【Course】Machine learning:Week 3-logistics regression
Machine learning week3
一、 Classification and Representation
1、binary classification problem
2、Hypothesis Representation
Sigmoid Function = Logistic Function,公式如下:
\(h_\theta (x)\)will give us the probability that our output is 1.
3、Decision Boundary
决策边界:\(z = \theta^Tx=0\)
二、Logistic Regression Model
1、Cost Function
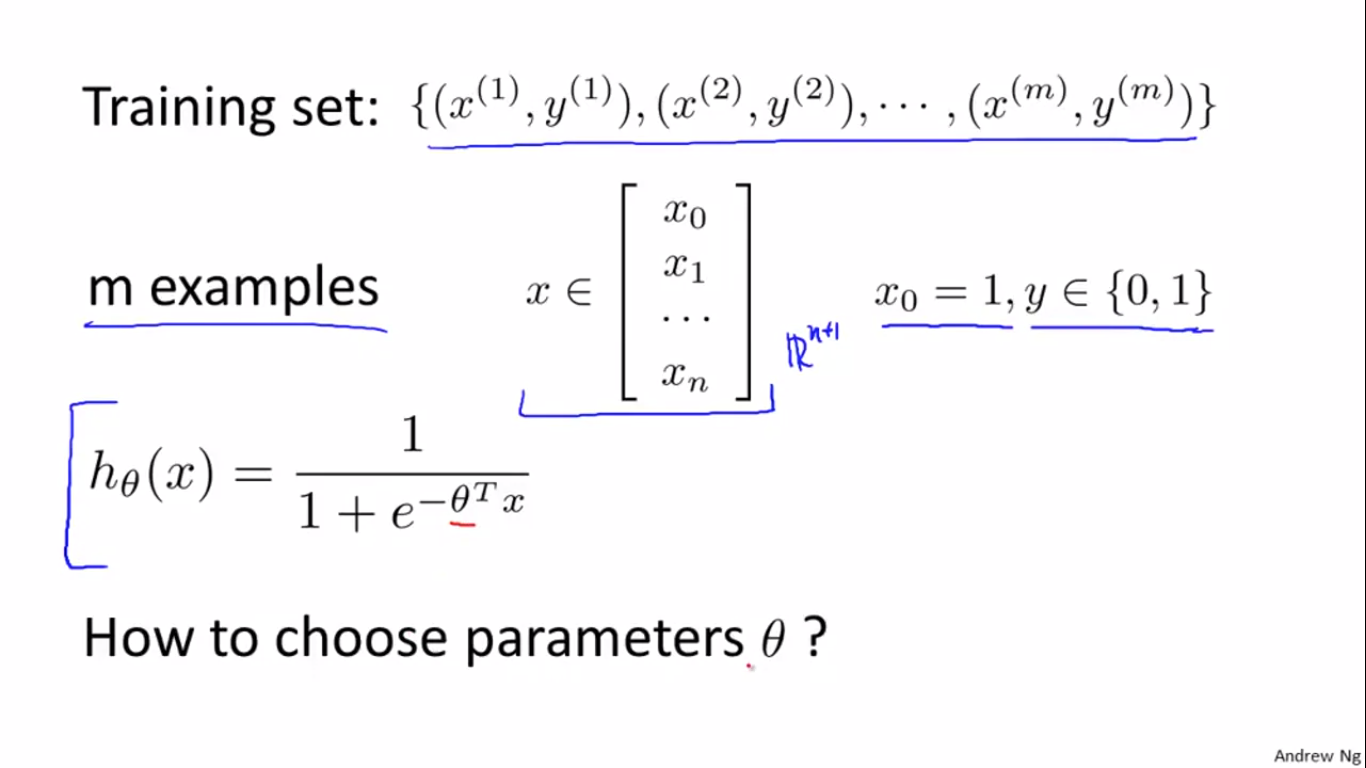
线性回归问题变成了logistic回归问题后,怎么去选择参数theta呢?
