The Anatomy of a Large-Scale Hypertextual Web Search Engine
Abstract
In this paper, we present Google, a prototype of a large-scale search engine which makes heavy use of the structure present in hypertext. Google is designed to crawl and index the Web efficiently and produce much more satisfying search results than existing systems. The prototype with a full text and hyperlink database of at least 24 million pages is available at http://google.stanford.edu/
To engineer a search engine is a challenging task. Search engines index tens to hundreds of millions of web pages involving a comparable number of distinct terms. They answer tens of millions of queries every day. Despite the importance of large-scale search engines on the web, very little academic research has been done on them. Furthermore, due to rapid advance in technology and web proliferation, creating a web search engine today is very different from three years ago. This paper provides an in-depth description of our large-scale web search engine -- the first such detailed public description we know of to date.
Apart from the problems of scaling traditional search techniques to data of this magnitude, there are new technical challenges involved with using the additional information present in hypertext to produce better search results. This paper addresses this question of how to build a practical large-scale system which can exploit the additional information present in hypertext. Also we look at the problem of how to effectively deal with uncontrolled hypertext collections where anyone can publish anything they want.Keywords: World Wide Web, Search Engines, Information Retrieval, PageRank, Google
1. Introduction
(Note: There are two versions of this paper -- a longer full version and a shorter printed version. The full version is available on the web and the conference CD-ROM.)The web creates new challenges for information retrieval. The amount of information on the web is growing rapidly, as well as the number of new users inexperienced in the art of web research. People are likely to surf the web using its link graph, often starting with high quality human maintained indices such as Yahoo! or with search engines. Human maintained lists cover popular topics effectively but are subjective, expensive to build and maintain, slow to improve, and cannot cover all esoteric topics. Automated search engines that rely on keyword matching usually return too many low quality matches. To make matters worse, some advertisers attempt to gain people's attention by taking measures meant to mislead automated search engines. We have built a large-scale search engine which addresses many of the problems of existing systems. It makes especially heavy use of the additional structure present in hypertext to provide much higher quality search results. We chose our system name, Google, because it is a common spelling of googol, or 10100 and fits well with our goal of building very large-scale search engines.
1.1 Web Search Engines -- Scaling Up: 1994 - 2000
Search engine technology has had to scale dramatically to keep up with the growth of the web. In 1994, one of the first web search engines, the World Wide Web Worm (WWWW) [McBryan 94] had an index of 110,000 web pages and web accessible documents. As of November, 1997, the top search engines claim to index from 2 million (WebCrawler) to 100 million web documents (from Search Engine Watch). It is foreseeable that by the year 2000, a comprehensive index of the Web will contain over a billion documents. At the same time, the number of queries search engines handle has grown incredibly too. In March and April 1994, the World Wide Web Worm received an average of about 1500 queries per day. In November 1997, Altavista claimed it handled roughly 20 million queries per day. With the increasing number of users on the web, and automated systems which query search engines, it is likely that top search engines will handle hundreds of millions of queries per day by the year 2000. The goal of our system is to address many of the problems, both in quality and scalability, introduced by scaling search engine technology to such extraordinary numbers.1.2. Google: Scaling with the Web
Creating a search engine which scales even to today's web presents many challenges. Fast crawling technology is needed to gather the web documents and keep them up to date. Storage space must be used efficiently to store indices and, optionally, the documents themselves. The indexing system must process hundreds of gigabytes of data efficiently. Queries must be handled quickly, at a rate of hundreds to thousands per second.These tasks are becoming increasingly difficult as the Web grows. However, hardware performance and cost have improved dramatically to partially offset the difficulty. There are, however, several notable exceptions to this progress such as disk seek time and operating system robustness. In designing Google, we have considered both the rate of growth of the Web and technological changes. Google is designed to scale well to extremely large data sets. It makes efficient use of storage space to store the index. Its data structures are optimized for fast and efficient access (see section 4.2). Further, we expect that the cost to index and store text or HTML will eventually decline relative to the amount that will be available (see Appendix B). This will result in favorable scaling properties for centralized systems like Google.
1.3 Design Goals
1.3.1 Improved Search Quality
Our main goal is to improve the quality of web search engines. In 1994, some people believed that a complete search index would make it possible to find anything easily. According to Best of the Web 1994 -- Navigators, "The best navigation service should make it easy to find almost anything on the Web (once all the data is entered)." However, the Web of 1997 is quite different. Anyone who has used a search engine recently, can readily testify that the completeness of the index is not the only factor in the quality of search results. "Junk results" often wash out any results that a user is interested in. In fact, as of November 1997, only one of the top four commercial search engines finds itself (returns its own search page in response to its name in the top ten results). One of the main causes of this problem is that the number of documents in the indices has been increasing by many orders of magnitude, but the user's ability to look at documents has not. People are still only willing to look at the first few tens of results. Because of this, as the collection size grows, we need tools that have very high precision (number of relevant documents returned, say in the top tens of results). Indeed, we want our notion of "relevant" to only include the very best documents since there may be tens of thousands of slightly relevant documents. This very high precision is important even at the expense of recall (the total number of relevant documents the system is able to return). There is quite a bit of recent optimism that the use of more hypertextual information can help improve search and other applications [Marchiori 97] [Spertus 97] [Weiss 96] [Kleinberg 98]. In particular, link structure [Page 98] and link text provide a lot of information for making relevance judgments and quality filtering. Google makes use of both link structure and anchor text (see Sections 2.1 and 2.2).1.3.2 Academic Search Engine Research
Aside from tremendous growth, the Web has also become increasingly commercial over time. In 1993, 1.5% of web servers were on .com domains. This number grew to over 60% in 1997. At the same time, search engines have migrated from the academic domain to the commercial. Up until now most search engine development has gone on at companies with little publication of technical details. This causes search engine technology to remain largely a black art and to be advertising oriented (see Appendix A). With Google, we have a strong goal to push more development and understanding into the academic realm.Another important design goal was to build systems that reasonable numbers of people can actually use. Usage was important to us because we think some of the most interesting research will involve leveraging the vast amount of usage data that is available from modern web systems. For example, there are many tens of millions of searches performed every day. However, it is very difficult to get this data, mainly because it is considered commercially valuable.
Our final design goal was to build an architecture that can support novel research activities on large-scale web data. To support novel research uses, Google stores all of the actual documents it crawls in compressed form. One of our main goals in designing Google was to set up an environment where other researchers can come in quickly, process large chunks of the web, and produce interesting results that would have been very difficult to produce otherwise. In the short time the system has been up, there have already been several papers using databases generated by Google, and many others are underway. Another goal we have is to set up a Spacelab-like environment where researchers or even students can propose and do interesting experiments on our large-scale web data.
2. System Features
The Google search engine has two important features that help it produce high precision results. First, it makes use of the link structure of the Web to calculate a quality ranking for each web page. This ranking is called PageRank and is described in detail in [Page 98]. Second, Google utilizes link to improve search results.
2.1 PageRank: Bringing Order to the Web
The citation (link) graph of the web is an important resource that has largely gone unused in existing web search engines. We have created maps containing as many as 518 million of these hyperlinks, a significant sample of the total. These maps allow rapid calculation of a web page's "PageRank", an objective measure of its citation importance that corresponds well with people's subjective idea of importance. Because of this correspondence, PageRank is an excellent way to prioritize the results of web keyword searches. For most popular subjects, a simple text matching search that is restricted to web page titles performs admirably when PageRank prioritizes the results (demo available at google.stanford.edu). For the type of full text searches in the main Google system, PageRank also helps a great deal.
2.1.1 Description of PageRank Calculation
Academic citation literature has been applied to the web, largely by counting citations or backlinks to a given page. This gives some approximation of a page's importance or quality. PageRank extends this idea by not counting links from all pages equally, and by normalizing by the number of links on a page. PageRank is defined as follows:We assume page A has pages T1...Tn which point to it (i.e., are citations). The parameter d is a damping factor which can be set between 0 and 1. We usually set d to 0.85. There are more details about d in the next section. Also C(A) is defined as the number of links going out of page A. The PageRank of a page A is given as follows:PageRank or PR(A) can be calculated using a simple iterative algorithm, and corresponds to the principal eigenvector of the normalized link matrix of the web. Also, a PageRank for 26 million web pages can be computed in a few hours on a medium size workstation. There are many other details which are beyond the scope of this paper.PR(A) = (1-d) + d (PR(T1)/C(T1) + ... + PR(Tn)/C(Tn))
Note that the PageRanks form a probability distribution over web pages, so the sum of all web pages' PageRanks will be one.
2.1.2 Intuitive Justification
PageRank can be thought of as a model of user behavior. We assume there is a "random surfer" who is given a web page at random and keeps clicking on links, never hitting "back" but eventually gets bored and starts on another random page. The probability that the random surfer visits a page is its PageRank. And, the d damping factor is the probability at each page the "random surfer" will get bored and request another random page. One important variation is to only add the damping factor d to a single page, or a group of pages. This allows for personalization and can make it nearly impossible to deliberately mislead the system in order to get a higher ranking. We have several other extensions to PageRank, again see [Page 98].Another intuitive justification is that a page can have a high PageRank if there are many pages that point to it, or if there are some pages that point to it and have a high PageRank. Intuitively, pages that are well cited from many places around the web are worth looking at. Also, pages that have perhaps only one citation from something like the Yahoo! homepage are also generally worth looking at. If a page was not high quality, or was a broken link, it is quite likely that Yahoo's homepage would not link to it. PageRank handles both these cases and everything in between by recursively propagating weights through the link structure of the web.
2.2 Anchor Text
The text of links is treated in a special way in our search engine. Most search engines associate the text of a link with the page that the link is on. In addition, we associate it with the page the link points to. This has several advantages. First, anchors often provide more accurate descriptions of web pages than the pages themselves. Second, anchors may exist for documents which cannot be indexed by a text-based search engine, such as images, programs, and databases. This makes it possible to return web pages which have not actually been crawled. Note that pages that have not been crawled can cause problems, since they are never checked for validity before being returned to the user. In this case, the search engine can even return a page that never actually existed, but had hyperlinks pointing to it. However, it is possible to sort the results, so that this particular problem rarely happens.
This idea of propagating anchor text to the page it refers to was implemented in the World Wide Web Worm [McBryan 94] especially because it helps search non-text information, and expands the search coverage with fewer downloaded documents. We use anchor propagation mostly because anchor text can help provide better quality results. Using anchor text efficiently is technically difficult because of the large amounts of data which must be processed. In our current crawl of 24 million pages, we had over 259 million anchors which we indexed.
