chapter6——KNN实现
1 导入相关模块
import numpy as np
from collections import Counter
import matplotlib.pyplot as plt
from sklearn import datasets
from sklearn.utils import shuffle
# 导入sklearn iris数据集
iris = datasets.load_iris()
print("iris.feature_names = ",iris.feature_names)
print("iris.target_names = ",iris.target_names)
# 打乱数据后的数据与标签
X, y = shuffle(iris.data, iris.target, random_state=13)
# 数据转换为float32格式
X = X.astype(np.float32)
# 训练集与测试集的简单划分,训练-测试比例为7:3
offset = int(X.shape[0] * 0.7)
X_train, y_train = X[:offset], y[:offset]
X_test, y_test = X[offset:], y[offset:]
# 将标签转换为竖向量
y_train = y_train.reshape((-1,1))
y_test = y_test.reshape((-1,1))
# 打印训练集和测试集大小
print('X_train=', X_train.shape)
print('X_test=', X_test.shape)
print('y_train=', y_train.shape)
print('y_test=', y_test.shape)
输出:
iris.feature_names = ['sepal length (cm)', 'sepal width (cm)', 'petal length (cm)', 'petal width (cm)']
iris.target_names = ['setosa' 'versicolor' 'virginica']
X_train= (105, 4)
X_test= (45, 4)
y_train= (105, 1)
y_test= (45, 1)
2 定义欧式距离
### 定义欧氏距离
def compute_distances(X, X_train):
'''
输入:
X:测试样本实例矩阵
X_train:训练样本实例矩阵
输出:
dists:欧式距离
'''
# 测试实例样本量
num_test = X.shape[0]
# 训练实例样本量
num_train = X_train.shape[0]
# 基于训练和测试维度的欧氏距离初始化
dists = np.zeros((num_test, num_train))
# 测试样本与训练样本的矩阵点乘
M = np.dot(X, X_train.T)
print("M.shape = ",M.shape)
# 测试样本矩阵平方
te = np.square(X).sum(axis=1)
print("te.shape = ",te.shape)
# 训练样本矩阵平方
tr = np.square(X_train).sum(axis=1)
print("tr.shape = ",tr.shape)
# 计算欧式距离
dists = np.sqrt(-2 * M + tr + np.matrix(te).T)
return dists
补充:
这里计算的欧式距离计算的是:测试样本和训练样本之间的距离。
距离公式:$\sqrt[]{|A-B|^{2}} = \sqrt[]{A^{2}-2AB+B^{2}}$
这里:
-
- M.shape() = (45,105)
欧式距离小例子:
a = np.array([[1,2],[1,1],[1,0] ])
b = np.array([[1,3],[1,2],[1,1] ,[1,0]])
print("a = \n",a)
print("b = \n",b)
M = np.dot(a,b.T)
print("M =\n",M)
tr =np.square(b).sum(axis=1)
print("tr = \n",tr)
te =np.square(a).sum(axis=1)
print("te = \n",te)
print("-2 * M = \n",-2 * M)
print("-2 * M + tr = \n",-2 * M + tr)
print("-2 * M + tr + np.matrix(te).T = \n",-2 * M + tr + np.matrix(te).T)
结果:
a =
[[1 2]
[1 1]
[1 0]]
b =
[[1 3]
[1 2]
[1 1]
[1 0]]
M =
[[7 5 3 1]
[4 3 2 1]
[1 1 1 1]]
tr =
[10 5 2 1]
te =
[5 2 1]
-2 * M =
[[-14 -10 -6 -2]
[ -8 -6 -4 -2]
[ -2 -2 -2 -2]]
-2 * M + tr =
[[-4 -5 -4 -1]
[ 2 -1 -2 -1]
[ 8 3 0 -1]]
-2 * M + tr + np.matrix(te).T =
[[1 0 1 4]
[4 1 0 1]
[9 4 1 0]]
3 距离度量可视化
dists = compute_distances(X_test, X_train)
plt.imshow(dists, interpolation='none')
plt.show();
输出:
M.shape = (45, 105)
te.shape = (45,)
tr.shape = (105,)
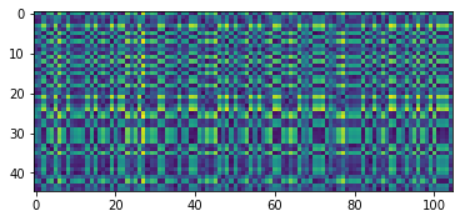
4 定义预测函数
def predict_labels(y_train, dists, k=1):
'''
输入:
y_train:训练集标签
dists:测试集与训练集之间的欧氏距离矩阵
k:k值
输出:
y_pred:测试集预测结果
'''
# 测试样本量
num_test = dists.shape[0]
# 初始化测试集预测结果
y_pred = np.zeros(num_test)
# 遍历
for i in range(num_test):
# 初始化最近邻列表
closest_y = []
# 按欧氏距离矩阵排序后取索引,并用训练集标签按排序后的索引取值,最后拉平列表
labels = y_train[np.argsort(dists[i, :])].flatten()
# 取最近的k个值
closest_y = labels[0:k]
# 对最近的k个值进行计数统计
# 这里注意collections模块中的计数器Counter的用法
c = Counter(closest_y)