下图中,如果在logistic regression中还是用linear regression的cost function,会出现左图的cost function曲线,无法保证收敛到全局最优解。希望的是右边的convex型曲线。
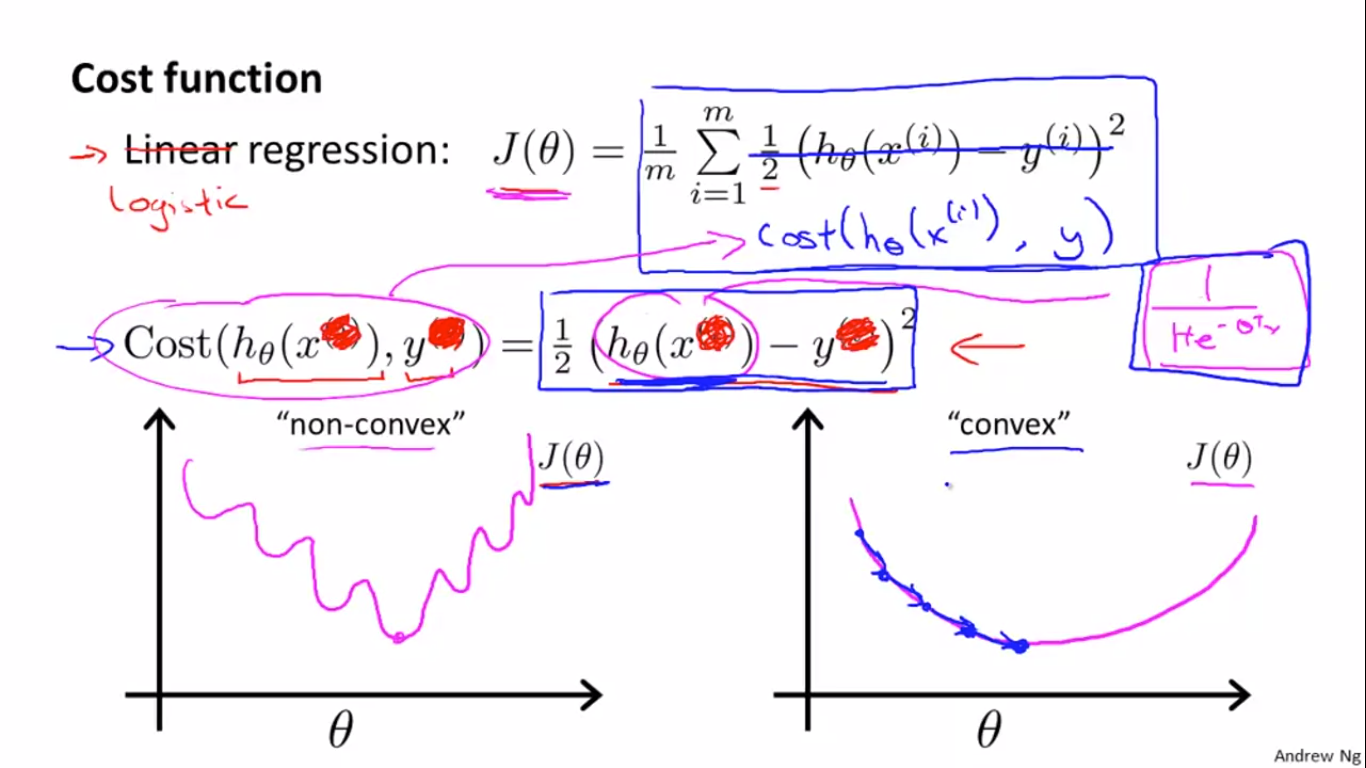
因此,logistic regression需要新的损失函数:
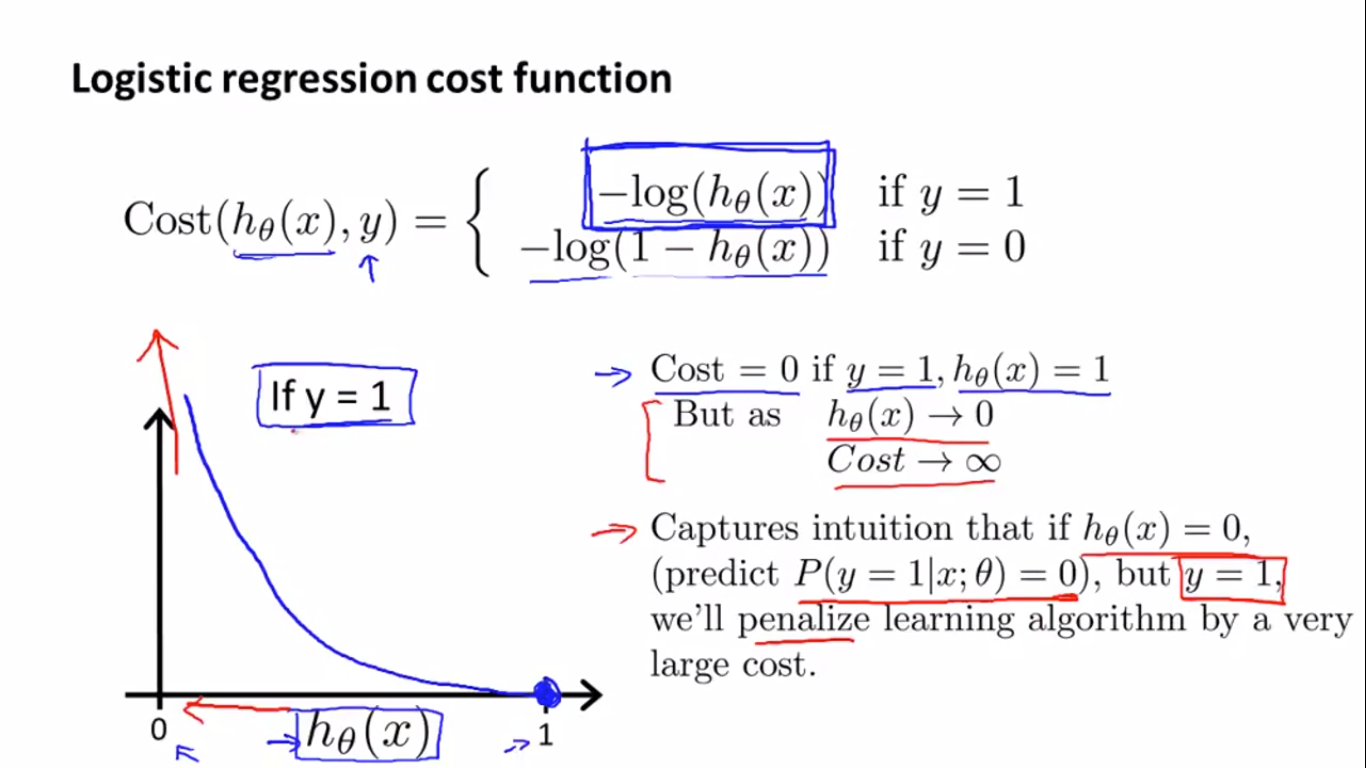
根据\(h_\theta (x)\)的定义,\(h_\theta (x)\)在[0,1]的范围内,根据log函数的曲线,就可以画出上图y=1时的损失函数\(-log(h_\theta (x))\)曲线,当y=1,\(h_\theta (x)=1\)时,证明预测值和真实值相同,Cost是等于0的。但是当\(h_\theta (x)\)-->0时,y=1时,预测错误,Cost就会很大。
下图是当y=0是的损失函数曲线:
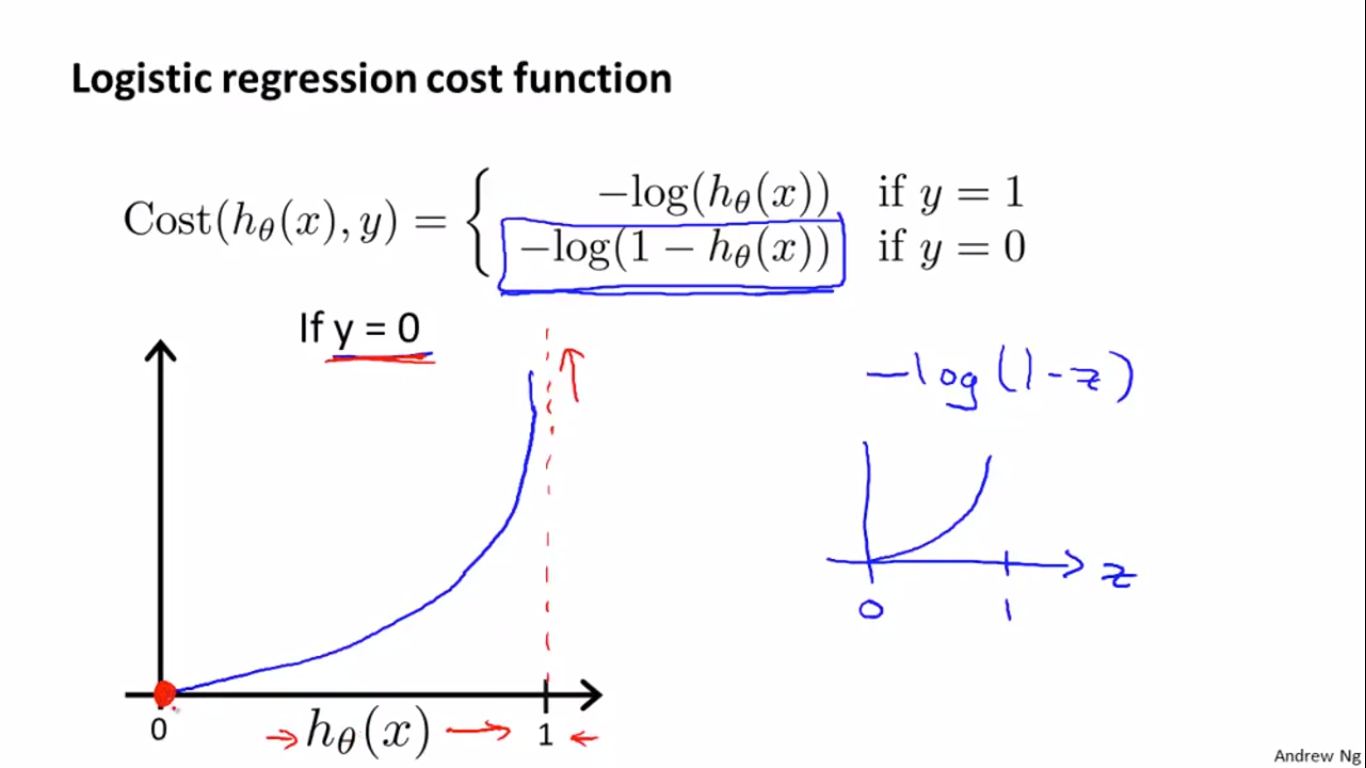
原理同y=1时,所以这个损失函数很适合于二分类的logistic regression。
2、Simplified Cost Function and Gradient Descent
logistic regression的损失函数可以合并到一个公式里面,其梯度下降如下,Repeat部分的内容和Linear regression差不多,但损失函数\(h_\theta(x)\)是不同的。
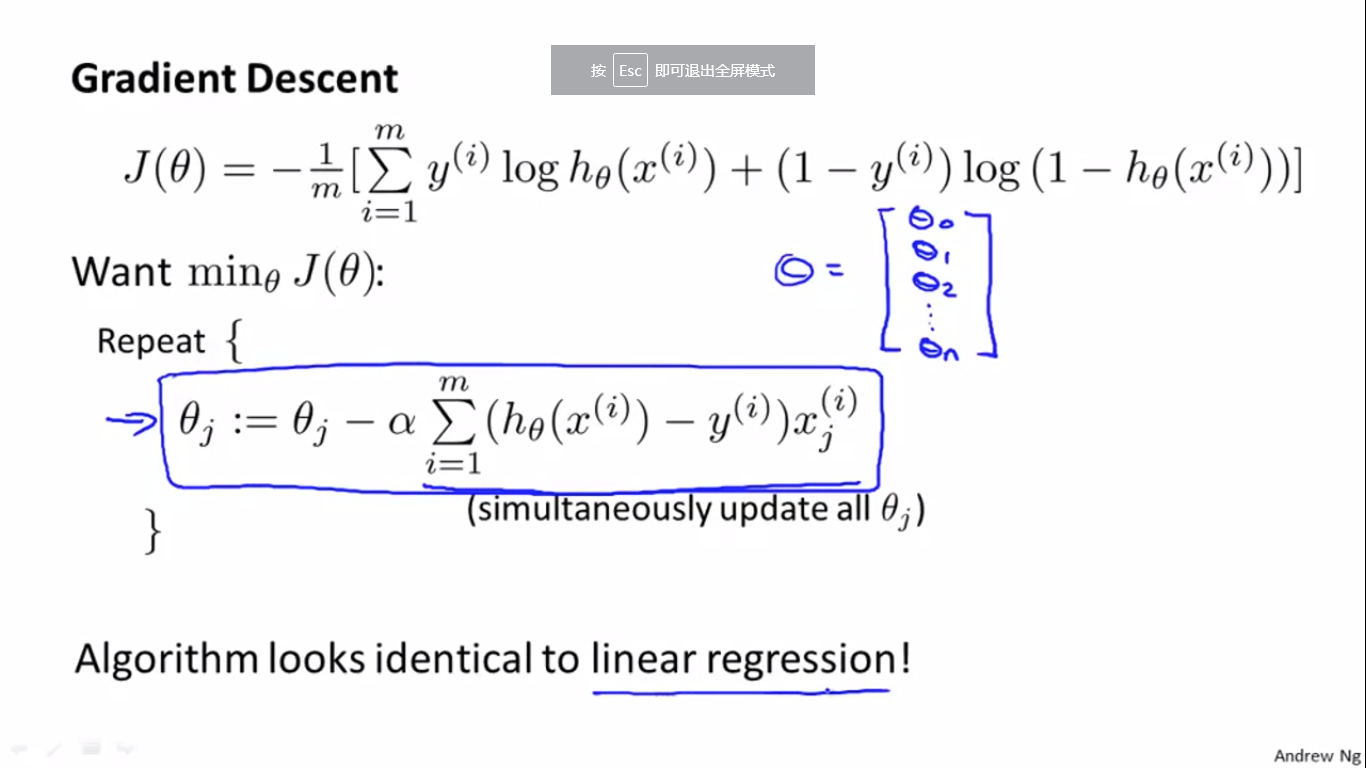
此外,和linear regression的vectorized implementation一样,logistic regression也有vectorized implementation。公式如下:
3、 Advanced Optimization
除了梯度下降,还有许多别的优化方法:
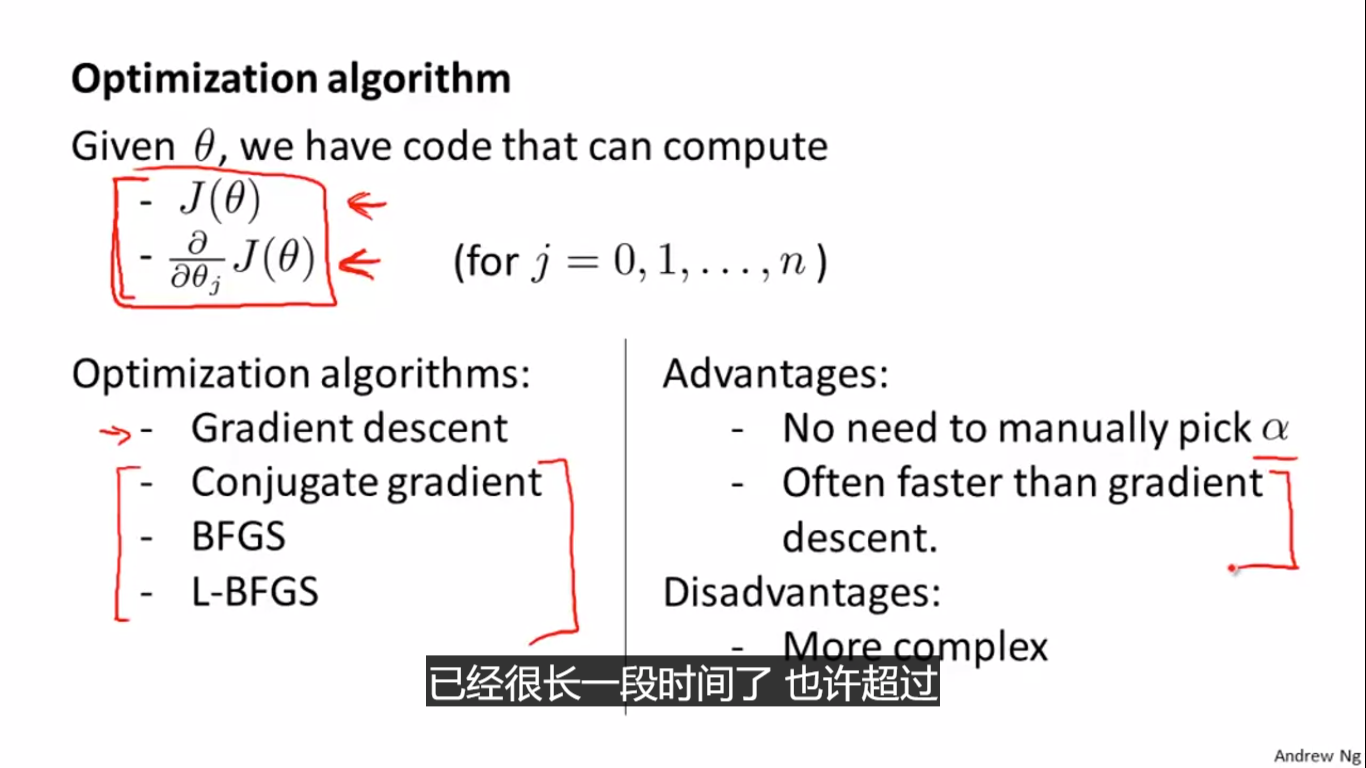
下图是调用方法:
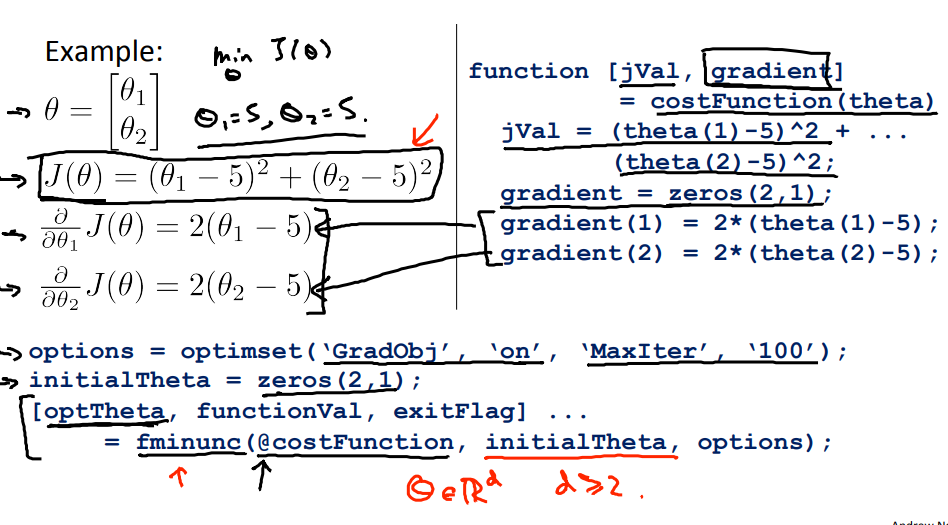
三、Multiclass Classification
1、Multiclass Classification: One-vs-all
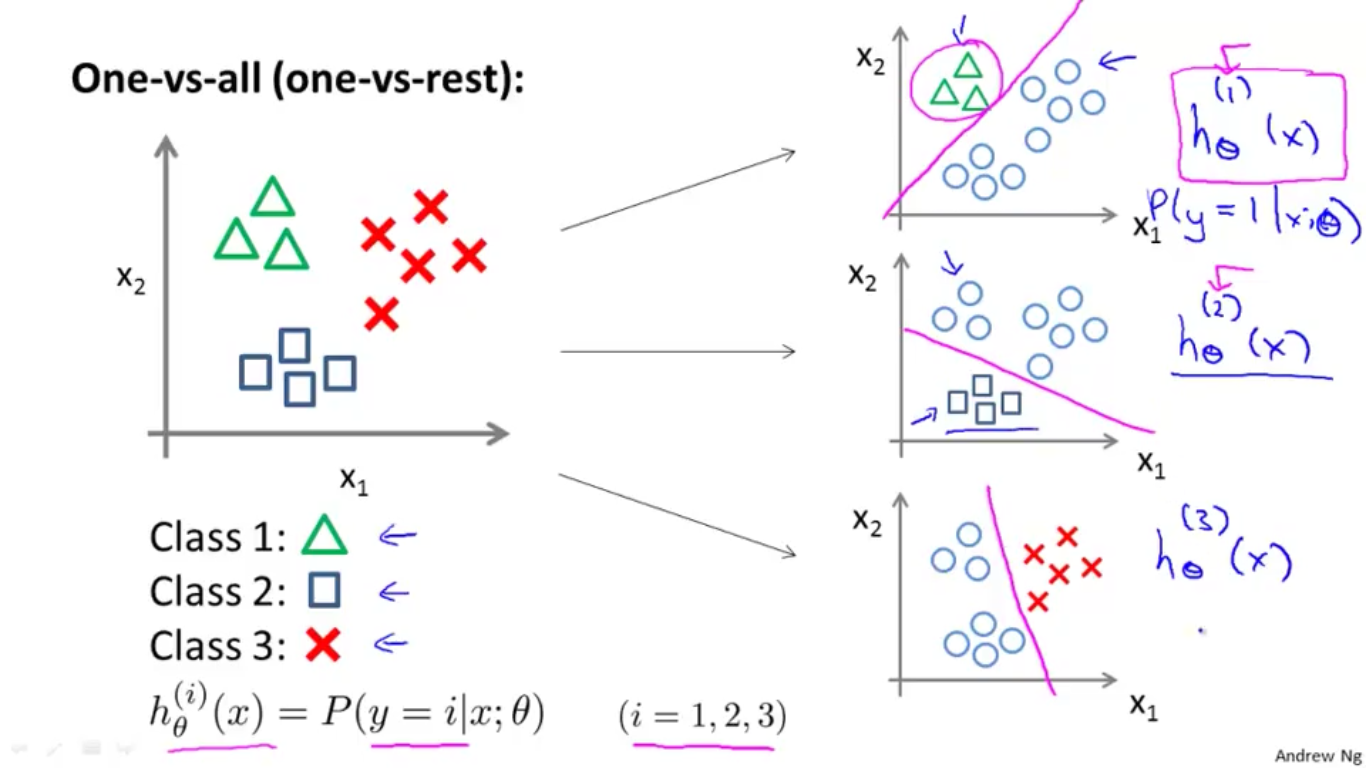
如上图,是一个一对多的分类。实际上是训练多个分类器,分别计算属于某一类的概率,再取概率最大的那个,如下图。
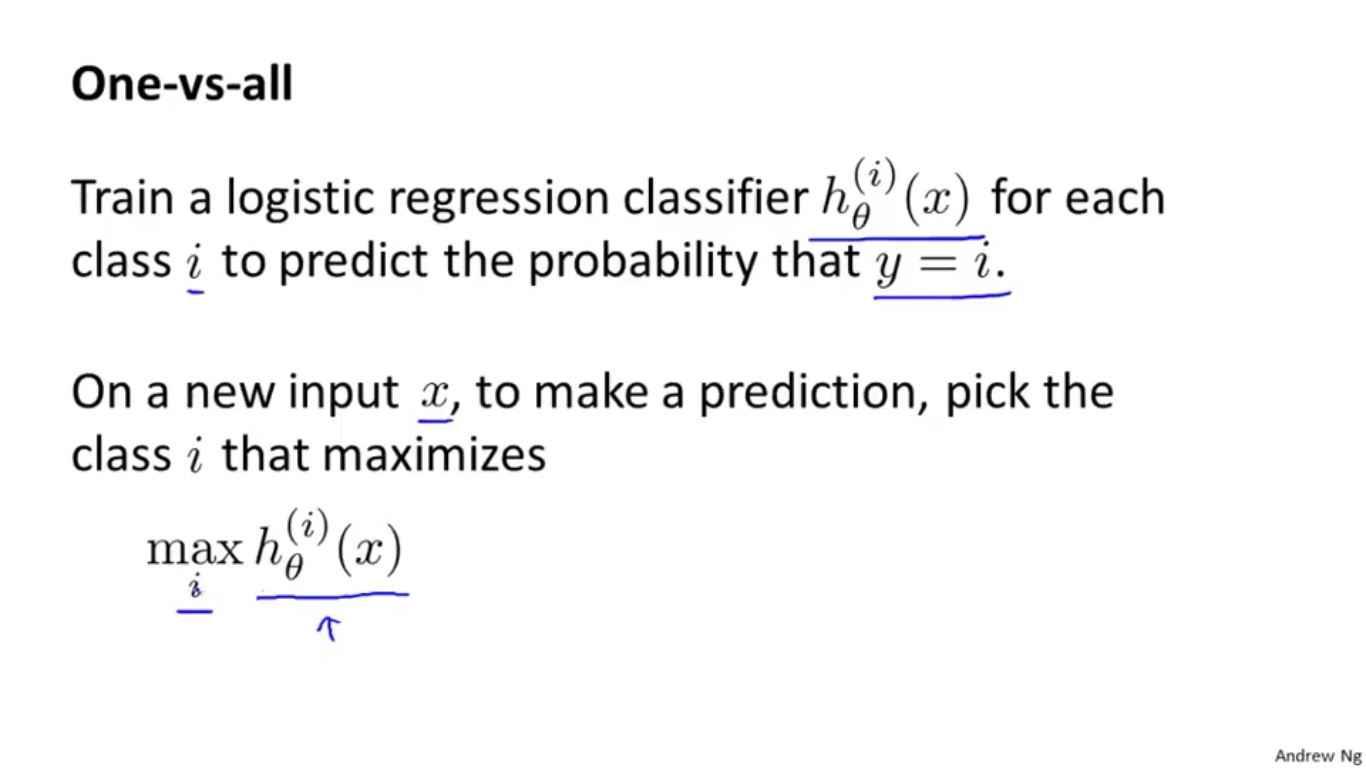
四、Solving the Problem of Overfitting
1、The Problem of Overfitting
There are two main options to address the issue of overfitting:
- Reduce the number of features:
- Manually select which features to keep.