2.3 Other Features
Aside from PageRank and the use of anchor text, Google has several other features. First, it has location information for all hits and so it makes extensive use of proximity in search. Second, Google keeps track of some visual presentation details such as font size of words. Words in a larger or bolder font are weighted higher than other words. Third, full raw HTML of pages is available in a repository.3 Related Work
Search research on the web has a short and concise history. The World Wide Web Worm (WWWW) [McBryan 94] was one of the first web search engines. It was subsequently followed by several other academic search engines, many of which are now public companies. Compared to the growth of the Web and the importance of search engines there are precious few documents about recent search engines [Pinkerton 94]. According to Michael Mauldin (chief scientist, Lycos Inc) [Mauldin], "the various services (including Lycos) closely guard the details of these databases". However, there has been a fair amount of work on specific features of search engines. Especially well represented is work which can get results by post-processing the results of existing commercial search engines, or produce small scale "individualized" search engines. Finally, there has been a lot of research on information retrieval systems, especially on well controlled collections. In the next two sections, we discuss some areas where this research needs to be extended to work better on the web.3.1 Information Retrieval
Work in information retrieval systems goes back many years and is well developed [Witten 94]. However, most of the research on information retrieval systems is on small well controlled homogeneous collections such as collections of scientific papers or news stories on a related topic. Indeed, the primary benchmark for information retrieval, the Text Retrieval Conference [TREC 96], uses a fairly small, well controlled collection for their benchmarks. The "Very Large Corpus" benchmark is only 20GB compared to the 147GB from our crawl of 24 million web pages. Things that work well on TREC often do not produce good results on the web. For example, the standard vector space model tries to return the document that most closely approximates the query, given that both query and document are vectors defined by their word occurrence. On the web, this strategy often returns very short documents that are the query plus a few words. For example, we have seen a major search engine return a page containing only "Bill Clinton Sucks" and picture from a "Bill Clinton" query. Some argue that on the web, users should specify more accurately what they want and add more words to their query. We disagree vehemently with this position. If a user issues a query like "Bill Clinton" they should get reasonable results since there is a enormous amount of high quality information available on this topic. Given examples like these, we believe that the standard information retrieval work needs to be extended to deal effectively with the web.3.2 Differences Between the Web and Well Controlled Collections
The web is a vast collection of completely uncontrolled heterogeneous documents. Documents on the web have extreme variation internal to the documents, and also in the external meta information that might be available. For example, documents differ internally in their language (both human and programming), vocabulary (email addresses, links, zip codes, phone numbers, product numbers), type or format (text, HTML, PDF, images, sounds), and may even be machine generated (log files or output from a database). On the other hand, we define external meta information as information that can be inferred about a document, but is not contained within it. Examples of external meta information include things like reputation of the source, update frequency, quality, popularity or usage, and citations. Not only are the possible sources of external meta information varied, but the things that are being measured vary many orders of magnitude as well. For example, compare the usage information from a major homepage, like Yahoo's which currently receives millions of page views every day with an obscure historical article which might receive one view every ten years. Clearly, these two items must be treated very differently by a search engine.Another big difference between the web and traditional well controlled collections is that there is virtually no control over what people can put on the web. Couple this flexibility to publish anything with the enormous influence of search engines to route traffic and companies which deliberately manipulating search engines for profit become a serious problem. This problem that has not been addressed in traditional closed information retrieval systems. Also, it is interesting to note that metadata efforts have largely failed with web search engines, because any text on the page which is not directly represented to the user is abused to manipulate search engines. There are even numerous companies which specialize in manipulating search engines for profit.
4 System Anatomy
First, we will provide a high level discussion of the architecture. Then, there is some in-depth descriptions of important data structures. Finally, the major applications: crawling, indexing, and searching will be examined in depth. ![]()
|
4.1 Google Architecture Overview
In this section, we will give a high level overview of how the whole system works as pictured in Figure 1. Further sections will discuss the applications and data structures not mentioned in this section. Most of Google is implemented in C or C++ for efficiency and can run in either Solaris or Linux.In Google, the web crawling (downloading of web pages) is done by several distributed crawlers. There is a URLserver that sends lists of URLs to be fetched to the crawlers. The web pages that are fetched are then sent to the storeserver. The storeserver then compresses and stores the web pages into a repository. Every web page has an associated ID number called a docID which is assigned whenever a new URL is parsed out of a web page. The indexing function is performed by the indexer and the sorter. The indexer performs a number of functions. It reads the repository, uncompresses the documents, and parses them. Each document is converted into a set of word occurrences called hits. The hits record the word, position in document, an approximation of font size, and capitalization. The indexer distributes these hits into a set of "barrels", creating a partially sorted forward index. The indexer performs another important function. It parses out all the links in every web page and stores important information about them in an anchors file. This file contains enough information to determine where each link points from and to, and the text of the link.
The URLresolver reads the anchors file and converts relative URLs into absolute URLs and in turn into docIDs. It puts the anchor text into the forward index, associated with the docID that the anchor points to. It also generates a database of links which are pairs of docIDs. The links database is used to compute PageRanks for all the documents.
The sorter takes the barrels, which are sorted by docID (this is a simplification, see Section 4.2.5), and resorts them by wordID to generate the inverted index. This is done in place so that little temporary space is needed for this operation. The sorter also produces a list of wordIDs and offsets into the inverted index. A program called DumpLexicon takes this list together with the lexicon produced by the indexer and generates a new lexicon to be used by the searcher. The searcher is run by a web server and uses the lexicon built by DumpLexicon together with the inverted index and the PageRanks to answer queries.
4.2 Major Data Structures
Google's data structures are optimized so that a large document collection can be crawled, indexed, and searched with little cost. Although, CPUs and bulk input output rates have improved dramatically over the years, a disk seek still requires about 10 ms to complete. Google is designed to avoid disk seeks whenever possible, and this has had a considerable influence on the design of the data structures.
4.2.1 BigFiles
BigFiles are virtual files spanning multiple file systems and are addressable by 64 bit integers. The allocation among multiple file systems is handled automatically. The BigFiles package also handles allocation and deallocation of file descriptors, since the operating systems do not provide enough for our needs. BigFiles also support rudimentary compression options.4.2.2 Repository
![]()
|
4.2.3 Document Index
The document index keeps information about each document. It is a fixed width ISAM (Index sequential access mode) index, ordered by docID. The information stored in each entry includes the current document status, a pointer into the repository, a document checksum, and various statistics. If the document has been crawled, it also contains a pointer into a variable width file called docinfo which contains its URL and title. Otherwise the pointer points into the URLlist which contains just the URL. This design decision was driven by the desire to have a reasonably compact data structure, and the ability to fetch a record in one disk seek during a searchAdditionally, there is a file which is used to convert URLs into docIDs. It is a list of URL checksums with their corresponding docIDs and is sorted by checksum. In order to find the docID of a particular URL, the URL's checksum is computed and a binary search is performed on the checksums file to find its docID. URLs may be converted into docIDs in batch by doing a merge with this file. This is the technique the URLresolver uses to turn URLs into docIDs. This batch mode of update is crucial because otherwise we must perform one seek for every link which assuming one disk would take more than a month for our 322 million link dataset.
4.2.4 Lexicon
The lexicon has several different forms. One important change from earlier systems is that the lexicon can fit in memory for a reasonable price. In the current implementation we can keep the lexicon in memory on a machine with 256 MB of main memory. The current lexicon contains 14 million words (though some rare words were not added to the lexicon). It is implemented in two parts -- a list of the words (concatenated together but separated by nulls) and a hash table of pointers. For various functions, the list of words has some auxiliary information which is beyond the scope of this paper to explain fully.
4.2.5 Hit Lists
A hit list corresponds to a list of occurrences of a particular word in a particular document including position, font, and capitalization information. Hit lists account for most of the space used in both the forward and the inverted indices. Because of this, it is important to represent them as efficiently as possible. We considered several alternatives for encoding position, font, and capitalization -- simple encoding (a triple of integers), a compact encoding (a hand optimized allocation of bits), and Huffman coding. In the end we chose a hand optimized compact encoding since it required far less space than the simple encoding and far less bit manipulation than Huffman coding. The details of the hits are shown in Figure 3.
Our compact encoding uses two bytes for every hit. There are two types of hits: fancy hits and plain hits. Fancy hits include hits occurring in a URL, title, anchor text, or meta tag. Plain hits include everything else. A plain hit consists of a capitalization bit, font size, and 12 bits of word position in a document (all positions higher than 4095 are labeled 4096). Font size is represented relative to the rest of the document using three bits (only 7 values are actually used because 111 is the flag that signals a fancy hit). A fancy hit consists of a capitalization bit, the font size set to 7 to indicate it is a fancy hit, 4 bits to encode the type of fancy hit, and 8 bits of position. For anchor hits, the 8 bits of position are split into 4 bits for position in anchor and 4 bits for a hash of the docID the anchor occurs in. This gives us some limited phrase searching as long as there are not that many anchors for a particular word. We expect to update the way that anchor hits are stored to allow for greater resolution in the position and docIDhash fields. We use font size relative to the rest of the document because when searching, you do not want to rank otherwise identical documents differently just because one of the documents is in a larger font.
![]()
|
The length of a hit list is stored before the hits themselves. To save space, the length of the hit list is combined with the wordID in the forward index and the docID in the inverted index. This limits it to 8 and 5 bits respectively (there are some tricks which allow 8 bits to be borrowed from the wordID). If the length is longer than would fit in that many bits, an escape code is used in those bits, and the next two bytes contain the actual length.
4.2.6 Forward Index
The forward index is actually already partially sorted. It is stored in a number of barrels (we used 64). Each barrel holds a range of wordID's. If a document contains words that fall into a particular barrel, the docID is recorded into the barrel, followed by a list of wordID's with hitlists which correspond to those words. This scheme requires slightly more storage because of duplicated docIDs but the difference is very small for a reasonable number of buckets and saves considerable time and coding complexity in the final indexing phase done by the sorter. Furthermore, instead of storing actual wordID's, we store each wordID as a relative difference from the minimum wordID that falls into the barrel the wordID is in. This way, we can use just 24 bits for the wordID's in the unsorted barrels, leaving 8 bits for the hit list length.4.2.7 Inverted Index
The inverted index consists of the same barrels as the forward index, except that they have been processed by the sorter. For every valid wordID, the lexicon contains a pointer into the barrel that wordID falls into. It points to a doclist of docID's together with their corresponding hit lists. This doclist represents all the occurrences of that word in all documents.An important issue is in what order the docID's should appear in the doclist. One simple solution is to store them sorted by docID. This allows for quick merging of different doclists for multiple word queries. Another option is to store them sorted by a ranking of the occurrence of the word in each document. This makes answering one word queries trivial and makes it likely that the answers to multiple word queries are near the start. However, merging is much more difficult. Also, this makes development much more difficult in that a change to the ranking function requires a rebuild of the index. We chose a compromise between these options, keeping two sets of inverted barrels -- one set for hit lists which include title or anchor hits and another set for all hit lists. This way, we check the first set of barrels first and if there are not enough matches within those barrels we check the larger ones.
4.3 Crawling the Web
Running a web crawler is a challenging task. There are tricky performance and reliability issues and even more importantly, there are social issues. Crawling is the most fragile application since it involves interacting with hundreds of thousands of web servers and various name servers which are all beyond the control of the system.In order to scale to hundreds of millions of web pages, Google has a fast distributed crawling system. A single URLserver serves lists of URLs to a number of crawlers (we typically ran about 3). Both the URLserver and the crawlers are implemented in Python. Each crawler keeps roughly 300 connections open at once. This is necessary to retrieve web pages at a fast enough pace. At peak speeds, the system can crawl over 100 web pages per second using four crawlers. This amounts to roughly 600K per second of data. A major performance stress is DNS lookup. Each crawler maintains a its own DNS cache so it does not need to do a DNS lookup before crawling each document. Each of the hundreds of connections can be in a number of different states: looking up DNS, connecting to host, sending request, and receiving response. These factors make the crawler a complex component of the system. It uses asynchronous IO to manage events, and a number of queues to move page fetches from state to state.
