Spark中的广播变量和累加器
一、前言
对于并行处理,Apache Spark使用共享变量。当驱动程序将任务发送到集群上的执行程序时,共享变量的副本将在集群的每个节点上运行,以便可以将其用于执行任务。
累加器(Accumulators)与广播变量(Broadcast Variables)共同作为Spark提供的两大共享变量,主要用于跨集群的数据节点之间的数据共享,突破数据在集群各个executor不能共享问题。
Apache Spark支持两种类型的共享变量
- Broadcast
- Accumulator
二、累加器
累加器(accumulator)是Spark中提供的一种分布式的变量机制,其原理类似于MapReduce,即分布式的改变,然后聚合这些改变。累加器用来把 Executor端变量信息聚合到 Driver 端。在Driver程序中定义的变量,在Executor端的每个 Task 都会得到这个变量的一份新的副本,每个 task 更新这些副本的值后, 传回 Driver 端进行 merge。Spark原生地支持数值型(numeric)的累加器,包含longAccumulator和DoubleAccumulator,运行在集群中的任务可以使用add方法来将数值累加到累加器上。但是这些任务只能做累加操作,不能读取累加器的值,只有任务的控制节点才可以使用value方法来获取累加器的值,除了数值类型的累加器外,程序开发人员可以通过继承AccumulatorV2类来支持新的类型。
Spark提供了三种常见的累加器,分别是LongAccumulator(参数支持Integer、Long)、DoubleAccumulator(参数支持Float、Double)、CollectionAccumulator(参数支持任意类型)累加器方法:
- reset:用来将累加器置为0;
- add:将一个值加到累加器中;
- merge:用来将另外一个同样类型的累加器合并到当前的累加器;
- copy:用来创建当前累加器的一个副本;
- isZero:判断当前累加器是否为0值;
- value:定义当前累加器的值
2.1 案例一:对1、2、3、4求和
import org.apache.spark.SparkContext import org.apache.spark.rdd.RDD import org.apache.spark.sql.{DataFrame, Row, SparkSession} import scala.collection.JavaConversions._ object TestAccumulator { def main(args: Array[String]): Unit = { val ss: SparkSession = SparkSession.builder().appName("test-ccumulator").master("local[2]").getOrCreate() val sc = ss.sparkContext val longAccumulator = sc.longAccumulator("My longAccumulator") // 定义LongAccumulator累加器
sc.makeRDD(Arrays.asList(1,2,3,4)).foreach(v => { longAccumulator.add(v) println(Thread.currentThread().getName+" longAccumulator="+longAccumulator.value) }) println("Driver "+Thread.currentThread().getName+" longAccumulator="+longAccumulator.value) } }
输出:
Executor task launch worker for task 1 longAccumulator=3 Executor task launch worker for task 0 longAccumulator=1 Executor task launch worker for task 0 longAccumulator=3 Executor task launch worker for task 1 longAccumulator=7 Driver main longAccumulator=10
可以看到 task0有两次,分别对1、2累加,两次累加结果分别为1、3,而 task1也有两次,分别对3、4累加,两次累加结果分别为3、7。最后在driver端进行聚合,3(task0)+7(task1)=10
2.2 案例二:给话费低于5元的用户统一发送短信提醒
import org.apache.spark.SparkContext import org.apache.spark.rdd.RDD import org.apache.spark.sql.{DataFrame, Row, SparkSession} import org.apache.spark.streaming.dstream.InputDStream import org.apache.spark.util.CollectionAccumulator import scala.collection.JavaConversions._ object TestAccumulator { def main(args: Array[String]): Unit = { val ss: SparkSession = SparkSession.builder().appName("test-ccumulator").master("local[2]").getOrCreate() val sc = ss.sparkContext
// 定义 collectionAccumulator累加器 val collectionAccumulator: CollectionAccumulator[Student] = sc.collectionAccumulator("My collectionAccumulator")
sc.makeRDD(Arrays.asList(new Student("18123451234","张三",3),new Student("17123451235","李四",2)))
.foreach(v => {
if(v.balance < 5) {
collectionAccumulator.add(v) println(Thread.currentThread().getName+" collectionAccumulator="+v.name)
}
})
for(obj <- collectionAccumulator.value){
println("Driver "+Thread.currentThread().getName+" collectionAccumulator="+obj.name)
