Meta Llama3 论文研读
一、 引言概述(Intro & Overview)
Llama3是一系列基于Transformer结构的大型多语言模型,通过优化数据质量、训练规模和模型架构,旨在提升模型在各种语言理解任务中的表现。
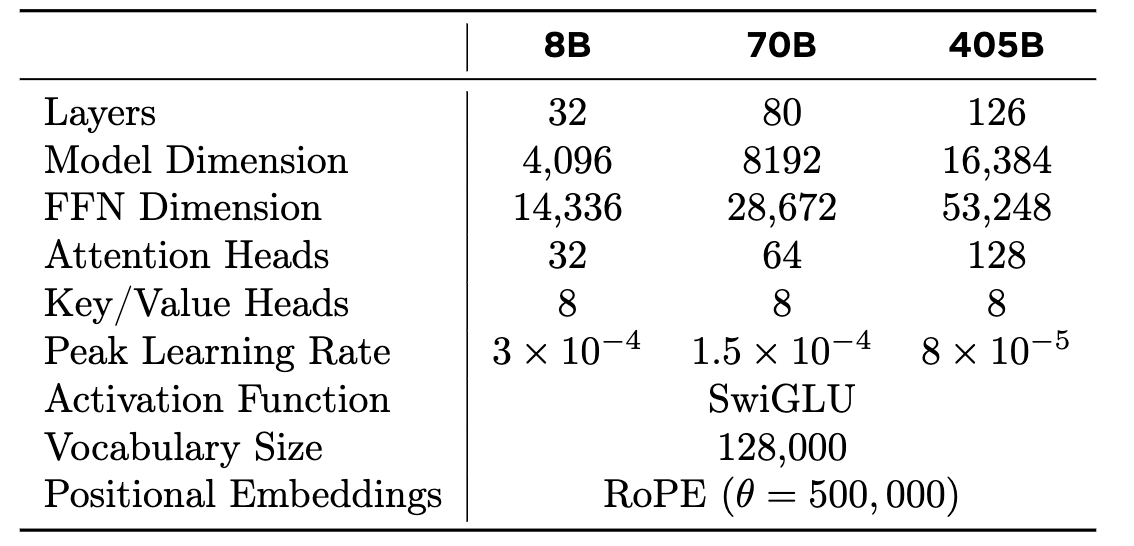
通过引入更优质的数据和更高效的训练方法,Llama3展示了在自然语言处理领域的巨大潜力。其创新点在于其综合了多种优化策略,以解决当前大型语言模型面临的挑战。
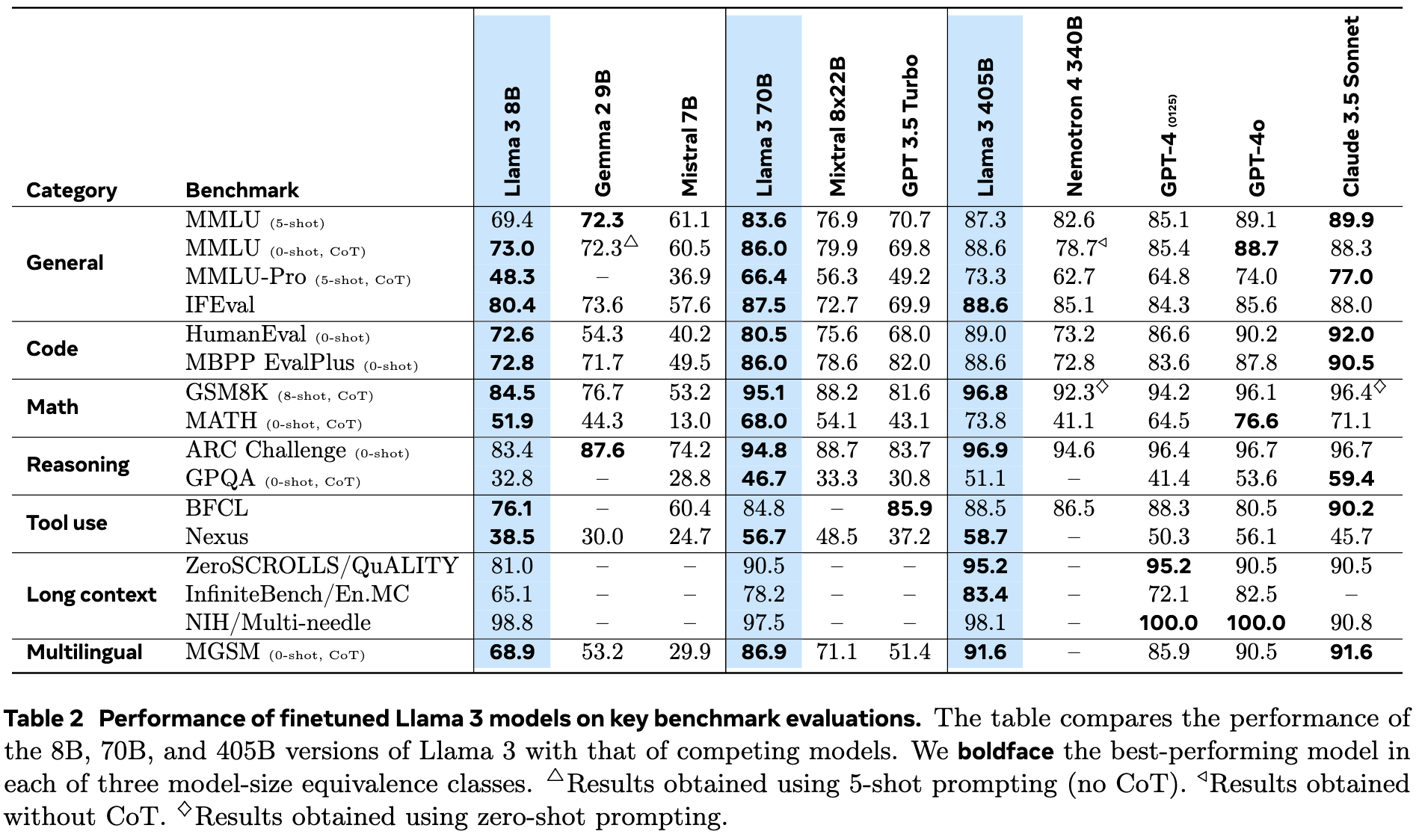
二、数据集(Dataset)
技术报告里详细描述了Llama3数据准备的全过程,包括数据采集、清洗、去重和混合策略的制定。通过开发自定义的HTML解析器和启发式过滤算法,确保了训练数据的高质量和多样性。同时,通过缩放定律实验动态调整数据混合比例,以优化模型性能。
可以看出,数据准备阶段的工作显著提升了Llama3训练数据的整体质量,为后续模型的训练奠定了坚实基础。实验结果表明,高质量的训练数据对提升模型性能具有关键作用。