It turns out that running a crawler which connects to more than half a million servers, and generates tens of millions of log entries generates a fair amount of email and phone calls. Because of the vast number of people coming on line, there are always those who do not know what a crawler is, because this is the first one they have seen. Almost daily, we receive an email something like, "Wow, you looked at a lot of pages from my web site. How did you like it?" There are also some people who do not know about the robots exclusion protocol, and think their page should be protected from indexing by a statement like, "This page is copyrighted and should not be indexed", which needless to say is difficult for web crawlers to understand. Also, because of the huge amount of data involved, unexpected things will happen. For example, our system tried to crawl an online game. This resulted in lots of garbage messages in the middle of their game! It turns out this was an easy problem to fix. But this problem had not come up until we had downloaded tens of millions of pages. Because of the immense variation in web pages and servers, it is virtually impossible to test a crawler without running it on large part of the Internet. Invariably, there are hundreds of obscure problems which may only occur on one page out of the whole web and cause the crawler to crash, or worse, cause unpredictable or incorrect behavior. Systems which access large parts of the Internet need to be designed to be very robust and carefully tested. Since large complex systems such as crawlers will invariably cause problems, there needs to be significant resources devoted to reading the email and solving these problems as they come up.
4.4 Indexing the Web
- Parsing -- Any parser which is designed to run on the entire Web must handle a huge array of possible errors. These range from typos in HTML tags to kilobytes of zeros in the middle of a tag, non-ASCII characters, HTML tags nested hundreds deep, and a great variety of other errors that challenge anyone's imagination to come up with equally creative ones. For maximum speed, instead of using YACC to generate a CFG parser, we use flex to generate a lexical analyzer which we outfit with its own stack. Developing this parser which runs at a reasonable speed and is very robust involved a fair amount of work.
- Indexing Documents into Barrels -- After each document is parsed, it is encoded into a number of barrels. Every word is converted into a wordID by using an in-memory hash table -- the lexicon. New additions to the lexicon hash table are logged to a file. Once the words are converted into wordID's, their occurrences in the current document are translated into hit lists and are written into the forward barrels. The main difficulty with parallelization of the indexing phase is that the lexicon needs to be shared. Instead of sharing the lexicon, we took the approach of writing a log of all the extra words that were not in a base lexicon, which we fixed at 14 million words. That way multiple indexers can run in parallel and then the small log file of extra words can be processed by one final indexer.
- Sorting -- In order to generate the inverted index, the sorter takes each of the forward barrels and sorts it by wordID to produce an inverted barrel for title and anchor hits and a full text inverted barrel. This process happens one barrel at a time, thus requiring little temporary storage. Also, we parallelize the sorting phase to use as many machines as we have simply by running multiple sorters, which can process different buckets at the same time. Since the barrels don't fit into main memory, the sorter further subdivides them into baskets which do fit into memory based on wordID and docID. Then the sorter, loads each basket into memory, sorts it and writes its contents into the short inverted barrel and the full inverted barrel.
4.5 Searching
The goal of searching is to provide quality search results efficiently. Many of the large commercial search engines seemed to have made great progress in terms of efficiency. Therefore, we have focused more on quality of search in our research, although we believe our solutions are scalable to commercial volumes with a bit more effort. The google query evaluation process is show in Figure 4.
Sort the documents that have matched by rank and return the top k.
|
To put a limit on response time, once a certain number (currently 40,000) of matching documents are found, the searcher automatically goes to step 8 in Figure 4. This means that it is possible that sub-optimal results would be returned. We are currently investigating other ways to solve this problem. In the past, we sorted the hits according to PageRank, which seemed to improve the situation.
4.5.1 The Ranking System
Google maintains much more information about web documents than typical search engines. Every hitlist includes position, font, and capitalization information. Additionally, we factor in hits from anchor text and the PageRank of the document. Combining all of this information into a rank is difficult. We designed our ranking function so that no particular factor can have too much influence. First, consider the simplest case -- a single word query. In order to rank a document with a single word query, Google looks at that document's hit list for that word. Google considers each hit to be one of several different types (title, anchor, URL, plain text large font, plain text small font, ...), each of which has its own type-weight. The type-weights make up a vector indexed by type. Google counts the number of hits of each type in the hit list. Then every count is converted into a count-weight. Count-weights increase linearly with counts at first but quickly taper off so that more than a certain count will not help. We take the dot product of the vector of count-weights with the vector of type-weights to compute an IR score for the document. Finally, the IR score is combined with PageRank to give a final rank to the document.For a multi-word search, the situation is more complicated. Now multiple hit lists must be scanned through at once so that hits occurring close together in a document are weighted higher than hits occurring far apart. The hits from the multiple hit lists are matched up so that nearby hits are matched together. For every matched set of hits, a proximity is computed. The proximity is based on how far apart the hits are in the document (or anchor) but is classified into 10 different value "bins" ranging from a phrase match to "not even close". Counts are computed not only for every type of hit but for every type and proximity. Every type and proximity pair has a type-prox-weight. The counts are converted into count-weights and we take the dot product of the count-weights and the type-prox-weights to compute an IR score. All of these numbers and matrices can all be displayed with the search results using a special debug mode. These displays have been very helpful in developing the ranking system.
4.5.2 Feedback
The ranking function has many parameters like the type-weights and the type-prox-weights. Figuring out the right values for these parameters is something of a black art. In order to do this, we have a user feedback mechanism in the search engine. A trusted user may optionally evaluate all of the results that are returned. This feedback is saved. Then when we modify the ranking function, we can see the impact of this change on all previous searches which were ranked. Although far from perfect, this gives us some idea of how a change in the ranking function affects the search results.5 Results and Performance
Query: bill clinton http://www.whitehouse.gov/ 100.00% ![]() ![]() http://www.whitehouse.gov/ Office of the President 99.67% ![]() ![]() http://www.whitehouse.gov/WH/EOP/OP/html/OP_Home.html Welcome To The White House 99.98% ![]() ![]() http://www.whitehouse.gov/WH/Welcome.html Send Electronic Mail to the President 99.86% ![]() ![]() http://www.whitehouse.gov/WH/Mail/html/Mail_President.html mailto:president@whitehouse.gov 99.98% ![]() ![]() mailto:President@whitehouse.gov 99.27% ![]() ![]() The "Unofficial" Bill Clinton 94.06% ![]() ![]() http://zpub.com/un/un-bc.html Bill Clinton Meets The Shrinks 86.27% ![]() ![]() http://zpub.com/un/un-bc9.html President Bill Clinton - The Dark Side 97.27% ![]() ![]() http://www.realchange.org/clinton.htm $3 Bill Clinton 94.73% ![]() ![]()
|
All of the results are reasonably high quality pages and, at last check, none were broken links. This is largely because they all have high PageRank. The PageRanks are the percentages in red along with bar graphs. Finally, there are no results about a Bill other than Clinton or about a Clinton other than Bill. This is because we place heavy importance on the proximity of word occurrences. Of course a true test of the quality of a search engine would involve an extensive user study or results analysis which we do not have room for here. Instead, we invite the reader to try Google for themselves at http://google.stanford.edu.
5.1 Storage Requirements
Aside from search quality, Google is designed to scale cost effectively to the size of the Web as it grows. One aspect of this is to use storage efficiently. Table 1 has a breakdown of some statistics and storage requirements of Google. Due to compression the total size of the repository is about 53 GB, just over one third of the total data it stores. At current disk prices this makes the repository a relatively cheap source of useful data. More importantly, the total of all the data used by the search engine requires a comparable amount of storage, about 55 GB. Furthermore, most queries can be answered using just the short inverted index. With better encoding and compression of the Document Index, a high quality web search engine may fit onto a 7GB drive of a new PC.
|
||||||||||||||||||||||
|
||||||||||||||||||||||
|
5.2 System Performance
It is important for a search engine to crawl and index efficiently. This way information can be kept up to date and major changes to the system can be tested relatively quickly. For Google, the major operations are Crawling, Indexing, and Sorting. It is difficult to measure how long crawling took overall because disks filled up, name servers crashed, or any number of other problems which stopped the system. In total it took roughly 9 days to download the 26 million pages (including errors). However, once the system was running smoothly, it ran much faster, downloading the last 11 million pages in just 63 hours, averaging just over 4 million pages per day or 48.5 pages per second. We ran the indexer and the crawler simultaneously. The indexer ran just faster than the crawlers. This is largely because we spent just enough time optimizing the indexer so that it would not be a bottleneck. These optimizations included bulk updates to the document index and placement of critical data structures on the local disk. The indexer runs at roughly 54 pages per second. The sorters can be run completely in parallel; using four machines, the whole process of sorting takes about 24 hours.5.3 Search Performance
Improving the performance of search was not the major focus of our research up to this point. The current version of Google answers most queries in between 1 and 10 seconds. This time is mostly dominated by disk IO over NFS (since disks are spread over a number of machines). Furthermore, Google does not have any optimizations such as query caching, subindices on common terms, and other common optimizations. We intend to speed up Google considerably through distribution and hardware, software, and algorithmic improvements. Our target is to be able to handle several hundred queries per second. Table 2 has some sample query times from the current version of Google. They are repeated to show the speedups resulting from cached IO.
|
||||||||||||||||||||||||||||||
|
6 Conclusions
Google is designed to be a scalable search engine. The primary goal is to provide high quality search results over a rapidly growing World Wide Web. Google employs a number of techniques to improve search quality including page rank, anchor text, and proximity information. Furthermore, Google is a complete architecture for gathering web pages, indexing them, and performing search queries over them.6.1 Future Work
A large-scale web search engine is a complex system and much remains to be done. Our immediate goals are to improve search efficiency and to scale to approximately 100 million web pages. Some simple improvements to efficiency include query caching, smart disk allocation, and subindices. Another area which requires much research is updates. We must have smart algorithms to decide what old web pages should be recrawled and what new ones should be crawled. Work toward this goal has been done in [Cho 98]. One promising area of research is using proxy caches to build search databases, since they are demand driven. We are planning to add simple features supported by commercial search engines like boolean operators, negation, and stemming. However, other features are just starting to be explored such as relevance feedback and clustering (Google currently supports a simple hostname based clustering). We also plan to support user context (like the user's location), and result summarization. We are also working to extend the use of link structure and link text. Simple experiments indicate PageRank can be personalized by increasing the weight of a user's home page or bookmarks. As for link text, we are experimenting with using text surrounding links in addition to the link text itself. A Web search engine is a very rich environment for research ideas. We have far too many to list here so we do not expect this Future Work section to become much shorter in the near future.6.2 High Quality Search
The biggest problem facing users of web search engines today is the quality of the results they get back. While the results are often amusing and expand users' horizons, they are often frustrating and consume precious time. For example, the top result for a search for "Bill Clinton" on one of the most popular commercial search engines was the Bill Clinton Joke of the Day: April 14, 1997. Google is designed to provide higher quality search so as the Web continues to grow rapidly, information can be found easily. In order to accomplish this Google makes heavy use of hypertextual information consisting of link structure and link (anchor) text. Google also uses proximity and font information. While evaluation of a search engine is difficult, we have subjectively found that Google returns higher quality search results than current commercial search engines. The analysis of link structure via PageRank allows Google to evaluate the quality of web pages. The use of link text as a description of what the link points to helps the search engine return relevant (and to some degree high quality) results. Finally, the use of proximity information helps increase relevance a great deal for many queries.6.3 Scalable Architecture
Aside from the quality of search, Google is designed to scale. It must be efficient in both space and time, and constant factors are very important when dealing with the entire Web. In implementing Google, we have seen bottlenecks in CPU, memory access, memory capacity, disk seeks, disk throughput, disk capacity, and network IO. Google has evolved to overcome a number of these bottlenecks during various operations. Google's major data structures make efficient use of available storage space. Furthermore, the crawling, indexing, and sorting operations are efficient enough to be able to build an index of a substantial portion of the web -- 24 million pages, in less than one week. We expect to be able to build an index of 100 million pages in less than a month.6.4 A Research Tool
In addition to being a high quality search engine, Google is a research tool. The data Google has collected has already resulted in many other papers submitted to conferences and many more on the way. Recent research such as [Abiteboul 97] has shown a number of limitations to queries about the Web that may be answered without having the Web available locally. This means that Google (or a similar system) is not only a valuable research tool but a necessary one for a wide range of applications. We hope Google will be a resource for searchers and researchers all around the world and will spark the next generation of search engine technology.7 Acknowledgments
Scott Hassan and Alan Steremberg have been critical to the development of Google. Their talented contributions are irreplaceable, and the authors owe them much gratitude. We would also like to thank Hector Garcia-Molina, Rajeev Motwani, Jeff Ullman, and Terry Winograd and the whole WebBase group for their support and insightful discussions. Finally we would like to recognize the generous support of our equipment donors IBM, Intel, and Sun and our funders. The research described here was conducted as part of the Stanford Integrated Digital Library Project, supported by the National Science Foundation under Cooperative Agreement IRI-9411306. Funding for this cooperative agreement is also provided by DARPA and NASA, and by Interval Research, and the industrial partners of the Stanford Digital Libraries Project.References
- Best of the Web 1994 -- Navigators http://botw.org/1994/awards/navigators.html
- Bill Clinton Joke of the Day: April 14, 1997. http://www.io.com/~cjburke/clinton/970414.html.
