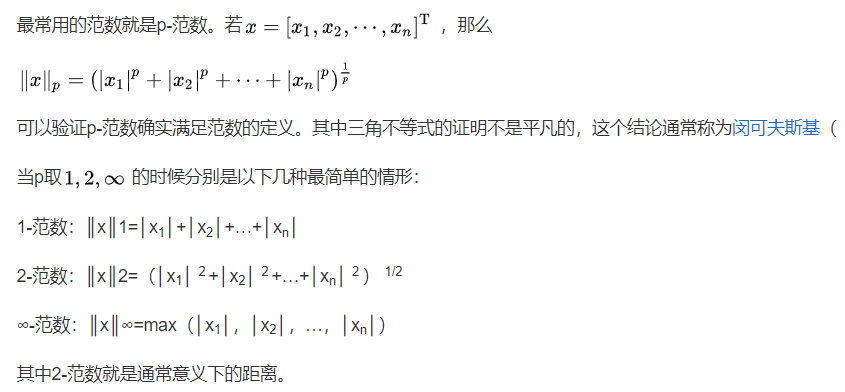
print(b.norm(1))
print(b.norm(1, dim=0))
print(b.norm(2))
print(b.norm(2, dim=0))
tensor(36.)
tensor([ 9., 12., 15.])
tensor(14.2829)
tensor([6.7082, 8.1240, 9.6437])
b.topk(2, dim=1, largest=False)
torch.return_types.topk(
values=tensor([[0., 1.],
[3., 4.],
[6., 7.]]),
indices=tensor([[0, 1],
[0, 1],
[0, 1]]))
b.kthvalue(3, dim=1)
torch.return_types.kthvalue(
values=tensor([2., 5., 8.]),
indices=tensor([2, 2, 2]))