Meta在训练Llama3系列时,提出了一系列高效的数据预处理和质量控制方法,有效解决了大规模语言模型训练中的数据质量问题,为其他类似项目的数据准备工作提供了可借鉴的经验和方案。
Data mix summary: Our final data mix contains roughly 50% of tokens corresponding to general knowledge, 25% of mathematical and reasoning tokens, 17% code tokens, and 8% multilingual tokens. ”
三、 模型架构与训练(Infra & Training)
此部分详细介绍了Llama3的模型架构和训练方法。Llama3采用了标准的Transformer模型结构(相对于Llama2并没有变),并在此基础上进行了小幅修改以提升训练效率和推理速度。通过引入分组查询注意力机制和4D并行化策略,实现了超大规模模型的高效训练。
实验结果表明,Llama3的模型架构和训练方法显著提升了模型的训练速度和推理性能。分组查询注意力机制有效降低了计算复杂度,而4D并行化策略则充分利用了现有硬件资源,实现了高效的模型训练。这二者解决了大规模语言模型训练中的关键瓶颈问题,为其他大型模型的训练提供了可复制和可扩展的技术方案。
3.1 Scaling-Law 实验
在论文中,关于缩放定律实验(Scaling law experiments)的研究采用了两阶段(two-stage)的方法论来精确预测大规模模型在下游任务中的性能。旨在解决现有缩放定律在预测大规模模型性能时存在的挑战,如只预测下一个词的预测损失而非具体基准任务的性能,以及缩放定律本身可能存在的噪声和不稳定性。
第一阶段:建立训练FLOPs与下游任务负对数似然损失之间的相关性。通过在不同计算规模下预训练多个小型模型,并利用这些模型来预测大型模型在计算FLOPs相同但Tokens不同情况下的性能。
第二阶段:将下游任务的负对数似然损失与任务准确率相关联。通过结合缩放定律模型和Llama2系列模型,利用这些模型在不同计算规模下的性能数据,进一步预测大规模模型(如Llama3 405B)在特定基准任务上的准确率。
实验方法
- 预训练不同规模的模型:研究团队在不同计算规模下预训练了多个小型模型,包括8B、70B等参数量的模型,以获取关于计算资源(FLOPs)与模型性能之间关系的初步数据。