# 取计数最多的那一个类别
y_pred[i] = c.most_common(1)[0][0]
return y_pred
补充:
- np.argsort()函数
例子:
#One dimensional array:
x = np.array([3, 1, 2])
print(np.argsort(x))#返回从小到大的数据的原位置索引
"array([1, 2, 0])"
#Two-dimensional array:
x = np.array([[0, 3], [2, 2]])
print(x)
"array([[0, 3],[2, 2]])"
print(np.argsort(x, axis=0))
"array([[0, 1], [1, 0]])"
print(np.argsort(x, axis=1))
"array([[0, 1],[0, 1]])"
#Sorting with keys:
x = np.array([(1, 0), (0, 1)], dtype=[('x', '<i4'), ('y', '<i4')])
print(x)
"array([(1, 0), (0, 1)],dtype=[('x', '<i4'), ('y', '<i4')])"
print(np.argsort(x, order=('x','y')))
"array([1, 0])"
print(np.argsort(x, order=('y','x')) )
"array([0, 1])"
-
Counter.most_common()
words = [
'look', 'into', 'my', 'eyes', 'look', 'into', 'my', 'eyes',
'the', 'eyes', 'the', 'eyes', 'the', 'eyes', 'not', 'around', 'the',
'eyes', "don't", 'look', 'around', 'the', 'eyes', 'look', 'into',
'my', 'eyes', "you're", 'under'
]
from collections import Counter
word_counts = Counter(words)
# 出现频率最高的3个单词
top_three = word_counts.most_common(3)
print(top_three)
top_three = word_counts.most_common(3)[0]
print(top_three)
top_three = word_counts.most_common(3)[0][0]
print(top_three)
结果:
[('eyes', 8), ('the', 5), ('look', 4)]
('eyes', 8)
eyes
5 测试集预测结果
# 测试集预测结果
y_test_pred = predict_labels(y_train, dists, k=1)
y_test_pred = y_test_pred.reshape((-1, 1))
# 找出预测正确的实例
num_correct = np.sum(y_test_pred == y_test)
# 计算准确率
accuracy = float(num_correct) / X_test.shape[0]
print('Got %d/%d correct=>accuracy:%f'% (num_correct, X_test.shape[0], accuracy))
结果:
Got 44/45 correct=>accuracy:0.977778
6 5折交叉验证
### 5折交叉验证
num_folds = 5
# 候选k值
k_choices = [1, 3, 5, 8, 10, 12, 15, 20, 50, 100]
X_train_folds = []
y_train_folds = []
# 训练数据划分
X_train_folds = np.array_split(X_train, num_folds)
# 训练标签划分
y_train_folds = np.array_split(y_train, num_folds)
k_to_accuracies = {}
# 遍历所有候选k值
for k in k_choices:
# 五折遍历
for fold in range(num_folds):
# 对传入的训练集单独划出一个验证集作为测试集
validation_X_test = X_train_folds[fold]
validation_y_test = y_train_folds[fold]
temp_X_train = np.concatenate(X_train_folds[:fold] + X_train_folds[fold + 1:])
temp_y_train = np.concatenate(y_train_folds[:fold] + y_train_folds[fold + 1:])
# 计算距离
temp_dists = compute_distances(validation_X_test, temp_X_train)
temp_y_test_pred = predict_labels(temp_y_train, temp_dists, k=k)
temp_y_test_pred = temp_y_test_pred.reshape((-1, 1))
# 查看分类准确率
num_correct = np.sum(temp_y_test_pred == validation_y_test)
num_test = validation_X_test.shape[0]
accuracy = float(num_correct) / num_test
k_to_accuracies[k] = k_to_accuracies.get(k,[]) + [accuracy]
# 打印不同 k 值不同折数下的分类准确率
for k in sorted(k_to_accuracies):
for accuracy in k_to_accuracies[k]:
print('k = %d, accuracy = %f' % (k, accuracy))
结果:
k = 1, accuracy = 0.904762
k = 1, accuracy = 1.000000
k = 1, accuracy = 0.952381
k = 1, accuracy = 0.857143
k = 1, accuracy = 0.952381
k = 3, accuracy = 0.857143
k = 3, accuracy = 1.000000
k = 3, accuracy = 0.952381
k = 3, accuracy = 0.857143
k = 3, accuracy = 0.952381
k = 5, accuracy = 0.857143
k = 5, accuracy = 1.000000