- Bzip2 Homepage http://www.muraroa.demon.co.uk/
- Google Search Engine http://google.stanford.edu/
- Harvest http://harvest.transarc.com/
- Mauldin, Michael L. Lycos Design Choices in an Internet Search Service, IEEE Expert Interview http://www.computer.org/pubs/expert/1997/trends/x1008/mauldin.htm
- The Effect of Cellular Phone Use Upon Driver Attention http://www.webfirst.com/aaa/text/cell/cell0toc.htm
- Search Engine Watch http://www.searchenginewatch.com/
- RFC 1950 (zlib) ftp://ftp.uu.net/graphics/png/documents/zlib/zdoc-index.html
- Robots Exclusion Protocol: http://info.webcrawler.com/mak/projects/robots/exclusion.htm
- Web Growth Summary: http://www.mit.edu/people/mkgray/net/web-growth-summary.html
- Yahoo! http://www.yahoo.com/
- [Abiteboul 97] Serge Abiteboul and Victor Vianu, Queries and Computation on the Web. Proceedings of the International Conference on Database Theory. Delphi, Greece 1997.
- [Bagdikian 97] Ben H. Bagdikian. The Media Monopoly. 5th Edition. Publisher: Beacon, ISBN: 0807061557
- [Chakrabarti 98] S.Chakrabarti, B.Dom, D.Gibson, J.Kleinberg, P. Raghavan and S. Rajagopalan. Automatic Resource Compilation by Analyzing Hyperlink Structure and Associated Text. Seventh International Web Conference (WWW 98). Brisbane, Australia, April 14-18, 1998.
- [Cho 98] Junghoo Cho, Hector Garcia-Molina, Lawrence Page. Efficient Crawling Through URL Ordering. Seventh International Web Conference (WWW 98). Brisbane, Australia, April 14-18, 1998.
- [Gravano 94] Luis Gravano, Hector Garcia-Molina, and A. Tomasic. The Effectiveness of GlOSS for the Text-Database Discovery Problem. Proc. of the 1994 ACM SIGMOD International Conference On Management Of Data, 1994.
- [Kleinberg 98] Jon Kleinberg, Authoritative Sources in a Hyperlinked Environment, Proc. ACM-SIAM Symposium on Discrete Algorithms, 1998.
- [Marchiori 97] Massimo Marchiori. The Quest for Correct Information on the Web: Hyper Search Engines. The Sixth International WWW Conference (WWW 97). Santa Clara, USA, April 7-11, 1997.
- [McBryan 94] Oliver A. McBryan. GENVL and WWWW: Tools for Taming the Web. First International Conference on the World Wide Web. CERN, Geneva (Switzerland), May 25-26-27 1994. http://www.cs.colorado.edu/home/mcbryan/mypapers/www94.ps
- [Page 98] Lawrence Page, Sergey Brin, Rajeev Motwani, Terry Winograd. The PageRank Citation Ranking: Bringing Order to the Web. Manuscript in progress. http://google.stanford.edu/~backrub/pageranksub.ps
- [Pinkerton 94] Brian Pinkerton, Finding What People Want: Experiences with the WebCrawler. The Second International WWW Conference Chicago, USA, October 17-20, 1994. http://info.webcrawler.com/bp/WWW94.html
- [Spertus 97] Ellen Spertus. ParaSite: Mining Structural Information on the Web. The Sixth International WWW Conference (WWW 97). Santa Clara, USA, April 7-11, 1997.
- [TREC 96] Proceedings of the fifth Text REtrieval Conference (TREC-5). Gaithersburg, Maryland, November 20-22, 1996. Publisher: Department of Commerce, National Institute of Standards and Technology. Editors: D. K. Harman and E. M. Voorhees. Full text at: http://trec.nist.gov/
- [Witten 94] Ian H Witten, Alistair Moffat, and Timothy C. Bell. Managing Gigabytes: Compressing and Indexing Documents and Images. New York: Van Nostrand Reinhold, 1994.
- [Weiss 96] Ron Weiss, Bienvenido Velez, Mark A. Sheldon, Chanathip Manprempre, Peter Szilagyi, Andrzej Duda, and David K. Gifford. HyPursuit: A Hierarchical Network Search Engine that Exploits Content-Link Hypertext Clustering. Proceedings of the 7th ACM Conference on Hypertext. New York, 1996.
Vitae
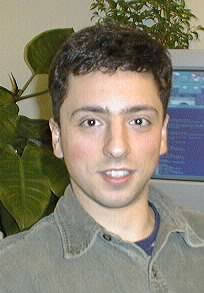
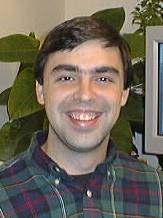
Sergey Brin received his B.S. degree in mathematics and computer science from the University of Maryland at College Park in 1993. Currently, he is a Ph.D. candidate in computer science at Stanford University where he received his M.S. in 1995. He is a recipient of a National Science Foundation Graduate Fellowship. His research interests include search engines, information extraction from unstructured sources, and data mining of large text collections and scientific data.
Lawrence Page was born in East Lansing, Michigan, and received a B.S.E. in Computer Engineering at the University of Michigan Ann Arbor in 1995. He is currently a Ph.D. candidate in Computer Science at Stanford University. Some of his research interests include the link structure of the web, human computer interaction, search engines, scalability of information access interfaces, and personal data mining.
8 Appendix A: Advertising and Mixed Motives
Currently, the predominant business model for commercial search engines is advertising. The goals of the advertising business model do not always correspond to providing quality search to users. For example, in our prototype search engine one of the top results for cellular phone is "The Effect of Cellular Phone Use Upon Driver Attention", a study which explains in great detail the distractions and risk associated with conversing on a cell phone while driving. This search result came up first because of its high importance as judged by the PageRank algorithm, an approximation of citation importance on the web [Page, 98]. It is clear that a search engine which was taking money for showing cellular phone ads would have difficulty justifying the page that our system returned to its paying advertisers. For this type of reason and historical experience with other media [Bagdikian 83], we expect that advertising funded search engines will be inherently biased towards the advertisers and away from the needs of the consumers.
Since it is very difficult even for experts to evaluate search engines, search engine bias is particularly insidious. A good example was OpenText, which was reported to be selling companies the right to be listed at the top of the search results for particular queries [Marchiori 97]. This type of bias is much more insidious than advertising, because it is not clear who "deserves" to be there, and who is willing to pay money to be listed. This business model resulted in an uproar, and OpenText has ceased to be a viable search engine. But less blatant bias are likely to be tolerated by the market. For example, a search engine could add a small factor to search results from "friendly" companies, and subtract a factor from results from competitors. This type of bias is very difficult to detect but could still have a significant effect on the market. Furthermore, advertising income often provides an incentive to provide poor quality search results. For example, we noticed a major search engine would not return a large airline's homepage when the airline's name was given as a query. It so happened that the airline had placed an expensive ad, linked to the query that was its name. A better search engine would not have required this ad, and possibly resulted in the loss of the revenue from the airline to the search engine. In general, it could be argued from the consumer point of view that the better the search engine is, the fewer advertisements will be needed for the consumer to find what they want. This of course erodes the advertising supported business model of the existing search engines. However, there will always be money from advertisers who want a customer to switch products, or have something that is genuinely new. But we believe the issue of advertising causes enough mixed incentives that it is crucial to have a competitive search engine that is transparent and in the academic realm.
9 Appendix B: Scalability
9. 1 Scalability of Google
We have designed Google to be scalable in the near term to a goal of 100 million web pages. We have just received disk and machines to handle roughly that amount. All of the time consuming parts of the system are parallelize and roughly linear time. These include things like the crawlers, indexers, and sorters. We also think that most of the data structures will deal gracefully with the expansion. However, at 100 million web pages we will be very close up against all sorts of operating system limits in the common operating systems (currently we run on both Solaris and Linux). These include things like addressable memory, number of open file descriptors, network sockets and bandwidth, and many others. We believe expanding to a lot more than 100 million pages would greatly increase the complexity of our system.9.2 Scalability of Centralized Indexing Architectures
As the capabilities of computers increase, it becomes possible to index a very large amount of text for a reasonable cost. Of course, other more bandwidth intensive media such as video is likely to become more pervasive. But, because the cost of production of text is low compared to media like video, text is likely to remain very pervasive. Also, it is likely that soon we will have speech recognition that does a reasonable job converting speech into text, expanding the amount of text available. All of this provides amazing possibilities for centralized indexing. Here is an illustrative example. We assume we want to index everything everyone in the US has written for a year. We assume that there are 250 million people in the US and they write an average of 10k per day. That works out to be about 850 terabytes. Also assume that indexing a terabyte can be done now for a reasonable cost. We also assume that the indexing methods used over the text are linear, or nearly linear in their complexity. Given all these assumptions we can compute how long it would take before we could index our 850 terabytes for a reasonable cost assuming certain growth factors. Moore's Law was defined in 1965 as a doubling every 18 months in processor power. It has held remarkably true, not just for processors, but for other important system parameters such as disk as well. If we assume that Moore's law holds for the future, we need only 10 more doublings, or 15 years to reach our goal of indexing everything everyone in the US has written for a year for a price that a small company could afford. Of course, hardware experts are somewhat concerned Moore's Law may not continue to hold for the next 15 years, but there are certainly a lot of interesting centralized applications even if we only get part of the way to our hypothetical example.Of course a distributed systems like Gloss [Gravano 94] or Harvest will often be the most efficient and elegant technical solution for indexing, but it seems difficult to convince the world to use these systems because of the high administration costs of setting up large numbers of installations. Of course, it is quite likely that reducing the administration cost drastically is possible. If that happens, and everyone starts running a distributed indexing system, searching would certainly improve drastically.
Because humans can only type or speak a finite amount, and as computers continue improving, text indexing will scale even better than it does now. Of course there could be an infinite amount of machine generated content, but just indexing huge amounts of human generated content seems tremendously useful. So we are optimistic that our centralized web search engine architecture will improve in its ability to cover the pertinent text information over time and that there is a bright future for search.