- Use a model selection algorithm (studied later in the course).
- Regularization
- Keep all the features, but reduce the magnitude of parameters \(\theta_j\).
- Regularization works well when we have a lot of slightly useful features.
2、Cost Function
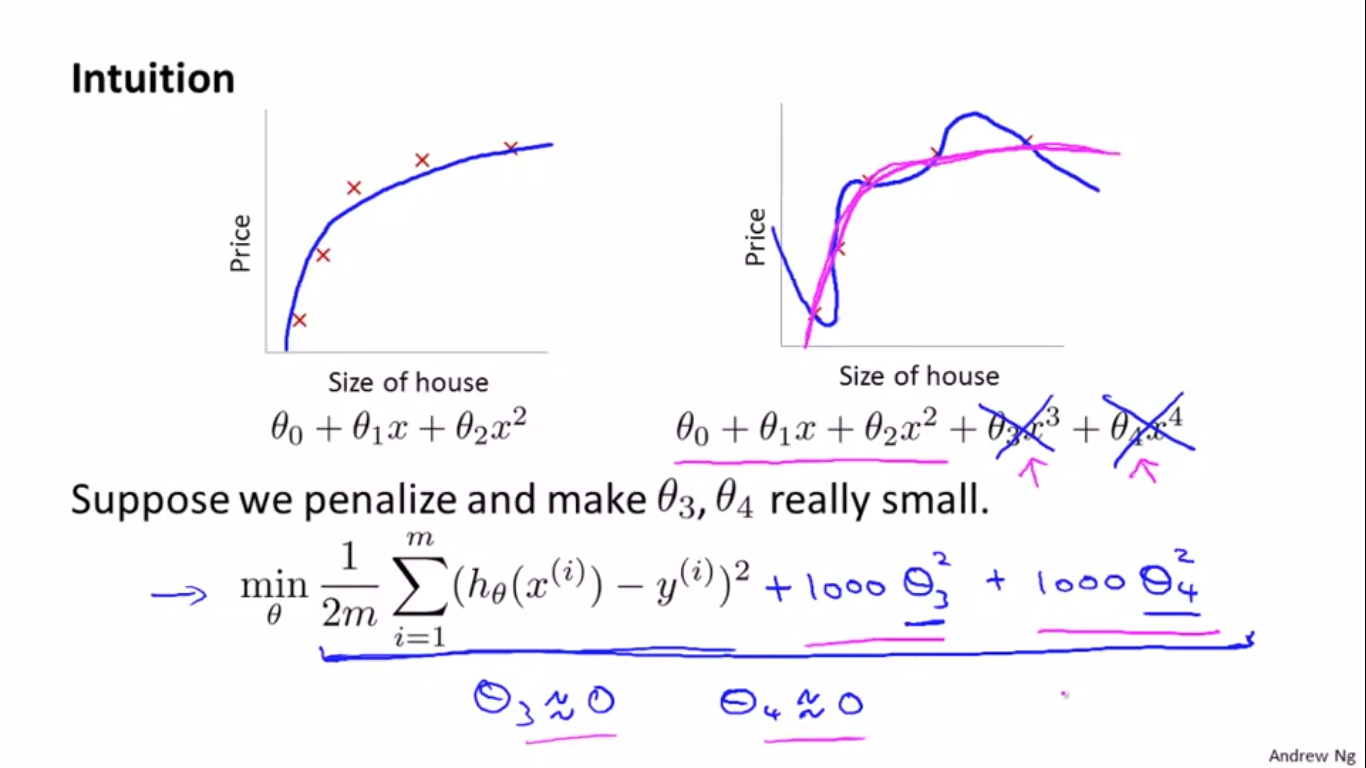
如上图所示,右图中的蓝色曲线过拟合了,因为\(\theta_3 x^3\)和\(\theta_4 x^4\)的贡献太大了,所以就想了一个办法,在最小化损失函数中加上$1000*\theta_3^2 $和\(1000*\theta_4^2\),这样的话损失函数要想最小,那必须降低\(\theta_3\)和\(\theta_4\)的值,这样就降低了\(\theta_3 x^3\)和\(\theta_4 x^4\)的贡献,实现避免过拟合的情况。
但是在实际中,当未知参数数量很多时,我们不知道惩罚哪个参数,所以,就加入一个正则项,缩小所有参数(正则项一般不包括\(\theta_0\),包不包括差别不大,只是一种习惯),如下:
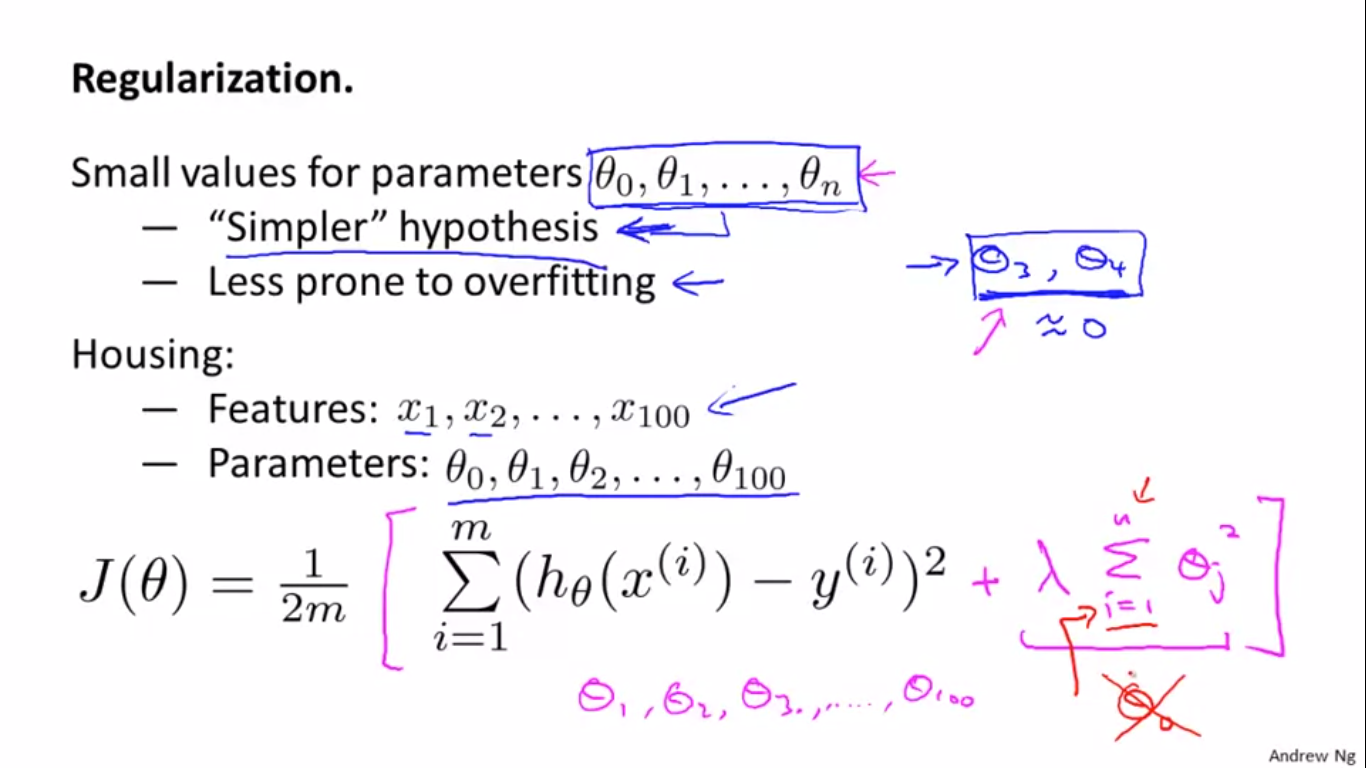
If lambda is chosen to be too large, it may smooth out the function too much and cause underfitting. 如下图:
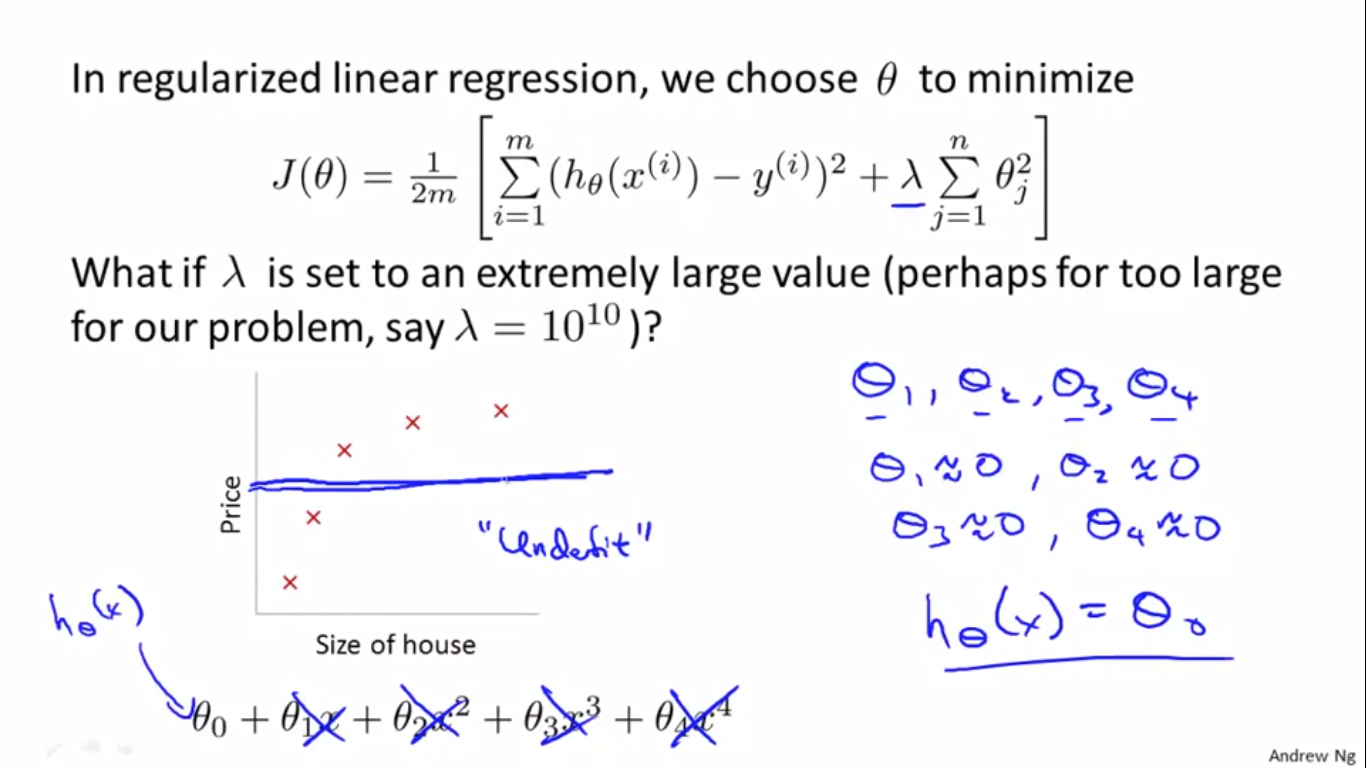
