STL测试3)优先级队列实现二叉堆
用法:
big_heap.empty();判断堆是否为空
big_heap.pop();弹出栈顶元素最大值
big_heap.push(x);将x添加到最大堆
big_heap.top();返回栈顶元素;
big_heap.size();返回堆中元素个数
简单的应用
#include<stdio.h> #include<queue> #include<vector> #include<functional> using namespace std; int main() { priority_queue <int> big_heap;//默认构造是最大堆 priority_queue<int, vector<int>, greater<int> > small_heap;//最小堆 priority_queue<int, vector<int>, less<int> > big_heap2;//最大堆 if(big_heap.empty()) { printf("big_heap is empty\n"); } int test[]={6,10,1,7,99,4,33}; for(int i=0;i< 7;i++) { big_heap.push(test[i]); small_heap.push(test[i]); printf("input %d,big_heap top is %d ,small_heap top is%d\n",test[i],big_heap.top(),small_heap.top()); } big_heap.push(1000); small_heap.push(0); printf("now big_heap top is %d\nnow small_heap top is %d\n",big_heap.top(),small_heap.top()); for(int i=0;i<3;i++) { big_heap.pop(); small_heap.pop(); } printf("now heap has %d num,the max is %d,the min is%d\n",big_heap.size(),big_heap.top(),small_heap.top()); return 0; }
下面来一个简单的应用
在未排序的数组中找到第 k 个最大的元素。请注意,你需要找的是数组排序后的第 k 个最大的元素,而不是第 k 个不同的元素。
示例 1:
输入: [3,2,1,5,6,4] 和 k = 2
输出: 5
示例 2:
输入: [3,2,3,1,2,4,5,5,6] 和 k = 4
输出: 4
说明:
你可以假设 k 总是有效的,且 1 ≤ k ≤ 数组的长度。
来源:力扣(LeetCode)
链接:https://leetcode-cn.com/problems/kth-largest-element-in-an-array
著作权归领扣网络所有。商业转载请联系官方授权,非商业转载请注明出处。
思路:
维护一个K大小的最小堆,如果堆中栈顶的元素<k,直接入堆;此外情况如果堆顶的元素小于新元素的时候,弹出堆顶,将新元素入堆。
这样保证堆外的元素都是比堆顶小的,不然会把该元素和堆顶的元素置换,这样保证最后堆里存的是k个最大的元素,且堆顶是这k个元素里最小的。那么堆顶倒数第k小的就是第k大的了。
代码写在下面。
下面是一个利用最大堆和最小堆求中位数的问题。
中位数是有序列表中间的数。如果列表长度是偶数,中位数则是中间两个数的平均值。
例如,
[2,3,4] 的中位数是 3
[2,3] 的中位数是 (2 + 3) / 2 = 2.5
设计一个支持以下两种操作的数据结构:
void addNum(int num) - 从数据流中添加一个整数到数据结构中。
double findMedian() - 返回目前所有元素的中位数。
示例:
addNum(1)
addNum(2)
findMedian() -> 1.5
addNum(3)
findMedian() -> 2
进阶:
如果数据流中所有整数都在 0 到 100 范围内,你将如何优化你的算法?
如果数据流中 99% 的整数都在 0 到 100 范围内,你将如何优化你的算法?
来源:力扣(LeetCode)
链接:https://leetcode-cn.com/problems/find-median-from-data-stream
著作权归领扣网络所有。商业转载请联系官方授权,非商业转载请注明出处。