【摘要】 这篇文章中,我们介绍了google,它是一个大型的搜索引擎(of a large-scale search engine)的原型,搜索引擎在超文本中应用广泛。Google的设计能够高效地抓网页并建立索引,它的查询结果比其它现有系统都高明。这个原型的全文 和超连接的数据库至少包含24000000个网页。我们可以从http://google.stanford.edu/ 下载。设计搜索引擎是一项富有挑战性的工作。搜索引擎为上亿个网页建立索引,其中包含大量迥然不同的词汇。而且每天要回答成千上万个查询。在网络中,尽管 大型搜索引擎非常重要,但是学术界却很少研究它。此外由于技术的快速发展和网页的大量增加,现在建立一个搜索引擎和三年前完全不同。本文详细介绍了我们的 大型搜索引擎,据我们所知,在公开发表的论文中,这是第一篇描述地如此详细。除了把传统数据搜索技术应用到如此大量级网页中所遇到的问题,还有许多新的技 术挑战,包括应用超文本中的附加信息改进搜索结果。本文将解决这个问题,描述如何运用超文本中的附加信息,建立一个大型实用系统。任何人都可以在网上随意 发布信息,如何有效地处理这些无组织的超文本集合,也是本文要关注的问题。
碧森尤信网
《The Anatomy of a Large-Scale Hypertextual Web Search Engine》
1 绪论
Web 给信息检索带来了新的挑战。Web上的信息量快速增长,同时不断有毫无经验的新用户来体验Web这门艺术。人们喜欢用超级链接来网上冲浪,通常都以象 Yahoo这样重要的网页或搜索引擎开始。大家认为List(目录)有效地包含了大家感兴趣的主题,但是它具有主观性,建立和维护的代价高,升级慢,不能 包括所有深奥的主题。基于关键词的自动搜索引擎通常返回太多的低质量的匹配。使问题更遭的是,一些广告为了赢得人们的关注想方设法误导自动搜索引擎。
我们建立了一个大型搜索引擎解决了现有系统中的很多问题。应用超文本结构,大大提高了查询质量。我们的系统命名为google,取名自googol的通俗拼法,即10的100次方,这和我们的目标建立一个大型搜索引擎不谋而合。
1.1网络搜索引擎—升级换代(scaling up):
1994-2000 搜索引擎技术不得不快速升级(scale dramatically)跟上成倍增长的web数量。1994年,第一个Web搜索引擎,World Wide Web Worm(WWWW)可以检索到110,000个网页和Web的文件。到1994年11月,顶级的搜索引擎声称可以检索到2‘000’000 (WebCrawler)至100‘000’000个网络文件(来自 Search Engine Watch)。可以预见到2000年,可检索到的网页将超过1‘000’000‘000。同时,搜索引擎的访问量也会以惊人的速度增长。在1997年的三 四月份,World Wide Web Worm 平均每天收到1500个查询。
在1997年11月,Altavista 声称它每天要处理大约20’000’000个查询。随着网络用户的增长,到2000年,自动搜索引擎每天将处理上亿个查询。我们系统的设计目标要解决许多 问题,包括质量和可升级性,引入升级搜索引擎技术(scaling search engine technology),把它升级到如此大量的数据上。
1.2 Google:
跟上 Web的步伐(Scaling with the Web)建立一个能够和当今web规模相适应的搜索引擎会面临许多挑战。抓网页技术必须足够快,才能跟上网页变化的速度(keep them up to date)。存储索引和文档的空间必须足够大。索引系统必须能够有效地处理上千亿的数据。处理查询必须快,达到每秒能处理成百上千个查询 (hundreds to thousands per second.)。随着Web的不断增长,这些任务变得越来越艰巨。然而硬件的执行效率和成本也在快速增长,可以部分抵消这些困难。
还有几个值得注意的因素,如磁盘的寻道时间(disk seek time),操作系统的效率(operating system robustness)。在设计Google的过程中,我们既考虑了Web的增长速度,又考虑了技术的更新。Google的设计能够很好的升级处理海量数 据集。它能够有效地利用存储空间来存储索引。优化的数据结构能够快速有效地存取(参考4.2节)。进一步,我们希望,相对于所抓取的文本文件和HTML网 页的数量而言,存储和建立索引的代价尽可能的小(参考附录B)。对于象Google这样的集中式系统,采取这些措施得到了令人满意的系统可升级性 (scaling properties)。
1. 3设计目标
1.3.1 提高搜索质量。我们的主要目标是提高Web搜索引擎的质量。
1994 年,有人认为建立全搜索索引(a complete search index)可以使查找任何数据都变得容易。根据Best of the Web 1994 -- Navigators ,“最好的导航服务可以使在Web上搜索任何信息都很容易(当时所有的数据都可以被登录)”。然而1997年的Web就迥然不同。近来搜索引擎的用户已经 证实索引的完整性不是评价搜索质量的唯一标准。用户感兴趣的搜索结果往往湮没在“垃圾结果Junk result”中。实际上,到1997年11月为止,四大商业搜索引擎中只有一个能够找到它自己(搜索自己名字时返回的前十个结果中有它自己)。导致这一 问题的主要原因是文档的索引数目增加了好几个数量级,但是用户能够看的文档数却没有增加。用户仍然只希望看前面几十个搜索结果。因此,当集合增大时,我们 就需要工具使结果精确(在返回的前几十个结果中,有关文档的数量)。由于是从成千上万个有点相关的文档中选出几十个,实际上,相关的概念就是指最好的文 档。高精确非常重要,甚至以响应(系统能够返回的有关文档的总数)为代价。令人高兴的是利用超文本链接提供的信息有助于改进搜索和其它应用 。尤其是链接结构和链接文本,为相关性的判断和高质量的过滤提供了大量的信息。Google既利用了链接结构又用到了anchor文本(见2.1和2.2 节)。
1.3.2 搜索引擎的学术研究随着时间的流逝,除了发展迅速,Web越来越商业化。
1993 年,只有1.5%的Web服务是来自.com域名。到1997年,超过了60%。同时,搜索引擎从学术领域走进商业。到现在大多数搜索引擎被公司所有,很 少技公开术细节。这就导致搜索引擎技术很大程度上仍然是暗箱操作,并倾向做广告(见附录A)。Google的主要目标是推动学术领域在此方面的发展,和对 它的了解。另一个设计目标是给大家一个实用的系统。应用对我们来说非常重要,因为现代网络系统中存在大量的有用数据(us because we think some of the most interesting research will involve leveraging the vast amount of usage data that is available from modern web systems)。例如,每天有几千万个研究。然而,得到这些数据却非常困难,主要因为它们没有商业价值。我们最后的设计目标是建立一个体系结构能够支持 新的关于海量Web数据的研究。为了支持新研究,Google以压缩的形式保存了实际所抓到的文档。设计Google 的目标之一就是要建立一个环境使其他研究者能够很快进入这个领域,处理海量Web数据,得到满意的结果,而通过其它方法却很难得到结果。系统在短时间内被 建立起来,已经有几篇论文用到了Google建的数据库,更多的在起步中。我们的另一个目标是建立一个宇宙空间实验室似的环境,在这里研究者甚至学生都可 以对我们的海量Web数据设计或做一些实验。
2. 系统特点
Google搜索引擎有两个重要特点,有助于得到高精度的搜索结果。
第一点,应用Web的链接结构计算每个网页的Rank值,称为PageRank,将在98页详细描述它。
第二点,Google利用超链接改进搜索结果。
2.1 PageRank:给网页排序:
Web 的引用(链接)图是重要的资源,却被当今的搜索引擎很大程度上忽视了。我们建立了一个包含518000000个超链接的图,它是一个具有重要意义的样本。 这些图能够快速地计算网页的PageRank值,它是一个客观的标准,较好的符合人们心目中对一个网页重要程度的评价,建立的基础是通过引用判断重要性。 因此在web中,PageRank能够优化关键词查询的结果。对于大多数的主题,在网页标题查询中用PageRank优化简单文本匹配,我们得到了令人惊 叹的结果(从google.stanford.edu可以得到演示)。对于Google主系统中的全文搜索,PageRank也帮了不少忙。
2.1.1计算PageRank
文献检索中的引用理论用到Web中,引用网页的链接数,一定程度上反映了该网页的重要性和质量。PageRank发展了这种思想,网页间的链接是不平等的。
PageRank定义如下: 我们假设T1…Tn指向网页A(例如,被引用)。参数d是制动因子,使结果在0,1之间。通常d等于0.85。在下一节将详细介绍d。C(A)定义为网页A指向其它网页的链接数,
网页A的PageRank值由下式给出: PR(A) = (1-d) + d (PR(T1)/C(T1) + ... + PR(Tn)/C(Tn))
注意PageRank的形式,分布到各个网页中,因此所有网页的PageRank和是1。 PageRank或PR(A)可以用简单的迭代算法计算,相应规格化Web链接矩阵的主特征向量。中等规模的网站计算26‘000’000网页的 PageRank值要花费几小时。还有一些技术细节超出了本文论述的范围。
2.1.2 直觉判断 PageRank被看作用户行为的模型。
我们假设网上冲浪是随机的,不断点击链接,从不返回,最终烦了,另外随机选一个网页重新开始冲浪。随机访问一个网页的可能性就是它的PageRank值。 制动因子d是随机访问一个网页烦了的可能性,随机另选一个网页。对单个网页或一组网页,一个重要的变量加入到制动因子d中。这允许个人可以故意地误导系 统,以得到较高的PageRank值。我们还有其它的PageRank算法,见98页。
另外的直觉判断是一个网页有很多网页指向它,或者一些PageRank值高的网页指向它,则这个网页很重要。直觉地,在Web中,一个网页被很多网页引 用,那么这个网页值得一看。一个网页被象Yahoo这样重要的主页引用即使一次,也值得一看。如果一个网页的质量不高,或者是死链接,象Yahoo这样的 主页不会链向它。PageRank处理了这两方面因素,并通过网络链接递归地传递。
2.2链接描述文字(Anchor Text):
我们的搜索引擎对链接文本进行了特殊的处理。大多数搜索引擎把链接文字和它所链向的网页(the page that the link is on)联系起来。另外,把它和链接所指向的网页联系起来。这有几点好处。
第一,通常链接描述文字比网页本身更精确地描述该网页。
第二,链接描述文字可能链向的文档不能被文本搜索引擎检索到,例如图像,程序和数据库。有可能使返回的网页不能被抓到。注意哪些抓不到的网页将会带来一些 问题。在返回给用户前检测不了它们的有效性。这种情况搜索引擎可能返回一个根本不存在的网页,但是有超级链接指向它。然而这种结果可以被挑出来的,所以此 类的问题很少发生。链接描述文字是对被链向网页的宣传,这个思想被用在World Wide Web Worm 中,主要因为它有助于搜索非文本信息,能够用少量的已下载文档扩大搜索范围。我们大量应用链接描述文字,因为它有助于提高搜索结果的质量。有效地利用链接 描述文字技术上存在一些困难,因为必须处理大量的数据。现在我们能抓到24000000个网页,已经检索到259000000多个链接描述文字。
2.3其它特点除了PageRank和应用链接描述文字外,Google还有一些其它特点。
第一,所有hit都有位置信息,所以它可以在搜索中广泛应用邻近性(proximity)。
第二,Google跟踪一些可视化外表细节,例如字号。黑体大号字比其它文字更重要。
第三,知识库存储了原始的全文html网页。
3 有关工作
Web 检索研究的历史简短。World Wide Web Worm()是最早的搜索引擎之一。后来出现了一些用于学术研究的搜索引擎,现在它们中的大多数被上市公司拥有。与Web的增长和搜索引擎的重要性相比, 有关当今搜索引擎技术的优秀论文相当少。根据Michael Mauldin(Lycos Inc的首席科学家)) ,“各种各样的服务(包括Lycos)非常关注这些数据库的细节。”虽然在搜索引擎的某些特点上做了大量工作。具有代表性的工作有,对现有商业搜索引擎的 结果进行传递,或建立小型的个性化的搜索引擎。最后有关信息检索系统的研究很多,尤其在有组织机构集合(well controlled collections)方面。在下面两节,我们将讨论在信息检索系统中的哪些领域需要改进以便更好的工作在Web上。
3.1信息检索信息检索系统诞生在几年前,并发展迅速。
然而大多数信息检索系统研究的对象是小规模的单一的有组织结构的集合,例如科学论文集,或相关主题的新闻故事。实际上,信息检索的主要基准,(the Text Retrieval Conference),用小规模的、有组织结构的集合作为它们的基准。
