chapter18——PCA实现
1 手写实现PCA
import numpy as np
class PCA():
# 计算协方差矩阵
def calc_cov(self, X):
m = X.shape[0]
# 数据标准化,X的每列减去列均值
X = (X - np.mean(X, axis=0))
return 1 / m * np.matmul(X.T, X)
def pca(self, X, n_components):
# 计算协方差矩阵
cov_matrix = self.calc_cov(X)
# 计算协方差矩阵的特征值和对应特征向量
eigenvalues, eigenvectors = np.linalg.eig(cov_matrix)
# 对特征值排序x
idx = eigenvalues.argsort()[::-1]
# 取最大的前n_component组
eigenvectors = eigenvectors[:, idx] #按特征值大小,从大到小排序
eigenvectors = eigenvectors[:, :n_components] #选取前 n_components 组特征向量
print("eigenvectors.shape = \n",eigenvectors.shape)
print("eigenvectors = \n",eigenvectors) #一个特征向量是一列
# Y=PX转换
return np.matmul(X, eigenvectors)
补充:
2 导入数据集
from sklearn import datasets
import matplotlib.pyplot as plt
# 导入sklearn数据集
iris = datasets.load_iris()
X = iris.data
y = iris.target
print(X.shape)
输出:
(150, 4)
3 手写版PCA可视化
# 将数据降维到3个主成分
X_trans = PCA().pca(X, 3)
# 颜色列表
colors = ['navy', 'turquoise', 'darkorange']
# 绘制不同类别
for c, i, target_name in zip(colors, [0,1,2], iris.target_names):
plt.scatter(X_trans[y == i, 0], X_trans[y == i, 1],color=c, lw=1, label=target_name)
# 添加图例
plt.legend()
plt.show();
输出:
eigenvectors.shape =
(4, 3)
eigenvectors =
[[ 0.36138659 -0.65658877 -0.58202985]
[-0.08452251 -0.73016143 0.59791083]
[ 0.85667061 0.17337266 0.07623608]
[ 0.3582892 0.07548102 0.54583143]]
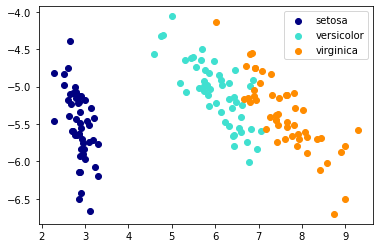
4 sklearn降维模块
# 导入sklearn降维模块
from sklearn import decomposition
# 创建pca模型实例,主成分个数为3个
pca = decomposition.PCA(n_components=3)
# 模型拟合
pca.fit(X)
# 拟合模型并将模型应用于数据X
X_trans = pca.transform(X)
# 颜色列表
colors = ['navy', 'turquoise', 'darkorange']
# 绘制不同类别
for c, i, target_name in zip(colors, [0,1,2], iris.target_names):
plt.scatter(X_trans[y == i, 0], X_trans[y == i, 1],
color=c, lw=2, label=target_name)
# 添加图例
plt.legend()
plt.show();
结果:
因上求缘,果上努力~~~~ 作者:别关注我了,私信我吧,转载请注明原文链接:https://www.cnblogs.com/BlairGrowing/p/15853516.html