Hive分布式数据仓库(大数据)
数据仓库
- 数据仓库是一个面向主题的(Subject Oriented),集成的(Integrated),相对稳定的(Non-Volatile)以及反映历史变化的(Time Variant)数据集合,用于支持管理决策。
- 面向主题:数据仓库会围绕一些主题来组织和构建。
- 集成:指构建数据仓库通常会将多个异构的数据源。
- 相对稳定:数据仓库大多会分开存储数据,数据仓库不需要进行事务处理,数据恢复和并发控制等。
- 反映历史变化:数据仓库是从历史的角度提供信息的。
数据仓库和数据库的区别
- 数据库的操作
- 联机事务处理(OLTP)
- OLTP面向一般的客户,关注企业的当前数据,主要用于存储和管理日常运营的数据。
- OLTP的访问模式由短的原子事务组成,同时需要考虑事务管理,并发控制和故障恢复。
- 数据仓库的操作
- 联机分析处理(OLAP)
- OLAP面向的是管理决策人员,提供数据分析的功能。
- 数据仓库与OLAP的关系是互补的。
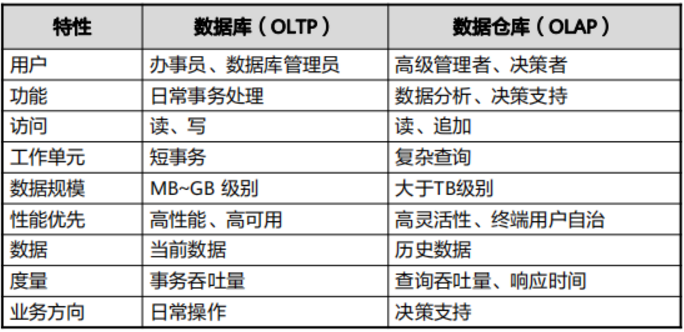
数据仓库的系统结构
- 数据源
- 数据存储和管理
- 数据服务
- 数据应用
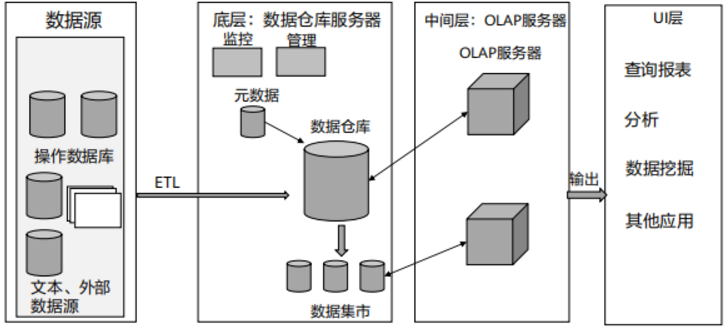
Hive概述和体系结构
Hive简介
- Hive是基于Hadoop的数据仓库软件,可以查询和管理pb级别的分布式数据。
- Hive特性:
- 灵活方便的ETL(extract/transform/load)
- 支持Tez,spark等多种计算引擎
- 可直接访问HDFS文件以及HBase
- 易用易编程
Hive应用场景
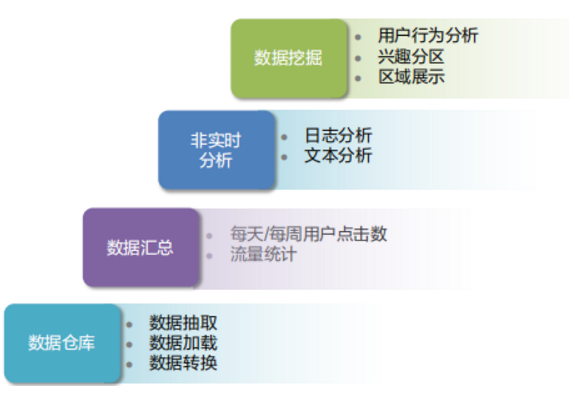
Hive体系结构
- Hive命令行界面(Command-Line Lnterface,CLI)
- THrift提供Hive远程访问,支持JDBC和ODBC
- 元数据服务
- Hive默认的执行引擎是MapReduce
- Hive Web界面
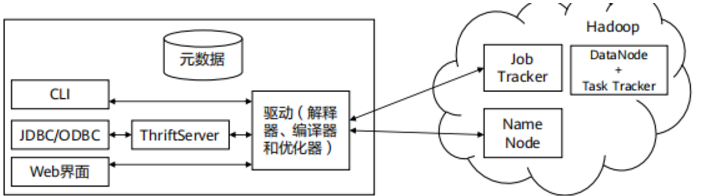
华为Hive架构
- 引入了WebHCat组件。
- WebHCat对外提供REST接口,使用户可以通过超文本传输安全协议(Hyper Text Transfer Protocol Secure, HTTPS)使用元数据访问,数据定义语言(Data Defination Language,DDL)查询等服务。
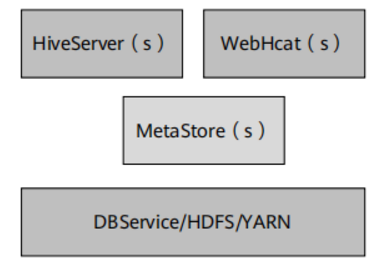
Hive与传统数据仓库比较(1)
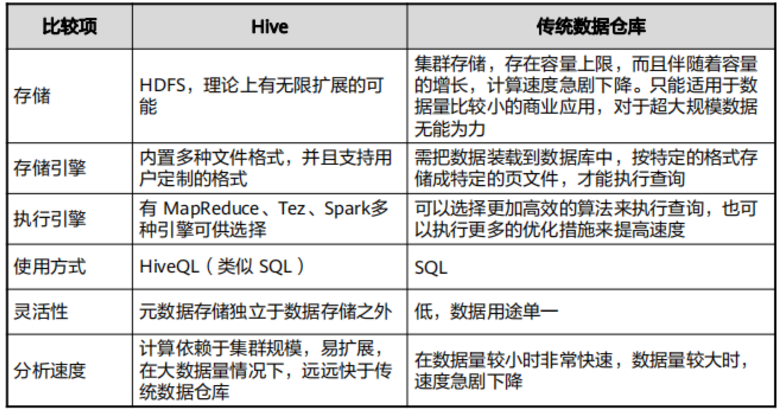
Hive与传统数据仓库比较(2)