k = 5, accuracy = 0.952381
k = 5, accuracy = 0.904762
k = 5, accuracy = 0.952381
k = 8, accuracy = 0.904762
k = 8, accuracy = 1.000000
k = 8, accuracy = 0.952381
k = 8, accuracy = 0.904762
k = 8, accuracy = 0.952381
k = 10, accuracy = 0.952381
k = 10, accuracy = 1.000000
k = 10, accuracy = 0.952381
k = 10, accuracy = 0.904762
k = 10, accuracy = 0.952381
k = 12, accuracy = 0.952381
k = 12, accuracy = 1.000000
k = 12, accuracy = 0.952381
k = 12, accuracy = 0.857143
k = 12, accuracy = 0.952381
k = 15, accuracy = 0.952381
k = 15, accuracy = 1.000000
k = 15, accuracy = 0.952381
k = 15, accuracy = 0.857143
k = 15, accuracy = 0.952381
k = 20, accuracy = 0.952381
k = 20, accuracy = 1.000000
k = 20, accuracy = 0.952381
k = 20, accuracy = 0.761905
k = 20, accuracy = 0.952381
k = 50, accuracy = 1.000000
k = 50, accuracy = 1.000000
k = 50, accuracy = 0.904762
k = 50, accuracy = 0.761905
k = 50, accuracy = 0.904762
k = 100, accuracy = 0.285714
k = 100, accuracy = 0.380952
k = 100, accuracy = 0.333333
k = 100, accuracy = 0.238095
k = 100, accuracy = 0.190476
补充
-
np.array_split()
np.split()
均等分割,不均等会报错np.array_split()
不均等分割,不会报错
例子:
a = np.array(np.arange(10))
print(a)
print(np.array_split(a,5))
print(np.array_split(a,6))
for i in range(5):
print("第 %d 份数据"%(i),np.array_split(a,6)[i])
结果:
[0 1 2 3 4 5 6 7 8 9]
[array([0, 1]), array([2, 3]), array([4, 5]), array([6, 7]), array([8, 9])]
[array([0, 1]), array([2, 3]), array([4, 5]), array([6, 7]), array([8]), array([9])]
第 0 份数据 [0 1]
第 1 份数据 [2 3]
第 2 份数据 [4 5]
第 3 份数据 [6 7]
第 4 份数据 [8]
- numpy.concatenate():拼接
例子:
a = np.array([[1, 2], [3, 4]])
b = np.array([[5, 6]])
print(np.concatenate((a,b) , axis = 0))
"""
array([[1, 2],
[3, 4],
[5, 6]])
"""
print(np.concatenate((a, b.T), axis=1) )
"""
array([[1, 2, 5],
[3, 4, 6]])
"""
print( np.concatenate((a, b), axis=None) )
"""
array([1, 2, 3, 4, 5, 6])
"""
7 打印不同 k 值不同折数下的分类准确率
# 打印不同 k 值不同折数下的分类准确率
for k in k_choices:
# 取出第k个k值的分类准确率
accuracies = k_to_accuracies[k]
# 绘制不同k值准确率的散点图
plt.scatter([k] * len(accuracies), accuracies)
# 计算准确率均值并排序
accuracies_mean = np.array([np.mean(v) for k,v in sorted(k_to_accuracies.items())])
# 计算准确率标准差并排序
accuracies_std = np.array([np.std(v) for k,v in sorted(k_to_accuracies.items())])
# 绘制有置信区间的误差棒图
plt.errorbar(k_choices, accuracies_mean, yerr=accuracies_std)
# 绘图标题
plt.title('Cross-validation on k')
# x轴标签
plt.xlabel('k')
# y轴标签
plt.ylabel('Cross-validation accuracy')
plt.show();
结果:
8 sklearn 实现
# 导入KneighborsClassifier模块
from sklearn.neighbors import KNeighborsClassifier
# 创建k近邻实例
neigh = KNeighborsClassifier(n_neighbors=10)
# k近邻模型拟合
neigh.fit(X_train, y_train)
# k近邻模型预测
y_pred = neigh.predict(X_test)
# 预测结果数组重塑
y_pred = y_pred.reshape((-1, 1))
# 统计预测正确的个数
num_correct = np.sum(y_pred == y_test)
# 计算准确率
accuracy = float(num_correct) / X_test.shape[0]
print('Got %d / %d correct => accuracy: %f' % (num_correct, X_test.shape[0], accuracy))
结果:
Got 44 / 45 correct => accuracy: 0.977778
因上求缘,果上努力~~~~ 作者:图神经网络,转载请注明原文链接:https://www.cnblogs.com/BlairGrowing/p/15852786.html