println("尊敬的"+obj.phone+"用户,您的话费余额较低,为了不影响使用,请尽快充值")
} } }
class Student(phonex: String, namex: String,balancex: Int) extends Serializable{
var phone: String = phonex
var name: String = namex
var balance: Int = balancex
}
输出:
Executor task launch worker for task 1 collectionAccumulator=李四 Executor task launch worker for task 0 collectionAccumulator=张三 Driver main collectionAccumulator=李四 尊敬的17123451235用户,您的话费余额较低,为了不影响使用,请尽快充值 Driver main collectionAccumulator=张三 尊敬的18123451234用户,您的话费余额较低,为了不影响使用,请尽快充值
案例三:
统计出在一次限次领券活动中,监控领取了多张优惠券的用户,并邮件告警及时处理漏洞,防止意想不到的刷券行为
用SparkStreaming批处理读取hive准实时领券表,把存在的被刷券信息,一次邮件告警出来
import org.apache.spark.SparkContext import org.apache.spark.rdd.RDD import org.apache.spark.sql.{DataFrame, Row, SparkSession} import org.apache.spark.streaming.dstream.InputDStream import org.apache.spark.streaming.{Minutes, StreamingContext} import org.slf4j.{Logger, LoggerFactory} import scala.collection.mutable import org.apache.spark.util.CollectionAccumulator import scala.collection.JavaConversions._ import org.apache.spark.util.AccumulatorV2 import java.util.ArrayList import java.util.Collections /** * Desc: * 刷券监控 */ object TestAccumulator { private val logger: Logger = LoggerFactory.getLogger(TestAccumulator.getClass) private lazy val ss: SparkSession = SparkSession.builder() .appName("test-ccumulator") //.master("local[2]") .config("spark.sql.adaptive.enabled", true) .config("spark.sql.autoBroadcastJoinThreshold", "-1") .config("spark.sql.crossJoin.enabled", true) .config("spark.task.maxFailures", 5) .config("spark.files.ignoreCorruptFiles", true) .config("spark.files.ignoreMissingFiles", true) .config("spark.sql.storeAssignmentPolicy", "LEGACY") .config("dfs.client.block.write.replace-datanode-on-failure.policy", "NEVER") .config("mapred.output.compress", true) .config("hive.exec.compress.output", true) .config("mapreduce.map.output.compress.codec", "org.apache.hadoop.io.compress.SnappyCodec") .config("mapreduce.output.fileoutputformat.compress", true) .config("mapreduce.output.fileoutputformat.compress.codec", "org.apache.hadoop.io.compress.SnappyCodec") .enableHiveSupport() .getOrCreate() def main(args: Array[String]): Unit = { val sc = ss.sparkContext val ssc: StreamingContext = new StreamingContext(sc, Minutes(5)) //每5分钟跑一批 //定义累加器 val myListAccumulator: MyListAccumulator[CpsActivity] = new MyListAccumulator //注册累加器 sc.register(myListAccumulator, "MyListAccumulator") val queue: mutable.Queue[RDD[String]] = new mutable.Queue[RDD[String]]() val queueDS: InputDStream[String] = ssc.queueStream(queue) queueDS.foreachRDD(rdd => { //统计出在一次限次领券活动中,监控领取了多张优惠券的用户,并邮件告警及时处理漏洞 val sql = "select cps_id,batch_id,activity_id,activity_name,user_id,count(*) from cps_coupon_record group by cps_id,batch_id,activity_id,activity_name,user_id having count(*) > 1" print(sql) val dataFrame: DataFrame = ss.sql(sql); dataFrame.foreachPartition { line => while (line.hasNext) { val row = line.next(); val cpsId = row.getAs("cps_id"); val batchId = row.getAs("batch_id"); val activityId = row.getAs("activity_id"); val