机器学习算法 --- Pruning (decision trees) & Random Forest Algorithm
一、Table for Content
在之前的文章中我们介绍了Decision Trees Agorithms,然而这个学习算法有一个很大的弊端,就是很容易出现Overfitting,为了解决此问题人们找到了一种方法,就是对Decision Trees 进行 Pruning(剪枝)操作。
为了提高Decision Tree Agorithm的正确率和避免overfitting,人们又尝试了对它进行集成,即使用多棵树决策,然后对于分类问题投票得出最终结果,而对于回归问题则计算平均结果。下面是几条是本篇要讲的主要内容。
- Pruning (decision trees)
- What is Random forest algorithm?
- Why Random Forest algorithm?
- How Random Forest algorithm works?
- Advantages of Random Forest algorithm.
- Random Forest algorithm real life example.
本文主要参考一下几篇文章,有能力的读者可自行前往阅读原文:
1. Wikipedia上的Pruning (decision trees) 和 Random Froest algorithm。
2. Dataaspirant上的《HOW THE RANDOM FOREST ALGORITHM WORKS IN MACHINE LEARNING》
3. medium上的《How Random Forest Algorithm Works in Machine Learning》
同时推荐读者去阅读《The Random Forest Algorithm》,因为这篇文章讲解了在scikit-learn中Random Forest Agorithm常用的重要参数。
二、Pruning(decision trees)
- Pre-pruning that stop growing the tree earlier, before it perfectly classifies the training set.
- Post-pruning that allows the tree to perfectly classify the training set, and then post prune the tree.
Pre-pruning(预剪枝),该方法是在建立决策树的过程中,判断当决策树的node满足一定条件(比如当树的深度达到事先设定的值,或者当该node下的样例个数小于等于某个数)时,不在继续建立子树,所以也叫Early stopping。
Post-pruning(后剪枝),对于此方法,先建立完整的决策树,然后通过一定的算法,将某个非leaf node设为leaf node(即将该node下的子树丢弃)实现pruning。
由于Pre-pruning较为简单就不做具体介绍,所以介绍一下Cost complexity pruning(通过此方法选择某个node设为leaf node,此方法来自wikipedia),当然还有许多其他的方法就不一一介绍了,读者可自行查阅。
三、What is Random Forest algorithm?
关于Random Froest algorithm(随机森林)算法的介绍,很多文章的介绍用例都大同小异,所以在这里也就不另起炉灶了,参考某篇文章的介绍,并做本土特色化翻译如下:
假设有一名学生叫小明,他今年暑假准备去旅游,但他不知道该去哪儿,于是就去问自己的好朋友小刚的意见,小刚则问他一些问题,比如你以前去过哪儿啊,你对要去地方的天气有什么要求啊等等,然后小刚通过这些问题给小明一个建议。决策树就是这样一种思想,通过对样本数据的各个特征值建立一定的规则,让后使用这些规则对新数据做出决策,跟此例非常相似。
但是小明觉得只是一个人的建议,可能比较片面,于是他就问去问了一下他的其他几个朋友,而这几个朋友也问了他一些问题,这些问题有的跟小刚的问题一样,有的不一样,然后他们各自给出了建议,小明拿到这些建议后,综合了一下,有5个朋友建议他去西安,3个朋友建议他去重庆,2个朋友建议他去成都,他最终就决定这个暑假去西安游玩。Random Froest algorithm(随机森林)算法也是如此,很多颗树使用随机样本的随机特征值建立不同的规则,然后各树对于新数据得出不同的结果,最终结果取综合(分类投票,回归取平均)。
Random Froest algorithm(随机森林)的维基百科定义如下:
Random forests or random decision forests are an ensemble learningmethod for classification, regression and other tasks, that operate by constructing a multitude of decision trees at training time and outputting the class that is the mode of the classes (classification) or mean prediction (regression) of the individual trees.
四、Why Random Forest algorithm?
关于这个问题,主要有以下几点理由:
- The same random forest algorithm or the random forest classifier can use for both classification and the regression task.
- Random forest classifier will handle the missing values.
- When we have more trees in the forest, random forest classifier won’t overfit the model.
- Can model the random forest classifier for categorical values also.
五、How Random Forest algorithm works?
建立随机森林的过程如下图:
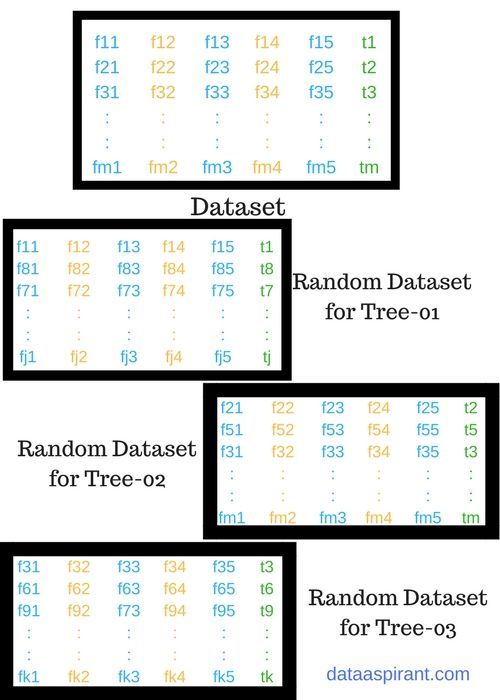
对左图中的Dataset创建包含三棵树的随机森林,过程如下:
step1:在Dataset的众多特征中,随机选取5个特征,在随机选取j个样本数据。
step2: 然后以这些数据构建一颗decesion tree。
step3:重做step1, step2,直到森林中树的数目满足要求。
所以构建Random Forest的通用算法如下:
1. Randomly select “K” features from total “m” features where k << m, then randomly seletct “J” samples from total “n” samples .
2. Among the “K” features of “J” samples, calculate the node “d” using the best split point.
3. Split the node into daughter nodes using the best split.
4. Repeat the 1 to 3 steps until “l” number of nodes has been reached.
5. Build forest by repeating steps a to d for “q” number times to create “q” number of trees.
Random Forest classifier的使用步骤如下:
1. Takes the test features and use the rules of each randomly created decision tree to predict the outcome and stores the predicted outcome(target).
2. Calculate the votes for each predicted target.
3. Consider the high voted predicted target as the final prediction from the random forest algorithm.
六、Advantages of Random Forest algorithm
至于Random Forest algorithm的优点,跟使用它的理由比较相似,主要如下:
1. 对于分类问题,永远不会出现overfitting。
2. 相同的Random Forest algorithm,对于分类问题和回归问题都适用。
3. 它可以识别出数据集中最重要的特征,这也叫feature engineering。
七、Random Forest algorithm real life example
主要应用场景如下:
1. 对于银行业务,它可以被用来分析诚信客户与欺诈客户,对于诚信客户可以给予他们更高的信用额度,而欺诈客户,将面临风险。
2. 对于医药行业,可以使用它来分析制药配方,或者对病人进行病情分析。
3. 对于股市,可以根据以往的数据记录预测将来的趋势,用来做获益或损失的决策。
4. 对于电子商务,那就更不用说了,可以对用户以往的交易记录、浏览记录做定制的广告推送。