使得参数全都等于零了,所以\(h_\theta(x)=\theta_0\),导致得到的曲线就是一条直线,出现了欠拟合(underfitting)的情况。
3、Regularized Linear Regression
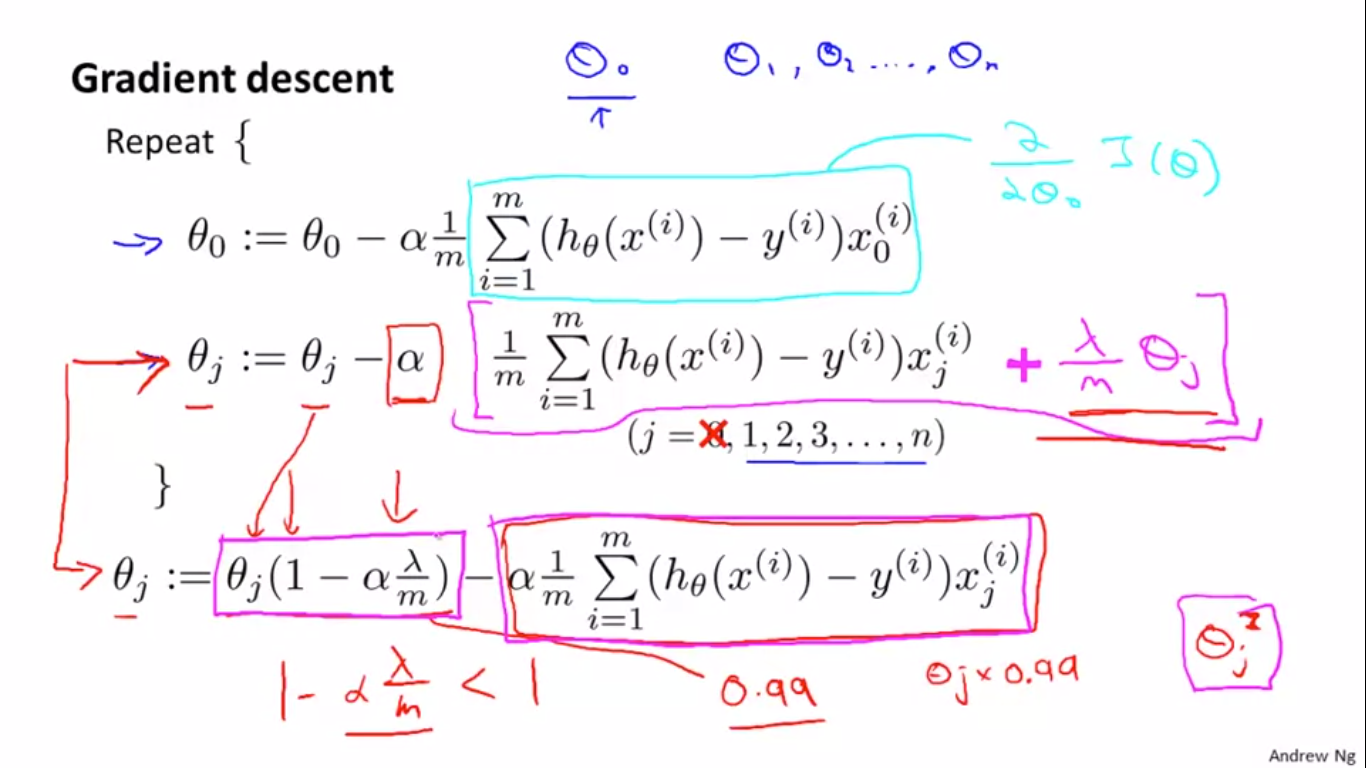
正则化后的梯度下降如上图,化简过后得到最后一排公式,直观上看比较有意思,没有正则化的线性回归的区别在于\(1-\frac{\lambda}{m}\)会是一个小于1的数,所以\(\theta_j\)会不断的去乘以一个小于一的数,后面的那一项倒是和线性回归一样。
- 正则化的正规方程(Normal Equation):
加入正则化后的正规方程求解会变成下图所示:
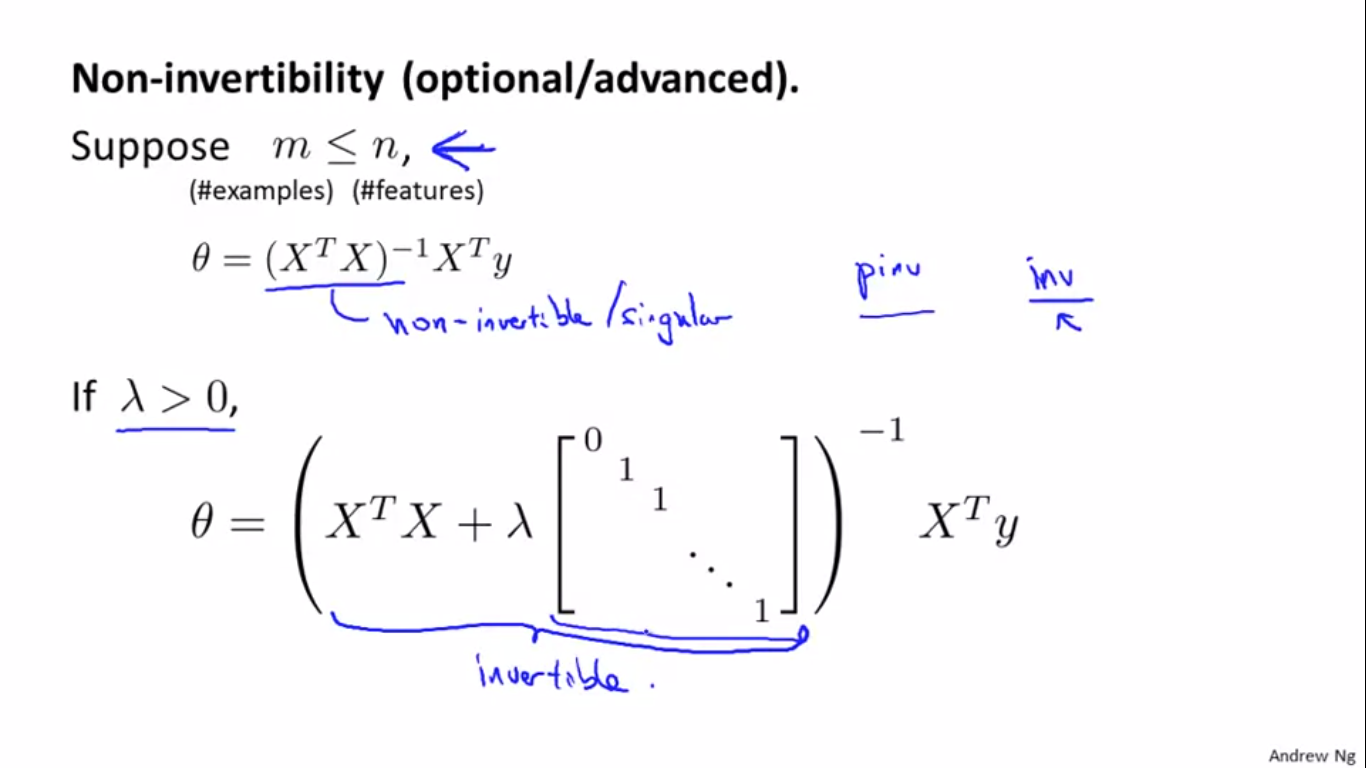