- 评估模型性能:在预训练完成后,对这些模型在多个下游基准任务上进行评估,记录它们在验证集上的负对数似然损失。
- 建立相关模型:利用第一阶段收集的数据,建立计算FLOPs与下游任务负对数似然损失之间的数学模型。
- 预测大规模模型性能:通过外推法,利用上述模型预测大规模模型(如Llama3 405B)在特定计算规模下的负对数似然损失。
- 结合基准任务性能:在第二阶段,将预测的负对数似然损失与下游基准任务的准确率相关联,利用Llama2系列模型在不同计算规模下的实际性能数据,进一步精确预测Llama3 405B在基准任务上的表现。
结论
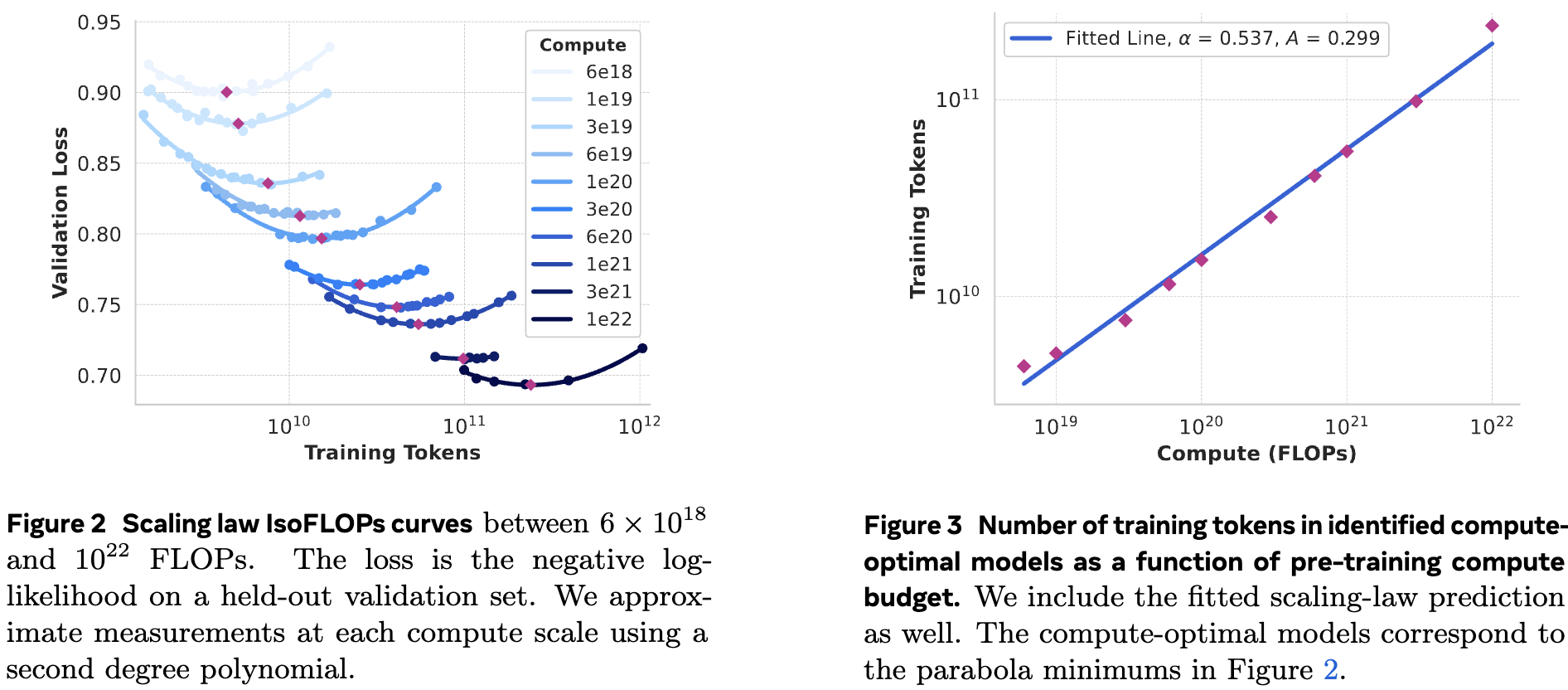
通过两阶段的方法论,研究团队成功预测了Llama3 405B在多个下游基准任务上的性能,并且预测结果与实际测试结果高度吻合。这一结果表明,该两阶段方法论在预测大规模语言模型性能方面具有高度的准确性和可靠性。此外,研究还揭示了缩放定律在不同计算规模下的稳健性,为未来大规模模型的训练和性能预测提供了有力的理论支持和实践指导。
3.2 4D 并行