思路就是设计一个最大堆和最小堆分别存储一般数据,并维持两个堆中最大堆的堆顶比最小堆小。这样如果两个堆大小相同,那么中位数就是两个堆顶的平均值,如果是堆大小不同,那么中位数就是size大的那个堆的堆顶。
看到一个别人画的示意图,非常形象,这里搬过来方便以后理解。
addNum 函数设计
addNum() 函数在添加元素的过程中保持两个堆的动态平衡:
Condition 1.保证两堆元素个数相差不超过 1
|
case 1:
- 如果两堆中的元素个数相同。这个时候无论插入哪一个堆,条件 1 都不会被破坏,因此考虑条件 2 ,将待插入元素与两堆的堆顶比较:若待插入元素为 5,显然这个时候若插入smaller会破坏条件 2,因此因插入bigger中。而若待插入为 9 则显然应插入 smaller 中。
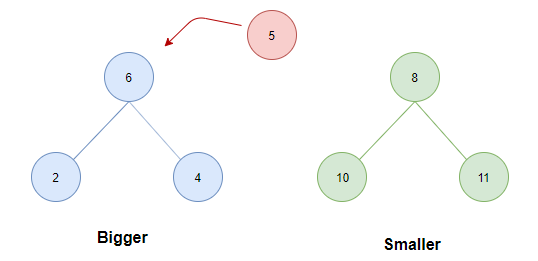
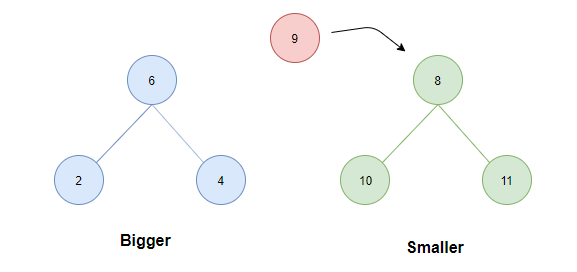
case 2:
- 如果大顶堆元素个数小于小顶堆的元素个数。此时,将待插入元素与两堆堆顶比较:
- 若小于等于Bigger.top则直接插入Bigger中;
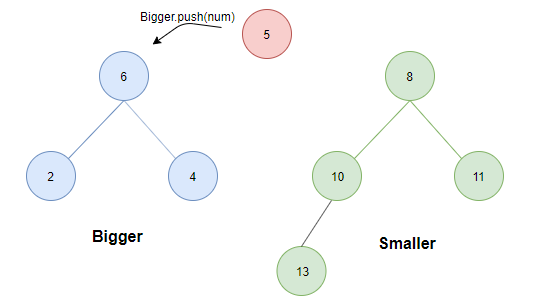
- 若大于smaller.top则为了保证条件1,需将smaller中的最小值(根)转存至Bigger中。
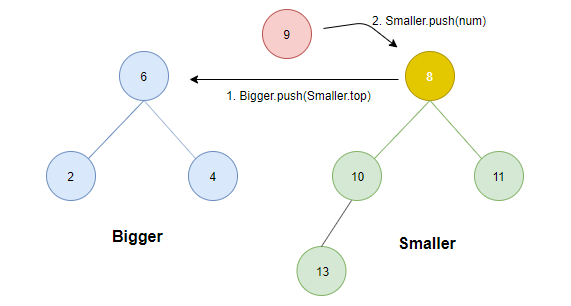
case 3:
- 如果大顶堆的元素个数大于小顶堆的元素个数。此时,将待插入元素与两堆堆顶比较:
- 若其大于等于Smaller.top则直接插入Smaller中;
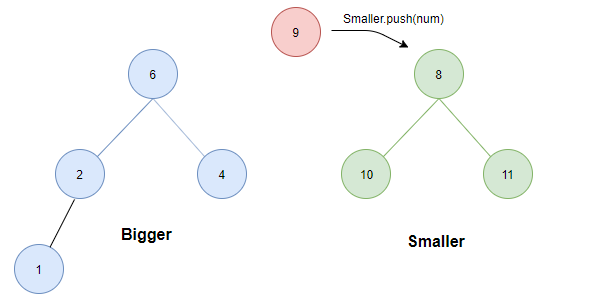
- 若小于Bigger.top则为了保证条件1,需将Bigger中的最大元素值(根)转存至Smaller中。
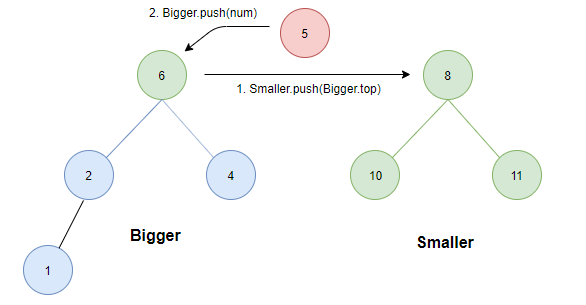
最后是实现以后的代码:
class MedianFinder { public: priority_queue <int> big_queue; priority_queue<int, vector <int>, greater <int> > small_queue; /** initialize your data structure here. */ MedianFinder() { } void addNum(int num) { if(big_queue.empty()) { big_queue.push(num); return; } if(big_queue.size() == small_queue.size()) { if(num < big_queue.top()) { big_queue.push(num); } else { small_queue.push(num); } } else if(big_queue.size() > small_queue.size()) { if(num > big_queue.top()) { small_queue.push(num); } else { small_queue.push(big_queue.top()); big_queue.pop(); big_queue.push(num); } } else if(big_queue.size() < small_queue.size()) { if(num < small_queue.top()) { big_queue.push(num); } else { big_queue.push(small_queue.top()); small_queue.pop(); small_queue.push(num); } } } double findMedian() { if(big_queue.size() == small_queue.size()) return ((big_queue.top()+small_queue.top())/2.0); else if(big_queue.size() > small_queue.size()) return big_queue.top(); else return small_queue.top(); } };
posted on 2020-01-18 23:19 KID_XiaoYuan 阅读(237) 评论(0) 编辑 收藏 举报