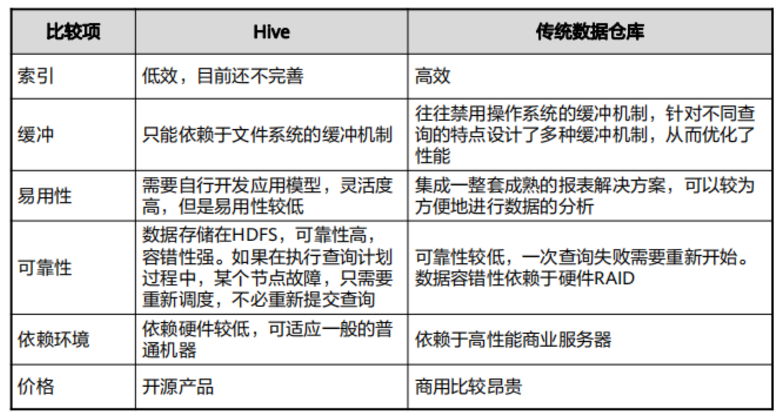
Hive优点
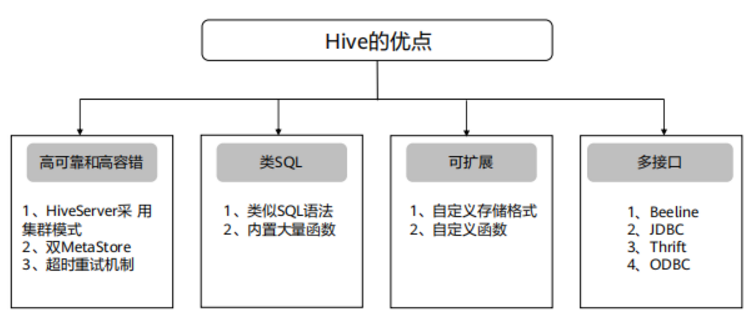
Hive缺点
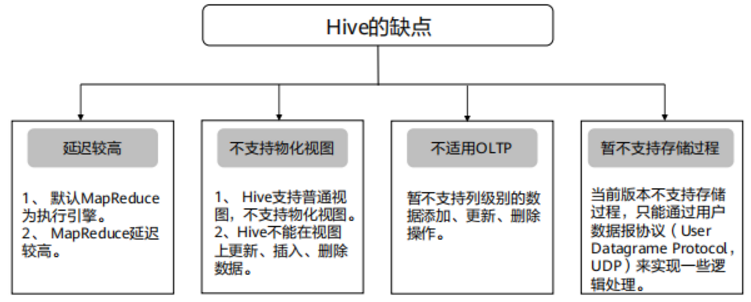
Hive数据存储模型
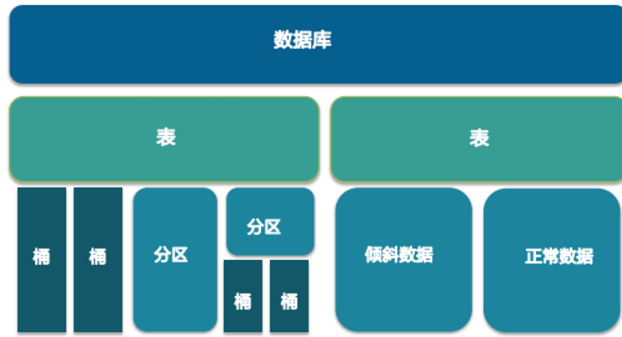
Hive分区和分桶
- 分区:数据表可以按照某给字段的值划分分区。
- 每个分区是一个目录。
- 分区数量不固定。
- 分区下可再有分区或者桶。
- 桶:数据可以根据桶的方式将不同数据放入不同的桶中。
- 每个桶是一个文件。
- 建表时指定桶个数,桶内可排序。
- 数据按照某个字段的值Hash后放入某个桶中。
Hive基本操作
Hive数据基本操作(1)
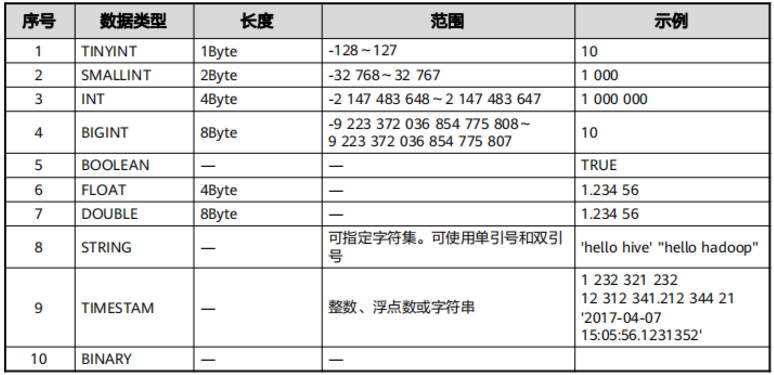
Hive数据基本操作(2)
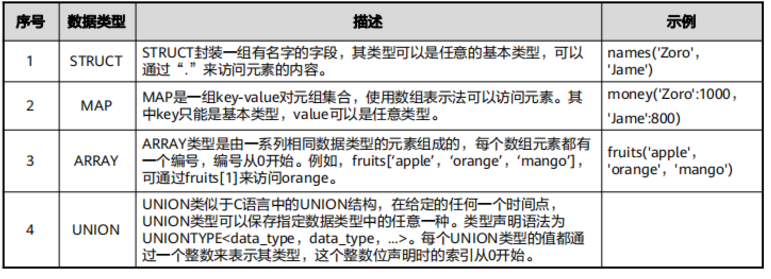
Hive SQL介绍
- DDL-数据定义的语言
- 建表,修改修,删表,分区,数据类型
- DML-数据管理语言
- 数据导入,数据导出
- DQL-数据查询语言
- 简单查询
- 父子查询Group by,Order by,Join等
DDL操作(1)
- 创建数据库
- create database|schema [if not exists] <database name>
- 删除数据库
- drop (database|schema)[if exists] database_name [restrict|cascade]
DDL操作(2)
- 创建表
create [temporary] [external] table [if not exists] [db_name.] table_name
[(col_name data_type [comment col_comment] ,...)]
[comment table_comment] [row format row_format]
[stored as file_format]
like table_name1
[location hdfs_path]
- 描述表
describe [tablename]
DDL操作(3)
- 浏览表
show tables
- 修改表
alter table [firest_table] rename to [second_table]
- 增加删除列
alter table table_name add|replace columns (col_name data_type [comment col_comment])
DML操作
- 向表里加载数据