大型文集基准只有20GB,相比之下,我们抓到的24000000个网页占147GB。在TREC上工作良好的系统,在Web上却不一定产生好的结果。例 如,标准向量空间模型企图返回和查询请求最相近的文档,把查询请求和文档都看作由出现在它们中的词汇组成的向量。在Web环境下,这种策略常常返回非常短 的文档,这些文档往往是查询词再加几个字。例如,查询“Bill Clinton”,返回的网页只包含“Bill Clinton Sucks”,这是我们从一个主要搜索引擎中看到的。网络上有些争议,用户应该更准确地表达他们想查询什么,在他们的查询请求中用更多的词。我们强烈反对 这种观点。如果用户提出象“Bill Clinton”这样的查询请求,应该得到理想的查询结果,因为这个主题有许多高质量的信息。象所给的例子,我们认为信息检索标准需要发展,以便有效地处 理Web数据。
3.2 有组织结构的集合(Well Controlled Collections)与Web的不同点
Web是完全无组织的异构的大量文档的集合。Web 中的文档无论内在信息还是隐含信息都存在大量的异构性。例如,文档内部就用了不同的语言(既有人类语言又有程序),词汇(email地址,链接,邮政编 码,电话号码,产品号),类型(文本,HTML,PDF,图像,声音),有些甚至是机器创建的文件(log文件,或数据库的输出)。可以从文档中推断出 来,但并不包含在文档中的信息称为隐含信息。隐含信息包括来源的信誉,更新频率,质量,访问量和引用。不但隐含信息的可能来源各种各样,而且被检测的信息 也大不相同,相差可达好几个数量级。例如,一个重要主页的使用量,象Yahoo 每天浏览数达到上百万次,于此相比无名的历史文章可能十年才被访问一次。很明显,搜索引擎对这两类信息的处理是不同的。 Web与有组织结构集合之间的另外一个明显区别是,事实上,向Web上传信息没有任何限制。灵活利用这点可以发布任何对搜索引擎影响重大的信息,使路由阻 塞,加上为牟利故意操纵搜索引擎,这些已经成为一个严重的问题。这些问题还没有被传统的封闭的信息检索系统所提出来。它关心的是元数据的努力,这在Web 搜索引擎中却不适用,因为网页中的任何文本都不会向用户声称企图操纵搜索引擎。甚至有些公司为牟利专门操纵搜索引擎。
4 系统分析(System Anatomy)
首先,我们提供高水平的有关体系结构的讨论。然后,详细描述重要的数据结构。最后,主要应用:抓网页,索引,搜索将被严格地检查。 Figure 1. High Level Google Architecture
4.1Google体系结构概述
这一节,我们将看看整个系统是如何工作的(give a high level),见图1。本节不讨论应用和数据结构,在后几节中讨论。为了效率大部分Google是用c或c++实现的,既可以在Solaris也可以在Linux上运行。
Google 系统中,抓网页(下载网页)是由几个分布式crawlers完成的。一个URL服务器负责向crawlers提供URL列表。抓来的网页交给存储服务器 storeserver。然后,由存储服务器压缩网页并把它们存到知识库repository中。每个网页都有一个ID,称作docID,当新URL从网 页中分析出时,就被分配一个docID。由索引器和排序器负责建立索引index function。索引器从知识库中读取文档,对其解压缩和分析。每个文档被转换成一组词的出现情况,称作命中hits。Hits 纪录了词,词在文档中的位置,最接近的字号,大小写。索引器把这些hits分配到一组桶barrel中,产生经过部分排序后的索引。索引器的另一个重要功 能是分析网页中所有的链接,将有关的重要信息存在链接描述anchors文件中。该文件包含了足够的信息,可以用来判断每个链接链出链入节点的信息,和链 接文本。 URL分解器resolver阅读链接描述anchors文件,并把相对URL转换成绝对URL,再转换成docID。为链接描述文本编制索引,并与它所 指向的docID关联起来。同时建立由docID对组成的链接数据库。用于计算所有文档的PageRank值。用docID分类后的barrels,送给 排序器sorter,再根据wordID进行分类,建立反向索引inverted index。这个操作要恰到好处,以便几乎不需要暂存空间。排序器还给出docID和偏移量列表,建立反向索引。一个叫DumpLexicon的程序把这 个列表和由索引器产生的字典结合在一起,建立一个新的字典,供搜索器使用。这个搜索器就是利用一个Web服务器,使用由DumpLexicon所生成的字 典,利用上述反向索引以及页面等级PageRank来回答用户的提问。
4.2 主要数据结构
经过优化的Google数据结构,能够用较小的代价抓取大量文档,建立索引和查询。虽然近几年CPU和输入输出速率迅速提高。磁盘寻道仍然需要10ms。任何时候Google系统的设计都尽可能地避免磁盘寻道。这对数据结构的设计影响很大。
4.2.1 大文件
大文件BigFiles是指虚拟文件生成的多文件系统,用长度是64位的整型数据寻址。多文件系统之间的空间分配是自动完成的。BigFiles包也处理已分配和未分配文件描述符。由于操纵系统不能满足我们的需要,BigFiles也支持基本的压缩选项。
4.2.2 知识库
Repository Data Structure 知识库包含每个网页的全部HTML。每个网页用zlib(见RFC1950)压缩。压缩技术的选择既要考虑速度又要考虑压缩率。我们选择zlib的速度而 不是压缩率很高的bzip。知识库用bzip的压缩率接近4:1。而用zlib的压缩率是3:1。文档一个挨着一个的存储在知识库中,前缀是docID, 长度,URL,见图2。访问知识库不需要其它的数据结构。这有助于数据一致性和升级。用其它数据结构重构系统,我们只需要修改知识库和crawler错误 列表文件。
4.2.3 文件索引
文件索引保存了有关文档的一些信息。索引以docID的顺序排列,定宽ISAM(Index sequential access mode)。每条记录包括当前文件状态,一个指向知识库的指针,文件校验和,各种统计表。如果一个文档已经被抓到,指针指向docinfo文件,该文件的 宽度可变,包含了URL和标题。否则指针指向包含这个URL的URL列表。这种设计考虑到简洁的数据结构,以及在查询中只需要一个磁盘寻道时间就能够访问 一条记录。还有一个文件用于把URL转换成docID。它是URL校验和与相应docID的列表,按校验和排序。要想知道某个URL的docID,需要计 算URL的校验和,然后在校验和文件中执行二进制查找,找到它的docID。通过对这个文件进行合并,可以把一批URL转换成对应的docID。URL分 析器用这项技术把URL转换成docID。这种成批更新的模式是至关重要的,否则每个链接都需要一次查询,假如用一块磁盘,322‘000’000个链接 的数据集合将花费一个多月的时间。
4.2.4 词典
词典有几种不同的形式。和以前系统的重要不同是,词典对内存的要求可以在合理的价格内。现在实现的系统,一台256M内存的机器就可以把词典装入到内存 中。现在的词典包含14000000词汇(虽然一些很少用的词汇没有加入到词典中)。它执行分两部分—词汇表(用null分隔的连续串)和指针的哈希表。 不同的函数,词汇表有一些辅助信息,这超出了本文论述的范围。
4.2.5 hit list
hit list是一篇文档中所出现的词的列表,包括位置,字号,大小写。Hit list占很大空间,用在正向和反向索引中。因此,它的表示形式越有效越好。我们考虑了几种方案来编码位置,字号,大小写—简单编码(3个整型数),紧凑 编码(支持优化分配比特位),哈夫曼编码。Hit的详细信息见图3。我们的紧凑编码每个hit用2字节。有两种类型hit,特殊hit和普通hit。特殊 hit包含URL,标题,链接描述文字,meta tag。普通hit包含其它每件事。它包括大小写特征位,字号,12比特用于描述词在文档中的位置(所有超过4095的位置标记为4096)。字号采用相 对于文档的其它部分的相对大小表示,占3比特(实际只用7个值,因为111标志是特殊hit)。特殊hit由大小写特征位,字号位为7表示它是特殊 hit,用4比特表示特殊hit的类型,8比特表示位置。对于anchor hit八比特位置位分出4比特用来表示在anchor中的位置,4比特用于表明anchor出现的哈希表hash of the docID。短语查询是有限的,对某些词没有足够多的anchor。我们希望更新anchor hit的存储方式,以便解决地址位和docIDhash域位数不足的问题。
因为搜索时,你不会因为文档的字号比别的文档大而特殊对待它,所以采用相对字号。 hit表的长度存储在hit前。为节省空间hit表长度,在正向索引中和wordID结合在一起,在反向索引中和docID结合存储。这就限制它相应地只 占8到5比特(用些技巧,可以从wordID中借8bit)如果大于这些比特所能表示的长度,用溢出码填充,其后两字节是真正的长度。 Figure 3. Forward and Reverse Indexes and the Lexicon
4.2.6 正向索引
实际上,正向索引已经部分排序。它被存在一定数量的barrel中(我们用64个barrels)。每个barrel装着一定范围的wordID。如果一 篇文档中的词落到某个barrel,它的docID将被记录到这个barrel中,紧跟着那些词(文档中所有的词汇,还是落入该barrel中的词汇)对 应的hitlist。这种模式需要稍多些的存储空间,因为一个docID被用多次,但是它节省了桶数和时间,最后排序器进行索引时降低编码的复杂度。更进 一步的措施是,我们不是存储docID本身,而是存储相对于该桶最小的docID的差。用这种方法,未排序的barrel的docID只需24 位,省下8位记录hitlist长。
4.2.7 反向索引
除了反向索引由sorter加工处理之外,它和正向索引包含相同的桶。对每个有效的docID,字典包含一个指向该词所在桶的指针。它指向由docID和 它的相应hitlist组成的doclish,这个doclist代表了所有包含该词的文档。 doclist中docID的顺序是一个重要的问题。最简单的解决办法是用doclish排序。这种方法合并多个词时很快。另一个可选方案是用文档中该词 出现的次数排序。这种方法回答单词查询,所用时间微不足道。当多词查询时几乎是从头开始。并且当用其它Rank算法改进索引时,非常困难。我们综合了这两 种方法,建立两组反向索引barrel,一组barrels的hitlist只包含标题和anchor hit,另一组barrel包含全部的hitlist。我们首先查第一组索引桶,看有没有匹配的项,然后查较大的那组桶。
4.3 抓网页运行
网络爬行机器人是一项具有挑战性的任务。执行的性能和可靠性甚至更重要,还有一些社会焦点。网络爬行是一项非常薄弱的应用,它需要成百上千的web服务器 和各种域名服务器的参与,这些服务器不是我们系统所能控制的。为了覆盖几十亿的网页,Google拥有快速的分布式网络爬行系统。一个URL服务器给若干 个网络爬行机器人(我们采用3个)提供URL列表。URL服务器和网络爬行机器人都是用Python实现的。每个网络爬行机器人可以同时打开300个链 接。抓取网页必须足够快。最快时,用4个网络爬行机器人每秒可以爬行100个网页。速率达每秒600K。执行的重点是找 DNS。每个网络爬行机器人有它自己的DNS cache,所以它不必每个网页都查DNS。每一百个连接都有几种不同的状态:查DNS,连接主机,发送请求,接收回答。这些因素使网络爬行机器人成为系 统比较复杂的部分。它用异步IO处理事件,若干请求队列从一个网站到另一个网站不停的抓取网页。运行一个链接到500多万台服务器的网页爬行机器人,产生 1千多万登陆口,导致了大量的Email和电话。因为网民众多,总有些人不知道网络爬行机器人是何物,这是他们看到的第一个网络爬行机器人。几乎每天我们 都会收到这样的Email“哦,你从我们的网站看了太多的网页,你想干什么?”还有一些人不知道网络搜索机器人避免协议(the robots exclusion protocol),以为他们的网页上写着“版权所有,勿被索引”的字样就会被保护不被索引,不必说,这样的话很难被web crawler理解。因为数据量如此之大,还会遇到一些意想不到的事情。例如,我们的系统曾经企图抓一个在线游戏,结果抓到了游戏中的大量垃圾信息。解决 这个问题很简单。但是我们下载了几千万网页后才发现了这个问题。因为网页和服务器的种类繁多,实际上不在大部分Internet上运行它就测试一个网页爬 行机器人是不可能。总是有几百个隐含的问题发生在整个web的一个网页上,导致网络爬行机器人崩溃,或者更糟,导致不可预测的不正确的行为。能够访问大部 分Internet的系统必须精力充沛并精心测试过。由于象crawler这样大型复杂的系统总是产生这样那样的问题,因此花费一些资源读这些 Email,当问题发生时解决它,是有必要的。
本站搜索: WorldWideWeb 搜索引擎 信息检索 PageRank Google