\(X^T X\)可能没有逆,虽然MATLAB/octave的pinv函数可以给出伪逆矩阵,但是求出的theta可能不太对,但是可以证明,\(X^T X+\lambda*[]\)后,\(X^T X+\lambda*[]\)就是可逆的(invertible)。
4、Regularized Logistic Regression
cost function for logistic regression was:
正则化后变成:
所以,梯度下降变为:
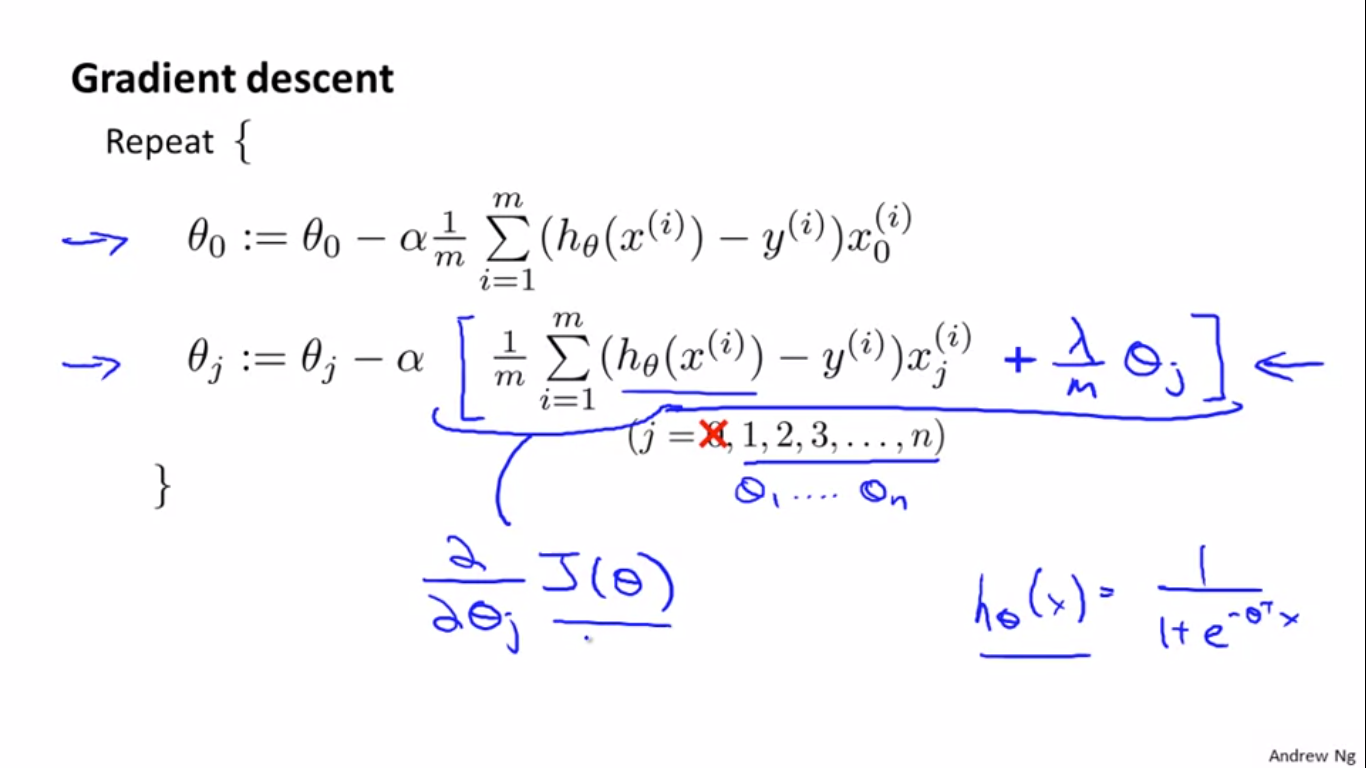
之前讲到了高级优化函数及其损失函数计算方法,在加入正则化后,变成了:
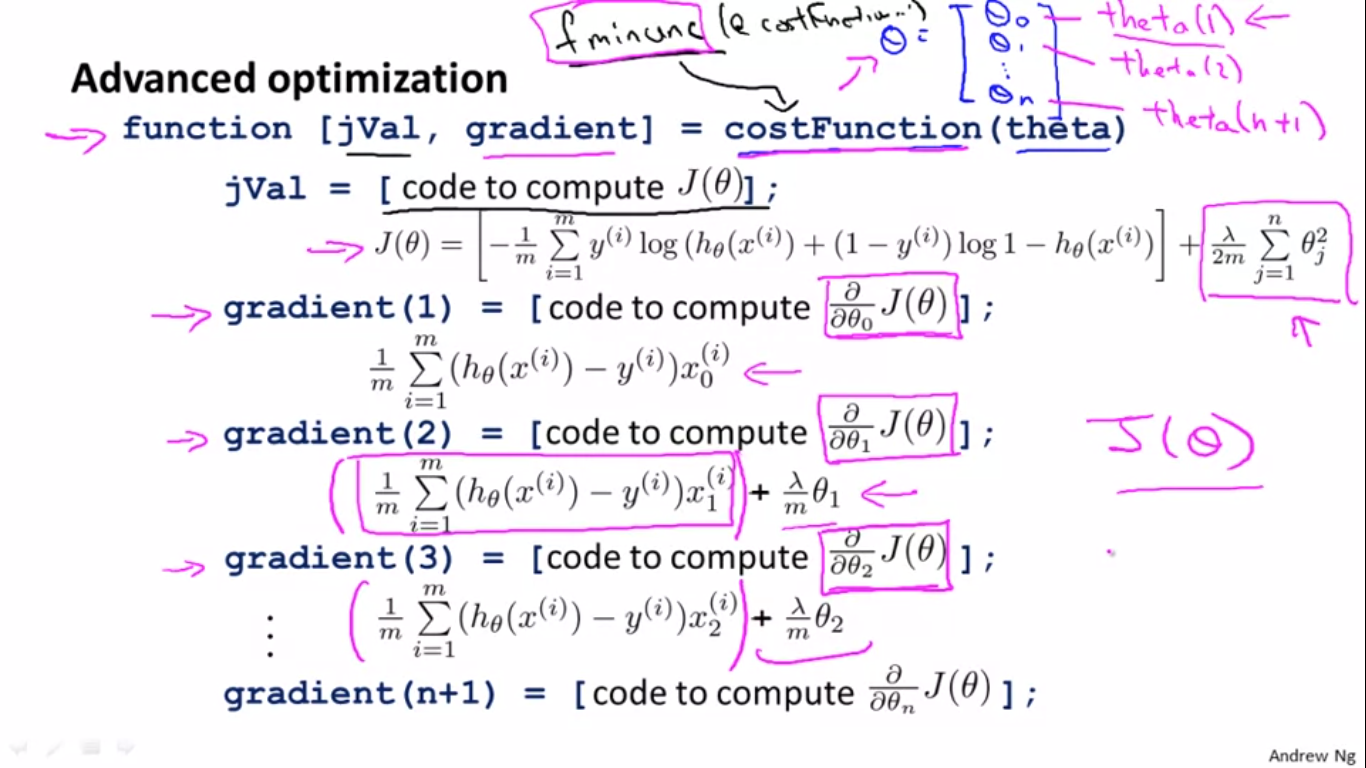
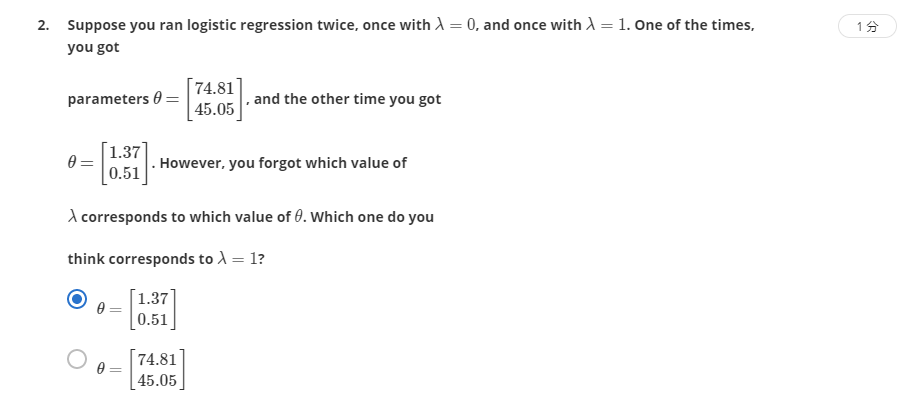
最后的作业也全部完成,效果还行。
posted on 2020-02-26 21:50 zhangqinghu 阅读(335) 评论(0) 编辑 收藏 举报