load data [local] inpath 'filepath'
[overwrite] info table tablename
[paetition (partcol1=val1,partcol2=val2)]
- 导出数据到
export table tablename to '/department'
DQL操作(1)
- select基本语句
select [all | distinct] select_expr,select_expr,....
from table_reference
[where where_condition]
[group by col_list[having condition]]
[cluster by col_list| [distribute by col_list]] [sort by| order by col_list]
[limit number]
DQL操作(2)
- JOIN基本语句
table_reference join table_factor [join_condition]
| table_reference {left|right|full} [outer] join table_reference join_condition
| table_reference left semi join table_reference join_condition
| table_reference cross join table_reference [join_condition] (as of Hive 0.10)
DQL操作(3)
- Map端JOIN
- 大的表通过mapper的时候将小标映射到内存中,这样join操作可以被转换伟只有一个任务,无法启动reduce
- 提高join效率
- hive>select /*+mapjoin(samlltable)*/ .key,value
>from smalltable
>join bigtable
>on smalltable.key = bigtable.key
Hive支持的函数
- Hive内置函数
- 数学函数:如round(),floor(),abs(),rand()等
- 日期函数:如to_date(),mouth(),day()等
- 字符串函数:如trim(),length(),substr()等
- UDF (user - defined funcation)
Hive数据压缩与文件存储格式
- Hive数据压缩
- gzip
- bzip2
- lzo
- snappy
- Hive文件存储格式
- textfile
- sequencefile
- rcfile
- orcfile
- parquet
希望和悲伤,都是一缕光。总有一天,我们会再相遇。