[在Google上搜索相关文章] [在百度上搜索相关文章]
原文链接:http://blog.csdn.net/chief1985/archive/2007/04/30/1593409.aspx 原文链接:http://www.pipcn.com/blog/user1/master/archives/2006/1100.shtml
【郑重声明】 本站所有文章除注有来源网址外均为互联网首发,按照创造共用方式授权,允许相关网站转载,但必须标明作者名称并在明显位置作好原文网址链接(复制以上链接),且不能运用于任何商业目的。
——建筑知识引擎小组
4.4 Web索引分析
任何运行在整个Web上的分析器必须能够处理可能包含错误的大型集合。范围从HTML标记到标记之间几K字节的0,非ASCII字符,几百层HTML标记 的嵌套,各种各样令人难以想象的错误。为了获得最大的速度,我们没有采用YACC产生上下文无关文法CFG分析器,而是采用灵活的方式产生词汇分析器,它 自己配有堆栈。分析器的改进大大提高了运行速度,它的精力如此充沛完成了大量工作。把文档装入barrel建立索引—分析完一篇文档,之后把该文档装入 barrel中,用内存中的hash表—字典,每个词汇被转换成一个 wordID。当hash表字典中加入新的项时,笨拙地存入文件。一旦词汇被转换成wordID,它们在当前文档的出现就转换成hitlist,被写进正 向barrel。索引阶段并行的主要困难是字典需要共享。
我们采用的方法是,基本字典中有140万个固定词汇,不在基本字典中的词汇写入日志,而不是共享字典。这种方法多个索引器可以并行工作,最后一个索引器只 需处理一个较小的额外词汇日志。排序—为了建立反向索引,排序器读取每个正向barrel,以 wordID排序,建立只有标题anchor hi t的反向索引barrel和全文反向索引barrel。这个过程一次只处理一个barrel,所以只需要少量暂存空间。排序阶段也是并行的,我们简单地同 时运行尽可能多的排序器,不同的排序器处理不同的桶。由于barrel不适合装入主存,排序器进一步依据wordID和docID把它分成若干篮子,以便 适合装入主存。然后排序器把每个篮子装入主存进行排序,并把它的内容写回到短反向barrel和全文反向barrel。
4.5搜索
搜索的目标是提供有效的高质量的搜索结果。多数大型商业搜索引擎好像在效率方面花费了很大力气。因此我们的研究以搜索质量为重点,相信我们的解决方案也可以用到那些商业系统中。
Google查询评价过程见图4。
1. 分析查询。
2. 把词汇转换成wordID。
3. 在短barrel中查找每个词汇doclist的开头。
4. 扫描doclist直到找到一篇匹配所有关键词的文档
5. 计算该文档的rank
6. 如果我们在短barrel,并且在所有doclist的末尾,开始从全文barrel的doclist的开头查找每个词,goto 第四步
7. 如果不在任何doclist的结尾,返回第四步。
8. 根据rank排序匹配文档,返回前k个。图4 Google查询评价在有限的响应时间内,一旦找到一定数量的匹配文档,搜索引擎自动执行步骤8。这意味着,返回的结果是子优化的。我们现在研究其它方法 来解决这个问题。过去根据PageRank排序hit,看来能够改进这种状况。
4.5.1 Ranking系统
Google比典型搜索引擎保存了更多的web信息。每个hitlist包括位置,字号,大小写。另外,我们还考虑了链接描述文字。Rank 综合所有这些信息是困难的。ranking函数设计依据是没有某个因素对rank影响重大。首先,考虑最简单的情况—单个词查询。为了单个词查询中一个文 档的rank,Goole在文档的hitlist中查找该词。Google认为每个hit是几种不同类型(标题,链接描述文字anchor,URL,普通 大字号文本,普通小字号文本,……)之一,每种有它自己的类型权重。类型权重建立了一个类型索引向量。Google计算hitlist中每种hit的数 量。然后每个hit数转换成count-weight。Count-weight开始随hit数线性增加,很快逐渐停止,以至于hit数与此不相关。我们 计算count-weight向量和type-weight向量的标量积作为文档的IR值。最后IR值结合PageRank作为文档的最后rank。 对于多词查询,更复杂些。现在,多词hitlist必须同时扫描,以便关键词出现在同一文档中的权重比分别出现时高。相邻词的hit一起匹配。对每个匹配 hit 的集合计算相邻度。相邻度基于hit在文档中的距离,分成10个不同的bin值,范围从短语匹配到根本不相关。不仅计算每类hit数,而且要计算每种类型 的相邻度,每个类型相似度对,有一个类型相邻度权type-prox-weight。Count转换成count-weight,计算count- weight、 type-prox-weight的标量积作为IR值。应用某种debug mode所有这些数和矩阵与查询结果一起显示出来。这些显示有助于改进rank系统。
4.5.2 反馈
rank 函数有很多参数象type-weight和type-prox-weight。指明这些参数的正确值有点黑色艺术black art。为此,我们的搜索引擎有一个用户反馈机制。值得信任的用户可以随意地评价返回的结果。保存反馈。然后,当修改rank函数时,对比以前搜索的 rank,我们可以看到修改带来的的影响。虽然不是十全十美,但是它给出了一些思路,当rank函数改变时对搜索结果的影响。
5 执行和结果
搜索结果的质量是搜索引擎最重要的度量标准。完全用户评价体系超出了本文的论述范围,对于大多数搜索,我们的经验说明Google的搜索结果比那些主要的 商业搜索引擎好。作为一个应用PageRank,链接描述文字,相邻度的例子,图4给出了Google搜索bill Clinton的结果。它说明了Google的一些特点。服务器对结果进行聚类。这对过滤结果集合相当有帮助。这个查询,相当一部分结果来自 whitehouse.gov域,这正是我们所需要的。现在大多数商业搜索引擎不会返回任何来自whitehouse.gov的结果,这是相当不对的。注 意第一个搜索结果没有标题。因为它不是被抓到的。Google是根据链接描述文字决定它是一个好的查询结果。同样地,第五个结果是一个Email地址,当 然是不可能抓到的。也是链接描述文字的结果。所有这些结果质量都很高,最后检查没有死链接。因为它们中的大部分PageRank值较高。PageRank 百分比用红色线条表示。没有结果只含Bill没有Clinton或只含Clinton没有Bill。因为词出现的相近性非常重要。当然搜索引擎质量的真实 测试包含广泛的用户学习或结果分析,此处篇幅有限,请读者自己去体验Google,http://google.stanford.edu/。
5.1存储需求
除了搜索质量,Google的设计可以随着Web规模的增大而有效地增大成本。一方面有效地利用存储空间。表1列出了一些统计数字的明细表和Google 存储的需求。由于压缩技术的应用知识库只需53GB的存储空间。是所有要存储数据的三分之一。按当今磁盘价格,知识库相对于有用的数据来说比较便宜。搜索 引擎需要的所有数据的存储空间大约55GB。大多数查询请求只需要短反向索引。文件索引应用先进的编码和压缩技术,一个高质量的搜索引擎可以运行在7GB 的新PC。
5.2 系统执行
搜索引擎抓网页和建立索引的效率非常重要。Google 的主要操作是抓网页,索引,排序。很难测试抓全部网页需要多少时间,因为磁盘满了,域名服务器崩溃,或者其它问题导致系统停止。总的来说,大约需要9天时 间下载26000000网页(包括错误)。然而,一旦系统运行顺利,速度非常快,下载最后11000000网页只需要63小时,平均每天4000000网 页,每秒48.5个网页。索引器和网络爬行机器人同步运行。索引器比网络爬行机器人快。因为我们花费了大量时间优化索引器,使它不是瓶颈。这些优化包括批 量更新文档索引,本地磁盘数据结构的安排。索引器每秒处理54个网页。排序器完全并行,用4台机器,排序的整个过程大概需要24小时。
5.3搜索执行改进
搜索执行不是我们研究的重点。当前版本的Google可以在1到10秒间回答查询请求。时间大部分花费在NFS磁盘IO上(由于磁盘普遍比机器慢)。进一 步说,Google没有做任何优化,例如查询缓冲区,常用词汇子索引,和其它常用的优化技术。我们倾向于通过分布式,硬件,软件,和算法的改进来提高 Google的速度。我们的目标是每秒能处理几百个请求。表2有几个现在版本Google响应查询时间的例子。它们说明IO缓冲区对再次搜索速度的影响。
6 结论
Google设计成可伸缩的搜索引擎。主要目标是在快速发展的World Wide Web上提供高质量的搜索结果。Google应用了一些技术改进搜索质量包括PageRank,链接描述文字,相邻信息。进一步说,Google是一个收 集网页,建立索引,执行搜索请求的完整的体系结构。
6.1 未来的工作
大型Web搜索引擎是个复杂的系统,还有很多事情要做。我们直接的目标是提高搜索效率,覆盖大约100000000个网页。一些简单的改进提高了效率包括 请求缓冲区,巧妙地分配磁盘空间,子索引。另一个需要研究的领域是更新。我们必须有一个巧妙的算法来决定哪些旧网页需要重新抓取,哪些新网页需要被抓取。 这个目标已经由实现了。受需求驱动,用代理cache创建搜索数据库是一个有前途的研究领域。我们计划加一些简单的已经被商业搜索引擎支持的特征,例如布 尔算术符号,否定,填充。然而另外一些应用刚刚开始探索,例如相关反馈,聚类(Google现在支持简单的基于主机名的聚类)。我们还计划支持用户上下文 (象用户地址),结果摘要。我们正在扩大链接结构和链接文本的应用。简单的实验证明,通过增加用户主页的权重或书签,PageRank可以个性化。对于链 接文本,我们正在试验用链接周围的文本加入到链接文本。Web搜索引擎提供了丰富的研究课题。如此之多以至于我们不能在此一一列举,因此在不久的将来,我 们希望所做的工作不止本节提到的。
6.2 高质量搜索
当今Web搜索引擎用户所面临的最大问题是搜索结果的质量。结果常常是好笑的,并且超出用户的眼界,他们常常灰心丧气浪费了宝贵的时间。例如,一个最流行 的商业搜索引擎搜索“Bill Clillton”的结果是the Bill Clinton Joke of the Day: April 14, 1997。Google的设计目标是随着Web的快速发展提供高质量的搜索结果,容易找到信息。为此,Google大量应用超文本信息包括链接结构和链接 文本。Google还用到了相邻性和字号信息。评价搜索引擎是困难的,我们主观地发现Google的搜索质量比当今商业搜索引擎高。通过PageRank 分析链接结构使Google能够评价网页的质量。用链接文本描述链接所指向的网页有助于搜索引擎返回相关的结果(某种程度上提高了质量)。最后,利用相邻 性信息大大提高了很多搜索的相关性。
6.3可升级的体系结构
除了搜索质量,Google设计成可升级的。空间和时间必须高效,处理整个Web时固定的几个因素非常重要。实现Google系统,CPU、访存、内存容 量、磁盘寻道时间、磁盘吞吐量、磁盘容量、网络IO都是瓶颈。在一些操作中,已经改进的Google克服了一些瓶颈。Google的主要数据结构能够有效 利用存储空间。进一步,网页爬行,索引,排序已经足够建立大部分web索引,共24000000个网页,用时不到一星期。我们希望能在一个月内建立 100000000网页的索引。
6.4 研究工具
Google不仅是高质量的搜索引擎,它还是研究工具。Google搜集的数据已经用在许多其它论文中,提交给学术会议和许多其它方式。最近的研究,例 如,提出了Web查询的局限性,不需要网络就可以回答。这说明Google不仅是重要的研究工具,而且必不可少,应用广泛。我们希望Google是全世界 研究者的资源,带动搜索引擎技术的更新换代。
7、致谢
Scott Hassan and Alan Steremberg评价了Google的改进。他们的才智无可替代,作者由衷地感谢他们。感谢Hector Garcia-Molina, Rajeev Motwani, Jeff Ullman, and Terry Winograd和全部WebBase开发组的支持和富有深刻见解的讨论。最后感谢IBM,Intel,Sun和投资者的慷慨支持,为我们提供设备。这里 所描述的研究是Stanford综合数字图书馆计划的一部分,由国家科学自然基金支持,合作协议号IRI-9411306。DARPA ,NASA,Interva研究,Stanford数字图书馆计划的工业合作伙伴也为这项合作协议提供了资金。
参考文献。
Best of the Web 1994 -- Navigators http://botw.org/1994/awards/navigators.html
Bill Clinton Joke of the Day: April 14, 1997. http://www.io.com/~cjburke/clinton/970414.html.