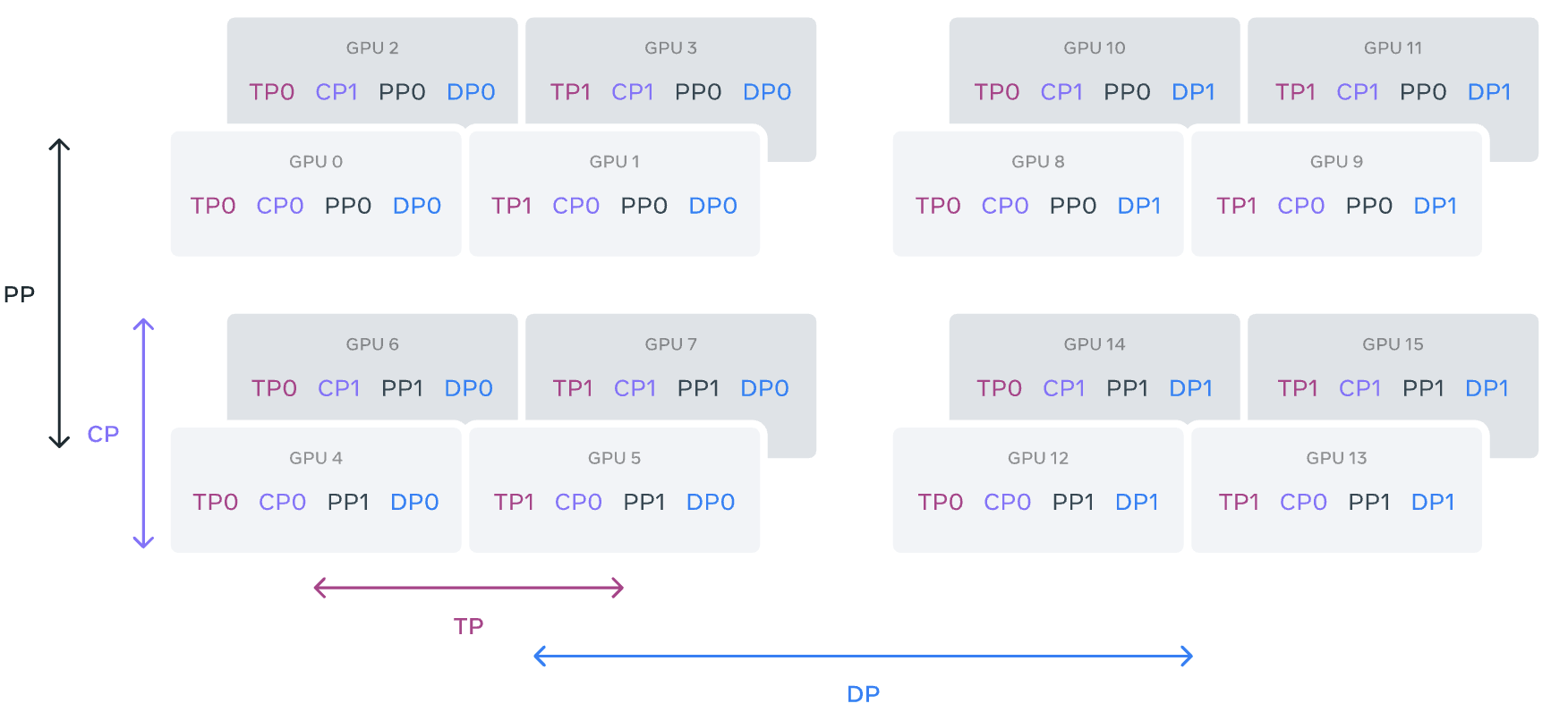
3.3 训练故障率分布
在Llama 3 405B模型为期54天的预训练期间,对意外中断的根本原因进行了分类。约78%的意外中断归因于已确认或疑似硬件问题。
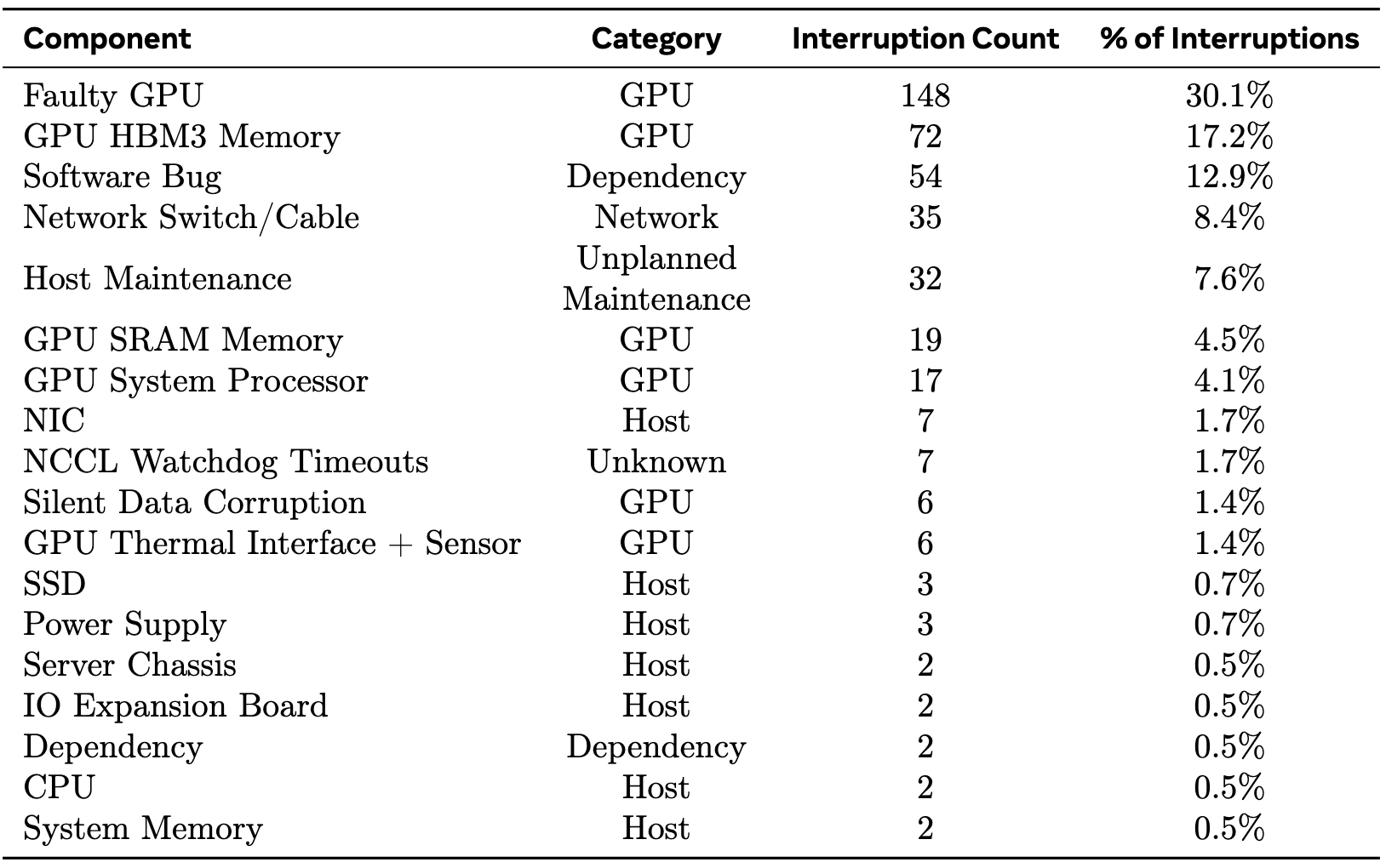
四、后训练与优化(Post-Training)
主要介绍Llama3的后训练与优化过程,包括奖励建模、监督微调(SFT)和直接偏好优化(DPO)。通过多消息聊天协议和复杂对话场景的支持,Llama3的交互能力得到了显著提升。同时,通过数据修剪和质量控制策略确保了高质量的训练数据输入。
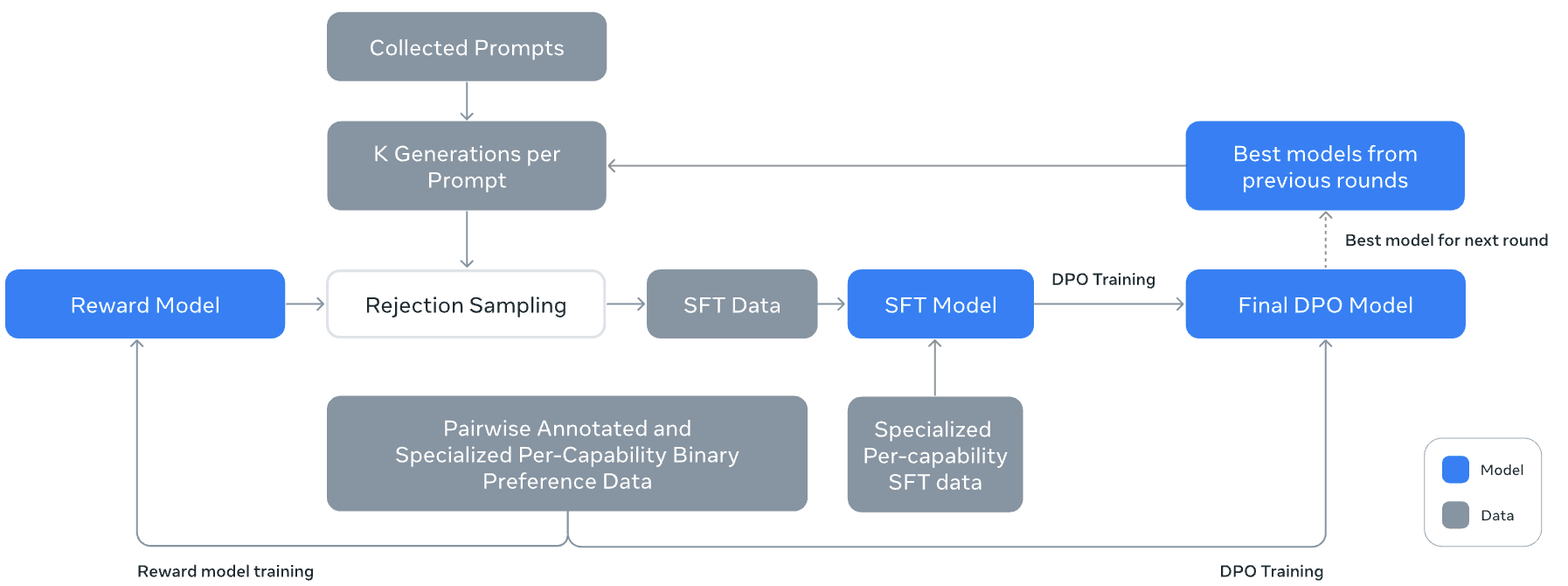
可以看出,后训练与优化阶段的工作显著提高了Llama3的模型性能和用户满意度,经过后训练的Llama3在多个自然语言处理任务上的表现均达到了领先水平。
4.1 SFT
依然使用了非常高质量的数据集进行FT,数据集来源和分布如下:
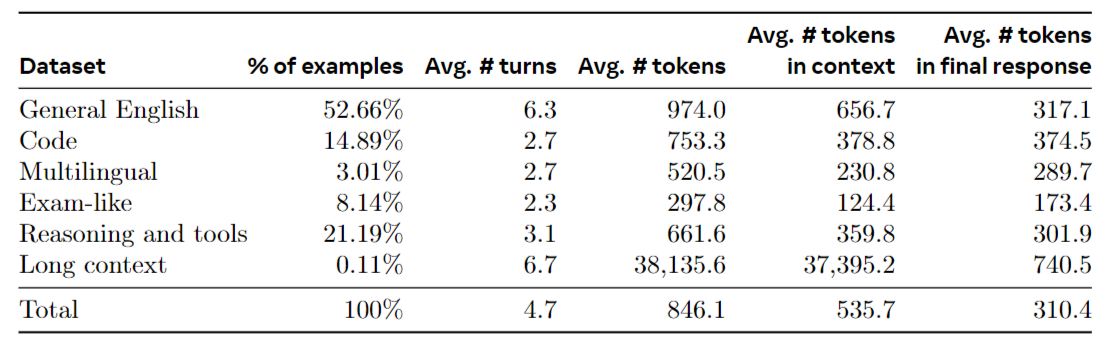
- Prompts from our human annotation collection with rejection-sampled responses
- Synthetic data targeting specific capabilities
- mall amounts of human-curated data
Llama3在后训练与FT阶段的创新点在于,提出了结合奖励建模、监督微调和直接偏好优化的综合后训练方法,有效提升了模型的实用性和泛化能力。
4.2 各维度能力
4.2.1 Code
-
Expert training:We train a code expert which we use to collect high quality human annotations for code
throughout subsequent rounds of post-training. Continued pre-training on domain-specific data has been shown to be effective for improving performance in a specific domain.