activityName = row.getAs("activity_name"); val cpsActivity = new CpsActivity(cpsId, batchId, activityId, activityName) myListAccumulator.add(cpsActivity); } } //每次执行完成清空累加器 myListAccumulator.reset(); ss.sqlContext.clearCache() }) //发送邮件或执行其它操作,这里就省略了发邮件代码 val msg = "您好,巡检系统监控到以下优惠券活动,存在同一个用户领取了多张优惠券情况,可能存在刷券行为,请及时核对并处理\n" var sb = new StringBuilder() sb.append(msg) sb.append(String.format("%s,%s,%s,%s", "活动名称","活动ID","批次号","券ID")).append("\n") for ( obj <- myListAccumulator.value) { val text = String.format("%s,%s,%s,%s\n", obj.activityName,obj.activityId,obj.batchId,obj.cpsId); sb.append(text) } println(sb.toString()) ssc.start() ssc.awaitTermination() } } /** * 券信息 */ class CpsActivity(cpsIdx: String, batchIdx: String,activityIdx: String, activityNamex: String) extends Serializable{ var cpsId: String = cpsIdx var batchId: String = batchIdx var activityId: String = activityIdx var activityName: String = activityNamex } /** * 自定义累加器 */ class MyListAccumulator[T] extends AccumulatorV2[T, java.util.List[T]] { private val _list: java.util.List[T] = Collections.synchronizedList(new ArrayList[T]()) override def isZero: Boolean = _list.isEmpty override def copyAndReset(): MyListAccumulator[T] = new MyListAccumulator override def copy(): MyListAccumulator[T] = { val newAcc = new MyListAccumulator[T] _list.synchronized { newAcc._list.addAll(_list) } newAcc } override def reset(): Unit = _list.clear() override def add(v: T): Unit = _list.add(v) override def merge(other: AccumulatorV2[T, java.util.List[T]]): Unit = other match { case o: MyListAccumulator[T] => _list.addAll(o.value) case _ => throw new UnsupportedOperationException( s"Cannot merge ${this.getClass.getName} with ${other.getClass.getName}") } override def value: java.util.List[T] = _list.synchronized { java.util.Collections.unmodifiableList(new ArrayList[T](_list)) } }
输出:
您好,巡检系统监控到以下优惠券活动,存在同一个用户领取了多张优惠券情况,可能存在刷券行为,请及时核对并处理 活动名称,活动ID,批次号,券ID 新人专享券,12345,8888,1234445 新人专享券,12345,8888,1234446
原文链接:https://blog.csdn.net/lzxlfly/article/details/123604181
三、广播变量
如果我们要在分布式计算里面分发大对象,例如:字典,集合,黑白名单等,这个都会有Driver端进行分发。一般来说,如果这个变量不是广播变量,那么每个task都会持有该变量的一个副本,这在task数目十分多的情况下,Driver的带宽会成为系统的瓶颈,而且会大量消耗task服务器上的资源。如果将这个资源声明为广播变量,那么此时每个Executor节点只会包含一份该变量的副本,那么此Executor上启动的task都可以访问到此变量,故节省了网络传输的成本和服务器的资源。
下面的两幅图展示了不使用和使用广播变量的区别:
不使用广播变量:下面的两幅图展示了不使用和使用广播变量的区别:
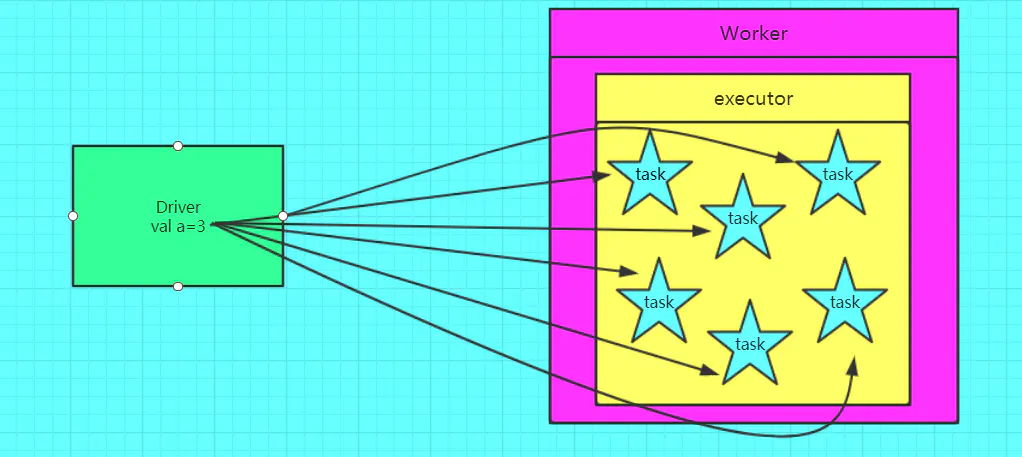
使用广播变量:
广播变量的特点:
- 广播变量:分布式只读变量。
- 如果Executor端需要访问Driver端的某个变量,spark会向Executor端每个task都发送一个此变量的副本。如果此变量很大,就会占用大量的Executor节点的内存。
- 利用广播变量,spark只会给一个Executor节点发送一个变量。
object Broadcast { def main(args: Array[String]): Unit = { val sparkConf= new SparkConf().setMaster("local[*]").setAppName("broadcast") val sc = new SparkContext(sparkConf) val rdd = sc.makeRDD(List( ("a", 1), ("b", 2), ("c", 3),("c", 3) ),4) // val map=mutable.Map("b"->3,"c"->5,"d"->7) val value1 = sc.broadcast(map) val broadRDD = rdd.map { case (key, value) => { if (value1.value.contains(key)) { (key, value + 1) }else{ (key,1000) } } } broadRDD .collect() sc.stop() } }