Bzip2 Homepage http://www.muraroa.demon.co.uk/
Google Search Engine http://google.stanford.edu/
Harvest http://harvest.transarc.com/
Mauldin, Michael L. Lycos Design Choices in an Internet Search Service, IEEE Expert Interview http://www.computer.org/pubs/expert/1997/trends/x1008/mauldin.htm
The Effect of Cellular Phone Use Upon Driver Attention http://www.webfirst.com/aaa/text/cell/cell0toc.htm
Search Engine Watch http://www.searchenginewatch.com/
RFC 1950 (zlib) ftp://ftp.uu.net/graphics/png/documents/zlib/zdoc-index.html
Robots Exclusion Protocol: http://info.webcrawler.com/mak/projects/robots/exclusion.htm
Web Growth Summary: http://www.mit.edu/people/mkgray/net/web-growth-summary.html
Yahoo! http://www.yahoo.com/
[Abiteboul 97] Serge Abiteboul and Victor Vianu, Queries and Computation on the Web. Proceedings of the International Conference on Database Theory. Delphi, Greece 1997.
[Bagdikian 97] Ben H. Bagdikian. The Media Monopoly. 5th Edition. Publisher: Beacon, ISBN: 0807061557
[Chakrabarti 98] S.Chakrabarti, B.Dom, D.Gibson, J.Kleinberg, P. Raghavan and S. Rajagopalan. Automatic Resource Compilation by Analyzing Hyperlink Structure and Associated Text. Seventh International Web Conference (WWW 98). Brisbane, Australia, April 14-18, 1998.
[Cho 98] Junghoo Cho, Hector Garcia-Molina, Lawrence Page. Efficient Crawling Through URL Ordering. Seventh International Web Conference (WWW 98). Brisbane, Australia, April 14-18, 1998.
[Gravano 94] Luis Gravano, Hector Garcia-Molina, and A. Tomasic. The Effectiveness of GlOSS for the Text-Database Discovery Problem. Proc. of the 1994 ACM SIGMOD International Conference On Management Of Data, 1994.
[Kleinberg 98] Jon Kleinberg, Authoritative Sources in a Hyperlinked Environment, Proc. ACM-SIAM Symposium on Discrete Algorithms, 1998.
[Marchiori 97] Massimo Marchiori. The Quest for Correct Information on the Web: Hyper Search Engines. The Sixth International WWW Conference (WWW 97). Santa Clara, USA, April 7-11, 1997.
[McBryan 94] Oliver A. McBryan. GENVL and WWWW: Tools for Taming the Web. First International Conference on the World Wide Web. CERN, Geneva (Switzerland), May 25-26-27 1994. http://www.cs.colorado.edu/home/mcbryan/mypapers/www94.ps
[Page 98] Lawrence Page, Sergey Brin, Rajeev Motwani, Terry Winograd. The PageRank Citation Ranking: Bringing Order to the Web. Manuscript in progress. http://google.stanford.edu/~backrub/pageranksub.ps
[Pinkerton 94] Brian Pinkerton, Finding What People Want: Experiences with the WebCrawler. The Second International WWW Conference Chicago, USA, October 17-20, 1994. http://info.webcrawler.com/bp/WWW94.html
[Spertus 97] Ellen Spertus. ParaSite: Mining Structural Information on the Web. The Sixth International WWW Conference (WWW 97). Santa Clara, USA, April 7-11, 1997.
[TREC 96] Proceedings of the fifth Text REtrieval Conference (TREC-5). Gaithersburg, Maryland, November 20-22, 1996. Publisher: Department of Commerce, National Institute of Standards and Technology. Editors: D. K. Harman and E. M. Voorhees. Full text at: http://trec.nist.gov/
[Witten 94] Ian H Witten, Alistair Moffat, and Timothy C. Bell. Managing Gigabytes: Compressing and Indexing Documents and Images. New York: Van Nostrand Reinhold, 1994.
[Weiss 96] Ron Weiss, Bienvenido Velez, Mark A. Sheldon, Chanathip Manprempre, Peter Szilagyi, Andrzej Duda, and David K. Gifford. HyPursuit: A Hierarchical Network Search Engine that Exploits Content-Link Hypertext Clustering. Proceedings of the 7th ACM Conference on Hypertext. New York, 1996.
图片附录:
图1 Google系统的工作流程图
(注:原图来自Sergey Brin and Lawrence Page, The Anatomy of a Large-Scale Hypertextual. Web Search Engine, 1998.http://www-db.stanford.edu/%7Ebackrub/Google.html)
①Google使用高速的分布式爬行器(Crawler)系统中的漫游遍历器(Googlebot)定时地遍历网页,将遍历到的网页送到存储服务器(Store Server)中。
② 存储服务器使用zlib格式压缩软件将这些网页进行无损压缩处理后存入数据库Repository中。Repository获得了每个网页的完全Html 代码后,对其压缩后的网页及URL进行分析,记录下网页长度、URL、URL长度和网页内容,并赋予每个网页一个文档号(docID),以便当系统出现故 障的时候,可以及时完整地进行网页的数据恢复。
③索引器(Indexer)从Repository中读取数据,以后做以下四步工作:
④(a) 将读取的数据解压缩后进行分析,它将网页中每个有意义的词进行统计后,转化为关键词(wordID)的若干索引项(Hits),生成索引项列表,该列表包 括关键词、关键词的位置、关键词的大小和大小写状态等。索引项列表被存入到数据桶(Barrels)中,并生成以文档号(docID)部分排序的顺排档索 引。
索引项根据其重要程度分为两种:当索引项中的关键词出现在URL、标题、锚文本(Anchor Text)和标签中时,表示该索引项比较重要,称为特殊索引项(Fancy Hits);其余情况则称为普通索引项(Plain Hits)。在系统中每个Hit用两个字节(byte)存储结构表示:特殊索引项用1位(bit)表示大小写,用二进制代码111(占3位)表示是特殊索 引项,其余12位有4位表示特殊索引项的类型(即hit是出现在URL、标题、链接结点还是标签中),剩下8位表示hit在网页中的具体位置;普通索引项 是用1位表示大小写,3位表示字体大小,其余12位表示在网页中的具体位置。
顺排档索引和Hit的存储结构如图3所示。
图3 顺排档索引和Hit的存储结构
值得注意的是,当特殊索引项来自Anchor Text时,特殊索引项用来表示位置的信息(8位)将分为两部分:4位表示Anchor Text出现的具体位置,另4位则用来与表示Anchor Text所链接网页的docID相连接,这个docID是由URL Resolver经过转化存入顺排档索引的。
(b)索引器除了对网页中有意义的词进行分析外,还分析网页的所有超文本链接,将其Anchor Text、URL指向等关键信息存入到Anchor文档库中。
(c)索引器生成一个索引词表(Lexicon),它包括两个部分:关键词的列表和指针列表,用于倒排档文档相连接(如图3所示)。
(d) 索引器还将分析过的网页编排成一个与Repository相连接的文档索引(Document Index),并记录下网页的URL和标题,以便可以准确查找出在Repository中存储的原网页内容。而且把没有分析的网页传给URL Server,以便在下一次工作流程中进行索引分析。
⑤URL分析器(URL Resolver)读取Anchor文档中的信息,然后做⑥中的工作。
⑥(a) 将其锚文本(Anchor Text)所指向的URL转换成网页的docID;(b)将该docID与原网页的docID形成“链接对”,存入Link数据库中;(c)将 Anchor Text指向的网页的docID与顺排档特殊索引项Anchor Hits相连接。
⑦数据库Link记录了网页的链接关系,用来计算网页的PageRank值。
⑧文档索引(Document Index)把没有进行索引分析的网页传递给URL Server,URL Server则向Crawler提供待遍历的URL,这样,这些未被索引的网页在下一次工作流程中将被索引分析。
⑨排序器(Sorter)对数据桶(Barrels)的顺排档索引重新进行排序,生成以关键词(wordID)为索引的倒排档索引。倒排档索引结构如图4所示:
图4 倒排档索引结构
⑩ 将生成的倒排档索引与先前由索引器产生的索引词表(Lexicon)相连接产生一个新的索引词表供搜索器(Searcher)使用。搜索器的功能是由网页 服务器实现的,根据新产生的索引词表结合上述的文档索引(Document Index)和Link数据库计算的网页PageRank值来匹配检索。
在执行检索时,Google通常遵循以下步骤(以下所指的是单个检索词的情况):
(1)将检索词转化成相应的wordID;
(2)利用Lexicon,检索出包含该wordID的网页的docID;
(3)根据与Lexicon相连的倒排档索引,分析各网页中的相关索引项的情况,计算各网页和检索词的匹配程度,必要时调用顺排档索引;
(4)根据各网页的匹配程度,结合根据Link产生的相应网页的PageRank情况,对检索结果进行排序;
(5)调用Document Index中的docID及其相应的URL,将排序结果生成检索结果的最终列表,提供给检索用户。
用户检索包含多个检索词的情况与以上单个检索词的情况类似:先做单个检索词的检索,然后根据检索式中检索符号的要求进行必要的布尔操作或其他操作。