-
Synthetic data generation: synthetic data generation offers a complementary approach at a lower cost and higher scale, unconstrained by the expertise level of annotator. In total, we generate over 2.7M synthetic examples which were used during SFT
-
Problem description generation: First, we generate a large collection of programming problem descriptions that span a diverse range of topics, including those in the long tail distribution.
-
Solution generation: Then, we prompt Llama 3 to solve each problem in a given programming language
-
Correctness analysis: we extract the source code from the generated solution and applied a combination of
static and dynamic analysis techniques to test its correctness, including: Static analysis、Unit test generation and execution
-
Error feedback and iterative self-correction
-
Fine-tuning and iterative improvement
-
4.2.2 Multilinguality
-
Expert training: we train a multilingualexpert by branching off the pre-training run and continuing to pre-train on a data mix that consists of 90% multilingual token.
-
Multilingualdatacollection: 2.4% human annotations, 44.2% data from other NLP tasks, 18.8% rejection sampled
data, and 34.6% translated reasoning data.
4.2.3 Math and Reasoning
推理能力本质是一个multi-step 计算得到最终答案的过程,主要面临如下维度的挑战:
- Lack of prompts
- Lack of ground truth chain of thought
- Incorrect intermediate steps
- Teaching models to use external tools
- Discrepancy between training and inference
Llama3 采用的方法论是:
- Addressing the lack of prompts: we create a taxonomy of mathematical skills (Didolkar et al., 2024) and ask humans to provide relevant prompts/questions accordingly.
- Augmenting training data with step-wise reasoning traces: We train outcome and stepwise reward models to filter training data where the intermediate reasoning steps were incorrect.
- Interleaving code and text reasoning: We prompt Llama 3 to solve reasoning problems through a combination of textual reasoning and associated Python code.
- Learning from feedback and mistakes: we utilize incorrect generations and perform error correction by prompting Llama 3 to yield correct generation.
4.2.4 Long Context
During the final pre-training stage, we extend the context length of Llama 3 from 8K tokens to 128K tokens.
五、模型能力评估(Eval)
Llama3在多个基准测试集上的性能进行了全面评估。通过训练多语言专家模型和采用逐步推理策略,评估了Llama3在知识问答、指令跟随、推理任务等多个领域的表现。同时,还对Llama3在长文本处理任务上的能力进行了深入分析。
从评测结果来看,Llama3在多个自然语言处理任务上均表现出了卓越的性能。特别是在多语言处理和数学推理任务上,Llama3的优势尤为明显。此外,Llama3在处理超长文本时也展现出了强大的能力。
六、安全性与可靠性(Security)
主要探讨了Llama3在安全性和可靠性方面的考虑和实践。通过构建内部基准测试集和开发Llama Guard3安全分类器,有效检测和过滤了模型生成内容中的不安全或有害信息。同时,还提出了多种策略来减少模型生成内容中的偏见和误导性信息。
这部分为什么重要?安全性与可靠性阶段的工作可以显著提高Llama3的模型安全性和用户信任度。通过内部基准测试集和安全分类器的应用,有效减少了模型生成内容中的潜在风险。
七、多模态与语音实验
主要介绍了将视觉识别、语音识别和语音生成能力集成到Llama3中的研究尝试。通过组合式方法,实现了对图像、视频和语音内容的深入理解与处理。同时,在多个多模态基准测试集上对Llama3的多模态处理能力进行了评估。
实验结果表明,Llama3在多模态处理任务上展现出了出色的性能。通过集成视觉识别和语音识别能力,Llama3能够更好地理解用户的多样化输入,并生成更准确的响应。